- COVID-19 Tracker
- Biochemistry
- Anatomy & Physiology
- Microbiology
- Neuroscience
- Animal Kingdom
- NGSS High School
- Latest News
- Editors’ Picks
- Weekly Digest
- Quotes about Biology
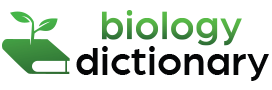
Reviewed by: BD Editors
The term in vivo refers to a type of experiment that is carried out within a whole, living organism , such as a plant or animal.
In vivo means “within the living” in Latin, which aptly fits its modern definition.
In vivo refers to a specific type of experimentation that involves living animals. Living animals are very complex, which provides both benefits and detriments to experimentation. On one hand, in vivo experiments are much more complex than experiments carried out in a test tube – so the results can be less revealing and may inspire more questions instead of providing concrete answers.
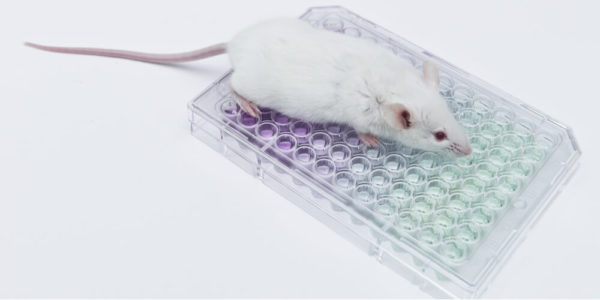
On the other hand, in vivo experiments are a very important part of understanding the complexities of life. Whether it comes to understanding the brain, testing a new drug, or understanding how behavior works, these processes are not possible in a test tube because the level of complexity found in a living animal cannot be replicated easily. While some researchers are working on advanced computer modeling to replace in vivo testing, these models are not yet as robust or complex as a living organism.

In vivo vs. In vitro
So we know that in vivo refers to experiments carried out on a living organism, but what’s the difference between this form of experimentation and in vitro experimentation?
In vitro is pretty much the exact opposite. Instead of complex, living organisms, in vitro experiments refer to techniques that use biological components such as cells or biological molecules and are carried out outside of a living organism , whether that be in a test tube, culture dish, or so on.
Tests using in vitro methods are often used to observe things such as bacterial or animal cells in a more controlled environment , which is one of their main advantages over in vivo testing.
In vitro methods also have other advantages including the fact that they:
- Are usually cheaper
- Can be used for large-scale production
- Reduce the amount of animal testing, which is more ethical
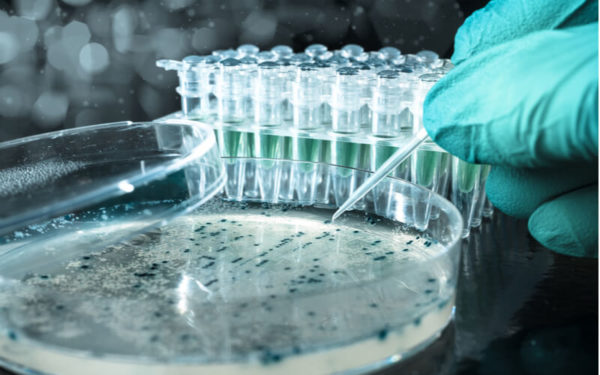
In vivo vs. Ex vivo
The difference between in vivo and ex vivo is simple.
Ex vivo means ‘outside of a living body’ in Latin and refers to methods wherein living tissues are taken directly from a living organism rather than created artificially, and testing is carried out on them with very minimal changes to the tissue’s natural state.
This varies slightly from in vitro , where things such as cells are separated and purified from their natural surroundings and are tested on in very controlled environments.
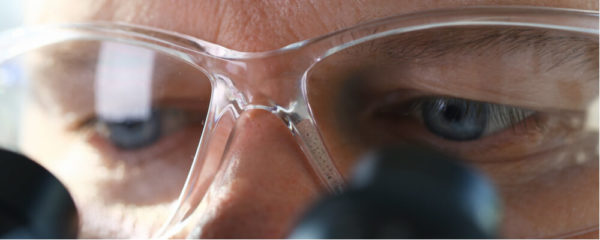
Advantages of in vivo experiments
There are several advantages to using living organisms over in vitro or ex vivo methods. These include:
- Evaluation of the effects of certain substances is more accurate in a complex model
- You can easily view all the side effects that a substance produces in all parts of the body
- The procedure may be easier as fewer variables need to be (or can be) controlled
- They are more clinically relevant
Disadvantages of in vivo experiments
While using in vivo methods in studies has its advantages, every scientific method comes with its own drawbacks. In vivo experimentation is no exception.
Some of the disadvantages of in vivo studies include:
- Whole, living organisms are used, which can prove to be unethical if harm or distress is caused
- It is a lot harder to control every variable , so the results may not be reliable nor applicable to a wider population
- Can sometimes be expensive
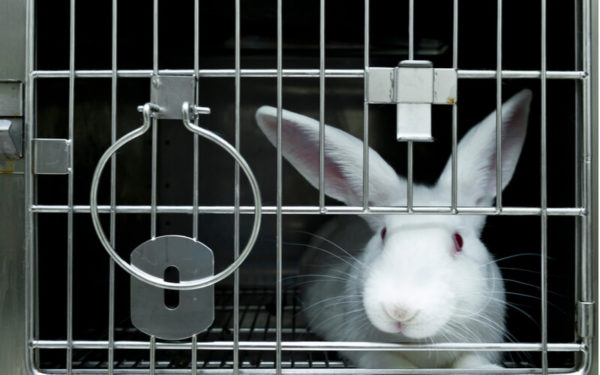
Examples of in vivo experiments
In vivo experiments have been around for a long time, and were one of the earliest methods of investigation.
However, even though technology has advanced so much over the past few decades, we still use live animals in experiments very frequently to this day.
Here are some examples:
Clinical trials
In vivo experiments are the best way through which clinical trials are carried out.
First of all, this is because it’s important to test the effects of a particular substance on the body as a whole , rather than in one localized area or on one particular biological process.
These clinical trials help us to see all of the side effects that a certain drug has on other parts of the body, so we can better evaluate how safe and effective it is.
For instance, though a substance may seem to be highly effective during an initial in vitro study, it may not be so effective in an actual animal due to unforeseen factors. For example, the drug might not be absorbed when it passes through the stomach.
This is why it’s very important to carry out an in vivo experiment after an in vitro one – before releasing a drug to the public.
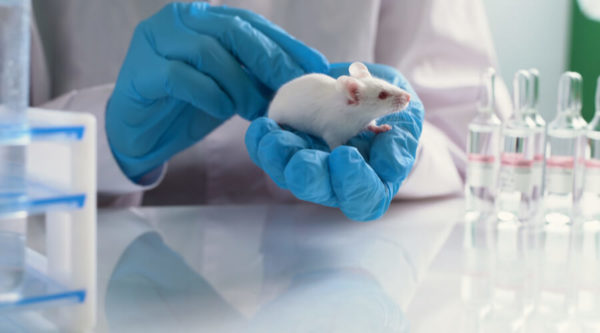
Animal studies
In vivo methods are also used during animal studies , which often come before the clinical trials used on humans.
Animal studies are a useful way of gathering data on the effects of a substance on a living body, without risking human life first.
Things that are often tested on animals include beauty products such as shampoos and soaps, as well as drugs for human consumption.
Some of the most common animals used in animal studies include mice , rabbits , non-human primates , and rats .
For example, non-human primates were used extensively in animal testing to find vaccines for polio , which has since lead to two out of three wild poliovirus strains being pretty much completely eradicated!
On the other hand, other studies have shown that animal testing is not always predictive of human outcomes. In fact, up to 90% of drugs that pass in vivo animal trials fail in subsequent human trials !
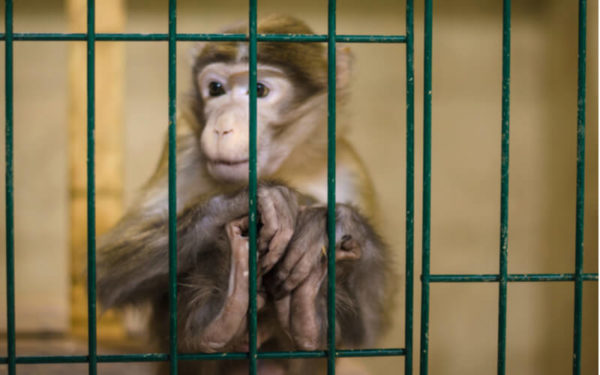
1. Which of the following describes an in vivo experiment?
2. Are in vivo experiments always the best?
3. Which of these organisms is NOT a common laboratory animal used in in vivo experimentation?
4. A clinical trial that aims to test the safety of a new vaccine represents which type of experimentation?
5. Which of the following forms of experimentation has the fewest uncontrollable variables ?
Enter your email to receive results:
Cite This Article
Subscribe to our newsletter, privacy policy, terms of service, scholarship, latest posts, white blood cell, t cell immunity, satellite cells, embryonic stem cells, popular topics, translation, photosynthesis, horticulture, cellular respiration.
- Type 2 Diabetes
- Heart Disease
- Digestive Health
- Multiple Sclerosis
- Diet & Nutrition
- Health Insurance
- Public Health
- Patient Rights
- Caregivers & Loved Ones
- End of Life Concerns
- Health News
- Thyroid Test Analyzer
- Doctor Discussion Guides
- Hemoglobin A1c Test Analyzer
- Lipid Test Analyzer
- Complete Blood Count (CBC) Analyzer
- What to Buy
- Editorial Process
- Meet Our Medical Expert Board
In Vivo vs. In Vitro: What Are the Differences?
Both can advance medical knowledge but have unique limitations
- Definitions
In Vitro Medical Studies
In vivo clinical trials.
The terms "in vivo" and "in vitro" describe different types of scientific research. "In vivo" means research done on a living organism, while "in vitro" means research done in a laboratory dish or test tube.
Both types of studies are used by medical researchers developing drugs or studying diseases. Each type has benefits and drawbacks.
In Vivo vs. In Vitro: Definitions
In vitro : The term in vitro comes from the Latin "in glass." It refers to a medical study or experiment that is done in the laboratory within the confines of a test tube or laboratory dish. This means tissue, cells, or other parts of an organism are removed and placed in a laboratory dish. Researchers may use these samples to test the action of a drug or study a disease process.
In vivo : The term in vivo comes from the Latin "in (something) living." It refers to a medical test, experiment, or procedure that is done on (or in) a living organism, such as a laboratory animal or human.
Clinical trials or medical studies may be performed either in vivo or in vitro. These approaches are similar in that they are both done in order to make advances in the knowledge and treatment of illness and disease as well as understanding "wellness" and normal bodily functions.
But there are also many important differences in how in vivo and in vitro studies are conducted, how they can be interpreted, and the practical applications of any discoveries which are made.
Medical studies (such as looking at the ability of a drug to treat cancer) are often first performed in vitro—either in a test tube or laboratory dish. An example would be growing cancer cells in a dish outside of the body to study them and possible treatments.
Studies are usually done in vitro first for ethical reasons. In vitro studies allow a substance to be studied safely, without subjecting humans or animals to the possible side effects or toxicity of a new drug.
Researchers learn as much as possible about a drug before exposing humans to potential negative effects. If a chemotherapy drug , for example, does not work on cancer cells grown in a dish, it would be unethical to have humans use the drug and risk the potential toxicity.
In vitro studies are important in that they allow more rapid development of new treatments—many drugs can be studied at one time (and they can be studied in a large number of samples of cells) and only those that appear to be efficacious go on to human studies.
An absence of biokinetics (how the body transports and metabolized drugs and toxins) is one of the significant drawbacks of in vitro studies. This, as well as several other factors, can make it very difficult to extrapolate the results of in vitro tests. Thus, it's hard to know what might be expected when the drug is used in vivo.
In contrast to in vitro studies, in vivo studies are needed to see how the body as a whole will respond to a particular substance.
In some cases in vitro studies of a drug will be promising, but subsequent in vivo studies fail to show any efficacy (or, on the other hand, find a drug to be unsafe) when used within the multiple metabolic processes that are continually taking place in the body.
An example of how in vivo studies are needed to evaluate drugs is with respect to drug absorption in the body. A new drug may appear to work in a dish, but not in the human body. It could be that the drug is not absorbed when it passes through the stomach, so it has little effect on humans.
In other cases, even if a drug is given intravenously , the drug might break down through continuous body reactions. Therefore, the drug would not be effective when used directly in humans.
It's important to note that oftentimes in vivo studies are first done in non-human animals such as mice. These studies allow researchers an opportunity to see how a drug works amid other bodily processes.
Mice and humans have important differences. Sometimes a drug that is effective in mice will not be effective in humans (and vice versa) due to inherent differences in the species.
A Word From Verywell
When you look at studies done to evaluate cancer treatments—or any other treatments—checking to see which kind of study it is (in vivo vs in vitro) is an important first step.
In vitro studies are extremely important and lay the groundwork for further research, but many of these studies declare findings that are interesting—but will not affect you as an individual for quite some time to come.
In contrast, in vivo studies are looking at the actual effect on an organism—whether a laboratory animal or a human.
Saeidnia, S., Manayi, A., and M. Abdollahi. From in vitro experiments to in vivo and clinical studies; Pros and cons . Current Drug Discovery Technologies . 2015. 12(4):218-24. doi:10.2174/1570163813666160114093140
Kilkenny C, Browne W, Cuthill IC, Emerson M, Altman DG; NC3Rs Reporting Guidelines Working Group. Animal research: Reporting in vivo experiments: the ARRIVE guidelines . Br J Pharmacol . 2010;160(7):1577–1579. doi:10.1111/j.1476-5381.2010.00872.x
By Lynne Eldridge, MD Lynne Eldrige, MD, is a lung cancer physician, patient advocate, and award-winning author of "Avoiding Cancer One Day at a Time."
- support @. -->@ @. -->novoprolabs.com
- +86-21-61941042
- 86-216-194-1042
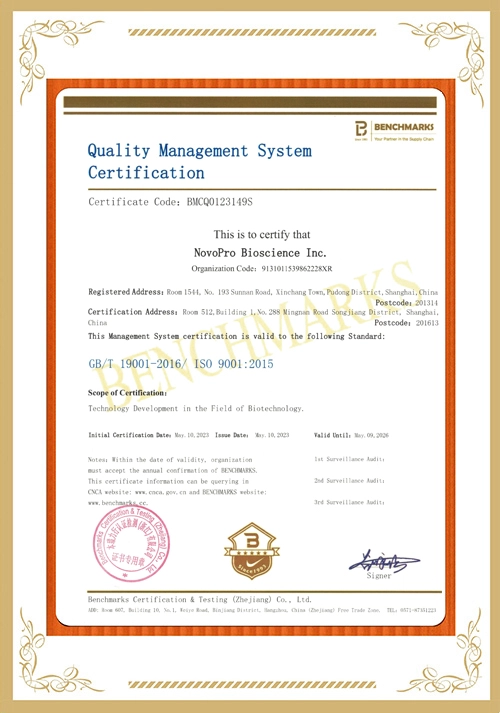
What is the difference between Ex vivo, In vivo and In vitro?
In vitro (Latin: in glass) studies in experimental biology are those that are conducted using components of an organism that have been isolated from their usual biological surroundings in order to permit a more detailed or more convenient analysis than can be done with whole organisms. Colloquially, these experiments are commonly called "test tube experiments". In contrast, in vivo work is that which is conducted with living organisms in their normal, intact state, while ex vivo studies are conducted on functional organs that have been removed from the intact organism.
Common examples of in vitro experiments include work that uses (a) cells derived from multicellular organisms (cell culture or tissue culture), (b) subcellular components (e.g.mitochondria or ribosomes), (c) cellular or subcellular extracts (e.g. wheat germ or reticulocyte extracts), or (d) purified molecules in the test tube (often proteins, DNA, or RNA, either individually or in combination).
In vivo (Latin for "within the living") is experimentation using a whole, living organism as opposed to a partial or dead organism, or an in vitro ("within the glass", i.e., in a test tube or petri dish) controlled environment. Animal testing and clinical trials are two forms of in vivoresearch. In vivo testing is often employed over in vitro because it is better suited for observing the overall effects of an experiment on a living subject. The maxim in vivo veritas ("in a living thing [there is] truth")[1] used to describe this type of testing is a play on words fromin vino veritas, in wine [there is] truth.
In microbiology in vivo is often used to refer to experimentation done in live isolated cells rather than in a whole organism, for example, cultured cells derived from biopsies. In this situation, the more specific term is ex vivo. Once cells are disrupted and individual parts are tested or analyzed, this is known as in vitro.
Ex vivo (Latin: "out of the living") means that which takes place outside an organism. In science, ex vivo refers to experimentation or measurements done in or on tissue in an artificial environment outside the organism with the minimum alteration of natural conditions. Ex vivo conditions allow experimentation under more controlled conditions than is possible in in vivo experiments (in the intact organism), at the expense of altering the "natural" environment.
The term ex vivo is often differentiated from the term in vitro ("within the glass") in that the tissue or cells need not be in culture; these two terms are not synonymous.
In cell biology, ex vivo procedures often involve living cells or tissues taken from an organism and cultured in a laboratory apparatus, usually under sterile conditions with no alterations for up to 24 hours. Experiments lasting longer than this using living cells or tissue are typically considered to be in vitro. One widely performed ex vivo study is the chickchorioallantoic membrane (CAM) assay. In this assay, angiogenesis is promoted on the CAM membrane of a chicken embryo outside the organism (chicken).
Souce: NovoPro 2018-02-26
pregnancy health center / pregnancy a-z list / why is in vivo better than in vitro article
Why Is In Vivo Better Than In Vitro?
- Medical Author: Pallavi Suyog Uttekar, MD
- Medical Reviewer: Shaziya Allarakha, MD
What are in vivo and in vitro?
- Comments **COMMENTSTAGLIST**
- More **OTHERTAGLIST**

While the terms in vivo and in vitro sound very similar, their meanings are not. In vivo is Latin for “within the living.” It is a study model used for a process or procedure that is conducted “on” a living being rather than in cell samples. The in vivo method simulates biological conditions found in a living subject. In vivo studies may be conducted in animals and humans.
In vitro is Latin for “in the glass.” It is a study model in which a procedure is conducted in labs. Interventions may be done on a specific cell culture to grow a virus, bacterium, or fungus, or to test a new drug. Because we look at specific cells during an “in vitro” procedure and not the whole organism, these experiments are more suited for research and analysis.
Given that an in vivo model involves the internal environment of a living being, the results of in vivo studies are considered more reliable or more relevant than those of in vitro studies. This does not mean that an in vitro model is in any way less valuable.
For cases in which normal conception or in vivo fertilization fails, the doctor may advise the couple to try in vitro fertilization (IVF). In vitro fertilization happens when the ovum (female egg cell) and sperm (male reproductive cell) are united outside the body or in a “glass” petri dish.
In the world of research, “in vivo” and “in vitro” models are used in clinical trials , scientific studies, and up-and-coming medical procedures. Scientists use both these models to understand the way a drug (or intervention) affects the body (pharmacodynamic profile) and the way the body affects/breaks down the drug (pharmacokinetic profile). Both these studies serve to create a safer and effective drug profile before they are approved for use by the general population.
Let us look at an example. Suppose scientists want to study a new anti-epilepsy drug. This study goes through various stages. Initially, in vitro studies are conducted in specialized acute seizure models that only need slices of the brain tissue. These models create electroencephalography ( EEG ) signals (brain signals) that are identical to those seen in a patient having a seizure.
During initial testing of the drug, scientists prefer acute seizure models (in vitro) over time-consuming human trials that often involve high levels of individual variation and ethical concerns. Only those drug molecules that show promising results in the in vitro stage will be tested in human clinical trials (in vivo). Here, scientists will see if trends observed in preclinical data (in vitro model) still hold in human volunteers consistently and if the results are reproducible. Thus, drug development needs both in vivo and in vitro models before it is U.S. Food and Drug Administration (FDA)-approved.
Both in vitro and in vivo studies have their own set of advantages and disadvantages, which are explained in the table below.
Category | In vivo method | In vitro model |
---|---|---|
Cost and preparation | ||
Time | ||
Result | ||
Testing regulations | ||
Applications |
In vitro models do not put live subjects at risk. However, they cannot capture the inherent complexity of organ systems and the internal environment of the human body . For example, in vitro cell culture may not account for interactions between various body procedures and cellular biochemistry. Therefore, in vitro studies are often followed by animal studies (in vivo).
Drugs may be tested on animals such as rabbits, hamsters, mice, rats, guinea pigs, dogs , and primates (including monkeys, gibbons, and chimpanzees). Scientists can better evaluate the safety, toxicity, and efficacy of a drug candidate in a complex model (animal). The majority of animals in laboratories are purpose-bred (bred specifically to be used in experiments).
Besides ethical concerns over the use of animals for experiments, the problem of translatability persists because there are considerable physiological differences between humans and animals, and drug absorption, distribution, and excretion may differ.
Only those drugs that appear safe and effective in the in vitro stage eventually go on to the clinical trial stage or in vivo stage.
Top Why Is In Vivo Better Than In Vitro Related Articles

Early Pregnancy Symptoms Before Missed Period

16 Early Signs & Symptoms of Pregnancy: Could You Be Pregnant?

Am I Pregnant Quiz

Exercise & Pregnancy

How Soon Can You Get Symptoms of Pregnancy?

Ovulation & Fertility

Pregnancy Myths and Facts Quiz

Pregnancy Planning (Tips)

Stages of Pregnancy: Week by Week

Pregnancy: 7 Common Third Trimester Tests

Home » Science » Biology » Difference Between in vitro and in vivo
Difference Between in vitro and in vivo
Main difference – in vitro vs in vivo.
In vitro , in vivo , and in silico are the three types of experimental models used in biological science laboratories. The main difference between in vitro and in vivo is that in vitro refers to the experimental procedures performed outside a living organism whereas in vivo refers to the experimental procedures performed within a living organism . In silico refers to the experiments performed on the computer. In vivo experiments are performed under physiological conditions while i n vitro experiments are performed under controlled laboratory conditions.
Key Areas Covered
1. What is in vitro – Definition, Facts, Examples 2. What is in vivo – Definition, Facts, Examples 3. What are the Similarities Between in vitro and in vivo – Outline of Common Features 4. What is the Difference Between in vitro and in vivo – Comparison of Key Differences
Key Terms: Experimental Models, In vitro, In silico, In vivo, Laboratory Conditions, Physiological Conditions

What is i n vitro
In vitro refers to a phenomenon in which a given procedure is performed in a controlled environment outside of a living organism. The majority of cellular experiments are performed in vitro as it is less expensive. But, the regeneration of the physiological conditions of an organism is difficult inside a test tube. Therefore, the results of the in vitro experiments are less precise. This means the results of in vitro experiments do not correspond to the circumstances occurring around living organisms. A bacterial culture is shown in figure 1.

Figure 1: Bacterial Culture
In vitro experiments are performed using the extracted cellular components from their regular biological environments. The cellular components can be microorganisms, cells, organelles or biological molecules. The cells and microorganisms are grown in artificial culture media while biological molecules are studied in solutions. The in vitro experiments are performed in Petri dishes, test tubes or flasks.
What is in vivo
In vivo refers to a phenomenon in which experiments are performed using a whole, living organism. The two forms of in vivo experiments are animal studies and clinical trials during drug development. The overall effect of the experiment on a living organism can be observed in in vivo techniques. Thus, in vivo experiments are more precise than in vitro experiments. The main objective of in vivo experiments is to gain knowledge about biological systems or discover drugs. A lab mouse is shown in figure 2.

Figure 2: Lab Mouse
However, in vivo experiments are more expensive and require more advanced techniques during the experiment. Mice, rabbit, and apes are the three main types of living organisms used in in vivo techniques.
Similarities Between in vitro and in vivo
- In vitro and in vivo are two types of experimental models used in laboratories.
- Both in vitro and in vivo experiments are performed under a given set of conditions.
- Fertilization can be performed in both in vitro and in vivo
Difference Between in vitro and in vivo
In vitro: In vitro refers to a phenomenon in which a given procedure is performed in a controlled environment outside of a living organism.
In vivo: In vivo refers to a phenomenon in which experiments are performed using a whole, living organism.
Types of Samples
In vitro: Dead organisms or isolated cellular components are used in in vitro experiments.
In vivo: A whole living organism is used in in vivo experiments.
In vitro: In vitro experiments are performed under controlled laboratory conditions.
In vivo: In vivo experiments are performed under physiological conditions.
In vitro: In vitro experiments are less expensive.
In vivo: In vivo experiments are expensive.
In vitro: In vitro experiments are less time-consuming.
In vivo: In vivo experiments are more time-consuming.
In vitro: In vitro experiments are less precise.
In vivo: In vivo experiments are more precise.
In vitro: Cell culture experiments in Petri dishes and experiments in test tubes are examples of in vitro.
In vivo: Drug testing experiments performed by using model organisms such as mice, rabbit, apes etc. are examples.
Fertilization
In vitro: In vitro fertilization (IVF) refers to the artificial fertilization method in which fusion of male and female gametes occurs outside the human body.
In vivo: Regular fertilization mechanism in which the fusion of male and female gametes occurs within the body is referred to as in vivo fertilization.
In vitro and in vivo are two types of experimental methods used in laboratories. In vitro experiments are performed in test tubes. These experiments are performed under laboratory conditions. But, in vivo experiments are performed within living organisms. These experiments take place under physiological conditions. The main difference between in vitro and in vivo is the type of conditions under which each type of experiments are performed.
1. Pearson, R M. “In-Vitro techniques: can they replace animal testing?” Human reproduction (Oxford, England)., U.S. National Library of Medicine, Dec. 1986, Available here . 2.“In vivo.” Wikipedia, Wikimedia Foundation, 11 Jan. 2018, Available here .
Image Courtesy:
1. “Bacterial culture” By Joydeep – Own work (CC BY-SA 3.0) via Commons Wikimedia 2. “Lab mouse mg 3213” By Rama – Own work (CC BY-SA 2.0 fr) via Commons Wikimedia
About the Author: Lakna
Lakna, a graduate in Molecular Biology and Biochemistry, is a Molecular Biologist and has a broad and keen interest in the discovery of nature related things. She has a keen interest in writing articles regarding science.
You May Also Like These
Leave a reply cancel reply.

An official website of the United States government
The .gov means it’s official. Federal government websites often end in .gov or .mil. Before sharing sensitive information, make sure you’re on a federal government site.
The site is secure. The https:// ensures that you are connecting to the official website and that any information you provide is encrypted and transmitted securely.
- Publications
- Account settings
Preview improvements coming to the PMC website in October 2024. Learn More or Try it out now .
- Advanced Search
- Journal List
- Microb Biotechnol
- v.15(1); 2022 Jan

In vivo , in vitro and in silico : an open space for the development of microbe‐based applications of synthetic biology
Antoine danchin.
1 Kodikos Labs, Institut Cochin, 24 rue du Faubourg Saint‐Jacques, Paris 75014 France
Living systems are studied using three complementary approaches: living cells, cell‐free systems and computer‐mediated modelling. Progresses in understanding, allowing researchers to create novel chassis and industrial processes rest on a cycle that combines in vivo , in vitro and in silico studies. This design–build–test–learn iteration loop cycle between experiments and analyses combines together physiology, genetics, biochemistry and bioinformatics in a way that keeps going forward. Because computer‐aided approaches are not directly constrained by the material nature of the entities of interest, we illustrate here how this virtuous cycle allows researchers to explore chemistry which is foreign to that present in extant life, from whole chassis to novel metabolic cycles. Particular emphasis is placed on the importance of evolution.
The iteration cycle: design–build–test–learn, between experiments and analyses combining physiology, genetics, biochemistry and bioinformatics in an ever more advanced way, generates the most interesting future developments in microbial synthetic biology of industrial interest.

Introduction: complementary avenues for the exploration of life
It took a long time for science to understand that there was no difference between ‘organic’ and ‘inorganic’ matter. The nature of the chemical compounds of life had no specificity other than, perhaps, the fact that they were based on the chemistry of carbon. Still, the status of biology is somewhat different from that of standard chemistry, for living organisms are deeply connected with a surprising quality of ‘animation’, which at first sight seems alien to the laws of physics. As a consequence of this observation, studies of living organisms were separated into in vivo (using living cells and organisms as the basic entities of interest) and in vitro (using parts of living entities combined together in test tubes) studies. More recently, with the enormous development of computing facilities, it has become possible to replace experimental studies using authentic material entities with studies of the living using computers. Although little developed before the advent of DNA sequencing techniques, this change in the study of life was greatly stimulated when it became apparent that knowledge of genomic sequences would rapidly become ubiquitous. The need to develop this endeavour was highlighted for the first time at a meeting of the European Biotechnology Action Programme in Tutzing, Germany, in 1989: ‘ From the information technologies perspective, we are faced with the need to create databases where we will recognize relevant features in response to learning processes. The creation of descriptors, the genesis of exploratory hypotheses will add to the traditional methods of biology, in vivo and in vitro , experiments in silico’. Although this emphasis is already three decades old, it can be safely anticipated that in silico approaches will gain in importance in the coming decades, especially for developments in microbial biotechnology. In what follows, we briefly summarize the state of in vivo microbial studies, focusing on the forthcoming development of novel artificial chassis, then focus on current trends in cell‐free syntheses and conclude with progress in in silico research. One of the conclusions of this study is that to understand the functions responsible for the apparent animation of living systems, the activities that dissipate energy in unanticipated ways should be given priority in the coming years.
A future for in vivo microbial studies
Microbial biotechnology exploits a substantial collection of microbes widely used to generate products/processes of interest to industry. Industrial applications go hand in hand with understanding the puzzles of their environmental niches and metabolism. We can anticipate that, using these same organisms, metabolic engineering will continue to evolve over the next few decades, now benefiting from rapid advances in synthetic biology (SynBio), with a likely emphasis on the use of large‐scale DNA synthesis (Panke, 2020 ). This topic has been the subject of many studies [see, e.g. Calero and Nikel ( 2019 )], and we do not explore it further here, except for a brief review of recent uses showing how novel living chassis will gradually gain impact in the near future. Following this pattern, we describe in this section how the emergence of novel microbial chassis is about to revolutionize microbial engineering.
Brief landscape of the use of genetically modified natural microbial chassis
Until recently, the majority of microbial biotechnology advances that did not rest on microbes with idiosyncratic properties – typically, specific synthesis of metabolites such as vitamin B12 (Balabanova et al ., 2021 ) – used a variety of Escherichia coli strains as the basis for elaborate metabolic engineering. This is not discontinued: sophisticated SynBio designs are already implemented in this organism, as seen, for example, in the use of optogenetics to control expression of the plant lycopene biosynthesis pathway in this organism (Raghavan et al ., 2020 ). Such innovative leads could soon become routine. The ubiquitous role of E. coli as a chassis was not unexpected due to the considerable accumulation of knowledge gained for this species. Relevant access to accurate sequence annotation is key for productive industrial developments. Alas, errors keep percolating in automatic genome annotation procedures that are in effect essentially based on the still imperfect knowledge of model organisms (Bell and Lord, 2017 ). Yet, complete, up‐to‐date annotation of the E. coli genome sequence is unfortunately not properly maintained on a perennial basis. The best public annotation of the sequence at this time is perhaps the one developed using orthologue‐based annotation propagation (Paley et al ., 2021 ), but it is not adapted to the subtle requirements of industrial demands. This drawback implies that the use of chassis other than E. coli requires even more tedious manual annotation to account for the inevitable idiosyncrasies uncovered in the course of engineering [see the importance of ‘kludges’, by definition unexpected, in (Danchin, 2021 )].
SynBio studies have long aimed at getting rid of seemingly superfluous gene‐encoded functions, creating universal minimal chassis in which biotechnology‐relevant pathways can be introduced. In line with this objective, the Mollicutes clade was perceived as an interesting paradigm because these organisms had already evolved streamlined genomes (Piñero‐Lambea et al ., 2020 ). Mollicutes have been and will still be used to understand the minimal constraints of cell survival and multiplication (Garcia‐Morales et al ., 2020 ). However, because these organisms have a highly reduced genome and minimal macromolecule synthesizing machineries, they are intrinsically fragile and limited in their metabolic power, and thus will hardly be convenient for widespread industrial developments. In contrast, a large collection of organisms that thrive in more or less extreme environments are likely to take the limelight in the near future (Liu and Deutschbauer, 2018 ). Here is a brief list of some relevant candidates [see (Calero and Nikel, 2019 ) for a detailed account of an exhaustive list of non‐traditional industrial microbes.]
The Pseudomonas genus is well suited to metabolize aromatic compounds and harbour novel metabolic pathways (Bitzenhofer et al ., 2021 ). The genome of Pseudomonas putida has already been streamlined and shown to allow improved heterologous gene expression (Lieder et al ., 2015 ). A variety of tools are available for this organism, which now becomes a widespread platform for SynBio constructs (Martin‐Pascual et al ., 2021 ). For example, even though this organism has its metabolism poised to develop in the presence of dioxygen, strain KT2440 has been modified to multiply in anaerobic conditions (Kampers et al ., 2021 ). A companion, Comamonas testosteroni, a species belonging to a related clade, has become a promising chassis for bioremediation due to its natural pollutant‐degrading capacity (Aksu et al ., 2021 ).
In the actinobacterial clade, Corynebacterium glutamicum has been used for decades as a major industrial source of vitamins, amino acids, carbohydrates and a variety of other metabolites. It is now the subject of a fair number of improvements to accommodate novel metabolic pathways and SynBio constructs (Chen et al ., 2021a ; Wang et al ., 2021 ; Wolf et al ., 2021 ). With many industrial facilities already using this organism, it seems likely that further improvements will accumulate rapidly. In the same way, but in the Firmicutes clade, Bacillus subtilis has also been much discussed as an important industrial chassis (Liu et al ., 2020 ; Xiang et al ., 2020 ). Besides applications similar to those discussed previously with other organisms, as well as often ignored roles, such as that in heavy industry where it is used for improving concrete ageing via creating self‐healing properties, for example (Nielsen et al ., 2020 ), the fact that Bacilli generate extremely resistant spores is likely to generate original methods for new types of applications. Using a 3D printer, Christopher Voigt and his colleagues printed B. subtilis spores within an agarose scaffold, showing that they germinated on its exterior surface, including spontaneously in cracks. The agarose‐spores material sustained desiccation and returned to life after rehydration. When containing spores of B. subtilis engineered to produce antibiotics, the material could be used to kill Staphylococcus aureus , a pathogen causing a variety of infections (González et al ., 2020 ). This family of advances are so interesting that they have even been used for 3D printing of a mixture of recombinant E. coli‐ expressing metallothionein/gold nanocomposites used as agitating paddles for the catalytic reduction of 4‐nitrophenol (Long et al ., 2021 ).
Still in the domain of bacteria, Cyanobacteria, being able to fix carbon dioxide, have a distinctive status, especially under conditions when the steady increase in carbon dioxide in the atmosphere of our planet is a matter of concern. The species of this phylum are likely to become a preferred chassis for biotechnological developments in the near future (Wang et al ., 2020 ). However, they display specific structural features that make them difficult to manipulate. Their metabolism, resting on gasses and reactive metabolites, must be compartmentalized (de Lorenzo et al ., 2015 ; Flechsler et al ., 2021 ). This means that future progress with these organisms is likely to require considerable effort to account for the existence of compartments before they are suitable for versatile metabolic engineering. Nevertheless, the proliferation of research facilities based on this family of chassis is already showing us how these bacteria will soon be commonly used (Yang et al ., 2020 ; Cui et al ., 2021 ). Other photosynthetic bacteria have also been proposed as new chassis, especially for water treatment. Some non‐sulphur bacteria, the oldest of the photomicroorganisms, not only treat different types of wastewater but are also non‐toxic bioresources, containing many value‐added products. These bioresources can be used as raw materials in the agricultural, food and medical industries (Lu et al ., 2021 ).
Finally, besides a few applications using Archaea [see, e.g. Aldridge et al . ( 2021 )], the Eukarya domain of life is also a common source for microbial biotechnology. In this context, the model Saccharomyces cerevisiae plays a role similar to that of E. coli , but other yeasts are also widely used, such as Yarrowia lipolytica , for lipid‐related applications (Park and Nicaud, 2020 ). The lines of research we have just outlined with Bacteria will also be developed with these organisms, including after their genomes have been reworked to facilitate genetic manipulation (Schindler, 2020 ). This is already seen in the high‐level original developments such as those listed in Voigt ( 2020 ). These examples demonstrate that we are on the verge of an enormous number of applications based on SynBio in vivo metabolic engineering and material improvement of extant microbes, notwithstanding the variation associated with inevitable evolution of all living organisms (Wassenaar and Zimmermann, 2020 ).
Alien chassis and xenobiotics
Most contemporary advances in SynBio are variations on a theme based on reconstructed metabolic pathways derived from natural sources, and artificial constructs deployed in a variety of natural chassis. Conclusive innovation, however, will really come to life when heterologous implementation of known pathways will be replaced by counterparts that have not evolved in extant organisms, as well as chassis modified to be distinct from standard living cells. A considerable amount of work is being done in this direction. Here, we describe some of the corresponding endeavours, while recognizing that, for the time being, examples of modification of the core chemistry of extant chassis remain rare. Perhaps the best illustration of promising work is the creation of Escherichia chlori , an avatar of E. coli where thymine has been entirely replaced by 5‐chlorouracil in DNA (Marlière et al ., 2011 ). However, there is only little data available about the stability of the construct. We should expect to understand before long the functions that have been altered in this organism, allowing it to grow on 5‐chlorouracil while being inhibited by thymine. This entails understanding how these chassis will behave in the long run, but we can be confident that there is some hope for stability as demonstrated in stable evolution after 100 days of an E. coli strain after continuous co‐culture with mammalian cells (Kunjapur et al ., 2021 ).
A large number of avenues are being explored to further modify the nature of the genetic material of cells based on xenonucleic acids (Fiers et al ., 2016 ). While there is continuous progress in the domain (Chaput and Herdewijn, 2019 ), the emergence of autonomous novel chassis amenable to industrial applications seems unlikely for the next decade or so. In the meantime, less dramatic changes continue to be designed. Since the beginnings of SynBio research, researchers attempted to reassign codons (Robertson et al ., 2021 ), often to non‐natural proteinogenic amino acids, as for example illustrated early on (Döring and Marlière, 1998 ). The most efficient approaches attempt to device orthogonal constructs, for the time being devoted to a limited fraction of the translation machinery (Schmied et al ., 2018 ). Recent attempts have created an artificial genetic code of 68 codons that could allow the incorporation of four non‐canonical amino acids into synthetic proteins (Dunkelmann et al ., 2021 ). In other advances, the synthesis of proteins containing d‐amino acids, β‐amino acids, phosphorylated amino acids as well as long‐chain and cyclic amino acids in which the nucleophilic amino group is not in the α‐position has been achieved using modified peptidyl transferase regions in the 23 ribosomal RNA. Dipeptides and dipeptidomimetics of varying utility were also obtained (Hecht, 2021 ). Because this is sophisticated work – relevant cells must maintain both the standard machinery and an orthogonal one – industry‐relevant progress has been slow. With an increasing number of laboratories involved in these attempts, the number of successes is expected to increase rapidly. However, again, much of the corresponding works are more proofs of concept than ready to become, biotechnology‐adapted synthetic microbes growing in industrial fermenters. More limited approaches have already been used to produce synthetic variants of proteins, for example, using analogues of amino acids such as selenomethionine for bioconjugations (Flood et al ., 2021 ) or variants of norleucine, known to replace methionine in proteins in E. coli for a very long time (Moroder and Budisa, 2010 ; van Eldijk and van Hest, 2018 ). Nevertheless, a creation somewhat similar in its concept to that of E. chlori was based on growth of cells on tryptophan analogues. In the earliest experiments, full replacement of tryptophan residues by an analogue could not yet be achieved (Bacher and Ellington, 2001 ). Later on, complete replacement was successful in a B. subtilis mutant that swapped all tryptophan residues for 4‐fluorotryptophan after serial selection and mutagenesis (Yu et al ., 2014 ).
Yet another highly focused advance, isobiology, has been proposed. It is based on the observation that biological processes differentiate the isotopes of atoms used to form cells. This line of experiments involves a very small modification of the chassis. It is, therefore, likely to have minimal consequences. The most important effect is expected when the standard cell composition replaces hydrogen by deuterium, in particular when heavy water (D 2 O) replaces standard water (H 2 O). Notwithstanding its financial cost, this procedure limits the consequences of changes while allowing the fine properties of the cell chassis to be explored (Danchin, 2020 ). This type of effort is likely to be important for understanding functional phase transitions in cells (Choi et al ., 2020 ), a concept that emerged in parallel with the onset of SynBio, and which is likely to be crucial in the construction of novel chassis as it defines previously neglected membrane‐less compartments (Gomes and Shorter, 2019 ). As an illustration of future progress, specific isotope‐sensitive microbial models could be used for the development of biotechnological processes, based on the behaviour of thermophilic Archaea (Nishizawa et al ., 2016 ). This would be motivated, for example, by the fact that the use of carbon, nitrogen and oxygen isotopes is already well accepted – despite the cost of the experiments – as safe substitutes for tracer experiments (Davies, 2020 ). This opens up a niche for the industrial microbial production of amino acids and nucleotides (Nicolás Carcelén et al ., 2017 ).
Adaptive evolution
Life is a dynamic process, not only during the lifetime of an organism but also in the long term, as it keeps generating offspring. Evolution is an essential feature of life. Therefore, many SynBio projects have enabled constructs established in well‐identified laboratory strains to evolve under various conditions [see, e.g. Li et al . ( 2021 )]. Adaptive evolution has even been successfully developed for co‐evolving communities of organisms (Konstantinidis et al ., 2021 ). In most cases, the operation has developed in large volume vessels, controlled as chemostats are. Among promising approaches, a variation on chemostats driven by growth medium dilution found that, in a continuous culture, growth chambers were invaded by mutants that escape dilution by attaching to the surface of the vessel. As expected, this trait is positively selected. It generates persistent subpopulations whose size and growth rate are uncontrollable, preventing the use of standard detection methods such as turbidity measurements to monitor growth. To overcome this limitation, a device with two growth chambers subjected to brief periodic alkaline sterilization phases has been designed (de Crécy‐Lagard et al ., 2001 ; de Crécy et al ., 2007 ). Yet, because these vessels are at least a few millilitres in volume, this setting precludes carrying out large‐scale experiments with many vessels working in parallel. This drawback triggered the development of continuous culture systems based on open reactors managing microfluidic droplet s [see (Ito et al ., 2016 ) and the special issue on recent advances of molecular machines and molecular robots edited by Takinoue and Kawano, which describes advances in the domain, mainly in Japan (Takinoue and Kawano, 2020 )]. The future of laboratory‐set evolution is now likely to rest on nanolitre reactors such as those already used for production of important metabolites (Xu et al ., 2020 ), allowing in vivo directed evolution (Femmer et al ., 2020 ). This is at the cost of the evolving population's size, however, so that it is likely that a significant family of evolution devices will keep using sizeable vessels in the future.
The design of alien metabolism
Intermediary metabolism is an intrinsic component of all live chassis. In most cases, researchers have attempted to streamline their pathways of interest in existing organisms with a small series of efforts to completely replace essential pathways with artificial ones. Still, extant pathways remain important: E . coli can be reprogrammed to fix carbon dioxide by simple modifications in its canonic carbon metabolism pathways (Satanowski et al ., 2020 ). However, an entirely novel pathway for carbon fixation could be implemented in this organism. The standard core one‐carbon metabolism has been reprogrammed with the design of an alternative cyclic pathway that substitutes 4‐hydroxy‐2‐oxobutanoic acid (HOB), a compound absent from canonical metabolism – except perhaps as an accidental consequence of aminotransferases’ promiscuity (Walther et al ., 2018 ) –, for the amino acids serine and glycine as omnipresent one‐carbon donors. This pathway is based on two novel reactions, transamination of l‐homoserine and transfer of a one‐carbon unit from HOB to tetrahydrofolate, releasing pyruvate. Canonic reactions regenerate l‐homoserine from pyruvate by carboxylation and subsequent reduction. In this way, any one‐carbon moiety made available for anabolic reactions may originate from CO 2 . The HOB‐dependent pathway was established in an E. coli auxotroph selected for prototrophy after enrichment in a long‐term cultivation system (Marlière et al ., 2011 ). Monitoring its stability will be interesting, as l‐homoserine, an analogue of authentic proteinogenic amino acids, interferes with translation (Jakubowski, 1997 ). Living organisms have found solutions to circumvent this obstacle, for example, via protection/deprotection systems (Danchin and Sekowska, 2015 ), and we should expect that, if the pathway’s industrial role requires to raise its production level, such solutions will emerge before long in laboratory‐set evolution experiments. Indeed, a cognate cycle that uses a homoserine phosphorylation step was successfully designed to use methanol to feed general SynBio‐derived bioproduction processes, and it likely to be improved in the near future [(He et al ., 2020 ) and Fig. 1 ].

Natural methanol assimilation compared with methanol assimilation in a HOB‐dependent cycle.
The usual input of methanol/formate into carbon metabolism (left) is compared (right) with the novel HOB‐dependent cycle described in He et al . ( 2020 ). Note that hydroxypyruvate is potentially toxic.
While it remains essential for SynBio developments to design integrated living units, the very fact that living cells are constantly evolving makes it crucial to bring out the symmetry of synthesis and analysis – breaking down cells into their components – both to understand the details of life processes and to implement industry‐friendly activities that are more stable over time than inventions with life. This involves exploring the principles of synthetic life in vitro , bearing in mind that we must both master the choice and availability of relevant components and advance the conceptual understanding of all the relevant processes that take place in living cells in order to make them work in vitro .
In vitro syntheses and evolution
Molecular biology is a combination of genetics and biochemistry ( https://plato.stanford.edu/entries/molecular‐biology/ ). Until these fields of biology were merged, the study of life was divided between the exploration of organisms in vivo – alive – and the chemical analysis of some of their components once synthesized in test tubes, in vitro . However, what was successful in vivo did not overlap much with what was discovered in vitro . The apparent ‘animation’ of cells and organisms remained a mystery. As in the early XIX th century, when chemicals were divided between organic and inorganic matter, some biologists believed – some still believe – that macromolecules, in particular, have mysterious properties, specific to life. The actual, purely chemical synthesis of macromolecules was, therefore, a prerequisite for the exploration of life, accepted as belonging to the realm of physics and chemistry. This feat was achieved with small proteins such as insulin. Today, the chemical synthesis of proteins is a difficult challenge that remains relevant, not in response to philosophical debates, but as an industrial target (Hui et al ., 2021 ): high‐level accuracy in the protein sequence of amino acids appears to be easier to control in vitro than in vivo (Karas et al ., 2021 ). The same situation prevailed with nucleic acids (Caruthers, 2013 ), with the same consequences in terms of industrial developments: For example, gene and vaccine syntheses require a very high precision, with accessible controls at each stage, a requirement which remains still difficult to achieve with in vivo constructs. While life seems to have evolved a way to keep error rate in many processes to some minimum (Bradley et al ., 2019 ; Mordret et al ., 2019 ), the trade‐off between speed and accuracy in biosyntheses has been adopted by natural selection as a means to input resilience in the build‐up of living entities (Danchin et al ., 2011 ), making a significant level of errors an inevitable consequence (and cause) of evolution (Médigue et al ., 1991 ; Piñeros and Tlusty, 2020 ; Jones and Uphoff, 2021 ).
Cell‐free protein synthesis, a half‐century ongoing story
Once it was recognized that there was no magic involved in enzymatic activity, it was widely – but not always – accepted that enzyme‐based synthesis of molecules in vitro could be accepted as yet another artificial means of constructing macromolecules to complement purely chemical syntheses. In a first step – in an experiment with impact similar to that of the synthesis of urea by Woehler, as it established that there was no animation principle in RNA synthesis –, Sol Spiegelman used the replicase of RNA bacteriophage Qβ to synthesize phage RNA in the test tube. The ‘little monster’, thus, produced was physically and chemically indistinguishable from the original virus RNA. Indeed, to establish that the test‐tube RNA was infective, Spiegelman injected it in a solution of all four nucleoside triphosphates and Qβ replicase, waiting for the RNA to replicate. He then incubated it with live bacteria, and demonstrated that the RNA led to generation of infective viral particles (Haruna and Spiegelman, 1965 ). In another family of in vitro experiments, RNA synthesis of more or less random sequence was successful with an enzyme that did not use nucleic acids as templates, polynucleotide phosphorylase (Grunberg‐Manago, 1963 ). Much of the discovery of the genetic code table used synthetic RNA made with this enzyme, further blurring the line between chemistry and biology.
With synthesis of RNA and proteins available in vitro , the goal was to shift experiments to the upper level of biosyntheses and set up the multi‐level process of translation in the test tube. Mimicking macromolecule biosynthesis as it unfolds in vivo was a true challenge. To go further in the direction of authentic protein synthesis, it was important to demonstrate translation of a monocistronic message. This was established early on (Clark et al ., 1965 ). This difficult undertaking, which began in the early 1960s, is still under development as more and more components are integrated into the synthetic machinery of test tube. We will gradually gain a better understanding of the reasons for the difference between these processes when they take place in vivo and in vitro . With synthetic RNAs, the importance of the pH and the role of magnesium (Jiang et al ., 2021 ), polyamines (Fukuma and Cohen, 1975 ) and potassium (Fritz et al ., 2018 ) were identified as crucial for success (Hammerling et al ., 2020 ). Translation with ribosome preparations, endowed of progressively increasing specificity and accuracy, was much improved after the discovery of the need to start and stop translation, using initiation factors (Clark and Marcker, 1966 ) and subsequently other factors such as the ribosome release factor (Kung et al ., 1977 ). A further step was gained when DNA, much more stable than RNA, could be used as a template for in vitro gene expression, allowing protein synthesis via coupling transcription with translation in the test tube (Austin and McGeoch, 1973 ; Isturiz and Wolf, 1975 ).
Improved ‘cell‐free’ RNA and protein synthesis systems based on bacterial and eukaryotic extracts then became common, with cloned or synthetic genes as DNA templates (Billerbeck et al ., 2013 ; Caschera and Noireaux, 2014 ; Li et al ., 2014 ). The knowledge developed in the early decades of transcription–translation coupling in the test tube has been used to design a prototype flow microreactor for synthetic biology in vitro providing solutions for industrial biosyntheses (Boehm et al ., 2013 ). Reconstituted cell‐free protein synthesis systems such as the protein synthesis using recombinant elements (PURE) system allowed synthesis of a wealth of recombinant proteins. This family of cell‐free systems keeps being improved (Grasemann et al ., 2021 ). Yet, despite continuous progresses (Zhang et al ., 2021b ), sometimes for industrial purposes (at least for the synthesis of proteins used by research laboratories), the speed and accuracy of the translation process remained significantly below what happens in vivo .
Several biological functions were identified as critical for improving the outcome of cell‐free syntheses. For example, proper protein folding was critical. In contrast to the widely accepted, but wrong, Anfinsen’s postulate that assumes that the whole folding potential is present in the protein primary sequence, it is now established that most unfolded proteins cannot spontaneously fold properly (To et al ., 2021 ). As a first step, the ribosome itself is important as a folding device during translation, but the trigger factor (Piette et al ., 2011 ; Koubek et al ., 2021 ) and other molecular chaperones (Yang et al ., 2019 ) have often a decisive role. Many are dissipating energy (Boel et al ., 2019 ; Mallory et al ., 2019 ), which impacts the energy balance of the procedure, a feature rarely discussed. Furthermore, it appeared progressively that the role of water and molecular crowding were critical to ensure fidelity of translation [see, e.g. Garenne et al . ( 2020 )]. The importance of these functions is not yet fully understood and it is likely that their role will impact future developments of cell‐free syntheses. Their role is to discriminate between classes of entities (young and aged objects and also spatial positions or alternative structures, for example). This is illustrated, for example, by innate immune sensors such as RIG‐I, which sensitively detect and respond to viral RNAs that enter the cytoplasm, while remaining unresponsive to the abundance of structurally similar RNAs that are the products of host metabolism (Ren et al ., 2019 ). Similarly, the SecA ATPase, which binds its substrates post‐translationally, scans the ribosomal tunnel for potential substrates to secrete the correct ones (Knüpffer et al ., 2019 ). The information for discrimination is managed by the energy dissipation reflected by the ATP dependence of the enzyme (Boel et al ., 2019 ). In terms of SynBio developments, consideration of these agents, particularly ATP‐dependent proteases such as protease Lon, will have a considerable impact on the production of canonical proteins (Tzeng et al ., 2021 ).
Yet another idiosyncratic feature of proteins has also been identified as having a likely impact on cell‐free protein biosynthesis. While proteinogenic residues are universally considered to be ‘amino acids’, proline is a secondary cyclic amine (formerly, called ‘imino acid’). This characteristic requires the translation machinery to take this chemical singularity into account. Fast and accurate translation of proline residues requires a specific variant of a post‐translationally modified translation elongation factor, EF‐P, or other counterparts depending on the organism (Hummels and Kearns, 2020 ). Yet, the explicit use of EF‐P has seldom been explored as an explicit partner in cell‐free protein synthesis (Li et al ., 2017 ; Hammerling et al ., 2020 ). Sequences of three consecutive prolines can fold into polyproline helices, structures that join alpha helices and beta folds as architectural motifs in protein configuration. Triproline helices are involved in protein–protein signalling interactions. Management of elongation factor EF‐P in cell‐free synthesis of proteins with series of proline residues should improve the outcome of a protein production line. This is not a minor point, as, for example, more than 4% of the human proteome contains series of three or more prolines (Morgan and Rubenstein, 2013 ). Furthermore, since EF‐P binds to the 9 nt D‐loop found in tRNAPro isoacceptors, and not to proline itself (Katoh et al ., 2016 ), the use of this factor can be expected to play a key role in the precision of cell‐free translation engineering, a feature essential for SynBio developments. After half a century of hard work, the various obstacles to the development of in vitro synthesis continue to be improved, with the emphasis on speed (Burrington et al ., 2021 ). Much remains to be understood to allow the industrial process of translation to be stable in time, with proper maintenance activities in particular. We may expect that exploring these weak points will dominate research in the domain in the near future. This is more important because, during the spontaneous process of protein ageing – which is protein specific and spanning a considerable time frame, from minutes to centuries (Zhang et al ., 2020 ) –, proteins tend to aggregate after subtle conformation changes in the absence of the energy requirements that allow a subset of NTP‐dependent activities to refold proteins in their proper shape, dissolving aggregates (Dewachter et al ., 2021 ).
In addition to using in vitro protein synthesis as a means of identifying all the key partners of the in vivo process, cell‐free protein synthesis also allows proteins to be synthesized with a variety of non‐proteinogenic amino acid analogues. Worth highlighting, the ‘flexizyme’ system based on ribozymes that are able to load a large number of chemical substrates onto tRNAs [(Passioura and Suga, 2014 ) and Fig. 2 ] eliminates the need to modify aminoacyl‐tRNA synthetases prior to synthesis. We can expect that this family of cell‐free approaches will make a considerable part of next‐generation SynBio developments, in particular for the synthesis of polymers of industrial interest. This will enable the synthesis of polymers involving structurally disparate non‐α‐amino acids that bear little resemblance to canonical proteinogenic amino acids. Examples include long‐chain carboxylic acids, substituted benzoic acids, 1,3‐dicarbonyls, peptides and helical aromatic oligomer‐peptide hybrids. For example, substituted benzoic acids are the precursors of aramids, a class of aromatic polyamides with a rich progeny. Similarly, long‐chain amino acids are monomeric precursors of nylon (Tharp et al ., 2021 ).

Flexizyme RNAs as substitutes for aminoacyl‐tRNA ligases [from Ohuchi et al . ( 2007 )].
Starting from an aptamer that binds phenylalanyl‐AMP, synthetic variants (random sequences in the region highlighted in green) are constructed and submitted first for selection for binding to the 3’‐CCA end of phenylalanine tRNA, then to a further selection after variants (region highlighted in orange) are proposed for the binding of analogues of phenylalanyl‐AMP (orange star).
These novel trends will benefit both from development of RNA SynBio and from streamlined cell‐free systems amenable to scaling up.
As their name suggests, cells are compartments, specifically delimited by membranes built around lipid bilayers. Early on, as it became apparent that DNA‐driven in vitro protein synthesis was often difficult to achieve, some researchers resorted to the spontaneous ejection of chromosome‐less vesicles by some cells as an alternative to a purely in vitro system (Bose et al ., 2020 ). These vesicles, ‘mini‐cells’, often kept plasmids in their cytoplasm, allowing protein expression from artificial constructs (Grindley et al ., 1977 ; Miller et al ., 1977 ; Bose et al ., 2020 ). Similarly, in animal cell‐free systems, exosomes or cell‐derived small extracellular vesicles have been used in therapeutic intervention (Pedrioli et al ., 2021 ). Lipid vesicles have also been used for some time and they are now used for glycosylation of proteins in cell‐free systems (Hershewe et al ., 2021 ). Among the various hurdles still remaining to resolve are the synthesis of the lipid bilayer of the cell‐free systems membranes (Bhattacharya et al ., 2021 ) as well as routine synthesis of active membrane proteins. Novel transcription–translation systems are now able to express integral membrane proteins. This allowed researchers to highlight the importance of lipid–protein interaction in maintaining structural–functional integrity of ion channels (Vaish et al ., 2018 ). Membrane‐augmented cell‐free systems have been used in a wide array of cellular processes from primary and secondary metabolite synthesis to electron transport and one‐carbon metabolism (Kruyer et al ., 2021 ).
Following on from Spiegelman’s early experiments, an important step in understanding the boundary between in vivo and in vitro syntheses, the synthesis of a fully active virus has been achieved (Rustad et al ., 2017 ). While viruses cannot be considered alive – they need a live cell to multiply – the very fact that they can be made in vitro blurs further the boundary between chemistry and biology. Still, the cell‐free systems used to achieve this milestone are extracted from living cells, and are far from sufficient to be able to duplicate spontaneously. Nevertheless, this achievement paves the road to identify the biological functions that are responsible for the signature ‘animation’ of live cells (Danchin, 2021 ). However, much work remains to be developed to implement them knowingly in cell‐free systems. We can expect that this will be understood in a not too far away future, in particular if we understand better the role of apparently expletive energy‐dissipating functions, such as those involved in the control of folding and aggregation (Boel et al ., 2019 ; Dewachter et al ., 2021 ).
Streamlined cell‐free syntheses
In parallel with these advances, we are now in a new age of cell‐free biology, allowing applications of three variations on the theme: Not only authentic cell‐free transcription–translation systems, as just discussed but also protein‐based and nucleic acids‐based systems (Noireaux and Liu, 2020 ). Protein‐based streamlined cell‐free systems can be used to produce fine chemicals as well as precursors of heavy industry‐relevant compounds. As these processes do not require the industrial setup to sustain life, they tolerate the presence of otherwise toxic molecules (Rasor et al ., 2021 ). Among these, a very innovative approach has been explored early on. Rather than reconstituting in vitro the conditions prevailing in vivo , the idea, intended to improve industrial metabolic engineering, was to find a way of isolating all the enzymes needed to put together a metabolic pathway in a common container. Interestingly, the container could be of considerable size, an industrial reactor, for example. It is obviously time‐consuming to synthesize and purify individually all the enzymes of a pathway of interest. Why not use the established outcome of natural selection, with all enzymes in correct proportions in relevant cells, while getting rid of all components not required in the pathway? The operation would develop into two steps: (i) growing cells at a high density under conditions when the pathway is fully functional and boosted for production of the metabolites of interest; and (ii) making a whole extract of the cells, finding some intelligent ways to separate the metabolic fraction from the bulk of the cell's machinery, and then finding a way to keep in a reactor only those enzymes that are required in the pathway.
This can be achieved after ‘parasitic’ reactions have been identified if one can discard the parasitic elements. Panke and co‐workers proposed an astute approach to solve this quandary. In a first analysis, they boosted, via standard SynBio approaches, the pathway of interest. So doing, they also monitored all the changes that went up in parallel, drawing key resources away from the required pathway (Bujara and Panke, 2012 ). This interference was mediated by proteins, mainly enzymes and their regulators. How to get rid of them? Inactivating the corresponding genes directly would jeopardize production via destroying the network of interactions that make the cell functional. However, it is possible to tag the corresponding proteins by including in their genes, at positions that do not interfere with function (Oesterle et al ., 2017 ), sites that code for protease‐sensitive peptide motifs. In this way, when the cell extract is obtained, it can be incubated with a protease acting specifically on the tagged regions and, thus, removing unwanted proteins. After a purification step, the extract essentially contains the enzymes of the pathway of interest and, with adequate stabilization, can be used in a chemical reactor.
In line with this approach, another family of advances is being developed. When extracted directly from living cells, cell‐free systems always develop endogenous metabolic activity. The details of this activity are not yet understood but, as just de v eloped, they interfere with the production of the compounds of interest. Using metabolomics to characterize time‐dependent metabolic changes in cell‐free systems and their components, including significant metabolic activity in central carbon and amino acid metabolism, Styczynski and colleagues developed several approaches to prepare extracts prior to production development. They observed that while changing the starting state of the reaction via pre‐incubation of the lysate has an impact on protein production, its impact on the metabolic state was comparatively small. Changes in lysate preparation had a greater effect on protein yield and time‐dependent metabolic profiles, while general metabolic trends were maintained. Finally, while targeted supplementation of metabolic enzymes improved protein production, endogenous metabolic activity remained resistant to these enzymatic perturbations, calling for further development to improve the processes of interest (Miguez et al ., 2021 ). We can expect that in the next decade or so these families of approaches, combined in a variety of sequential reactors, including some involved in standard chemistry, will create novel designs for fine chemical syntheses.
Evolution in vitro
Another crucial family of experiments in cell‐free systems is the exploration of evolution, a feature recognized as critical to biology and commonly exploited in vivo . It is indeed possible to develop evolution experiments in cell‐free conditions. As an illustration, Spiegelman again took an aliquot of the Qβ RNA synthesized in the test tube and added it to a second identical text tube, then repeated the procedure 15 times. At the end of this sequence, the original RNA was almost completely diluted out by the transfers, leaving in the final tube RNA that was all generated in vitro . Repeating these experiments allowed him to witness the evolution of RNAs. By varying the selective pressure on the system (such as temperature, nutrient mix or time allowed for reproduction), he could generate mutant RNA molecules with a wide range of properties. One of those, for instance, gradually got trimmed down from the original 3300 nucleotides to 470 – just sufficient to bind the replicase and replicate the RNA without further loss of its sequence. This small molecule had shed sections that coded for the virus' protein coat and other components it did not need in the artificial environment (Levisohn and Spiegelman, 1969 ). It should be noted that processes similar to this cell‐free evolution also occur in vivo and are important for recombination, for example, with existing viruses such as SARS‐CoV‐2. This largely explains the ability of viruses to evade innate and acquired immunity, as observed several decades ago (Meyer and Southern, 1997 ). In parallel with functional copies of the authentic virus, cells keep shedding short counterparts that essentially kept the 3' and 5' end required to initiate and terminate replication (Gribble et al ., 2021 ). This indicates that the process of viral replication is prone to produce a large number of variants that could be exploited for use in cell‐free expression systems. Based on this early work and recent observations, advances in the in vitro synthesis and evolution of DNA, RNA and polypeptides have already been designed to accelerate the construction of biopolymers, pathways and organisms with new functions (Forster and Church, 2007 ). This trend is set to continue.
A most important sequel of Spiegelman’s early experiment has been the systematic evolution of ligands by exponential enrichment (SELEX) procedure devised by Larry Gold and his colleagues (Tuerk et al ., 1992 ). After the discovery of ribozymes, it was clear that RNA molecules could fold into complex structures able to bind foreign molecules [aptamers, riboswitches and ribozymes (Ge and Marchisio, 2021 )]. This prompted exploration of novel RNA‐based activities, substrate‐dependent ribozymes in particular. Using a variety of ad hoc selection procedures, SELEX allowed isolating functional RNAs from a pool of random sequences of RNA, in particular endowed of catalytic activities. Among the critical functions, as discussed above, the mimicry of aminoacyl‐tRNA synthetases by ribozymes was an interesting target. Perhaps the first example of ribozymes capable of charging certain amino acids onto RNA was developed by Yarus and co‐workers. They isolated synthetic ribozymes that catalyse aminoacylation of their own CCG‐terminal 2’(3’)‐OH with phenylalanyl‐AMP or tyrosyl‐AMP as an aminoacylation donor (Illangasekare et al ., 1995 ). However, this early class of ribozymes was still unable to aminoacylate the CCA 3’ end of a tRNA. Using a first family of ribozymes, Suga and co‐workers designed variants of an artificial ribozyme able to bind CCA motifs and comprising random segments. These RNA molecules were submitted to a selection procedure meant to isolate those which got the ability to aminoacylate various tRNAs with phenylalanine or non‐natural derivatives (Fig. 2 ). One of those was able to aminoacylate its cognate tRNA with a high degree of specificity, while failing to aminoacylate non‐cognate tRNAs. Further evolution in vitro allowed the authors to load analogues of phenylalanine of a specific tRNA. Changing the tRNA load specificity could be easily programmed into such flexizymes, fashioning them into custom‐made catalysts to generate non‐natural aminoacyl‐tRNAs (Ramaswamy et al ., 2004 ). This family of advances, based on in vitro RNA evolution, is likely to see considerable developments in the near future (Kofman et al ., 2021 ).
All these advances require complex laboratory facilities, with development time limited by the constraints of physical experiments, hours, days, months or even years. As with a considerable number of existing technological developments, it is now possible to replace approaches that involve wet laboratories with computer‐based exploration. State‐of‐the‐art computer modelling and design technologies allow us to achieve results that engineers could not even dream of a couple of decades ago. For example, the manufacture of environmentally interesting devices starts with the succession, concept, model, computer‐aided testing of functions and resilience, and finally the creation of a prototype, with feedback to material experiments. The conceptual development of an aircraft starts from a creative search stage: choice of style, composite approach, creation of sketches and drawing of the future model, and all this can be done with the help of computers (Abbasov, 2019 ). While laboratory experiments were the rule rather than the exception, it soon became apparent that, starting with data analysis and storage, true in silico experiments – using computers – could and should be developed.
Synthetic biology in silico
In silico biology is now a major domain of SynBio developments, with one caveat. The exponential development of computers and the speed of related advances relative to the time required to obtain results from laboratory experiments have led to the creation of a large community of researchers and engineers who claim to be able to substitute in silico biology for experimental (‘wet’) biology. Alas, this is often at the cost of understanding what life is. The main consequence of the shift from in vivo / in vitro to in silico research is that a significant fraction of the community has turned inward, with its own journals and evaluation rules, reviewing and evaluating the work it produces without the critical steps that would require predictions or explanations – validation – based on authentic biological experiments. Furthermore, the very fact that in silico biology – modelling in particular – requires skills in both mathematics/computer sciences and biology means that a great many studies escape proper evaluation (when they are about biology, they are considered to be computer sciences, and vice versa). This results in a large amount of work which is not relevant for answering the questions posed by SynBio (Danchin et al ., 2018 ). This downside has a considerable impact when we have to follow the explosion of articles in the domain. It is virtually impossible to go beyond scraping the surface of the amount of literature claiming to be in silico biology. At the risk of missing important work, we have tried here to avoid this pitfall by giving an overview of a limited number of the work and trends that may provide constructive developments in the near future.
Mathematics/computer‐based models of a variety of processes essential to life have long been developed, with two different aims. For diagnostic purposes, models were meant to produce a phenomenological account of the dynamics of processes of interest. The purpose of such simulations was not to understand their causes but to allow researchers to make predictions about the short‐term and sometimes long‐term outcome of the processes. This family of approaches is well established. It is still witnessing substantial developments, in particular in the domain of health‐related studies.
Simulation vs. explanation
In the context of SynBio, simulations can be used as a first pass meant to measure whether a particular family of experiments behaves consistently as predicted, thus substantiating the relevance of the collected data and pointing out missing elements. The power of this family of models parallels the continuous advances of computing capacities associated with the continuous emergence of faster computers. In recent times, artificial intelligence (AI) approaches based on deep learning flourished as tools of choice in the domain of diagnosis (Akay and Hess, 2019 ). Many AI‐based models are currently limited to diagnosis because successful predictions are not sufficient to tell correlation from causality, in particular when the underlying reasons for a prediction ‘success’ cannot be traced easily. To make the most of these models and use them as help to discovery requires that the outcome of their operation can be traced back to a causality chain. This restriction explains why legal regulatory instances have now asked creators of AI‐based models to be able to highlight the internal chain of causality in their successful models. This is understood as a way to associate prediction with understanding [ https://eur‐lex.europa.eu/legal‐content/EN/TXT/?uri=CELEX:52021PC0206 and for an example of the way understanding can be visualized in an AI model, see Prifti et al . ( 2020 )]. This limitation should be extended to a vast number of models. Here is an illustration of the difference between phenomenology (simulations) and identification of causality (rational scientific modelling).
The behaviour of cells on plates is often used to illustrate SynBio construction [see a famous early illustration in (Elowitz and Leibler, 2000 )]. However, a large number of phenomenological models can be used to account for this behaviour (McCallum and Potvin‐Trottier, 2021 ). For example, it has long been observed that colonies on plates often generate patterns that have dendritic shapes (Fall et al ., 2004 ). This is generally ‘explained’ by models involving repulsion, multi‐scale patterns, self‐organization and all kinds of buzzwords [see, e.g. Deng et al . ( 2014 )]. Yet, analysis of the causal chain of events that lead to these patterns demonstrates that the explanation of the apparent repulsion is not mediated by any ‘self‐organization’ magic force. Notwithstanding a possible Marangoni effect, if movement involves surfactants and quorum sensing (Daniels et al ., 2006 ), ‘repulsion’ is driven by the – almost trivial – role of the nutrient source in the cell’s behaviour: Bacteria need nutrients to multiply. They evolved a chemotactic response that makes them move towards nutrient sources (and, therefore, avoid regions where they have been depleted). Understanding the authentic chain of causality is important: What appears to the naïve mind as a self‐organized repulsion is in fact the distribution of moving cells in regions where nutrients are available (Sekowska et al ., 2009 ). This experiment illustrates the dichotomy of modelling, which can lead either to phenomenology or to understanding; the latter being the only way to generate real advances. To move beyond the phenomenology of simulations, one must interpret the data critically, looking for genuine causality and refraining from appealing to folk magic.
Standardization
To make the most of computer‐mediated exploration, it is critical that models are built on very precise definitions and cardinal foundations, not on fuzzy vocabulary. This topic, in particular, the need of accurate definitions, is discussed in details by Clark and Hicks in the field of chemistry, showing how the choice of models impacts the outcomes of research, including with modern AI approaches (Clark and Hicks, 2020 ). Because models rest on the collection of relevant data, the key question of standardization and nomenclature must be asked, with expedient approaches (Decoene et al ., 2018 ). A great many developments of SynBio are based on manipulation of nucleic acids. Because these polymers are made of the chaining of elementary building block, they are generally handled by computers as are abstract linear alphabetic texts. Intelligent storage and analysis of nucleic acid and also protein sequence data began four decades ago (Blumenthal et al ., 1982 ). Subsequently, an explosion of applications resulted in the creation of a new domain of biology, bioinformatics, with a slight change in the initial meaning of the word, now often restricted to genome‐based studies (Hogeweg, 2011 ). Yet, while this restriction is commonplace, the advent of genomics, with its related ‘omics’ studies (Yadav, 2007 ), has considerably expanded the domain, with only fuzzy boundaries with recently fashionable domains such as AI and image analysis (Burian et al ., 2021 ).
Gene, genome and some transcriptomics data are collected, managed and released in a highly standardized way by the International Nucleotide Sequence Database Collaboration (DNA Database of Japan, Mishima, Japan; European Nucleotide Archive, Hinxton, UK; and GenBank, Bethesda, USA), which has developed standards for more than three decades (Arita et al ., 2021 ), and controlled vocabulary is implemented for proteins by UniProtKB (Feuermann et al ., 2021 ). The Gene Ontology Consortium maintains a knowledge base that ‘ develops a comprehensive, computational model of biological systems, ranging from the molecular to the organism level, across the multiplicity of species in the tree of life ’, also based on controlled vocabulary (Gene Ontology Consortium, 2021 ). However, while the nomenclature of chemicals and chemical reactions tend to follow well‐accepted rules [such as in CheBi (Hastings et al ., 2016 ) or Rhea (Lombardot et al ., 2019 ) for example], with efforts to allow interoperability (Navelkar et al ., 2021 ), the nomenclature of enzymes – besides the EC number standard – is still quite variable. Gene names are considerably dependent on the goodwill of authors and this has considerable negative impact on sequence annotation (and, therefore, discovery). Also, the data structure of specialized databases is still extremely disparate, which prevents interoperability (Danchin et al ., 2018 ). When dealing with SynBio, the situation is possibly less disappointing. The iGEM repository of Biobricks ( https://biobricks.org ) and, in Europe, the SEVA effort (Martínez‐García et al ., 2020 ) are pushing hard for widespread standardization. A further incentive for an international coordinated effort in this direction would be more than welcome to support the development of SynBio in the next decades.
All the same, three major domains of in silico research for SynBio develop in parallel: ‘omics‐based’ SynBio, whole‐cell models and in silico integration of metabolic pathways.
‘Omics‐based’ synthetic biology
Besides the domain of genomics, born and diversifying into a number of subdomains, the fields of transcriptomics, proteomics and metabolomics that progressively sprouted out of this original domain (Tyo et al ., 2010 ; Pühler, 2012 ) are now key for SynBio developments. The high‐dimensional datasets generated by these experimental approaches require considerable storage capacity and widespread sharing. A large number of analysis methods must also be made available. Sometimes it is possible to combine both storage and availability of analysis methods. In the case of bacterial genome data, this is the case, for example, with the MicroScope platform and its implementation of the MaGe methods (Vallenet et al ., 2020 ). Repositories such as GitHub with its community of open‐source developers are progressively gaining more and more impact (see, e.g. its SynBio entry: https://github.com/topics/synthetic‐biology ). Cloud computing provides generally low‐cost and highly flexible solutions in the domain of molecular modelling, omics data analytics (e.g. RNA sequencing, metabolomics or proteomics datasets) and for the integration, analysis and interpretation of phenomes (Koppad et al ., 2021 ). Merging highly heterogeneous datasets has been a prime goal early on (Arakawa and Tomita, 2013 ). This is critical because multi‐omics may be used to improve systematic genome manipulation for novel synthetic constructs (Fontana et al ., 2020 ) or improve industrial processes. Among the many illustrations of the approaches for SynBio applications, we may notice fine cellulose biosynthesis (Ryngajłło et al ., 2020 ), treatment of natural biomass, in particular lignocellulose (Xie et al ., 2014 ; Chen and Dou, 2016 ), or in the present context of general concern triggered by climate change, genome manipulation carried out for the metabolic engineering of Cyanobacteria (Hagemann and Hess, 2018 ; Ng et al ., 2020 ).
The data introduced in modern omics analyses allow for efficient characterization of the genetic, regulatory and metabolic phenotypes of engineered microbes. Yet, designing genetic interventions to achieve the desired phenotype remains a challenge. With recent developments in genetic engineering techniques, the time frames associated with constructing and testing strain designs have been greatly reduced through in silico modelling. This has created an efficient design–build–test–learn loop iteration cycle between experiment and analysis. However, the scale and complexity associated with multi‐omics datasets still require manual biological reasoning about the mechanisms driving phenotypic changes. As a consequence, the use of traditional statistical approaches can still reduce the dimensionality of these datasets and help compare strains of interest and improve metabolic engineering (Danchin et al ., 2018 ; St John and Bomble, 2019 ).
Besides modelling individual cells, modelling is also used to understand how microbial communities and their synthetic counterparts may form stable ensembles. Models allow researchers to better monitor the changes in microbiome composition and, for example, how this affects disease onset and development. Construction of models that integrate genomics, transcriptomics, proteomics and metabolomics allows researchers to visualize the meta‐metabolism of the community. Constraint programming (Brown and Miguel, 2006 ) is used to couple meta‐metabolism and multi‐omics analyses (Ebrahim et al ., 2013 ). As an illustration, COBRA modelling combined with meta‐omics analyses and multivariate statistical analyses are used as a tool to add value to clinical trials and ultimately propose therapeutic interventions (Heinken et al ., 2021 ). This type of work, when submitted to relevant constraints, allowed construction of a synthetic gut microbiome (Mabwi et al ., 2021 ).
Finally, we have seen that miniaturization was involved in developing novel approaches for parallel evolution of microbial cultures. This domain is also used to explore the consequences of the use of novel technologies based on new materials, for example, nanomaterials, which, because of their size, may be a matter of concern. Modelling using multi‐omics approaches explored the impact of nanomaterials on cells or pathways via interpretation of omics data (Mortimer et al ., 2021 ).
Simulating whole‐cell behaviour
In parallel with the sequencing of whole genomes, researchers proposed to shift efforts from in vivo biology to reconstitution of whole cell in computer‐mediated models. Early attempts focused on E. coli at a time when DNA sequencing was not yet available (Weinberg and Berkus, 1971 ). The revolution brought about by sequencing resulted in a nucleic acid‐, then genome‐centric view of cells, but models were initially restricted to simulations based on the way macromolecule and intermediary metabolism could integrate functions previously identified from the study of individual genes.
As an early illustration of the integrated behaviour of cells, modelling was developed around a generic software environment that would explore, from the knowledge of their genome, how cells behave in all their biological properties. A first simulation software pipeline, E‐Cell, was made publicly available in 1997, poised to exploit the then recently acquired genome data of Mycoplasma genitalium (Tomita et al ., 1997 ). This generic software environment for simulating a cell was subsequently improved to conduct in silico experiments based on numerical data obtained from in vivo experiments involving other organisms (Tomita et al ., 1997 ; Tomita, 2001 ; Ishii et al ., 2004 ). Among applications of the software was the exploration of an osmoregulatory switch in the growth properties of E. coli (Srividhya and Krishnaswamy, 2004 ) and the deciphering of red blood cells’ metabolism (Yachie‐Kinoshita et al ., 2010 ). The domain of mathematics involved in computation was based on differential equations. This preference is not without consequences, as it implies that biological objects are assumed to display a continuous behaviour, with their concentration represented as a real number not as a discontinuous integer. While this is good enough for objects having a concentration in the millimolar range or above, this is inappropriate when individual objects must be identified, such as regulatory molecules. For example, regulators or co‐factors have often a concentration in the sub‐micromolar range, or are present in cells such as an individual E. coli in less than a handful of individual molecules. Ten years after its start, the E‐Cell project experienced a thorough reorganization, now focusing on simulations meant to help industrial applications. This focus on simulation rather than understanding may explain why, after a series of improvements, the project, while still existing ( https://www.e‐cell.org/about.html ) progressively stalled. At present, the whole‐cell modelling of E. coli follows another turn, still based on standard approaches but with some input of stochasticity to take into account entities that are present at a low number in cells (Sun et al ., 2021 ).
More fitting to SynBio approaches, whole‐cell models may be used as a basis for genome design based on previous knowledge. The bacterium Mycoplasma genitalium is represented by an updated whole‐cell model ( https://github.com/CovertLab/WholeCell ). At this time, this framework combines 28 cellular submodels, integrating all the known functions of every gene and molecule in the cell, running individual models on multiple supercomputers (Rees‐Garbutt et al ., 2021 ). This design allows researchers to propose knockouts of individual protein‐coding gene, phasing out genes previously identified by their importance for growth and to test possible phenotypes to identify synthetic lethal associations. This semi‐empiric work depends on known phenotypes, conducting whole‐cell model simulations in three steps: design (the outputs of algorithms select possible gene deletions), simulation (behaviour of the genome minus those deletions) and testing (the behaviour of the resulting cell is analysed). Several simulations are conducted in parallel. Those that removed the most genes from the genome and produced cells that were still able to divide are kept for further study. Once this step is completed, the software proceeds to the next cycle, increasing the number of gene deletions and generating progressively smaller genomes which can be subsequently used as synthetic constructs. This bypasses the development of slower experimental approaches based on the construction of multiple knockout mutants, such as those which uncovered hidden reactions in E. coli (Nakahigashi et al ., 2009 ), showing how enzyme promiscuity could combine with paralogous metabolism (Chan et al ., 2014 ; Guzmán et al ., 2019 ) to generate unexpected pathways. Similar genome‐scale modelling is also developed using Eukarya such as Yarrowia (Czajka et al ., 2021 ).
Based on genome data, these models are very general but not realistic. Their properties of symmetry do not take into account the asymmetry of the cell’s geometry. Cells are generally not spheres, and taking into account the real time‐dependent distribution of their metabolites is far from straightforward. Furthermore, compartmentalization is a critical feature of the development of metabolism (de Lorenzo et al ., 2015 ; Bohrer and Xiao, 2020 ) and this implies that realistic models must make the most of representations that consider the cells’ shape and the way they divide. Exactly as illustrated previously with the case of populations of cells moving on plates, many phenomenological models try to fit with 3D observations to represent the cell’s geometry. Bacteria are often rod‐like structures. Analysis of the constraints that account for this family of shapes provides interesting views [see, e.g. Chang and Huang ( 2014 ); Banerjee et al . ( 2016 )]. Yet, the ultimately disparate causes resulting in bacteria being rods have a common source based on metabolism: Growth must put together compartments that have widely different spatial properties, while the core of metabolism that generates major building blocks is likely to grow in three dimensions. Indeed, growth of the cytoplasm is three dimensional, while membranes are two‐dimensional, and the genome is unidimensional. The major source of building blocks is expressed in the cytoplasm, and therefore, grows in three dimensions, unless specifically regulated or organized metabolic limitations prevent non‐homothetic growth of the various cell’s compartments. Remarkably, it is cytosine metabolism (and more generally de novo pyrimidine synthesis) that overcomes this obstacle, with all pyrimidine‐related pathways converging on the activity of CTP synthetase (Ou et al ., 2020 ; Danchin, 2021 ). The consequences of this metabolic adaptation have not yet been input in models of bacteria in silico , but this will have considerable impact for the future of SynBio metabolic engineering developments. Many microbes have a structure that departs from that of spheres or bacillus shapes. They make filaments during their growth, either under natural circumstances (e.g. streptomycetes and filamentous fungi), but they also make filaments under stressful conditions or during ageing. This phenomenon is important for cell factories, and phenomenological models have been proposed to understand and control filamentation, with the aim of designing the morphogenesis of fungi (Meyer et al ., 2021 ) or Cyanobacteria (Yamamoto et al ., 2021 ). The process of filamentation has been studied for a long time, but the link to metabolism has generally not been explored, apart from the identification of targets such as LexA in bacteria, with limited in silico modelling (Bellio et al ., 2020 ).
Finally, in yet another register, modelling was used to improve cell‐free protein synthesis, in a typical application of in silico approaches to complement in vitro experiments (Müller et al ., 2020 ). For example, the domain of heterologous protein expression benefited from approaches allowing codon optimization, a domain explored at the very beginning of genomics (Ang et al ., 2016 ). At a finer level of granularity, the evolution of proteins was modelled as a function of their abundance as well as environmental parameters such as temperature. Interestingly, in addition to their expression level, protein evolution is determined by the concentration of chaperones, highlighting the critical role of these neglected essential factors (Agozzino and Dill, 2018 ). More in line with larger scale physiological data, integration of a variety of approaches investigated the role of stress and helped in the improvement of recombinant protein synthesis (Chen et al ., 2021c ). At this point, we may understand that we are still a long way from having whole‐cell models that go far beyond simulation. Nevertheless, modelling can be successfully used to guide SynBio designs. Moreover, based on semi‐empirical approaches, intermediary metabolism is now more suitable for building predictive models that help streamline existing metabolism while exploring the novel avenues we mentioned earlier.
Whole‐cell metabolism
It is common to assume that metabolism follows textbook pathways. Yet, unexpected metabolic pathways are the rule rather than the exception and the metabolic capacity of virtually all organisms is vastly underappreciated (Medema et al ., 2021 ). How can we connect our knowledge of genomes and whole‐cell metabolism? Back in the XIX th century, August Weisman postulated that living organisms combined two major characters, with a germen , that was responsible for the hereditary properties of the organism and a soma that harboured the germen while displaying observable features. This distinction is still valid and recognized as the genotype/phenotype dichotomy. With the advent of DNA sequencing and identification of the genome sequence, this dichotomy is now often referred to as the genome/phenome question, and this is the subject of explicit in silico investigations (Norsigian et al ., 2020 ). Metabolic engineering, developed along an integrated view of the genome/phenome dichotomy, associates traditional metabolic engineering (Bailey, 1991 ) with systems biology, synthetic biology and evolutionary engineering (Jang et al ., 2019 ). It is enabling the development of microbial cell factories capable of producing efficiently a myriad of chemicals and materials, including biofuels, bulk and fine chemicals, polymers, amino acids, natural products and drugs. These developments are based on integrated circuits within metabolic networks, preferably using multi‐omics high‐throughput data collection (Chen and Li, 2016 ).
Combined with genome engineering and genome‐wide metabolic simulations, as discussed above, many tools and strategies have been developed to generate microbial cell factories (Ko et al ., 2020 ). Here, we focus on a family of models that can be used as predictive tools to assess metabolic constructs. Based on flux balance analysis [FBA, (Edwards and Palsson, 1998 )], genome‐scale metabolic models [GSMM (Edwards and Palsson, 1999 ; Xu et al ., 2013 )] have been developed to explore the metabolism of a large number of microorganisms of industrial interest, such as P. putida (Belda et al ., 2016 ) or B. subtilis (Belda et al ., 2013 ). Being based on the general constraints of stoichiometry (Moyer et al ., 2021 ), FBA is generic and may accommodate the network structure of any metabolic pathway (Antoniewicz, 2021 ). For example, the details of the complex metabolism of actinomycetes are now clarified, allowing metabolic engineering in these organisms (Palazzotto et al ., 2019 ). Furthermore, these applications are not restricted to bacteria: Flux balance analysis guided the evolution of a yeast chassis (Pereira et al ., 2021 ).
Other genome‐wide metabolism models have been implemented to explore in silico the metabolism of short‐genome bacteria such as Mesoplasma florum . Using sequence and structure homology, the set of metabolic functions that its genome encodes was identified, allowing the reconstruction of a metabolic network representing one third of its protein‐coding genes. Simplification of the growth medium allowed quantification of substrate uptake and product secretion rates, which, together with the experimental biomass composition, were integrated as species‐specific constraints to produce a functional model of metabolism at the genome scale (Lachance et al ., 2021 ). At a larger scale, a whole‐cell modelling with emphasis on metabolism is continuously improved for the model E. coli (Sun et al ., 2021 ). This effort is particularly important because E. coli remains the best known organism, with continued progress in understanding the functions encoded in its genome, based on laboratory experiments.
Modelling of metabolism is also useful to explore communities of organisms (Vázquez‐Castellanos et al ., 2019 ). This family of approaches is particularly timely because we are beginning to understand, through the study of seawater, what metabolic ‘currencies’ are shared by the millions of microbes that coexist in this environment (Durham, 2021 ). Microbial co‐cultures have been studied using FBA, with interesting observations involving cross‐feeding (Konstantinidis et al ., 2021 ). Another type of interaction between microbes is witnessed by the role of viruses, which are able to reprogram the metabolism of their host (Jacobson et al ., 2021 ). Understanding the corresponding processes may allow construction of specific pathways for metabolic engineering.
Evolution in silico
Many evolutionary experiments use model organisms, but they are slow (Sekowska et al ., 2016 ; Grant et al ., 2021 ) and the study of evolution in silico remains the approach of choice. Adaptive evolution in the laboratory has been developed for organisms of industrial interest. The process benefits greatly from in silico modelling (Lee and Kim, 2020 ). In this context, exploration of the potential deleterious effects of synthetic mobile elements in genomes has been studied in silico (Zamdborg et al ., 2015 ) and early work was designed to predict the evolution of the virulence of bacteria of interest to agriculture (Strauß et al ., 2016 ). In line with the objectives of SynBio, based on families of enzyme variants, modelling is used to design synthetic counterparts with ad hoc catalytic properties (Broom et al ., 2020 ; Bunzel et al ., 2021 ). More generally, in silico modelling allows engineers to explore the evolutionary landscape along lines that would be impossible to follow in wet lab experiments, particularly when several alternative pathways are possible (Ambrus et al ., 2020 ). Furthermore, frequent evolution by gene duplication creates unexpected avenues that must be explored for the consequences of the regulatory properties they create (Marchant et al ., 2019 ).
Understanding gene networks is crucial for interpreting evolution and predicting evolutionary pathways (Gautam and Kumar Sinha, 2021 ). This task is difficult in the presence of noise, a pervasive feature, and requires special treatment (Vatsa and Agarwal, 2021 ). It is particularly important to understand the evolution of regulator binding sites. Comparative analysis of bacterial genomes has revealed that such sites are rapidly created at random during evolution, and then retained when they have a positive effect on the organism. Interestingly, the patterns of evolution depend on the nature of the regulator (Mrázek and Karls, 2019 ). A parallel software package, GeNESiS, was designed for the modelling and simulation of the evolution of gene regulatory networks (Kratz et al ., 2008 ). Various models of gene regulatory networks are available (Santibáñez et al ., 2020 ; Chen et al ., 2021b ), in particular dynamic models will be of interest to take evolution into account (Handzlik et al ., 2021 ). Analyses involving co‐evolution patterns via comparative genomics (Zhang et al ., 2021a ; Guo and Amir, 2021 ) will considerably benefit SynBio studies.
Conclusions
The development of SynBio is at a turning point. Over the past two decades or so, a large number of proofs of concept have been established, demonstrating the feasibility of a considerable number of SynBio developments. We are now at a point where even the most far‐fetched ideas can be implemented in constructions of considerable industrial interest. The integration of approaches combining experimentation with living chassis, cell‐free synthesis and modelling has created a virtuous cycle of design, construction, testing and learning loops between experiments and analysis, combining physiology, genetics, biochemistry and bioinformatics in a way that is constantly progressing. Perhaps the most promising approaches in terms of industrial production are experiments using building blocks that differ from those established during the evolution of life. It also seems important to open up strategies that combine biology and chemistry, for example, in factories where a succession of reactors combining in vivo fermenters and biochemical and chemical reactors creates a production line starting with low‐cost inputs and leading to high‐value products. At the same time, understanding the processes will greatly improve the safety of the facilities, with prevention locks in place that will foil the unwanted spread of chemical or biological contamination. In particular, it is essential to remember that the closer we get to existing living organisms, the more difficult it is to control their spread. Contrary to popular belief, artifice is much less dangerous than nature (Danchin, 2016 ).
Funding information
No funding information provided.
Conflict of interest
None declared.
Acknowledgements
This work benefited from discussions with members of the Stanislas Noria seminar.
Microbial Biotechnology (2021) 15 ( 1 ), 42–64 [ PMC free article ] [ PubMed ] [ Google Scholar ]
- Abbasov, I.B. (2019) Computer simulation in aircraft. In Computer Modeling in the Aerospace Industry . Abbasov, I.B. (ed). Hoboken, NJ: Wiley, pp. 1–21. [ Google Scholar ]
- Agozzino, L. , and Dill, K.A. (2018) Protein evolution speed depends on its stability and abundance and on chaperone concentrations . Proc Natl Acad Sci USA 115 : 9092–9097. [ PMC free article ] [ PubMed ] [ Google Scholar ]
- Akay, A. , and Hess, H. (2019) Deep learning: current and emerging applications in medicine and technology . IEEE J Biomed Health Inform 23 : 906–920. [ PubMed ] [ Google Scholar ]
- Aksu, D. , Diallo, M.M. , Şahar, U. , Uyaniker, T.A.L.P.A.Y. , and Ozdemir, G. (2021) High expression of ring‐hydroxylating dioxygenase genes ensure efficient degradation of p‐toluate, phthalate, and terephthalate by Comamonas testosteroni strain 3a2 . Arch Microbiol 203 : 4101–4112 [ PubMed ] [ Google Scholar ]
- Aldridge, J. , Carr, S. , Weber, K.A. , and Buan, N.R. (2021) Anaerobic production of isoprene by engineered Methanosarcina species Archaea . Appl Environ Microbiol 87 : e02417–e2420. [ PMC free article ] [ PubMed ] [ Google Scholar ]
- Ambrus, V. , Hoffka, G. , and Fuxreiter, M. (2020) Asymmetric dynamic coupling promotes alternative evolutionary pathways in an enzyme dimer . Sci Rep 10 : 18866. [ PMC free article ] [ PubMed ] [ Google Scholar ]
- Ang, K.S. , Kyriakopoulos, S. , Li, W. , and Lee, D.‐Y. (2016) Multi‐omics data driven analysis establishes reference codon biases for synthetic gene design in microbial and mammalian cells . Methods 102 : 26–35. [ PubMed ] [ Google Scholar ]
- Antoniewicz, M.R. (2021) A guide to metabolic flux analysis in metabolic engineering: methods, tools and applications . Metab Eng 63 : 2–12. [ PubMed ] [ Google Scholar ]
- Arakawa, K. , and Tomita, M. (2013) Merging multiple omics datasets in silico : statistical analyses and data interpretation . Methods Mol Biol 985 : 459–470. [ PubMed ] [ Google Scholar ]
- Arita, M. , Karsch‐Mizrachi, I. , and Cochrane, G. (2021) The international nucleotide sequence database collaboration . Nucleic Acids Res 49 : D121–D124. [ PMC free article ] [ PubMed ] [ Google Scholar ]
- Austin, S. , and McGeoch, D. (1973) The synthesis in vitro of RNA polymerase subunits of Escherichia coli . Proc Natl Acad Sci USA 70 : 2420–2423. [ PMC free article ] [ PubMed ] [ Google Scholar ]
- Bacher, J.M. , and Ellington, A.D. (2001) Selection and characterization of Escherichia coli variants capable of growth on an otherwise toxic tryptophan analogue . J Bacteriol 183 : 5414–5425. [ PMC free article ] [ PubMed ] [ Google Scholar ]
- Bailey, J.E. (1991) Toward a science of metabolic engineering . Science 252 : 1668–1675. [ PubMed ] [ Google Scholar ]
- Balabanova, L. , Averianova, L. , Marchenok, M. , Son, O. , and Tekutyeva, L. (2021) Microbial and genetic resources for cobalamin (Vitamin B12) biosynthesis: from ecosystems to industrial biotechnology . Int J Mol Sci 22 : 4522. [ PMC free article ] [ PubMed ] [ Google Scholar ]
- Banerjee, S. , Scherer, N.F. , and Dinner, A.R. (2016) Shape dynamics of growing cell walls . Soft Matter 12 : 3442–3450. [ PubMed ] [ Google Scholar ]
- Belda, E. , van Heck, R.G.A. , José Lopez‐Sanchez, M. , Cruveiller, S. , Barbe, V. , Fraser, C. , et al . (2016) The revisited genome of Pseudomonas putida KT2440 enlightens its value as a robust metabolic chassis . Environ Microbiol 18 : 3403–3424. [ PubMed ] [ Google Scholar ]
- Belda, E. , Sekowska, A. , Le Fèvre, F. , Morgat, A. , Mornico, D. , Ouzounis, C. , et al . (2013) An updated metabolic view of the Bacillus subtilis 168 genome . Microbiology 159 : 757–770. [ PubMed ] [ Google Scholar ]
- Bell, M.J. , and Lord, P. (2017) On patterns and re‐use in bioinformatics databases . Bioinformatics 33 : 2731–2736. [ PMC free article ] [ PubMed ] [ Google Scholar ]
- Bellio, P. , Mancini, A. , Di Pietro, L. , Cracchiolo, S. , Franceschini, N. , Reale, S. , et al . (2020) Inhibition of the transcriptional repressor LexA: Withstanding drug resistance by inhibiting the bacterial mechanisms of adaptation to antimicrobials . Life Sci 241 : 117116. [ PubMed ] [ Google Scholar ]
- Bhattacharya, A. , Cho, C.J. , Brea, R.J. , and Devaraj, N.K. (2021) Expression of fatty acyl‐CoA ligase drives one‐pot de novo synthesis of membrane‐bound vesicles in a cell‐free transcription‐translation system . J Am Chem Soc 143 : 11235–11242. [ PubMed ] [ Google Scholar ]
- Billerbeck, S. , Härle, J. , and Panke, S. (2013) The good of two worlds: increasing complexity in cell‐free systems . Curr Opin Biotechnol 24 : 1037–1043. [ PubMed ] [ Google Scholar ]
- Bitzenhofer, N.L. , Kruse, L. , Thies, S. , Wynands, B. , Lechtenberg, T. , Rönitz, J. , et al . (2021) Towards robust Pseudomonas cell factories to harbour novel biosynthetic pathways . Essays Biochem 65 : 319–336. [ PMC free article ] [ PubMed ] [ Google Scholar ]
- Blumenthal, R.M. , Rice, P.J. , and Roberts, R.J. (1982) Computer programs for nucleic acid sequence manipulation . Nucleic Acids Res 10 : 91–101. [ PMC free article ] [ PubMed ] [ Google Scholar ]
- Boehm, C.R. , Freemont, P.S. , and Ces, O. (2013) Design of a prototype flow microreactor for synthetic biology in vitro . Lab Chip 13 : 3426–3432. [ PubMed ] [ Google Scholar ]
- Boel, G. , Danot, O. , de Lorenzo, V. , and Danchin, A. (2019) Omnipresent Maxwell’s demons orchestrate information management in living cells . Microb Biotechnol 12 : 210–242. [ PMC free article ] [ PubMed ] [ Google Scholar ]
- Bohrer, C.H. , and Xiao, J. (2020) Complex diffusion in bacteria . Adv Exp Med Biol 1267 : 15–43. [ PMC free article ] [ PubMed ] [ Google Scholar ]
- Bose, S. , Aggarwal, S. , Singh, D.V. , and Acharya, N. (2020) Extracellular vesicles: an emerging platform in gram‐positive bacteria . Microb Cell 7 : 312–322. [ PMC free article ] [ PubMed ] [ Google Scholar ]
- Bradley, C.C. , Gordon, A.J.E. , Halliday, J.A. , and Herman, C. (2019) Transcription fidelity: new paradigms in epigenetic inheritance, genome instability and disease . DNA Repair (Amst) 81 : 102652. [ PMC free article ] [ PubMed ] [ Google Scholar ]
- Broom, A. , Rakotoharisoa, R.V. , Thompson, M.C. , Zarifi, N. , Nguyen, E. , Mukhametzhanov, N. , et al . (2020) Ensemble‐based enzyme design can recapitulate the effects of laboratory directed evolution in silico . Nat Commun 11 : 4808. [ PMC free article ] [ PubMed ] [ Google Scholar ]
- Brown, K.N. , and Miguel, I. (2006) Uncertainty and change. In Handbook of Constraint Programming . Foundations of Artificial Intelligence. Rossi, F. , van Beek, P. , and Walsh, T. (eds). Amsterdam: Elsevier Science, pp. 731–760. [ Google Scholar ]
- Bujara, M. , and Panke, S. (2012) In silico assessment of cell‐free systems . Biotechnol Bioeng 109 : 2620–2629. [ PubMed ] [ Google Scholar ]
- Bunzel, H.A. , Anderson, J.L.R. , and Mulholland, A.J. (2021) Designing better enzymes: insights from directed evolution . Curr Opin Struct Biol 67 : 212–218. [ PubMed ] [ Google Scholar ]
- Burian, A.N. , Zhao, W. , Lo, T.‐W. , and Thurtle‐Schmidt, D.M. (2021) Genome sequencing guide: an introductory toolbox to whole‐genome analysis methods . Biochem Mol Biol Educ 49 : 815‐825. [ PMC free article ] [ PubMed ] [ Google Scholar ]
- Burrington, L.R. , Watts, K.R. , and Oza, J.P. (2021) Characterizing and improving reaction times for E. coli ‐based cell‐free protein synthesis . ACS Synth Biol 10 : 1821–1829. [ PubMed ] [ Google Scholar ]
- Calero, P. , and Nikel, P.I. (2019) Chasing bacterial chassis for metabolic engineering: a perspective review from classical to non‐traditional microorganisms . Microb Biotechnol 12 : 98–124. [ PMC free article ] [ PubMed ] [ Google Scholar ]
- Caruthers, M.H. (2013) The chemical synthesis of DNA/RNA: our gift to science . J Biol Chem 288 : 1420–1427. [ PMC free article ] [ PubMed ] [ Google Scholar ]
- Caschera, F. , and Noireaux, V. (2014) Synthesis of 2.3 mg/ml of protein with an all Escherichia coli cell‐free transcription‐translation system . Biochimie 99 : 162–168. [ PubMed ] [ Google Scholar ]
- Chan, C.M. , Danchin, A. , Marliere, P. , and Sekowska, A. (2014) Paralogous metabolism: S‐alkyl‐cysteine degradation in Bacillus subtilis . Environ Microbiol 16 : 101–117. [ PubMed ] [ Google Scholar ]
- Chang, F. , and Huang, K.C. (2014) How and why cells grow as rods . BMC Biol 12 : 54. [ PMC free article ] [ PubMed ] [ Google Scholar ]
- Chaput, J.C. , and Herdewijn, P. (2019) What is XNA? Angew Chem Int Ed Engl 58 : 11570–11572. [ PubMed ] [ Google Scholar ]
- Chen, B.‐S. , and Li, C.‐W. (2016) Constructing an integrated genetic and epigenetic cellular network for whole cellular mechanism using high‐throughput next‐generation sequencing data . BMC Syst Biol 10 : 18. [ PMC free article ] [ PubMed ] [ Google Scholar ]
- Chen, C. , Cui, Z. , Zhao, J. , Li, S. , Ren, X. , Chen, T. , and Wang, Z. (2021a) Improving diacetyl production in Corynebacterium glutamicum via modifying respiratory chain . J Biotechnol 332 : 20–28. [ PubMed ] [ Google Scholar ]
- Chen, C. , Hou, J. , Shi, X. , Yang, H. , Birchler, J.A. , and Cheng, J. (2021b) GNET2: an R package for constructing gene regulatory networks from transcriptomic data . Bioinformatics 37 : 2068–2069. [ PubMed ] [ Google Scholar ]
- Chen, R. , and Dou, J. (2016) Biofuels and bio‐based chemicals from lignocellulose: metabolic engineering strategies in strain development . Biotechnol Lett 38 : 213–221. [ PubMed ] [ Google Scholar ]
- Chen, X. , Li, C. , and Liu, H. (2021c) Enhanced recombinant protein production under special environmental stress . Front Microbiol 12 : 630814. [ PMC free article ] [ PubMed ] [ Google Scholar ]
- Choi, J.‐M. , Holehouse, A.S. , and Pappu, R.V. (2020) Physical principles underlying the complex biology of intracellular phase transitions . Annu Rev Biophys 49 : 107–133. [ PMC free article ] [ PubMed ] [ Google Scholar ]
- Clark, B.F. , and Marcker, K.A. (1966) The role of N‐formyl‐methionyl‐sRNA in protein biosynthesis . J Mol Biol 17 : 394–406. [ PubMed ] [ Google Scholar ]
- Clark, J.M. , Chang, A.Y. , Spiegelman, S. , and Reichmann, M.E. (1965) The in vitro translation of a monocistronic message . Proc Natl Acad Sci USA 54 : 1193–1197. [ PMC free article ] [ PubMed ] [ Google Scholar ]
- Clark, T. , and Hicks, M.G. (2020) Models of necessity . Beilstein J Org Chem 16 : 1649–1661. [ PMC free article ] [ PubMed ] [ Google Scholar ]
- de Crécy, E. , Metzgar, D. , Allen, C. , Pénicaud, M. , Lyons, B. , Hansen, C.J. , and de Crécy‐Lagard, V. (2007) Development of a novel continuous culture device for experimental evolution of bacterial populations . Appl Microbiol Biotechnol 77 : 489–496. [ PubMed ] [ Google Scholar ]
- de Crécy‐Lagard, V.A. , Bellalou, J. , Mutzel, R. , and Marlière, P. (2001) Long term adaptation of a microbial population to a permanent metabolic constraint: overcoming thymineless death by experimental evolution of Escherichia coli . BMC Biotechnol 1 : 10. [ PMC free article ] [ PubMed ] [ Google Scholar ]
- Cui, J. , Sun, T. , Chen, L. , and Zhang, W. (2021) Salt‐tolerant Synechococcus elongatus UTEX 2973 obtained via engineering of heterologous synthesis of compatible solute glucosylglycerol . Front Microbiol 12 : 650217. [ PMC free article ] [ PubMed ] [ Google Scholar ]
- Czajka, J.J. , Oyetunde, T. , and Tang, Y.J. (2021) Integrated knowledge mining, genome‐scale modeling, and machine learning for predicting Yarrowia lipolytica bioproduction . Metab Eng 67 : 227–236. [ PubMed ] [ Google Scholar ]
- Danchin, A. (2016) Nature or manufacture: what should we fear most? C R Biol 339 : 329–335. [ PubMed ] [ Google Scholar ]
- Danchin, A. (2020) Isobiology: a variational principle for exploring synthetic life . ChemBioChem 21 : 1781–1792. [ PubMed ] [ Google Scholar ]
- Danchin, A. (2021) Three overlooked key functional classes for building up minimal synthetic cells . Synth Biol 6 : ysab010. [ PMC free article ] [ PubMed ] [ Google Scholar ]
- Danchin, A. , Binder, P.M. , and Noria, S. (2011) Antifragility and tinkering in biology (and in business) flexibility provides an efficient epigenetic way to manage risk . Genes (Basel) 2 : 998–1016. [ PMC free article ] [ PubMed ] [ Google Scholar ]
- Danchin, A. , Ouzounis, C. , Tokuyasu, T. , and Zucker, J.‐D. (2018) No wisdom in the crowd: genome annotation in the era of big data ‐ current status and future prospects . Microb Biotechnol 11 : 588–605. [ PMC free article ] [ PubMed ] [ Google Scholar ]
- Danchin, A. , and Sekowska, A. (2015) The logic of metabolism . Perspect Sci 6 : 15–26. [ Google Scholar ]
- Daniels, R. , Reynaert, S. , Hoekstra, H. , Verreth, C. , Janssens, J. , Braeken, K. , et al . (2006) Quorum signal molecules as biosurfactants affecting swarming in Rhizobium etli . Proc Natl Acad Sci USA 103 : 14965–14970. [ PMC free article ] [ PubMed ] [ Google Scholar ]
- Davies, P.S.W. (2020) Stable isotopes: their use and safety in human nutrition studies . Eur J Clin Nutr 74 : 362–365. [ PMC free article ] [ PubMed ] [ Google Scholar ]
- Decoene, T. , De Paepe, B. , Maertens, J. , Coussement, P. , Peters, G. , De Maeseneire, S.L. , and De Mey, M. (2018) Standardization in synthetic biology: an engineering discipline coming of age . Crit Rev Biotechnol 38 : 647–656. [ PubMed ] [ Google Scholar ]
- Deng, P. , de Vargas Roditi, L. , van Ditmarsch, D. , and Xavier, J.B. (2014) The ecological basis of morphogenesis: branching patterns in swarming colonies of bacteria . New J Phys 16 : 015006. [ PMC free article ] [ PubMed ] [ Google Scholar ]
- Dewachter, L. , Bollen, C. , Wilmaerts, D. , Louwagie, E. , Herpels, P. , Matthay, P. , et al. (2021) The dynamic transition of persistence toward the viable but nonculturable state during stationary phase is driven by protein aggregation . mBio 12 : e0070321. [ PMC free article ] [ PubMed ] [ Google Scholar ]
- Döring, V. , and Marlière, P. (1998) Reassigning cysteine in the genetic code of Escherichia coli . Genetics 150 : 543–551. [ PMC free article ] [ PubMed ] [ Google Scholar ]
- Dunkelmann, D.L. , Oehm, S.B. , Beattie, A.T. , and Chin, J.W. (2021) A 68‐codon genetic code to incorporate four distinct non‐canonical amino acids enabled by automated orthogonal mRNA design . Nat Chem . in press. [ PMC free article ] [ PubMed ] [ Google Scholar ]
- Durham, B.P. (2021) Deciphering metabolic currencies that support marine microbial networks . mSystems 6 : e0076321. [ PMC free article ] [ PubMed ] [ Google Scholar ]
- Ebrahim, A. , Lerman, J.A. , Palsson, B.O. , and Hyduke, D.R. (2013) COBRApy: COnstraints‐based reconstruction and analysis for python . BMC Syst Biol 7 : 74. [ PMC free article ] [ PubMed ] [ Google Scholar ]
- Edwards, J.S. , and Palsson, B.O. (1998) How will bioinformatics influence metabolic engineering? Biotechnol Bioeng 58 : 162–169. [ PubMed ] [ Google Scholar ]
- Edwards, J.S. , and Palsson, B.O. (1999) Systems properties of the Haemophilus influenzae Rd metabolic genotype . J Biol Chem 274 : 17410–17416. [ PubMed ] [ Google Scholar ]
- van Eldijk, M.B. , and van Hest, J.C.M. (2018) Residue‐specific incorporation of noncanonical amino acids for protein engineering . Methods Mol Biol 1728 : 137–145. [ PubMed ] [ Google Scholar ]
- Elowitz, M.B. , and Leibler, S. (2000) A synthetic oscillatory network of transcriptional regulators . Nature 403 : 335–338. [ PubMed ] [ Google Scholar ]
- Fall, R. , Kinsinger, R.F. , and Wheeler, K.A. (2004) A simple method to isolate biofilm‐forming Bacillus subtilis and related species from plant roots . Syst Appl Microbiol 27 : 372–379. [ PubMed ] [ Google Scholar ]
- Femmer, C. , Bechtold, M. , Held, M. , and Panke, S. (2020) In vivo directed enzyme evolution in nanoliter reactors with antimetabolite selection . Metab Eng 59 : 15–23. [ PubMed ] [ Google Scholar ]
- Feuermann, M. , Boutet, E. , Morgat, A. , Axelsen, K.B. , Bansal, P. , Bolleman, J. , et al . (2021) Diverse taxonomies for diverse chemistries: enhanced representation of natural product metabolism in UniProtKB . Metabolites 11 : 48. [ PMC free article ] [ PubMed ] [ Google Scholar ]
- Fiers, G. , Chouikhi, D. , Oswald, L. , Al Ouahabi, A. , Chan‐Seng, D. , Charles, L. , and Lutz, J.‐F. (2016) Orthogonal synthesis of xeno nucleic acids . Chemistry 22 : 17945–17948. [ PubMed ] [ Google Scholar ]
- Flechsler, J. , Heimerl, T. , Huber, H. , Rachel, R. , and Berg, I.A. (2021) Functional compartmentalization and metabolic separation in a prokaryotic cell . Proc Natl Acad Sci USA 118 : e2022114118. [ PMC free article ] [ PubMed ] [ Google Scholar ]
- Flood, D.T. , Hintzen, J.C.J. , Knouse, K.W. , Hill, D.E. , Lu, C. , Cistrone, P.A. , et al . (2021) Selenomethionine as an expressible handle for bioconjugations . Proc Natl Acad Sci USA 118 : e2005164118. [ PMC free article ] [ PubMed ] [ Google Scholar ]
- Fontana, J. , Sparkman‐Yager, D. , Zalatan, J.G. , and Carothers, J.M. (2020) Challenges and opportunities with CRISPR activation in bacteria for data‐driven metabolic engineering . Curr Opin Biotechnol 64 : 190–198. [ PubMed ] [ Google Scholar ]
- Forster, A.C. , and Church, G.M. (2007) Synthetic biology projects in vitro . Genome Res 17 : 1–6. [ PubMed ] [ Google Scholar ]
- Fritz, S.E. , Haque, N. , and Hogg, J.R. (2018) Highly efficient in vitro translation of authentic affinity‐purified messenger ribonucleoprotein complexes . RNA 24 : 982–989. [ PMC free article ] [ PubMed ] [ Google Scholar ]
- Fukuma, I. , and Cohen, S.S. (1975) Polyamines in bacteriophage R17 and its RNA . J Virol 16 : 222–227. [ PMC free article ] [ PubMed ] [ Google Scholar ]
- Garcia‐Morales, L. , Ruiz, E. , Gourgues, G. , Rideau, F. , Piñero‐Lambea, C. , Lluch‐Senar, M. , et al . (2020) A RAGE based strategy for the genome engineering of the human respiratory pathogen Mycoplasma pneumoniae . ACS Synth Biol 9 : 2737–2748. [ PubMed ] [ Google Scholar ]
- Garenne, D. , Libchaber, A. , and Noireaux, V. (2020) Membrane molecular crowding enhances MreB polymerization to shape synthetic cells from spheres to rods . Proc Natl Acad Sci USA 117 : 1902–1909. [ PMC free article ] [ PubMed ] [ Google Scholar ]
- Gautam, P. , and Kumar Sinha, S. (2021) Anticipating response function in gene regulatory networks . J R Soc Interface 18 : 20210206. [ PMC free article ] [ PubMed ] [ Google Scholar ]
- Ge, H. , and Marchisio, M.A. (2021) Aptamers, riboswitches, and ribozymes in S. cerevisiae synthetic biology . Life (Basel) 11 : 248. [ PMC free article ] [ PubMed ] [ Google Scholar ]
- Gene Ontology Consortium (2021) The Gene Ontology resource: enriching a GOld mine . Nucleic Acids Res 49 : D325–D334. [ PMC free article ] [ PubMed ] [ Google Scholar ]
- Gomes, E. , and Shorter, J. (2019) The molecular language of membraneless organelles . J Biol Chem 294 : 7115–7127. [ PMC free article ] [ PubMed ] [ Google Scholar ]
- González, L.M. , Mukhitov, N. , and Voigt, C.A. (2020) Resilient living materials built by printing bacterial spores . Nat Chem Biol 16 : 126–133. [ PubMed ] [ Google Scholar ]
- Grant, N.A. , Abdel Magid, A. , Franklin, J. , Dufour, Y. , and Lenski, R.E. (2021) Generations of experimental evolution with Escherichia coli . J Bacteriol 203 : e00469‐20. [ PMC free article ] [ PubMed ] [ Google Scholar ]
- Grasemann, L. , Lavickova, B. , Elizondo‐Cantú, M.C. , and Maerkl, S.J. (2021) OnePot PURE cell‐free system . J vis Exp . in press. [ PubMed ] [ Google Scholar ]
- Gribble, J. , Stevens, L.J. , Agostini, M.L. , Anderson‐Daniels, J. , Chappell, J.D. , Lu, X. , et al . (2021) The coronavirus proofreading exoribonuclease mediates extensive viral recombination . PLoS Pathog 17 : e1009226. [ PMC free article ] [ PubMed ] [ Google Scholar ]
- Grindley, J.N. , Blumberg, R. , and Nakada, D. (1977) Synthesis of R‐plasmid‐coded beta‐lactamase in minicells and in an in vitro system . J Bacteriol 130 : 852–859. [ PMC free article ] [ PubMed ] [ Google Scholar ]
- Grunberg‐Manago, M. (1963) Enzymatic synthesis of nucleic acids . Prog Biophys Mol Biol 13 : 175–239. [ PubMed ] [ Google Scholar ]
- Guo, Y. , and Amir, A. (2021) Exploring the effect of network topology, mRNA and protein dynamics on gene regulatory network stability . Nat Commun 12 : 130. [ PMC free article ] [ PubMed ] [ Google Scholar ]
- Guzmán, G.I. , Sandberg, T.E. , LaCroix, R.A. , Nyerges, Á. , Papp, H. , de Raad, M. , et al . (2019) Enzyme promiscuity shapes adaptation to novel growth substrates . Mol Syst Biol 15 : e8462. [ PMC free article ] [ PubMed ] [ Google Scholar ]
- Hagemann, M. , and Hess, W.R. (2018) Systems and synthetic biology for the biotechnological application of cyanobacteria . Curr Opin Biotechnol 49 : 94–99. [ PubMed ] [ Google Scholar ]
- Hammerling, M.J. , Krüger, A. , and Jewett, M.C. (2020) Strategies for in vitro engineering of the translation machinery . Nucleic Acids Res 48 : 1068–1083. [ PMC free article ] [ PubMed ] [ Google Scholar ]
- Handzlik, J.E. , Loh, Y.L. , and Manu, N. (2021) Dynamic modeling of transcriptional gene regulatory networks . Methods Mol Biol 2328 : 67–97. [ PubMed ] [ Google Scholar ]
- Haruna, I. , and Spiegelman, S. (1965) Autocatalytic synthesis of a viral RNA in vitro . Science 150 : 884–886. [ PubMed ] [ Google Scholar ]
- Hastings, J. , Owen, G. , Dekker, A. , Ennis, M. , Kale, N. , Muthukrishnan, V. , et al . (2016) ChEBI in 2016: improved services and an expanding collection of metabolites . Nucleic Acids Res 44 : D1214–1219. [ PMC free article ] [ PubMed ] [ Google Scholar ]
- He, H. , Höper, R. , Dodenhöft, M. , Marlière, P. , and Bar‐Even, A. (2020) An optimized methanol assimilation pathway relying on promiscuous formaldehyde‐condensing aldolases in E. coli . Metab Eng 60 : 1–13. [ PubMed ] [ Google Scholar ]
- Hecht, S.M. (2021) Expansion of the genetic code through the use of modified bacterial ribosomes . J Mol Biol 167211 . [ PMC free article ] [ PubMed ] [ Google Scholar ]
- Heinken, A. , Basile, A. , Hertel, J. , Thinnes, C. , and Thiele, I. (2021) Genome‐scale metabolic modeling of the human microbiome in the era of personalized medicine . Annu Rev Microbiol . in press. [ PubMed ] [ Google Scholar ]
- Hershewe, J.M. , Warfel, K.F. , Iyer, S.M. , Peruzzi, J.A. , Sullivan, C.J. , Roth, E.W. , et al . (2021) Improving cell‐free glycoprotein synthesis by characterizing and enriching native membrane vesicles . Nat Commun 12 : 2363. [ PMC free article ] [ PubMed ] [ Google Scholar ]
- Hogeweg, P. (2011) The roots of bioinformatics in theoretical biology . PLoS Comput Biol 7 : e1002021. [ PMC free article ] [ PubMed ] [ Google Scholar ]
- Hui, J.O. , Flick, T. , Loo, J.A. , and Campuzano, I.D.G. (2021) Unequivocal identification of aspartic acid and isoaspartic acid by MALDI‐TOF/TOF: from peptide standards to a therapeutic antibody . J Am Soc Mass Spectrom 32 : 1901‐1909. [ PubMed ] [ Google Scholar ]
- Hummels, K.R. , and Kearns, D.B. (2020) Translation elongation factor P (EF‐P) . FEMS Microbiol Rev 44 : 208–218. [ PMC free article ] [ PubMed ] [ Google Scholar ]
- Illangasekare, M. , Sanchez, G. , Nickles, T. , and Yarus, M. (1995) Aminoacyl‐RNA synthesis catalyzed by an RNA . Science 267 : 643–647. [ PubMed ] [ Google Scholar ]
- Ishii, N. , Robert, M. , Nakayama, Y. , Kanai, A. , and Tomita, M. (2004) Toward large‐scale modeling of the microbial cell for computer simulation . J Biotechnol 113 : 281–294. [ PubMed ] [ Google Scholar ]
- Isturiz, T. , and Wolf, R.E. (1975) In vitro synthesis of a constitutive enzyme of Escherichia coli , 6‐phosphogluconate dehydrogenase . Proc Natl Acad Sci USA 72 : 4381–4384. [ PMC free article ] [ PubMed ] [ Google Scholar ]
- Ito, M. , Sugiura, H. , Ayukawa, S. , Kiga, D. , and Takinoue, M. (2016) A bacterial continuous culture system based on a microfluidic droplet open reactor . Anal Sci 32 : 61–66. [ PubMed ] [ Google Scholar ]
- Jacobson, T.B. , Callaghan, M.M. , and Amador‐Noguez, D. (2021) Hostile takeover: how viruses reprogram prokaryotic metabolism . Annu Rev Microbiol 75 . in press. [ PubMed ] [ Google Scholar ]
- Jakubowski, H. (1997) Aminoacyl thioester chemistry of class II aminoacyl‐tRNA synthetases . Biochemistry 36 : 11077–11085. [ PubMed ] [ Google Scholar ]
- Jang, S. , Kim, M. , Hwang, J. , and Jung, G.Y. (2019) Tools and systems for evolutionary engineering of biomolecules and microorganisms . J Ind Microbiol Biotechnol 46 : 1313–1326. [ PubMed ] [ Google Scholar ]
- Jiang, N. , Ding, X. , and Lu, Y. (2021) Development of a robust Escherichia coli ‐based cell‐free protein synthesis application platform . Biochem Eng J 165 : 107830. [ PMC free article ] [ PubMed ] [ Google Scholar ]
- Jones, E.C. , and Uphoff, S. (2021) Single‐molecule imaging of LexA degradation in Escherichia coli elucidates regulatory mechanisms and heterogeneity of the SOS response . Nat Microbiol 6 : 981–990. [ PMC free article ] [ PubMed ] [ Google Scholar ]
- Kampers, L.F.C. , Koehorst, J.J. , van Heck, R.J.A. , Suarez‐Diez, M. , Stams, A.J.M. , and Schaap, P.J. (2021) A metabolic and physiological design study of Pseudomonas putida KT2440 capable of anaerobic respiration . BMC Microbiol 21 : 9. [ PMC free article ] [ PubMed ] [ Google Scholar ]
- Karas, J.A. , Wade, J.D. , and Hossain, M.A. (2021) The chemical synthesis of insulin: an enduring challenge . Chem Rev 121 : 4531–4560. [ PubMed ] [ Google Scholar ]
- Katoh, T. , Wohlgemuth, I. , Nagano, M. , Rodnina, M.V. , and Suga, H. (2016) Essential structural elements in tRNA(Pro) for EF‐P‐mediated alleviation of translation stalling . Nat Commun 7 : 11657. [ PMC free article ] [ PubMed ] [ Google Scholar ]
- Knüpffer, L. , Fehrenbach, C. , Denks, K. , Erichsen, V. , Petriman, N.‐A. , and Koch, H.‐G. (2019) Molecular mimicry of SecA and signal recognition particle binding to the bacterial ribosome . mBio 10 : e01317–e1319. [ PMC free article ] [ PubMed ] [ Google Scholar ]
- Ko, Y.‐S. , Kim, J.W. , Lee, J.A. , Han, T. , Kim, G.B. , Park, J.E. , and Lee, S.Y. (2020) Tools and strategies of systems metabolic engineering for the development of microbial cell factories for chemical production . Chem Soc Rev 49 : 4615–4636. [ PubMed ] [ Google Scholar ]
- Kofman, C. , Lee, J. , and Jewett, M.C. (2021) Engineering molecular translation systems . Cell Syst 12 : 593–607. [ PubMed ] [ Google Scholar ]
- Konstantinidis, D. , Pereira, F. , Geissen, E.‐M. , Grkovska, K. , Kafkia, E. , Jouhten, P. , et al . (2021) Adaptive laboratory evolution of microbial co‐cultures for improved metabolite secretion . Mol Syst Biol 17 : e10189. [ PMC free article ] [ PubMed ] [ Google Scholar ]
- Koppad, S. , B, A. , Gkoutos, G.V. , and Acharjee, A. (2021) Cloud computing enabled big multi‐omics data analytics . Bioinform Biol Insights 15 : 11779322211035920. [ PMC free article ] [ PubMed ] [ Google Scholar ]
- Koubek, J. , Schmitt, J. , Galmozzi, C.V. , and Kramer, G. (2021) Mechanisms of cotranslational protein maturation in bacteria . Front Mol Biosci 8 : 689755. [ PMC free article ] [ PubMed ] [ Google Scholar ]
- Kratz, A. , Tomita, M. , and Krishnan, A. (2008) GeNESiS: gene network evolution simulation software . BMC Bioinformatics 9 : 541. [ PMC free article ] [ PubMed ] [ Google Scholar ]
- Kruyer, N.S. , Sugianto, W. , Tickman, B.I. , Alba Burbano, D. , Noireaux, V. , Carothers, J.M. , and Peralta‐Yahya, P. (2021) Membrane augmented cell‐free systems: a new frontier in biotechnology . ACS Synth Biol 10 : 670–681. [ PubMed ] [ Google Scholar ]
- Kung, H.F. , Treadwell, B.V. , Spears, C. , Tai, P.C. , and Weissbach, H. (1977) DNA‐directed synthesis in vitro of beta‐galactosidase: requirement for a ribosome release factor . Proc Natl Acad Sci USA 74 : 3217–3221. [ PMC free article ] [ PubMed ] [ Google Scholar ]
- Kunjapur, A.M. , Napolitano, M.G. , Hysolli, E. , Noguera, K. , Appleton, E.M. , Schubert, M.G. , et al . (2021) Synthetic auxotrophy remains stable after continuous evolution and in coculture with mammalian cells . Sci Adv 7 : eabf5851. [ PMC free article ] [ PubMed ] [ Google Scholar ]
- Lachance, J.‐C. , Matteau, D. , Brodeur, J. , Lloyd, C.J. , Mih, N. , King, Z.A. , et al . (2021) Genome‐scale metabolic modeling reveals key features of a minimal gene set . Mol Syst Biol 17 : e10099. [ PMC free article ] [ PubMed ] [ Google Scholar ]
- Lee, S. , and Kim, P. (2020) Current status and applications of adaptive laboratory evolution in industrial microorganisms . J Microbiol Biotechnol 30 : 793–803. [ PMC free article ] [ PubMed ] [ Google Scholar ]
- Levisohn, R. , and Spiegelman, S. (1969) Further extracellular Darwinian experiments with replicating RNA molecules: diverse variants isolated under different selective conditions . Proc Natl Acad Sci USA 63 : 805–811. [ PMC free article ] [ PubMed ] [ Google Scholar ]
- Li, J. , Gu, L. , Aach, J. , and Church, G.M. (2014) Improved cell‐free RNA and protein synthesis system . PLoS One 9 : e106232. [ PMC free article ] [ PubMed ] [ Google Scholar ]
- Li, J. , Zhang, C. , Huang, P. , Kuru, E. , Forster‐Benson, E.T.C. , Li, T. , and Church, G.M. (2017) Dissecting limiting factors of the Protein synthesis Using Recombinant Elements (PURE) system . Translation (Austin) 5 : e1327006. [ PMC free article ] [ PubMed ] [ Google Scholar ]
- Li, Y. , Cheng, Z. , Zhao, C. , Gao, C. , Song, W. , Liu, L. , and Chen, X. (2021) Reprogramming Escherichia coli metabolism for bioplastics synthesis from waste cooking oil . ACS Synth Biol 8 : 1966–1979. [ PubMed ] [ Google Scholar ]
- Lieder, S. , Nikel, P.I. , de Lorenzo, V. , and Takors, R. (2015) Genome reduction boosts heterologous gene expression in Pseudomonas putida . Microb Cell Fact 14 : 23. [ PMC free article ] [ PubMed ] [ Google Scholar ]
- Liu, H. , and Deutschbauer, A.M. (2018) Rapidly moving new bacteria to model‐organism status . Curr Opin Biotechnol 51 : 116–122. [ PubMed ] [ Google Scholar ]
- Liu, Y. , Su, A. , Tian, R. , Li, J. , Liu, L. , and Du, G. (2020) Developing rapid growing Bacillus subtilis for improved biochemical and recombinant protein production . Metab Eng Commun 11 : e00141. [ PMC free article ] [ PubMed ] [ Google Scholar ]
- Lombardot, T. , Morgat, A. , Axelsen, K.B. , Aimo, L. , Hyka‐Nouspikel, N. , Niknejad, A. , et al . (2019) Updates in Rhea: SPARQLing biochemical reaction data . Nucleic Acids Res 47 : D596–D600. [ PMC free article ] [ PubMed ] [ Google Scholar ]
- Long, L. , Pei, R. , Liu, Y. , Rao, X. , Wang, Y. , Zhou, S.‐F. , and Zhan, G. (2021) 3D printing of recombinant Escherichia coli /Au nanocomposites as agitating paddles towards robust catalytic reduction of 4‐nitrophenol . J Hazard Mater 423 : 126983. [ PubMed ] [ Google Scholar ]
- de Lorenzo, V. , Sekowska, A. , and Danchin, A. (2015) Chemical reactivity drives spatiotemporal organisation of bacterial metabolism . FEMS Microbiol Rev 39 : 96–119. [ PubMed ] [ Google Scholar ]
- Lu, H. , Zhang, G. , He, S. , Zhao, R. , and Zhu, D. (2021) Purple non‐sulfur bacteria technology: a promising and potential approach for wastewater treatment and bioresources recovery . World J Microbiol Biotechnol 37 : 161. [ PubMed ] [ Google Scholar ]
- Mabwi, H.A. , Kim, E. , Song, D.‐G. , Yoon, H.S. , Pan, C.‐H. , Komba, E.V.G. , et al . (2021) Synthetic gut microbiome: advances and challenges . Comput Struct Biotechnol J 19 : 363–371. [ PMC free article ] [ PubMed ] [ Google Scholar ]
- Mallory, J.D. , Kolomeisky, A.B. , and Igoshin, O.A. (2019) Trade‐offs between error, speed, noise, and energy dissipation in biological processes with proofreading . J Phys Chem B 123 : 4718–4725. [ PubMed ] [ Google Scholar ]
- Marchant, A. , Cisneros, A.F. , Dubé, A.K. , Gagnon‐Arsenault, I. , Ascencio, D. , Jain, H. , et al . (2019) The role of structural pleiotropy and regulatory evolution in the retention of heteromers of paralogs . Elife 8 : e46754. [ PMC free article ] [ PubMed ] [ Google Scholar ]
- Marlière, P. , Patrouix, J. , Döring, V. , Herdewijn, P. , Tricot, S. , Cruveiller, S. , et al . (2011) Chemical evolution of a bacterium’s genome . Angew Chem Int Ed Engl 50 : 7109–7114. [ PubMed ] [ Google Scholar ]
- Martínez‐García, E. , Goñi‐Moreno, A. , Bartley, B. , McLaughlin, J. , Sánchez‐Sampedro, L. , del Pozo, H.P. , et al . (2020) SEVA 3.0: an update of the Standard European Vector Architecture for enabling portability of genetic constructs among diverse bacterial hosts . Nucleic Acids Res 48 : 3395. [ PMC free article ] [ PubMed ] [ Google Scholar ]
- Martin‐Pascual, M. , Batianis, C. , Bruinsma, L. , Asin‐Garcia, E. , Garcia‐Morales, L. , Weusthuis, R.A. , et al . (2021) A navigation guide of synthetic biology tools for Pseudomonas putida . Biotechnol Adv 49 : 107732. [ PubMed ] [ Google Scholar ]
- McCallum, G. , and Potvin‐Trottier, L. (2021) Using models to (re‐)design synthetic circuits . Methods Mol Biol 2229 : 91–118. [ PubMed ] [ Google Scholar ]
- Medema, M.H. , de Rond, T. , and Moore, B.S. (2021) Mining genomes to illuminate the specialized chemistry of life . Nat Rev Genet 22 : 553–571. [ PMC free article ] [ PubMed ] [ Google Scholar ]
- Médigue, C. , Rouxel, T. , Vigier, P. , Hénaut, A. , and Danchin, A. (1991) Evidence for horizontal gene transfer in Escherichia coli speciation . J Mol Biol 222 : 851–856. [ PubMed ] [ Google Scholar ]
- Meyer, B.J. , and Southern, P.J. (1997) A novel type of defective viral genome suggests a unique strategy to establish and maintain persistent lymphocytic choriomeningitis virus infections . J Virol 71 : 6757–6764. [ PMC free article ] [ PubMed ] [ Google Scholar ]
- Meyer, V. , Cairns, T. , Barthel, L. , King, R. , Kunz, P. , Schmideder, S. , et al . (2021) Understanding and controlling filamentous growth of fungal cell factories: novel tools and opportunities for targeted morphology engineering . Fungal Biol Biotechnol 8 : 8. [ PMC free article ] [ PubMed ] [ Google Scholar ]
- Miguez, A.M. , Zhang, Y. , Piorino, F. , and Styczynski, M.P. (2021) Metabolic dynamics in Escherichia coli ‐based cell‐free systems . ACS Synth Biol 10 : 2252–2265. [ PMC free article ] [ PubMed ] [ Google Scholar ]
- Miller, D.L. , Andreone, T.L. , and Donelson, J.E. (1977) Translation in Escherichia coli mini‐cells containing hamster mitochondrial DNA‐Co1E1 ‐ Ampr recombinant plasmids . Biochim Biophys Acta 477 : 323–333. [ PubMed ] [ Google Scholar ]
- Mordret, E. , Dahan, O. , Asraf, O. , Rak, R. , Yehonadav, A. , Barnabas, G.D. , et al . (2019) Systematic detection of amino acid substitutions in proteomes reveals mechanistic basis of ribosome errors and eslection for translation fidelity . Mol Cell 75 : 427–441.e5. [ PubMed ] [ Google Scholar ]
- Morgan, A.A. , and Rubenstein, E. (2013) Proline: the distribution, frequency, positioning, and common functional roles of proline and polyproline sequences in the human proteome . PLoS One 8 : e53785. [ PMC free article ] [ PubMed ] [ Google Scholar ]
- Moroder, L. , and Budisa, N. (2010) Synthetic biology of protein folding . ChemPhysChem 11 : 1181–1187. [ PubMed ] [ Google Scholar ]
- Mortimer, M. , Wang, Y. , and Holden, P.A. (2021) Molecular mechanisms of nanomaterial‐bacterial interactions revealed by omics‐The role of nanomaterial effect level . Front Bioeng Biotechnol 9 : 683520. [ PMC free article ] [ PubMed ] [ Google Scholar ]
- Moyer, D. , Pacheco, A.R. , Bernstein, D.B. , and Segrè, D. (2021) Stoichiometric modeling of artificial string chemistries reveals constraints on metabolic network structure . J Mol Evol 89 : 472–483. [ PMC free article ] [ PubMed ] [ Google Scholar ]
- Mrázek, J. , and Karls, A.C. (2019) In silico simulations of occurrence of transcription factor binding sites in bacterial genomes . BMC Evol Biol 19 : 67. [ PMC free article ] [ PubMed ] [ Google Scholar ]
- Müller, J. , Siemann‐Herzberg, M. , and Takors, R. (2020) Modeling cell‐free protein synthesis systems‐Approaches and applications . Front Bioeng Biotechnol 8 : 584178. [ PMC free article ] [ PubMed ] [ Google Scholar ]
- Nakahigashi, K. , Toya, Y. , Ishii, N. , Soga, T. , Hasegawa, M. , Watanabe, H. , et al . (2009) Systematic phenome analysis of Escherichia coli multiple‐knockout mutants reveals hidden reactions in central carbon metabolism . Mol Syst Biol 5 : 306. [ PMC free article ] [ PubMed ] [ Google Scholar ]
- Navelkar, R. , Owen, G. , Mutherkrishnan, V. , Thiessen, P. , Cheng, T. , Bolerlton, E. , et al . (2021) Enhancing the interoperability of glycan data flow between ChEBI, PubChem, and GlyGen . Glycobiology cwab078. [ PMC free article ] [ PubMed ] [ Google Scholar ]
- Ng, I.‐S. , Keskin, B.B. , and Tan, S.‐I. (2020) A critical review of genome editing and synthetic biology applications in metabolic engineering of microalgae and cyanobacteria . Biotechnol J 15 : e1900228. [ PubMed ] [ Google Scholar ]
- Nicolás Carcelén, J. , Marchante‐Gayón, J.M. , González, P.R. , Valledor, L. , Cañal, M.J. , and Alonso, J.I.G. (2017) A cost‐effective approach to produce 15N‐labelled amino acids employing Chlamydomonas reinhardtii CC503 . Microb Cell Fact 16 : 146. [ PMC free article ] [ PubMed ] [ Google Scholar ]
- Nielsen, S.D. , Koren, K. , Löbmann, K. , Hinge, M. , Scoma, A. , Kjeldsen, K.U. , and Røy, H. (2020) Constraints on CaCO 3 precipitation in superabsorbent polymer by aerobic bacteria . Appl Microbiol Biotechnol 104 : 365–375. [ PubMed ] [ Google Scholar ]
- Nishizawa, M. , Sakai, S. , Konno, U. , Nakahara, N. , Takaki, Y. , Saito, Y. , et al . (2016) Nitrogen and oxygen isotope effects of ammonia oxidation by thermophilic Thaumarchaeota from a geothermal water stream . Appl Environ Microbiol 82 : 4492–4504. [ PMC free article ] [ PubMed ] [ Google Scholar ]
- Noireaux, V. , and Liu, A.P. (2020) The new age of cell‐free biology . Annu Rev Biomed Eng 22 : 51–77. [ PubMed ] [ Google Scholar ]
- Norsigian, C.J. , Fang, X. , Palsson, B.O. , and Monk, J.M. (2020) Pangenome flux balance analysis toward panphenomes. In The Pangenome: Diversity, Dynamics and Evolution of Genomes . Tettelin, H. , and Medini, D. (eds). Cham: Springer. [ PubMed ] [ Google Scholar ]
- Oesterle, S. , Roberts, T.M. , Widmer, L.A. , Mustafa, H. , Panke, S. , and Billerbeck, S. (2017) Sequence‐based prediction of permissive stretches for internal protein tagging and knockdown . BMC Biol 15 : 100. [ PMC free article ] [ PubMed ] [ Google Scholar ]
- Ohuchi, M. , Murakami, H. , and Suga, H. (2007) The flexizyme system: a highly flexible tRNA aminoacylation tool for the translation apparatus . Curr Opin Chem Biol 11 : 537–542. [ PubMed ] [ Google Scholar ]
- Ou, Z. , Ouzounis, C. , Wang, D. , Sun, W. , Li, J. , Chen, W. , et al. (2020) A path towards SARS‐CoV‐2 attenuation: metabolic pressure on CTP synthesis rules the virus evolution . Genome Biol Evol 12 : 2467–2485. [ PMC free article ] [ PubMed ] [ Google Scholar ]
- Palazzotto, E. , Tong, Y. , Lee, S.Y. , and Weber, T. (2019) Synthetic biology and metabolic engineering of actinomycetes for natural product discovery . Biotechnol Adv 37 : 107366. [ PubMed ] [ Google Scholar ]
- Paley, S. , Keseler, I.M. , Krummenacker, M. , and Karp, P.D. (2021) Leveraging curation among Escherichia coli pathway/genome databases using ortholog‐based annotation propagation . Front Microbiol 12 : 614355. [ PMC free article ] [ PubMed ] [ Google Scholar ]
- Panke, S. (2020) Taming the beast of biology: synthetic biology and biological systems engineering . Chimia (Aarau) 74 : 402–406. [ PubMed ] [ Google Scholar ]
- Park, Y.‐K. , and Nicaud, J.‐M. (2020) Metabolic engineering for unusual lipid production in Yarrowia lipolytica . Microorganisms 8 : E1937. [ PMC free article ] [ PubMed ] [ Google Scholar ]
- Passioura, T. , and Suga, H. (2014) Flexizymes, their evolutionary history and diverse utilities . Top Curr Chem 344 : 331–345. [ PubMed ] [ Google Scholar ]
- Pedrioli, G. , Piovesana, E. , Vacchi, E. , and Balbi, C. (2021) Extracellular vesicles as promising carriers in drug delivery: considerations from a cell biologist’s perspective . Biology (Basel) 10 : 376. [ PMC free article ] [ PubMed ] [ Google Scholar ]
- Pereira, F. , Lopes, H. , Maia, P. , Meyer, B. , Nocon, J. , Jouhten, P. , et al . (2021) Model‐guided development of an evolutionarily stable yeast chassis . Mol Syst Biol 17 : e10253. [ PMC free article ] [ PubMed ] [ Google Scholar ]
- Piette, F. , D’Amico, S. , Mazzucchelli, G. , Danchin, A. , Leprince, P. , and Feller, G. (2011) Life in the cold: a proteomic study of cold‐repressed proteins in the antarctic bacterium pseudoalteromonas haloplanktis TAC125 . Appl Environ Microbiol 77 : 3881–3883. [ PMC free article ] [ PubMed ] [ Google Scholar ]
- Piñero‐Lambea, C. , Garcia‐Ramallo, E. , Martinez, S. , Delgado, J. , Serrano, L. , and Lluch‐Senar, M. (2020) Mycoplasma pneumoniae genome editing based on oligo recombineering and Cas9‐mediated counterselection . ACS Synth Biol 9 : 1693–1704. [ PMC free article ] [ PubMed ] [ Google Scholar ]
- Piñeros, W.D. , and Tlusty, T. (2020) Kinetic proofreading and the limits of thermodynamic uncertainty . Phys Rev E 101 : 022415. [ PubMed ] [ Google Scholar ]
- Prifti, E. , Chevaleyre, Y. , Hanczar, B. , Belda, E. , Danchin, A. , Clément, K. , and Zucker, J.‐D. (2020) Interpretable and accurate prediction models for metagenomics data . Gigascience 9 : giaa010. [ PMC free article ] [ PubMed ] [ Google Scholar ]
- Pühler, A. (2012) Genome‐based microbiology–from ‐omics research to systems and synthetic biology . J Biotechnol 160 : 1–2. [ PubMed ] [ Google Scholar ]
- Raghavan, A.R. , Salim, K. , and Yadav, V.G. (2020) Optogenetic control of heterologous metabolism in E. coli . ACS Synth Biol 9 : 2291–2300. [ PubMed ] [ Google Scholar ]
- Ramaswamy, K. , Saito, H. , Murakami, H. , Shiba, K. , and Suga, H. (2004) Designer ribozymes: programming the tRNA specificity into flexizyme . J Am Chem Soc 126 : 11454–11455. [ PubMed ] [ Google Scholar ]
- Rasor, B.J. , Vögeli, B. , Landwehr, G.M. , Bogart, J.W. , Karim, A.S. , and Jewett, M.C. (2021) Toward sustainable, cell‐free biomanufacturing . Curr Opin Biotechnol 69 : 136–144. [ PubMed ] [ Google Scholar ]
- Rees‐Garbutt, J. , Chalkley, O. , Grierson, C. , and Marucci, L. (2021) Minimal genome design algorithms using whole‐cell models . Methods Mol Biol 2189 : 183–198. [ PubMed ] [ Google Scholar ]
- Ren, X. , Linehan, M.M. , Iwasaki, A. , and Pyle, A.M. (2019) RIG‐I selectively discriminates against 5’‐monophosphate RNA . Cell Rep 26 : 2019–2027.e4. [ PubMed ] [ Google Scholar ]
- Robertson, W.E. , Funke, L.F.H. , de la Torre, D. , Fredens, J. , Elliott, T.S. , Spinck, M. , et al . (2021) Sense codon reassignment enables viral resistance and encoded polymer synthesis . Science 372 : 1057–1062. [ PMC free article ] [ PubMed ] [ Google Scholar ]
- Rustad, M. , Eastlund, A. , Marshall, R. , Jardine, P. , and Noireaux, V. (2017) Synthesis of infectious bacteriophages in an E. coli ‐based cell‐free expression system . J vis Exp 126 : 56144. [ PMC free article ] [ PubMed ] [ Google Scholar ]
- Ryngajłło, M. , Jędrzejczak‐Krzepkowska, M. , Kubiak, K. , Ludwicka, K. , and Bielecki, S. (2020) Towards control of cellulose biosynthesis by Komagataeibacter using systems‐level and strain engineering strategies: current progress and perspectives . Appl Microbiol Biotechnol 104 : 6565–6585. [ PMC free article ] [ PubMed ] [ Google Scholar ]
- Santibáñez, R. , Garrido, D. , and Martin, A.J.M. (2020) Atlas: automatic modeling of regulation of bacterial gene expression and metabolism using rule‐based languages . Bioinformatics 36 : 5473–5480. [ PMC free article ] [ PubMed ] [ Google Scholar ]
- Satanowski, A. , Dronsella, B. , Noor, E. , Vögeli, B. , He, H. , Wichmann, P. , et al . (2020) Awakening a latent carbon fixation cycle in Escherichia coli . Nat Commun 11 : 5812. [ PMC free article ] [ PubMed ] [ Google Scholar ]
- Schindler, D. (2020) Genetic engineering and synthetic genomics in yeast to understand life and boost biotechnology . Bioengineering (Basel) 7 : E137. [ PMC free article ] [ PubMed ] [ Google Scholar ]
- Schmied, W.H. , Tnimov, Z. , Uttamapinant, C. , Rae, C.D. , Fried, S.D. , and Chin, J.W. (2018) Controlling orthogonal ribosome subunit interactions enables evolution of new function . Nature 564 : 444–448. [ PMC free article ] [ PubMed ] [ Google Scholar ]
- Sekowska, A. , Masson, J.‐B. , Celani, A. , Danchin, A. , and Vergassola, M. (2009) Repulsion and metabolic switches in the collective behavior of bacterial colonies . Biophys J 97 : 688–698. [ PMC free article ] [ PubMed ] [ Google Scholar ]
- Sekowska, A. , Wendel, S. , Fischer, E.C. , Nørholm, M.H.H. , and Danchin, A. (2016) Generation of mutation hotspots in ageing bacterial colonies . Sci Rep 6 : 2. [ PMC free article ] [ PubMed ] [ Google Scholar ]
- Srividhya, K.V. , and Krishnaswamy, S. (2004) A simulation model of Escherichia coli osmoregulatory switch using E‐CELL system . BMC Microbiol 4 : 44. [ PMC free article ] [ PubMed ] [ Google Scholar ]
- St John, P.C. , and Bomble, Y.J. (2019) Approaches to computational strain design in the multiomics era . Front Microbiol 10 : 597. [ PMC free article ] [ PubMed ] [ Google Scholar ]
- Strauß, J.F. , Crain, P. , Schulenburg, H. , and Telschow, A. (2016) Experimental evolution in silico: a custom‐designed mathematical model for virulence evolution of Bacillus thuringiensis . Zoology (Jena) 119 : 359–365. [ PubMed ] [ Google Scholar ]
- Sun, G. , Ahn‐Horst, T.A. , and Covert, M.W. (2021) The E. coli whole‐cell modeling project . EcoSal plus eESP00012020. [ PMC free article ] [ PubMed ] [ Google Scholar ]
- Takinoue, M. , and Kawano, R. (2020) Editorial on the special issue on recent advances of molecular machines and molecular robots . Micromachines (Basel) 11 : E1031. [ PMC free article ] [ PubMed ] [ Google Scholar ]
- Tharp, J.M. , Walker, J.A. , Söll, D. , and Schepartz, A. (2021) Initiating protein synthesis with noncanonical monomers in vitro and in vivo. In Synthetic and Enzymatic Modifications of the Peptide Backbone . Methods in Enzymology. Vol 656 . Amsterdam: Elsevier Science, pp. 495–519. [ PMC free article ] [ PubMed ] [ Google Scholar ]
- To, P. , Whitehead, B. , Tarbox, H.E. , and Fried, S.D. (2021) Nonrefoldability is pervasive across the E. coli proteome . J Am Chem Soc 143 : 11435–11448. [ PMC free article ] [ PubMed ] [ Google Scholar ]
- Tomita, M. (2001) Whole‐cell simulation: a grand challenge of the 21st century . Trends Biotechnol 19 : 205–210. [ PubMed ] [ Google Scholar ]
- Tomita, M. , Hashimoto, K. , Takahashi, K. , Shimizu, T. , Matsuzaki, Y. , Miyoshi, F. , et al . (1997) E‐CELL: software environment for whole cell simulation . Genome Inform Ser Workshop Genome Inform 8 : 147–155. [ PubMed ] [ Google Scholar ]
- Tuerk, C. , MacDougal, S. , and Gold, L. (1992) RNA pseudoknots that inhibit human immunodeficiency virus type 1 reverse transcriptase . Proc Natl Acad Sci USA 89 : 6988–6992. [ PMC free article ] [ PubMed ] [ Google Scholar ]
- Tyo, K.E.J. , Kocharin, K. , and Nielsen, J. (2010) Toward design‐based engineering of industrial microbes . Curr Opin Microbiol 13 : 255–262. [ PMC free article ] [ PubMed ] [ Google Scholar ]
- Tzeng, S.‐R. , Tseng, Y.‐C. , Lin, C.‐C. , Hsu, C.‐Y. , Huang, S.‐J. , Kuo, Y.‐T. , and Chang, C.‐I. (2021) Molecular insights into substrate recognition and discrimination by the N‐terminal domain of Lon AAA+ protease . Elife 10 : e64056. [ PMC free article ] [ PubMed ] [ Google Scholar ]
- Vaish, A. , Guo, S. , Murray, R.M. , Grandsard, P.J. , and Chen, Q. (2018) On‐chip membrane protein cell‐free expression enables development of a direct binding assay: a curious case of potassium channel KcsA‐Kv1.3 . Anal Biochem 556 : 70–77. [ PubMed ] [ Google Scholar ]
- Vallenet, D. , Calteau, A. , Dubois, M. , Amours, P. , Bazin, A. , Beuvin, M. , et al . (2020) MicroScope: an integrated platform for the annotation and exploration of microbial gene functions through genomic, pangenomic and metabolic comparative analysis . Nucleic Acids Res 48 : D579–D589. [ PMC free article ] [ PubMed ] [ Google Scholar ]
- Vatsa, D. , and Agarwal, S. (2021) PEPN‐GRN: a Petri net‐based approach for the inference of gene regulatory networks from noisy gene expression data . PLoS One 16 : e0251666. [ PMC free article ] [ PubMed ] [ Google Scholar ]
- Vázquez‐Castellanos, J.F. , Biclot, A. , Vrancken, G. , Huys, G.R. , and Raes, J. (2019) Design of synthetic microbial consortia for gut microbiota modulation . Curr Opin Pharmacol 49 : 52–59. [ PubMed ] [ Google Scholar ]
- Voigt, C.A. (2020) Synthetic biology 2020–2030: six commercially‐available products that are changing our world . Nat Commun 11 : 6379. [ PMC free article ] [ PubMed ] [ Google Scholar ]
- Walther, T. , Calvayrac, F. , Malbert, Y. , Alkim, C. , Dressaire, C. , Cordier, H. , and François, J.M. (2018) Construction of a synthetic metabolic pathway for the production of 2,4‐dihydroxybutyric acid from homoserine . Metab Eng 45 : 237–245. [ PubMed ] [ Google Scholar ]
- Wang, F. , Gao, Y. , and Yang, G. (2020) Recent advances in synthetic biology of cyanobacteria for improved chemicals production . Bioengineered 11 : 1208–1220. [ PMC free article ] [ PubMed ] [ Google Scholar ]
- Wang, Q. , Zhang, J. , Al Makishah, N.H. , Sun, X. , Wen, Z. , Jiang, Y. , and Yang, S. (2021) Advances and perspectives for genome editing tools of Corynebacterium glutamicum . Front Microbiol 12 : 654058. [ PMC free article ] [ PubMed ] [ Google Scholar ]
- Wassenaar, T.M. , and Zimmermann, K. (2020) How industrial bacterial cultures can be kept stable over time . Lett Appl Microbiol 71 : 220–228. [ PMC free article ] [ PubMed ] [ Google Scholar ]
- Weinberg, R. , and Berkus, M. (1971) Computer simulation of a living cell: Part I . Int J Biomed Comput 2 : 95–120. [ PubMed ] [ Google Scholar ]
- Wolf, S. , Becker, J. , Tsuge, Y. , Kawaguchi, H. , Kondo, A. , Marienhagen, J. , et al . (2021) Advances in metabolic engineering of Corynebacterium glutamicum to produce high‐value active ingredients for food, feed, human health, and well‐being . Essays Biochem 65 : 197–212. [ PMC free article ] [ PubMed ] [ Google Scholar ]
- Xiang, M. , Kang, Q. , and Zhang, D. (2020) Advances on systems metabolic engineering of Bacillus subtilis as a chassis cell . Synth Syst Biotechnol 5 : 245–251. [ PMC free article ] [ PubMed ] [ Google Scholar ]
- Xie, S. , Syrenne, R. , Sun, S. , and Yuan, J.S. (2014) Exploration of Natural Biomass Utilization Systems (NBUS) for advanced biofuel–from systems biology to synthetic design . Curr Opin Biotechnol 27 : 195–203. [ PubMed ] [ Google Scholar ]
- Xu, C. , Liu, L. , Zhang, Z. , Jin, D. , Qiu, J. , and Chen, M. (2013) Genome‐scale metabolic model in guiding metabolic engineering of microbial improvement . Appl Microbiol Biotechnol 97 : 519–539. [ PubMed ] [ Google Scholar ]
- Xu, Z. , Wang, S. , Zhao, C. , Li, S. , Liu, X. , Wang, L. , et al . (2020) Photosynthetic hydrogen production by droplet‐based microbial micro‐reactors under aerobic conditions . Nat Commun 11 : 5985. [ PMC free article ] [ PubMed ] [ Google Scholar ]
- Yachie‐Kinoshita, A. , Nishino, T. , Shimo, H. , Suematsu, M. , and Tomita, M. (2010) A metabolic model of human erythrocytes: practical application of the E‐Cell simulation environment . J Biomed Biotechnol 2010 : 642420. [ PMC free article ] [ PubMed ] [ Google Scholar ]
- Yadav, S.P. (2007) The wholeness in suffix ‐omics, ‐omes, and the word om . J Biomol Tech 18 : 277. [ PMC free article ] [ PubMed ] [ Google Scholar ]
- Yamamoto, H. , Fukasawa, Y. , Shoji, Y. , Hisamoto, S. , Kikuchi, T. , Takamatsu, A. , and Iwasaki, H. (2021) Scattered migrating colony formation in the filamentous cyanobacterium, Pseudanabaena sp. NIES‐4403 . BMC Microbiol 21 : 227. [ PMC free article ] [ PubMed ] [ Google Scholar ]
- Yang, H.‐J. , Lee, K.‐H. , Lim, H.J. , and Kim, D.‐M. (2019) Tandem cell‐free protein synthesis as a tool for rapid screening of optimal molecular chaperones . Biotechnol J 14 : e1800523. [ PubMed ] [ Google Scholar ]
- Yang, J. , Zheng, P. , Li, Y. , Wu, J. , Tan, X. , Zhou, J. , et al. (2020) Landscapes of bacterial and metabolic signatures and their interaction in major depressive disorders . Sci Adv 6 : eaba8555. [ PMC free article ] [ PubMed ] [ Google Scholar ]
- Yu, A.‐S. , Yim, A.‐Y. , Mat, W.‐K. , Tong, A.‐Y. , Lok, S.I. , Xue, H. , et al . (2014) Mutations enabling displacement of tryptophan by 4‐fluorotryptophan as a canonical amino acid of the genetic code . Genome Biol Evol 6 : 629–641. [ PMC free article ] [ PubMed ] [ Google Scholar ]
- Zamdborg, L. , Holloway, D.M. , Merelo, J.J. , Levchenko, V.F. , and Spirov, A.V. (2015) Forced evolution in silico by artificial transposons and their genetic operators: the ant navigation problem . Inf Sci (N Y) 306 : 88–110. [ PMC free article ] [ PubMed ] [ Google Scholar ]
- Zhang, B. , Jiao, J. , Zhang, P. , Cui, W.‐J. , Zhang, Z. , and Tian, C.‐F. (2021) Comparative analysis of core and accessory genes in coexpression network . Methods Mol Biol 2242 : 45–58. [ PubMed ] [ Google Scholar ]
- Zhang, L. , Lin, X. , Wang, T. , Guo, W. , and Lu, Y. (2021) Development and comparison of cell‐free protein synthesis systems derived from typical bacterial chassis . Bioresour Bioprocess 8 : 58. [ PMC free article ] [ PubMed ] [ Google Scholar ]
- Zhang, T. , Hansen, K. , Politis, A. , and Müller, M.M. (2020) An unusually rapid protein backbone modification stabilizes the essential bacterial enzyme MurA . Biochemistry 59 : 3683–3695. [ PMC free article ] [ PubMed ] [ Google Scholar ]
- Introduction to the Marshall Protocol
- Length of the MP
- Immunopathology
- Managing immunopathology
- Food and drink
- Light restriction
- Vitamin D metabolite calculator - get feedback on vitamin D results
- Therapeutic probe - best method for determining MP suitability
- Starting the Marshall Protocol
- Resources for patients
- Marshall Protocol summary
- Palliative vs. curative treatments
- Patient interviews on Bacteriality
- Non-MP treatments
- Publications and presentations
- Science behind immunopathology
- Science behind olmesartan (Benicar)
- Safety of olmesartan
- Science behind MP antibiotics
- Safety of MP antibiotics
- Resources for physicians
- Physicians' concerns about the MP

- Science behind Marshall Pathogenesis
- Microbes in the human body
- Successive infection and variability in disease
- Autoimmune theory of disease
- Science behind vitamin D
- Metabolism of vitamin D and the VDR
- Th1 Spectrum Disorder - how chronic inflammatory diseases are related
- Transmission of bacteria and onset of chronic disease
- Evolutionary perspective on disease
- Incidence and prevalence of disease
- Evidence that chronic disease is caused by pathogens
- Chronic fatigue syndrome
- Chronic Lyme disease
- Sarcoidosis
- Fibromyalgia
- Multiple chemical sensitivity
- Cardiovascular diseases
- Crohn's disease
- Irritable Bowel Syndrome
- Multiple Sclerosis
- Rheumatoid Arthritis
- Complete List of Diseases
- Press releases
- Media coverage
- Autoimmunity Research Foundation
- Ways To Contribute
- History Timeline
- Autoimmunity Research Video Resources
- MarshallProtocol.com
- Microbeminded.com
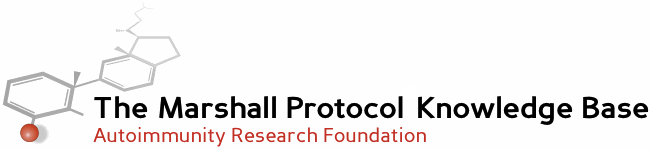
Table of Contents
In vitro studies, in vivo studies, in silico studies, read more about study design, differences between in vitro, in vivo, and in silico studies.
Related article: Animal models
There are three broad categories of experiments: in vitro A technique of performing a given procedure in a controlled environment outside of a living organism - usually a laboratory. studies, in vivo A type of scientific study that analyzes an organism in its natural living environment. studies, and in silico Experiment technique performed on computer or via computer emulation. studies. Each study type has conveniences and liabilities. Understanding the liabilities of study types offers insight into the validity of researchers' conclusions.
In vitro (Latin for within the glass) refers to the technique of performing a given procedure in a controlled environment outside of a living organism. Many experiments in cellular biology are conducted outside of organisms or cells. One of the abiding weaknesses of in vitro experiments is that they fail to replicate the precise cellular conditions of an organism, particularly a microbe. To cite one example among many, the lysates or extracts from culture-grown spirochetes do not reflect antigens expressed in the mammalian Borrelia :
Addressing this question is complicated by the fact that protein expression of culture-grown spirochetes does not fully resemble Borrelia in the host, i.e. the usefulness of protein lysates from culture-grown bacteria is limited as a source of antigen for ELISPOT analysis. Stefan S. Tunev 1)
Because of this, in vitro studies may lead to results that do not correspond to the circumstances occuring around a living organism.
Until the last several years, efforts to detect and identify microorganisms in the human body have depended almost exclusively on in vitro studies. As a result, many researchers began to assume that chronic diseases were not caused by microbes. The net effect of all this was that the understanding of pathogens in disease was driven by the study of well-known, easy-to-culture microbes–which, as it turns out, represent the vast minority of bacteria in the human body. By one estimate, 99.6% of the species in the human microbiota The bacterial community in the human body. Many species in the microbiota contribute to the development of chronic disease. have not or cannot be characterized through in vitro techniques. 2)
Another example of a shortcoming of in vitro studies relates to concentrations of molecules, especially as they compete for nuclear receptors Intracellular receptor proteins that bind to hydrophobic signal molecules (such as steroid and thyroid hormones) or intracellular metabolites and are thus activated to bind to specific DNA sequences which affect transcription. . For example, the vitamin D metabolite, 1,25-D Primary biologically active vitamin D hormone. Activates the vitamin D nuclear receptor. Produced by hydroxylation of 25-D. Also known as 1,25-dihydroxycholecalciferol, 1,25-hydroxyvitamin D and calcitirol. , exerts its effects at 30 picograms per milliliter, or 0.000000000003 grams per milliliter.
In vivo (Latin for “within the living”) refers to experimentation using a whole, living organism as opposed to a partial or dead organism. Animal studies and clinical trials are two forms of in vivo research. In vivo testing is often employed over in vitro because it is better suited for observing the overall effects of an experiment on a living subject.
While there are many reasons to believe in vivo studies have the potential to offer conclusive insights about the nature of medicine and disease, there is a number of ways that these conclusions can be misleading. For example, a therapy can offer a short-term benefit, but a long-term harm.
In silico is an expression used to mean “performed on computer or via computer simulation.” The expression in silico was first used in public in 1989 in the workshop “Cellular Automata: Theory and Applications” in Los Alamos, New Mexico. Pedro Miramontes, a mathematician from National Autonomous University of Mexico (UNAM), presented the report “DNA and RNA Physicochemical Constraints, Cellular Automata and Molecular Evolution.” In his talk, Miramontes used the term “ in silico ” to characterize biological experiments carried out entirely in a computer.
Although in silico studies represent a relatively new avenue of inquiry, it has begun to be used widely in studies which predict how drugs interact with the body and with pathogens. For example, a 2009 study used software emulations to predict how certain drugs already on the market could treat multiple-drug-resistant and extensively drug-resistant strains of tuberculosis. 3)
There is a variety of in silico techniques, but the two that are discussed the most in connection with the Marshall Protocol A curative medical treatment for chronic inflammatory disease. Based on the Marshall Pathogenesis. are:
- Bacterial sequencing techniques – As an alternative to in vitro methods for identifying bacteria, various in silico methods which sequence bacterial DNA and RNA have been developed. The most commonly used use is polymerase chain reaction ( PCR ). PCR takes a single or few copies of a piece of DNA and increases it across several orders of magnitude, generating millions or more copies of a particular DNA sequence. PCR has allowed researchers to detect bacteria associated with a variety of conditions with increasingly high sensitivity.
- Molecular modeling – Part of the Marshall Pathogenesis A description for how chronic inflammatory diseases originate and develop. is based on in silico work, demonstrating how drugs and other substances interact with the nuclear receptors of cells. In particular, Trevor Marshall, PhD, has used computer-based emulations to show that 25-D The vitamin D metabolite widely (and erroneously) considered best indicator of vitamin D "deficiency." Inactivates the Vitamin D Nuclear Receptor. Produced by hydroxylation of vitamin D3 in the liver. , one of the vitamin D metabolites, and Capnine, a substance produced by bacteria, turn off the Vitamin D Receptor A nuclear receptor located throughout the body that plays a key role in the innate immune response. . These conclusions have since been validated by clinical observations.
- Whole cell simulations – As described here , researchers have built a computer model of the crowded interior of a bacterial cell that—in a test of its response to sugar in its environment—accurately simulates the behavior of living cells. 4)
===== Notes and comments =====
- https://www.marshallprotocol.com/view_topic.php?id=4142&forum_id=37&jump_to=88745#p88745 s153
- https://www.marshallprotocol.com/view_topic.php?id=3139&forum_id=37&jump_to=35262#p35262 s229
===== References =====
home/patients/assessing_literature/in_vitro_studies.txt · Last modified: 09.14.2022 by 127.0.0.1
© 2015, Autoimmunity Research Foundation
All Rights Reserved. Privacy Policy

Ex Vivo vs. In Vivo
What's the difference.
Ex vivo and in vivo are two different approaches used in scientific research and experimentation. Ex vivo refers to experiments conducted outside of a living organism, typically using isolated cells or tissues in a controlled laboratory setting. In contrast, in vivo experiments are conducted within a living organism, allowing researchers to observe the effects of a treatment or intervention in a more complex biological system. While ex vivo studies offer more control and precision, in vivo studies provide a more realistic representation of how a treatment may work in a living organism. Both approaches have their own advantages and limitations, and researchers often use a combination of ex vivo and in vivo studies to gain a comprehensive understanding of a particular biological process or disease.
Attribute | Ex Vivo | In Vivo |
---|---|---|
Definition | Outside the living organism | Inside the living organism |
Sample source | Cells or tissues removed from the organism | Cells or tissues within the organism |
Experimental control | Higher control over experimental conditions | Less control over experimental conditions |
Duration | Short-term experiments | Long-term experiments |
Physiological relevance | May not fully represent in vivo conditions | Represents physiological conditions in the organism |
Further Detail
Introduction.
Ex vivo and in vivo are two terms commonly used in the field of biology and medicine to describe different experimental approaches. Both methods have their own set of advantages and limitations, making them suitable for specific research purposes. In this article, we will compare the attributes of ex vivo and in vivo studies to understand their differences and similarities.
Ex vivo refers to experiments or studies conducted outside of a living organism, typically using isolated cells, tissues, or organs. In contrast, in vivo studies involve experiments conducted within a living organism, such as a mouse, rat, or human. Both approaches are essential for understanding biological processes and developing new treatments for diseases.
Experimental Control
One of the key differences between ex vivo and in vivo studies is the level of experimental control. In ex vivo experiments, researchers have more control over the variables being studied since they can manipulate the isolated cells or tissues in a controlled environment. This allows for precise measurements and observations that may not be possible in a living organism.
In contrast, in vivo studies involve a more complex biological system with multiple interacting variables. While this can make it challenging to isolate the effects of a specific treatment or intervention, in vivo studies provide a more realistic representation of how a drug or therapy may work in a living organism.
Relevance to Human Physiology
Another important consideration when comparing ex vivo and in vivo studies is their relevance to human physiology. Ex vivo experiments can provide valuable insights into cellular and molecular processes, but they may not fully capture the complexity of interactions that occur within a living organism. In vivo studies, on the other hand, offer a more holistic view of how a treatment or intervention may affect the entire body.
For researchers developing new drugs or therapies, in vivo studies are essential for understanding how a treatment will behave in a real-world setting. While ex vivo experiments can provide valuable preliminary data, in vivo studies are ultimately necessary to determine the safety and efficacy of a new treatment in humans.
Ethical Considerations
When comparing ex vivo and in vivo studies, ethical considerations also play a significant role. Ex vivo experiments typically involve the use of cells or tissues obtained from animals or human donors, which raises ethical concerns about the treatment of living organisms. In contrast, in vivo studies involve the use of live animals, which can raise additional ethical considerations related to animal welfare.
Researchers conducting in vivo studies are required to follow strict ethical guidelines to ensure the humane treatment of animals and minimize any potential harm. While ex vivo experiments may involve fewer ethical concerns, researchers must still consider the source of the cells or tissues being used and ensure that they are obtained ethically and with proper consent.
Cost and Time Considerations
Cost and time are also important factors to consider when comparing ex vivo and in vivo studies. Ex vivo experiments are typically less expensive and time-consuming than in vivo studies since they do not require the care and maintenance of live animals. This can make ex vivo studies a more cost-effective option for researchers working with limited resources.
On the other hand, in vivo studies can be more time-consuming and costly due to the need for animal care, housing, and monitoring. Additionally, in vivo studies may require larger sample sizes to account for biological variability, which can further increase the cost and time required to complete the study.
In conclusion, both ex vivo and in vivo studies play important roles in biological and medical research. Each approach has its own set of advantages and limitations, making them suitable for specific research questions and objectives. Researchers must carefully consider the experimental design, ethical considerations, and cost and time constraints when choosing between ex vivo and in vivo studies to ensure the validity and relevance of their findings.
Comparisons may contain inaccurate information about people, places, or facts. Please report any issues.
We've updated our Privacy Policy to make it clearer how we use your personal data. We use cookies to provide you with a better experience. You can read our Cookie Policy here.
Drug Discovery
Stay up to date on the topics that matter to you
In Vivo vs In Vitro : Definition, Pros and Cons
In this article, we will define the terms in vivo and in vitro and further evaluate the pros and cons of analysis using these methods with detailed examples..
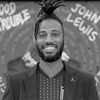
Complete the form below to unlock access to ALL audio articles.
In vivo vs in vitro definitions
If you have read any scientific study that involves biological or medical research, then you may have encountered the terms “ in vivo ” and “ in vitro ” throughout the text. The etymological origins of in vivo and in vitro come from Latin; in vivo describes something “within a living organism” while in vitro describes something “in glass” such as a test tube or petri dish. In this article, we will define these terms and further evaluate the pros and cons of analysis using in vivo and in vitro methods with detailed examples.
In vitro and in vivo models in drug development
Before an experimental drug is studied in vivo , its mechanism of action and complexity need to be thoroughly evaluated using in vitro models. In vitro models provide a starting point for researchers to gather insights into how a cell responds to a new drug in a controlled, isolated environment.
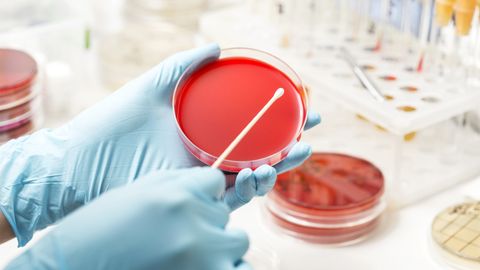
Cell Culture – Good Practice and Advanced Methods
For example, in vitro tumor models provide low-cost platforms for a pharmaceutical company to study the biological effects of experimental drug candidates against cancer cell lines. Results from these studies can inform researchers about the molecular mechanisms of each drug candidate and how these mechanisms may influence cancerous cells under defined conditions. However, these results are extremely difficult to extrapolate a clinical effect 2 from as they studies were not performed in an intact, living organism, where far more biological variables are in play. Once a drug candidate demonstrates effectiveness through a series of in vitro experiments, in vivo models can be employed to advance drug development studies. These preclinical studies typically involve the use of animals to further evaluate the safety, efficacy and delivery of a drug candidate. For example, rodent models inoculated with tumor cells 3 are commonly used to screen anti-tumor drug candidates that exhibited promising results throughout previously performed in vitro studies.
|
|
|
Definition | Studies “within a living organism” | Studies performed “in the glass” |
Cost | Very expensive | Relatively low cost |
Time | Long and extensive | Relatively fast |
Example | Clinical trials and animal studies | Organ-on-a-chip, , cell-based screening, diagnostic tests |
Pros | More specific and reliable for observing biological effects in a test subject | Relative simplicity, species specificity, experimental control |
Cons | Strict regulations and compliance standards | Physiologically limited |
What is in vitro testing?
Organ-on-a-chip, microphysiological system, what is in vivo testing.
Humans and animals share a lot of anatomical and physiological features with comparable functions. Consequently, animal testing and clinical trials make up the bedrock of in vivo studies involving drug discovery.
Animal models (preclinical)
Before a drug candidate is tested in human subjects, it must undergo rigorous animal testing to further evaluate its toxicity, metabolism and overall efficacy. Vertebrate animal models such as rodents, primates, dogs and rabbits are commonly used for preclinical in vivo studies. Mice are the most used vertebrate species due to a combination of factors such as size, ease of handling, fast reproduction and low cost. Mice also share 95% of their genes with humans, 5 which makes them very attractive models for studying human diseases. These factors contribute to their usefulness when it comes to evaluating the safety and efficacy of drug candidates at the preclinical stage.
Human trials (clinical)
Once a drug candidate has demonstrated sufficient efficacy and safety during animal studies, human studies may commence. Researchers at this stage are interested in assessing whether a treatment is safe and effective for its intended population of subjects. Humans who partake in a clinical trial will be closely monitored to evaluate the drug metabolism and pharmacokinetic qualities of the experimental therapy over time. These trials need to perform such analyses with continued monitoring afterward so that drug candidates can be thoroughly evaluated to be safe and effective.
References: 1. Lorian V. Differences between in vitro and in vivo studies. Antimicrob Agents Chemother . 1988;32(10):1600-1601. doi: 10.1128/AAC.32.10.1600 2. Gillette JR. Problems in correlating In vitro and In vivo studies of drug metabolism. In: Benet L.Z., Levy G., Ferraiolo B.L. (eds) Pharmacokinetics . Springer, Boston, MA. 1984. doi: 10.1007/978-1-4613-2799-8_19 3. Miwa T, Kanda M, Umeda S, et al. Establishment of peritoneal and hepatic metastasis mouse xenograft models using gastric cancer cell lines. In Vivo . 2019;33(6):1785-1792. doi: 10.21873/invivo.11669 4. Lee J, Kim S. Kidney-on-a-Chip: A new technology for predicting drug efficacy, interactions, and drug-induced nephrotoxicity. Curr Drug Metab . 2018;19(7):577-583. doi: 10.2174/1389200219666180309101844 5. Pennacchio LA. Insights from human/mouse genome comparisons. Mamm Genome. 2003;14(7):429-436. doi: 10.1007/s00335-002-4001-1
What is in vitro testing? In vitro testing is an essential component of biological research that provides a way to study the response of human, animal or microbial cells in culture.
What is in vivo testing? Humans and animals share a lot of anatomical and physiological features with comparable functions. Consequently, animal testing and clinical trials make up the bedrock of in vivo studies involving drug discovery.
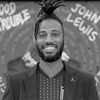

In Vitro vs In Vivo Preclinical Studies
- Download PDF Copy
Before a drug candidate can be tested in humans, its safety and efficacy must be explored in preclinical studies. These studies can either be ‘ in vitro ’, Latin for “ within the glass” and referring to studies using cell cultures studied outside of the body, or ‘ in vivo ’, Latin for “within the living”, referring to studies that take place in the body.
In preclinical studies, only animals are used for in vivo tests as this stage comes prior to human testing to ensure its safety.
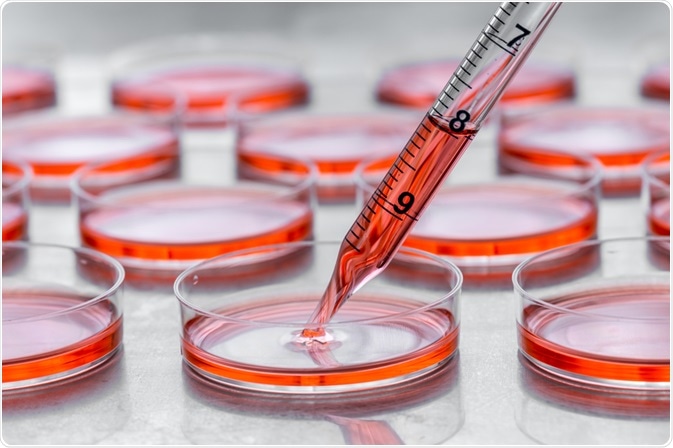
For in vitro studies, cell lines are derived from either humans or non-human animals and are introduced to the new pharmaceutical under development within a Petri dish or test tube. In vitro studies have many benefits. The first, and most obvious benefit is that they do not cause harm to the animal or person that the cell cultures have been derived from, they are free of the drawbacks of animal testing. Other benefits of in vitro models include their relative cheapness in set up and running; they are also reliable and efficient, and produce robust results.
However, while in vitro studies offer numerous significant benefits, they have one major drawback. In vitro studies are limited as they cannot model how a pharmaceutical compound may interact with all the molecules and cell types that exist within a complex organ. They can only be tested in isolation, whereas the human body is a dynamic environment where numerous pathways and cells are in constant communication. This can make it difficult for in vitro studies to predict the complexities of potential interactions.
Despite this limitation, in vitro studies remain a key part of preclinical research. Here, we will look at the example of in vitro testing in preclinical studies for new cancer therapeutics to show how in vitro studies are moving forward and how technology is developing to help overcome the limitations of in vitro studies.
Historically, preclinical in vitro cancer models have faced the drawback of being ineffective at reliably predicting patient outcomes. While in vitro testing in cell-based models has always been a fundamental part of cancer research, these preclinical studies have not always been successful at producing data that reflect the exact behavior of malignancies in response to candidate drugs.
To address this issue, scientists are working on developing the technology used in these trials. In particular, technology is now emerging that is based on more complex co-culture technology using live cells in vitro .
Recent decades have seen a shift from 2-dimensional cell culture toward 3-dimensional cell growth, an emerging technique that is more effective at capturing the physiologic environment and better reflects the growth patterns of tumor tissue. This new technology is able to provide three-dimensional architecture and preserved heterogeneity of tumor cells observed in vitro . In comparison to 2-D cell cultures, it is more accurate at demonstrating the complex microenvironments and surrounding stromal components.
In vivo studies, in comparison to in vitro , take place within a living organism. In preclinical trials, this happens within animal subjects. In clinical trials, in vivo studies can use either humans or animals as subjects,
Related Stories
- Mapping the Microbiome: Dr. Abidemi Junaid on the Groundbreaking Vagina Chip
In vivo studies are able to address the major limitation of in vitro studies, they are able to demonstrate the impact of a pharmaceutical on the body as a whole, rather than how it impacted isolated cells. This allows in vivo studies to better visualize potential interactions, which can improve its predictions of safety, toxicity , and efficacy. This helps scientists predict the impact of candidate drugs on human disease.
However, while in vivo studies address the drawback of in vitro studies, they have their own major setback. In vivo studies face significant ethical concerns, particularly for preclinical studies where just animal models are permitted. The debate over the ethics of animal testing has raged for decades.
Currently, the regulations and laws governing animal testing are tightening, and preclinical in vivo studies scientists wishing to conduct preclinical studies with animals are required to demonstrate that no other alternative methodology can be used to conduct the experiment. They are also required to demonstrate a sense of balance, that is, the benefits of the study (gain in knowledge) outweigh the drawbacks (suffering caused to the animals).
Like in vitro studies, in vivo studies are also undergoing a technological transformation. Emerging technologies such as CRISPR will make complex animal models increasingly simple to conduct, cheaper, and faster.
While in vivo studies face significant ethical considerations, it is likely that they will remain a fundamental part of preclinical studies. The future is predicted to bring significant advances in preclinical technologies, both in vitro and in vivo , that should facilitate the gathering of more accurate data, as well as faster and simpler methodologies. It is expected that these advances will improve the quality of preclinical data, as well as reduce the reliance on traditional animal models.
- Gargiulo, G., 2018. Next-Generation in vivo Modeling of Human Cancers. Frontiers in Oncology , 8. https://www.ncbi.nlm.nih.gov/pmc/articles/PMC6192385/
- Højelse, F., 2000. Preclinical Safety Assessment: In vitro - in vivo Testing. Pharmacology & Toxicology , 86, pp.6-7. https://pubmed.ncbi.nlm.nih.gov/10905745/
- Lorian, V., 1988. Differences between in vitro and in vivo studies. Antimicrobial Agents and Chemotherapy , 32(10), pp.1600-1601. https://www.ncbi.nlm.nih.gov/pmc/articles/PMC175930/
Last Updated: Feb 23, 2021
Sarah Moore
After studying Psychology and then Neuroscience, Sarah quickly found her enjoyment for researching and writing research papers; turning to a passion to connect ideas with people through writing.
Please use one of the following formats to cite this article in your essay, paper or report:
Moore, Sarah. (2021, February 23). In Vitro vs In Vivo Preclinical Studies. News-Medical. Retrieved on September 08, 2024 from https://www.news-medical.net/life-sciences/In-Vitro-vs-In-Vivo-Preclinical-Studies.aspx.
Moore, Sarah. "In Vitro vs In Vivo Preclinical Studies". News-Medical . 08 September 2024. <https://www.news-medical.net/life-sciences/In-Vitro-vs-In-Vivo-Preclinical-Studies.aspx>.
Moore, Sarah. "In Vitro vs In Vivo Preclinical Studies". News-Medical. https://www.news-medical.net/life-sciences/In-Vitro-vs-In-Vivo-Preclinical-Studies.aspx. (accessed September 08, 2024).
Moore, Sarah. 2021. In Vitro vs In Vivo Preclinical Studies . News-Medical, viewed 08 September 2024, https://www.news-medical.net/life-sciences/In-Vitro-vs-In-Vivo-Preclinical-Studies.aspx.
Cancel reply to comment
- Trending Stories
- Latest Interviews
- Top Life Sciences Articles

How can microdialysis benefit drug development
Ilona Vuist
In this interview, discover how Charles River uses the power of microdialysis for drug development as well as CNS therapeutics.

Global and Local Efforts to Take Action Against Hepatitis
Lindsey Hiebert and James Amugsi
In this interview, we explore global and local efforts to combat viral hepatitis with Lindsey Hiebert, Deputy Director of the Coalition for Global Hepatitis Elimination (CGHE), and James Amugsi, a Mandela Washington Fellow and Physician Assistant at Sandema Hospital in Ghana. Together, they provide valuable insights into the challenges, successes, and the importance of partnerships in the fight against hepatitis.

Addressing Important Cardiac Biology Questions with Shotgun Top-Down Proteomics
In this interview conducted at Pittcon 2024, we spoke to Professor John Yates about capturing cardiomyocyte cell-to-cell heterogeneity via shotgun top-down proteomics.

Latest Life Science News

Newsletters you may be interested in

Your AI Powered Scientific Assistant
Hi, I'm Azthena, you can trust me to find commercial scientific answers from News-Medical.net.
A few things you need to know before we start. Please read and accept to continue.
- Use of “Azthena” is subject to the terms and conditions of use as set out by OpenAI .
- Content provided on any AZoNetwork sites are subject to the site Terms & Conditions and Privacy Policy .
- Large Language Models can make mistakes. Consider checking important information.
Great. Ask your question.
Azthena may occasionally provide inaccurate responses. Read the full terms .
While we only use edited and approved content for Azthena answers, it may on occasions provide incorrect responses. Please confirm any data provided with the related suppliers or authors. We do not provide medical advice, if you search for medical information you must always consult a medical professional before acting on any information provided.
Your questions, but not your email details will be shared with OpenAI and retained for 30 days in accordance with their privacy principles.
Please do not ask questions that use sensitive or confidential information.
Read the full Terms & Conditions .
Provide Feedback
In vivo experiment
An in vivo experiment is a principle-testing experiment run in the context of an academic course. It is a laboratory-style multi-condition experiment conducted in the natural setting of student course work including the classroom, computer lab, study hall, dorm room, home, etc. The conditions in an in vivo experiment manipulate a small but crucial, well-defined instructional variable, as opposed to a whole curriculum or educational policy. How in vivo experimentation is related to other methodologies in the learning and educational sciences is illustrated in the following figure:
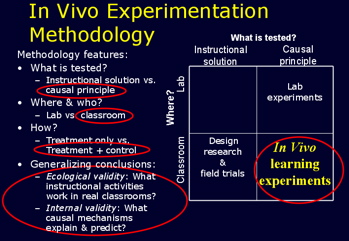
In vivo experimentation is different from other methodologies in the learning and educational sciences including 1) design-based research, which does not have control conditions, 2) randomized field trials, which do not test a principle, but a policy or curriculum, and 3) lab experiments, which are not run in a natural course setting. Of course, all of these methodologies have strengths and should be applied at the right time for the right purpose.
The motivation for the contrast with laboratory experimentation was well articulated by Russ Whitehurst in an APS Observer article (March, 2006) : "In contrast to learning in laboratory settings, learning in classrooms typically involves content of greater complexity and scope, delivered and tested over much longer periods of time, with much greater variability in delivery, and with far more distraction and competition for student time and effort. Before principles of learning from cognitive science can be applied to classroom instruction, we need to understand if the principles generalize beyond well controlled laboratory settings to the complex cognitive and social conditions of the classroom."
An in vivo experiment can be implemented either in a within classroom design (i.e. students are randomly assigned to conditions, regardless the class they belong to) or in a between classroom design (i.e. classes are randomly assigned to conditions).
In typical in vivo experiments within PSLC, educational technology (like an intelligent tutoring system or an on-line course) is used not only to provide part of the instruction, but also to monitor students' activities and progress through the year. The conditions in an in vivo experiment may be implemented within an advanced educational technology or outside of it. We strive to have the control condition be exactly what students would do normally in the course. We call this an ecological control group . Sometimes, however, the control activity is somewhat different from normal activity so as to be more like the treatment (i.e., just on change away). Here are a couple examples (to make this easier to follow, assume that there are just two conditions in the experiment, called the experimental and control conditions):
- Technology-based in vivo experiment with an ecological control : Here the educational technology is modified to implement the experimental manipulation (by changing just one aspect of the technology). Students assigned to the experimental condition do their work on the modified system. Students assigned to the control condition use the unmodified system, which is what they would normally do (see ecological control group ). Post-practice reflection (Katz) is an example of this approach.
- Activity-based in vivo experiment without an ecological control: For a limited time (e.g., a one-hour classroom period or a two-hour lab period), the control students do one activity and the experimental students do another (which, again, has a single principled difference from the control activity). In the Hausmann Study , for instance, students in both conditions watched videos of problems being solved by their instructor, but in the experimental condition students were prompted to self-explain steps of solutions whereas they were not in the control condition. While this manipulation did not change the advanced technology regularly used in this course (the Andes Physics tutor), the technology was in use and provided data on student performance. While the control in the Hausmann Study was much like normal practice in that it was the same content and instructor, it was not a strict ecological control in that students did not watch instructor videos in normal instruction.
Regardless of the experimental method used in an in vivo experiment, the educational technologies involved record log data that are used to evaluate the effects of the manipulation.
In vivo experiments are not new, for instance, Aleven & Koedinger (2002). Going back further, there have been many classroom studies that have had important features of in vivo experiments. For example, below are summaries from two dissertations that were the basis of the famous Bloom (1984) 2-sigma paper. These dissertations involved 6 experiments. These experiments are borderline in vivo experiments because mastery and, particularly, tutoring are not "small well-defined instructional variables". Instead, these treatments actually varied a number of different instructional methods at one time. Nevertheless, they illustrate a number of other important features of in vivo experimentation.
Burke’s (1983) experiments measured immediate learning, far transfer and long-term retention . This dissertation involved three classroom experiments comparing conventional instruction, mastery learning and 3-on-1 human tutoring. E1 taught 4th graders probability; E2 taught 5th graders probability; E3 taught fifth graders probability at a different site. Instruction was 3-week module. Tutors were undergrad education students trained for a week to ask good questions and give good feedback (pg. 85). The mastery learning students had to achieve 80% to go on; the tutoring students had to achieve 90%. The conventional instruction students got no feedback from the mastery tests. On immediate post-testing, for lower mental process (like a normal post-test , table 5 pg. 98) tutoring effect sizes averaged 1.66 (with 1.53, 1.34 and 2.11 for E1, E2 and E3, respectively) and mastery learning averaged 0.85 (with .73, .78 and 1.04 for E1, E2 and E3). For higher mental process (like far transfer , Table 6 pg. 104), got for tutoring effect sizes averaged 2.11 (with 1.58, 2.65 and 2.11) and mastery learning averaged 1.19 (with 0.90, 1.47 and 1.21). For long-term retention 3 weeks later; in E3, the effect size for tutoring was 1.71 for lower mental processes vs. 1.01 for mastery learning. For higher mental processes, effect size was 1.99 for tutoring vs. 1.13 for mastery learning. The researchers also measured time on task (tutoring was higher percentage) and affect rates.
The Anania (1981) thesis involved three classroom experiments, each lasting 3 weeks, comparing conventional teaching, mastery learning and tutoring. The tutors were undergrad education students with no training in tutoring. E1 taught probability to 4th graders; E2 taught probability to 5th graders; E3 taught cartography to 8th graders. E1 and E2 used 3-on-1 tutoring; E3 used 1-on-1 tutoring. Measured immediate learning post-test, time on task, and affect measures. For gains (table 3, pg. 72), effect sizes for tutoring were 1.93 (average of 1.77, 2.06 and 1.95 for E1, E2 and E3) and for mastery learning were 1.00 (avg of 0.61, 1.29 and 1.10). The cutoff score for mastery learning as 80% (pg. 77) vs. 90% for tutoring (pg. 81).
- Aleven, V., & Koedinger, K. R. (2002). An effective metacognitive strategy: Learning by doing and explaining with a computer-based Cognitive Tutor. Cognitive Science, 26(2).
- Anania, J. (1981). The Effects of Quality of Instruction on the Cognitive and Affective Learning of Students. Unpublished PhD, University of Chicago, Chicago, IL.
- Bloom, B.S. (1984). The 2-sigma problem: The search for methods of group instruction as effective as one-to-one tutoring. Educational Researcher, 13, 4-16.
- Burke, A. J. (1983). Student's Potential for Learning Contrasted under Tutorial and Group Approaches to Instruction. Unpublished PhD, University of Chicago, Chicago, IL.
- Koedinger, K.R., Aleven, V., Roll, I., & Baker, R. (2009). In vivo experiments on whether supporting metacognition in intelligent tutoring systems yields robust learning. In D.J. Hacker, J. Dunlosky, & A.C. Graesser (Eds.), Handbook of Metacognition in Education (pp. 897-964). The Educational Psychology Series. New York: Routledge.
- Koedinger, K. R., Corbett, A. C., & Perfetti, C. (2010). The Knowledge-Learning-Instruction (KLI) framework: Toward bridging the science-practice chasm to enhance robust student learning. CMU-HCII Tech Report 10-102. Accessible at [1] .
- Klahr, D., Perfetti, C. & Koedinger, K. R. (2009). Before Clinical Trials: How theory-guided research can inform educational science. Symposium at the second annual conference of the Society for Research on Educational Effectiveness. See [2] .
- Salden, R. J. C. M. & Aleven, V. (2009). In vivo experimentation on self-explanations across domains. Symposium at the Thirteenth Biennial Conference of the European Association for Research on Learning and Instruction.
- Salden, R. J. C. M. & Koedinger, K. R. (2009). In vivo experimentation on worked examples across domains. Symposium at the Thirteenth Biennial Conference of the European Association for Research on Learning and Instruction.
- VanLehn, K. & Koedinger, K. R. (2007). In vivo experimentation for understanding robust learning: Pros and cons. Symposium at the annual meeting of the American Educational Research Association.
Navigation menu
Personal tools.
- View source
- View history
- Instructional Principles
- Publications
- Recent changes
- Random page
- Cognitive Factors
- Social and Communicative Factors in Learning
- Metacognition and Motivation
- Computational Modeling and Data Mining
- Coordinative Learning
- Interactive Communication
- Refinement and Fluency
- Feature Wish List
other links
- LearnLab Home Page
- Summer School
- PSLC People
- PSLC Grad Students
- PSLC_Postdocs
- What links here
- Related changes
- Special pages
- Printable version
- Permanent link
- Page information
- This page was last edited on 8 September 2011, at 12:43.
- Privacy policy
- About LearnLab
- Disclaimers

Stack Exchange Network
Stack Exchange network consists of 183 Q&A communities including Stack Overflow , the largest, most trusted online community for developers to learn, share their knowledge, and build their careers.
Q&A for work
Connect and share knowledge within a single location that is structured and easy to search.
What is an 'ex vivo' experiment?
Simple terminology question:
- Is there a hard boundary between in vitro and ex vivo ?
- Is there a hard boundary between in vivo and ex vivo ?
Suppose a sensory neuron is electrically recorded in the following settings:
- Surgically accessing through the animal's skin, and patch-clamped,
- Tissue surrounding the neuron is almost separated, but still dangling from the larger part of the animal, and patch-clamped,
- Same as above, but the slight dangling connection is cut,
- The sensory neuron is isolated and put in an artificially oxygenated medium
The sensory neuron is assumed to have purely feedforward signal, and is perfectly healthy in all conditions. Which conditions, if any, are in vivo , in vitro , or ex vivo ? (Feel free to propose better hypothetical experiments. I know this example is not the best.)
Am I the only one confused about these terminologies?
- terminology
- experimental
- 1 $\begingroup$ It's simpler and broader than that. All depends on the source material for the experiment, if you're talking about experiments: in vivo: animal models ex vivo: cultured cells in vitro: purified molecules $\endgroup$ – kjf Commented Mar 18, 2019 at 19:30
- $\begingroup$ The terminologies are there to be helpful shorthands, i.e. they are not mutually exclusive terms and are not strictly defined jargon. Case 1 is in vivo , the rest are not. Concerning Case 2, removing the environment of a neuron cannot guarantee its native state as it exists in the organism, so it is not an in vivo experiment (at least not to most electrophysiologists). In vitro is just a shorthand for where it's taking place. You can simulate an observation on a computer in silico , or make observations on a plate in vitro . If you can't be clear with a shorthand, don't use it! $\endgroup$ – S Pr Commented Mar 19, 2019 at 13:47
4 Answers 4
Ex vivo simply means "outside the normal, living organism", whereas in vitro means "within glass", usually in a cultured system. They are not exactly same as there is no need for the work to be done in a culture system, although both are not in vivo . Harvested tissue could be examined ex vivo without being in a test tube, or surgical systems could be tested ex vivo on artificial organ models. In vivo requires the natural, living organism, although it depends on just how much you are perturbing the natural.
To answer your scenarios,
- ex vivo , as it is not within the normal organism, although I could understand someone taking issue with that; definitely not in vivo , which is usually for normal conditions only, even including temperature.
- If you mean the artificially oxygenated environment of a tissue culture system, then in vitro ; if you mean the artificially oxygenated environment of a lab bench with an open $O_2$ valve, then ex vivo would suffice.

- $\begingroup$ Comment to top answer (from Amory): in vivo is not for normal conditions only, half of the times it's not in the "normal" "natural" conditions, that's how you can learn something, you always have to compare a mutated version or diseased version to a healthy control, all is in vivo anyway (when it's in vivo), regardless of the conditions. So in the example 1, are you trying to say that if it is the mouse model for Alzheimer disease, for example, then it's not in vivo anymore?! Also, "vivo" doesn't mean "living organism", it just means "living". $\endgroup$ – kjf Commented Mar 21, 2019 at 14:57
- $\begingroup$ @kjf That was intended to convey the typical case of a living organism. Cell culture is living and is an organism, but it is not a "normal organism." It was intended to be colloquial, as noted where I say "although it depends on just how much you are perturbing the natural." $\endgroup$ – Amory Commented Mar 21, 2019 at 19:28
(Not only) Wikipedia states that in vitro and in vivo have a very hard boundary between them. I something is studied in the context of an organism, without being extracted, it is in vivo , as soon as it is extracted it is in vitro .
Strictly speaking, I would count only the first of your neuron examples as in vivo , because it is still expected to be working like it does inside the organism. As soon as you start having it dangling out, the original "environment" is destroyed.
The relation between ex vivo and in vitro is less defined. I would count example 2 as ex vivo , but not in vitro , as the Wikipedia article for ex vivo describes. Same goes for example 3.
Example 4 is of course in vitro . Debatable is, if in vitro excludes ex vivo . It is still taken out of an organism, not grown artificially, so I would still consider it ex vivo .
if you check articles in different fields, chemists and biochemists will usually say cultured cells is in vivo ("the molecules we always study in the tube are now inside a living cell!"), clinical doctors will say it's in vitro ("we take some cells from the patient and put them in a tube"), cell biologists working with clinical samples, animal models, cell cultures, purified molecules, the whole set, will say it's ex vivo ("the molecules that we study are in a living cell, but that living cell that we also study is out of the organism, ex vivo!").
- 2 $\begingroup$ Can you add some reliable references please? $\endgroup$ – L.B. Commented Mar 21, 2019 at 16:52
The term in vivo refers to a medical test, experiment or procedure that is done on (or in) a living organism, such as a laboratory animal or human.
The term in vitro, in contrast to in vivo, refers to a medical study or experiment which is done in the laboratory within the confines of a test tube or laboratory dish.
In contrast to in vitro studies, in vivo studies are needed to see how the body as a whole will respond to a particular substance. In some cases in vitro studies of a drug will be promising, but subsequent in vivo studies fail to show any efficacy (or, on the other hand, find a drug to be unsafe) when used within the multiple metabolic processes that are continually taking place in the body.

- $\begingroup$ Please provide a citation or link to supporting evidence. Thanks! $\endgroup$ – theforestecologist ♦ Commented Mar 21, 2019 at 18:52
You must log in to answer this question.
Not the answer you're looking for browse other questions tagged terminology experimental ..
- Featured on Meta
- Bringing clarity to status tag usage on meta sites
- Announcing a change to the data-dump process
Hot Network Questions
- Why isn't a confidence level of anything >50% "good enough"?
- Has anyone returned from space in a different vehicle from the one they went up in? And if so who was the first?
- Generate all the free polyominoes who's width and height is no larger than 8
- Would two switches in parallel in the same box meet code?
- Setting the desired kernel in GRUB menu
- Generating function for A261041
- Nothing to do with books but everything to do with "BANGS"!
- Book Request: Machshava by Topics
- Is "She played good" a grammatically correct sentence?
- How to raise and lower indices as a physicist would handle it?
- Where is this railroad track as seen in Rocky II during the training montage?
- What qualifies as a Cantor diagonal argument?
- How does a miner create multiple outputs in a coinbase transaction?
- Sum[] function not computing the sum
- Instance a different sets of geometry on different parts of mesh by index
- Do US universities invite faculty applicants from outside the US for an interview?
- Information theoretical interpretation of Free Energy
- Is this host and 'parasite' interaction feasible?
- What is the optimal number of function evaluations?
- Best approach to make lasagna fill pan
- Are fuel efficiency charts available for mainstream engines?
- What is the first work of fiction to feature a vampire-human hybrid or dhampir vampire hunter as a protagonist?
- What is the relationship between language and thought?
- What prevents random software installation popups from mis-interpreting our consents
Advertisement
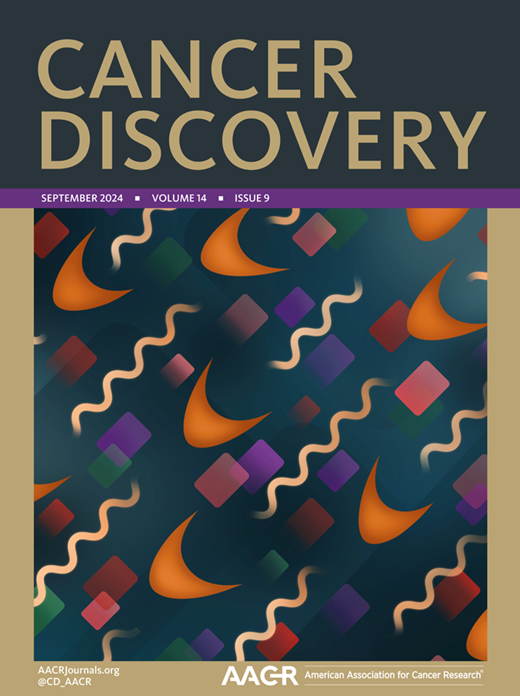
- Previous Article
- Next Article
Introduction
Authors’ disclosures, authors’ contributions, acknowledgments, a next-generation braf inhibitor overcomes resistance to braf inhibition in patients with braf -mutant cancers using pharmacokinetics-informed dose escalation.

Cancer Discov 2024;14:1599–611
- Funder(s): National Cancer Institute (NCI)
- Award Id(s): P30 CA008748

- Split-Screen
- Article contents
- Figures & tables
- Supplementary Data
- Peer Review
- Open the PDF for in another window
- Get Permissions
- Cite Icon Cite
- Search Site
- Version of Record September 4 2024
- Accepted Manuscript May 1 2024
Rona Yaeger , Meredith A. McKean , Rizwan Haq , J. Thaddeus Beck , Matthew H. Taylor , Jonathan E. Cohen , Daniel W. Bowles , Shirish M. Gadgeel , Catalin Mihalcioiu , Kyriakos P. Papadopoulos , Eli L. Diamond , Keren B. Sturtz , Gang Feng , Stefanie K. Drescher , Micaela B. Reddy , Bhaswati Sengupta , Arnab K. Maity , Suzy A. Brown , Anurag Singh , Eric N. Brown , Brian R. Baer , Jim Wong , Tung-Chung Mou , Wen-I Wu , Dean R. Kahn , Sunyana Gadal , Neal Rosen , John J. Gaudino , Patrice A. Lee , Dylan P. Hartley , S. Michael Rothenberg; A Next-Generation BRAF Inhibitor Overcomes Resistance to BRAF Inhibition in Patients with BRAF -Mutant Cancers Using Pharmacokinetics-Informed Dose Escalation. Cancer Discov 1 September 2024; 14 (9): 1599–1611. https://doi.org/10.1158/2159-8290.CD-24-0024
Download citation file:
- Ris (Zotero)
- Reference Manager
RAF inhibitors have transformed treatment for patients with BRAF V600 -mutant cancers, but clinical benefit is limited by adaptive induction of ERK signaling, genetic alterations that induce BRAF V600 dimerization, and poor brain penetration. Next-generation pan-RAF dimer inhibitors are limited by a narrow therapeutic index. PF-07799933 (ARRY-440) is a brain-penetrant, selective, pan-mutant BRAF inhibitor. PF-07799933 inhibited signaling in vitro , disrupted endogenous mutant-BRAF:wild-type-CRAF dimers, and spared wild-type ERK signaling. PF-07799933 ± binimetinib inhibited growth of mouse xenograft tumors driven by mutant BRAF that functions as dimers and by BRAF V600E with acquired resistance to current RAF inhibitors. We treated patients with treatment-refractory BRAF -mutant solid tumors in a first-in-human clinical trial (NCT05355701) that utilized a novel, flexible, pharmacokinetics-informed dose escalation design that allowed rapid achievement of PF-07799933 efficacious concentrations. PF-07799933 ± binimetinib was well-tolerated and resulted in multiple confirmed responses, systemically and in the brain, in patients with BRAF -mutant cancer who were refractory to approved RAF inhibitors.
Significance: PF-07799933 treatment was associated with antitumor activity against BRAF V600 - and non-V600-mutant cancers preclinically and in treatment-refractory patients, and PF-07799933 could be safely combined with a MEK inhibitor. The novel, rapid pharmacokinetics (PK)-informed dose escalation design provides a new paradigm for accelerating the testing of next-generation targeted therapies early in clinical development.
Oncogenic BRAF mutant protein monomers are categorized into Class I (monomers), II (Ras-independent, constitutively activated dimers), and III (Ras-dependent, hypoactive dimers) based on their activities as monomers or homo/heterodimers ( 1 , 2 ). Approved RAF kinase inhibitors are potent against Class I BRAF V600 mutants, which function as monomers. Despite effective immediate pathway suppression, long-term benefit is limited by several potential mechanisms: (i) adaptive induction of ERK signaling, (ii) clinical resistance most commonly arising from new genetic alterations that induce BRAF dimerization, and (iii) poor brain penetration enabling intracranial disease progression. In addition, approved RAF inhibitors cause paradoxical activation of ERK signaling in BRAF wild-type cells, allowing combination with MEK or EGFR inhibitors to both mitigate the resulting on-target toxicities and improve efficacy. However, the combination partners introduce additional toxicities, and resistance remains universal. Genetic causes of resistance are best understood in melanoma and largely converge on the development of RAF dimers. Resistance alterations include dimer-inducing BRAF splice variants, BRAF gene amplification, and RAS mutations in the setting of founder BRAF V600 mutations. Further, non-V600 dimer-forming BRAF Class II/III founder mutations (including fusions) have been identified in ∼4% to 8% of all solid tumors ( 3 – 13 ).
Next-generation RAF inhibitors have been developed with the goal of inhibiting ERK activation by BRAF mutants that form dimers as well as monomers. Many are pan-RAF dimer inhibitors that preclinically inhibit signaling from not only BRAF mutants but also all three wild-type RAF proteins. However, emerging clinical data demonstrate that efficacy is limited by on-target toxicity from pan-wild-type RAF inhibition, which limits combinability with MEK inhibitors and requires both intermittent dosing and dose modifications ( 14 – 19 ).
Selective inhibitors of BRAF-containing dimers such as plixorafenib (PLX-8394, FORE8394) also maintain activity against BRAF mutants, including V600 and non-V600 mutants ( 20 ). Because such compounds also spare CRAF homodimers and ARAF-containing dimers, they have the advantage of maintaining MAPK signaling in normal tissue. However, plixorafenib is subject to CYP3A4-dependent metabolism, thus requiring coadministration with the CYP3A inhibitor cobicistat to reach efficacious exposure ( 21 ). Furthermore, cobicistat cotreatment unfavorably increases hepatotoxicity and hampers rational combinations with MEK inhibitors, many of which are CYP3A4 substrates ( 22 , 23 ).
PF-07799933 (ARRY-440) is a next-generation, selective pan-mutant BRAF inhibitor that is not a pan-RAF inhibitor, does not possess the metabolic liability of plixorafenib, can be combined with MEK inhibitors, and is brain-penetrant. Given the significant unmet need faced by patients with BRAF -mutant cancers after the failure of available treatments, we aimed to implement a data-informed dose escalation approach in the first-in-human phase 1 trial to achieve therapeutic exposures in less time and with fewer patients overall.
PF-07799933 Disrupts Mutant BRAF Dimers to Overcome Diverse BRAF Mutants Preclinically
PF-07799933 (ARRY-440), an orthosteric, pan-mutant BRAF inhibitor, was discovered by Array BioPharma. We profiled PF-07799933 and three classes of BRAF inhibitors—BRAF V600 monomer inhibitor (encorafenib), selective BRAF dimer inhibitor (plixorafenib), and pan-RAF dimer inhibitors (belvarafenib, tovorafenib, naporafenib, and exarafenib)—in 1-hour assays of phosphorylated ERK (pERK), across 19 patient-derived cancer cell lines ( 20 , 24 – 27 ). These lines were BRAF wild-type or harbored founder mutations in BRAF , including Class I (i.e., V600E/K), Class II (i.e., L597V/R and G469A), or Class III (i.e., G466V and D594G) alterations ( 1 , 2 ), and indels (V487-P492, N486-P490, and L485-P490) with a different mechanism of activation ( 28 , 29 ). We also included two BRAF V600E -mutant melanoma cell lines harboring commonly occurring acquired resistance alterations identified in patients with melanoma after treatment with approved BRAF inhibitors: BRAF splice variant (p61) or RAS mutation ( NRAS -Q61K; Supplementary Table S1 summarizes each cell line with its specific BRAF mutation; refs. 3 , 4 ).
PF-07799933 demonstrated broad inhibition of pERK levels in cell lines harboring Class I (IC 50 = 0.7–7 nmol/L), II (10–14 nmol/L), III (0.8–7.8 nmol/L), and indel (113–179 nmol/L) mutants, acquired BRAF p61 splice variant (59 nmol/L), and acquired NRAS Q61K (16 nmol/L), but significantly spared pERK in BRAF wild-type cells (≥9,800 nmol/L; Fig. 1A ). Unsupervised hierarchical clustering demonstrated that PF-07799933 was most similar to plixorafenib, though with better potency. Encorafenib occupied a distinct branch, with more pERK inhibitory activity against Class I BRAF -mutant cell lines (IC50 = 3.4–58 nmol/L) than cell lines with Class II (40–2,700 nmol/L), Class III (308–990 nmol/L), indel (6.2–154 nmol/L), BRAF V600E + p61 splice variant (322 nmol/L), or BRAF V600E + NRAS Q61K (172 nmol/L) mutant cell lines. Pan-RAF dimer inhibitors clustered together based on similar response profiles across the cell lines evaluated, with similar pERK inhibitory activity against wild-type and mutant BRAF . In BRAF wild-type cells, PF-07799933 demonstrated no pERK inhibition, in contrast to the pan-RAF dimer inhibitors, and less paradoxical activation of pERK than encorafenib ( Fig. 1A ; Supplementary Fig. S1A). Isothermal stability shift dose–response assays (ITDR) showed that PF-07799933 bound BRAF V600E with a 10-fold higher affinity compared with wild-type CRAF protein in A375 BRAF V600E -mutant melanoma cell lysates (Supplementary Fig. S1B).
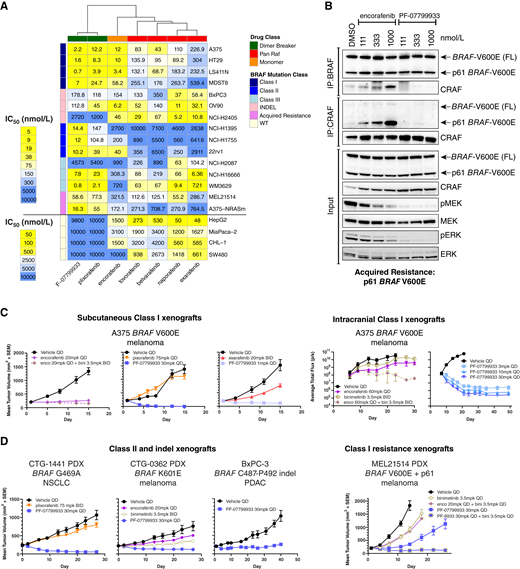
Preclinical characterization of pan-mutant BRAF selective monomer/dimer inhibitor PF-07799933. A, Heatmap of 50% inhibitory concentration (IC 50 ) values for inhibition of pERK in human cancer cell lines for PF-07799933 and comparator RAF inhibitors, as derived from dose–response curves in Supplementary Fig. S1. Each cell line with specific BRAF mutation is summarized in Supplementary Table S1. Note color scaling for BRAF wild-type cell lines is distinct from BRAF -mutant cell lines. Unsupervised clustering of compounds is shown using Euclidean distance as a similarity metric. B, Immunoprecipitation of BRAF and CRAF dimer complexes in the MEL21514 (p61 BRAF splice variant) melanoma cell line. Comparison of dimer-breaking effects of PF-07799933 and encorafenib are shown at indicated drug concentrations for a 1-hour incubation period. C, Efficacy curves of mean tumor volumes in mice ( n = 8–10) bearing subcutaneous xenografts (left) and change in flux measurements of intracranial xenografts (right) of Class I A375 ( BRAF V600E ) melanoma cells following oral treatment with the indicated agents. D, Efficacy curves of mean tumor volumes in mice ( n = 8–10) bearing subcutaneous patient-derived or cell line xenografts of Class II, indel, and Class I acquired resistance models following oral treatment with the indicated agents. IC 50 , 50% inhibitory concentration; nM, nanomolar; INDEL, insertion/deletion; WT, wild-type; IP, immunoprecipitated; FL, full-length; mm 3 , cubic millimeter; SEM, standard error of mean; QD, once daily; BID, twice daily; mpk, milligrams per kilogram; NSCLC, non–small cell lung cancer; PDAC, pancreatic adenocarcinoma.
To gain insight into the mechanism of mutant BRAF inhibition by PF-07799933, we immunoprecipitated endogenous BRAF/CRAF protein complexes with either BRAF- or CRAF-specific antibodies, in two BRAF V600E -mutant melanoma cell lines with an acquired BRAF p61 splice variant (in cis with the de novo V600E allele; Fig. 1B ) or with NRAS Q61K (Supplementary Fig. S1C), both of which promote mutant BRAF-dimer formation. As previously described, the approved BRAF monomer inhibitor encorafenib increased mutant- BRAF :wild-type- CRAF complexes in a dose-dependent manner ( Fig. 1B ; Supplementary Fig. S1C; refs. 30 – 32 ). In contrast, heterodimeric complexes containing wild-type CRAF with BRAF V600E or the BRAF V600E ; p61 splice variant were disrupted by PF-07799933 ( Fig. 1B ; Supplementary Fig. S1C). Notably, PF-07799933 failed to disrupt wild-type- BRAF :wild-type- CRAF dimer complexes in BRAF wild-type cells with exogenously expressed wild-type BRAF and wild-type CRAF (Supplementary Fig. S1D). The ability to break endogenous, mutant- BRAF :wild-type- CRAF dimers without disrupting wild-type- BRAF :wild-type- CRAF dimers contributes to the enhanced potency, efficacy, and safety of PF-07799933.
We also solved the cocrystal structures of the BRAF kinase domain with encorafenib and PF-07799933 (Supplementary Fig. S2A and S2B; Supplementary Table S2). Compared with encorafenib, PF-07799933 induced an ordered A-loop and caused an outward rotation in the αC-helix, both consistent with an inactive kinase conformation that may destabilize RAF dimers or prevent dimerization and reduce RAF affinity to MEK due to conformational hindrance. Based on published structures of RAF kinases, plixorafenib may also change the position of the αC-helix to disrupt select dimers ( 20 ).
In vivo antitumor activity was assessed in mouse xenografts harboring representative BRAF mutations and treated orally with different agents at doses selected to approximate the clinically achievable human exposures (when human data were available). PF-07799933 monotherapy drove deeper regressions than encorafenib + binimetinib in mouse xenografts harboring the founder BRAF V600E (Class I) mutation implanted subcutaneously [ Fig. 1C (left)] or intracranially [ Fig. 1C (right)]. PF-07799933 treatment also caused regressions of subcutaneously implanted BRAF G469A (Class II)-mutant NSCLC, BRAF K601E (Class II)-mutant melanoma and BRAF indel-mutant pancreatic cancer tumors [ Fig. 1D (left)]. In contrast, plixorafenib was less efficacious against BRAF V600E - and BRAF G469A -mutant models, consistent with less in vitro pERK inhibition and known in vivo metabolic vulnerability ( 23 , 33 ). Similarly, consistent with less in vitro pERK inhibition, exarafenib was less active against the BRAF V600E -mutant model [ Fig. 1C (left)]. For plixorafenib and exarafenib, we confirmed exposures achieved in animals approximated those achieved in humans (Supplementary Fig. S3A and S3B). Thus, the observed decreased efficacy relative to PF-07799933 ± binimetinib was not due to decreased exposures relative to clinically achievable doses in humans. In a BRAF V600 -mutant melanoma patient-derived xenograft (PDX) model harboring an acquired BRAF p61 splice variant, the combination of encorafenib + binimetinib did not improve the minimal antitumor activity of binimetinib monotherapy. However, single agent PF-07799933 demonstrated superior activity, and its combination with binimetinib further augmented efficacy, resulting in tumor regression, while remaining well-tolerated without body weight loss [ Fig. 1D (right)].
In summary, these data indicate that PF-07799933 is a brain-penetrant, selective, pan-mutant BRAF inhibitor. PF-07799933 inhibited pERK in vitro in cells driven by BRAF V600E -mutant monomers, BRAF Class II/III-mutant dimers, and treatment-acquired genetic alterations that induce mutant- BRAF V600E dimerization. Furthermore, PF-07799933 disrupted endogenous mutant- BRAF :wild-type- CRAF dimers in cells containing BRAF V600E + p61 splice variant or BRAF V600E + NRAS Q61K that induce mutant BRAF dimerization. However, PF-07799933 spared pERK and did not disrupt BRAF -wild-type: CRAF -wild-type dimers in vitro in BRAF wild-type cells. Finally, PF-07799933 had broad in vivo antitumor activity, systemically and in the brain, against BRAF V600E and non-V600 mutant proteins as monotherapy, and against BRAF V600E with a treatment-acquired BRAF p61 splice variant in combination with binimetinib.
Characteristics of the Patients
From August 2022 to July 2023, 30 patients with BRAF -mutant solid tumors at nine centers in the United States and Canada were treated with PF-07799933 at 50 mg QD ( n = 4 monotherapy), 150 mg QD [ n = 4 monotherapy, n = 4 + binimetinib, n = 4 + cetuximab (all colorectal cancer, CRC)], 225 mg BID ( n = 5 monotherapy, n = 4 + binimetinib), and 450 mg BID ( n = 5 monotherapy; Supplementary Table S3). Tumor types were melanoma ( n = 13/43%), CRC ( n = 5/17%), primary brain ( n = 4/13%), thyroid ( n = 3/10%), and breast adenoid cystic carcinoma (ACC), salivary gland adenocarcinoma, cholangiocarcinoma, esophageal squamous carcinoma, and NSCLC ( n = 1/3% each). BRAF mutations were Class I (V600E; 22/73%), Class II (4/13%), and Class III (4/13%). All patients with BRAF V600E -mutant cancers were previously treated with an approved BRAF inhibitor regimen [ n = 8/22 (36%) ≥2 lines; Supplementary Table S3]. All patients eligible for an immune checkpoint inhibitor (ICI) for an approved indication (including all patients with BRAF V600E -mutant melanoma) also received previous treatment with an ICI (Supplementary Table S3).
PK-Informed Dose Escalation Enables Rapid Achievement of Therapeutic PF-07799933 Dose
Traditional first-in-patient phase 1 dose escalation designs enroll patients in fixed dose levels of a single anticancer drug based on observed toxicities (DLT). This risks underdosing many patients early on who cannot benefit because the dose is suboptimal and prevents potential benefit from rationally selected combination partners. Therefore, we developed a flexible approach that considered both observed toxicity and actual drug exposures to determine the amount of each dose increase ( Fig. 2A ).
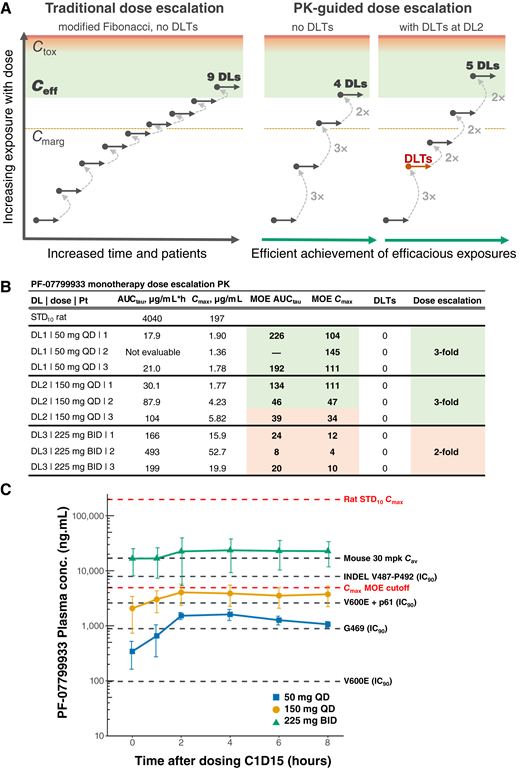
PF-07799933 safety and rapid PK-guided dose escalation. A, Examples of traditional (left) vs. PK-guided dose escalation (right) for a drug with a wide therapeutic index based on preclinical data and available clinical data for similar drugs. With traditional dose escalation (example of modified Fibonacci dose escalation design with decreasing dose increments at higher dose levels), even without DLTs, it can require many dose levels and patients to reach a potentially efficacious exposure ( C eff ). In contrast, PK-guided dose escalation may require fewer dose levels, time, and patients to reach a potentially efficacious exposure. An example shown is a threefold dose increase in the absence of DLTs and if drug exposures are lower than the potentially toxic exposure ( C tox ) by at least a prespecified safety margin ( C marg ); otherwise, a twofold dose increase. B, Preliminary C max , AUC tau , and MOE values for each patient on Cycle 1 Day 15 (C1D15) of dosing for patients with available PK at the time of dose escalation. At a given dose level, if MOEs (rat value at STD 10 /human value for C max and AUC tau ) are >40 in at least two out of three participants, and no DLTs are observed at the current and all prior dose levels, a threefold dose increase is allowed; otherwise, the maximum dose increase is twofold. C, Preliminary plasma concentration vs. time data (mean ± standard deviation for n = 3–5 per dose level) on C1D15. Horizontal reference lines show plasma concentrations for IC 90 BRAF -mutant protein target coverage based on the cell-based pERK assay, the average concentration ( C av ) in the mouse xenograft at a 30-mg/kg dose (shown to be efficacious for multiple tumor types), the C max at the rat (sensitive tox species) STD 10 and the C max at the cutoff allowing a threefold dose increase (i.e., C max /40). To enroll patients with symptomatic brain metastases, C trough on C1D15 had to exceed the G469A IC 90 in at least one-half of patients, achieved at 150 mg QD. PK, pharmacokinetics; DLT, dose-limiting toxicity; DL, dose level; PT, patient; AUC tau , area under the dose–response curve to end of dosing interval; MOE, margin of exposure; mg, milligrams; QD, daily; BID, twice daily; ng, nanograms; conc, concentration; ml, milliliters; STD 10 , severely toxic dose in 10% animals; mpk, milligrams per kilogram; C av , average concentration; C max , maximum concentration; IC 90 , 90% inhibitory concentration.
First, we defined safe steady-state C max and AUC tau in patients based on the nonclinical toxicology of PF-07799933 (see “Methods”). Next, we limited each allowable dose increase to 2×, with the actual dose increase determined by the Bayesian logistic regression model (BLRM)/escalation with overdose control (EWOC) model based on the DLT rate. However, if no DLTs were observed, and the ratios of nonclinical steady-state C max and AUC tau to the values observed in patients were >40 in ≥2/3 patients, the dose increase could be 3×. This margin of exposure (MOE) and lower-than-required starting dose (50 mg QD) maximized patient safety and were possible due to the preclinical potency and pharmaceutics properties of PF-07799933 (Supplementary Methods). We also allowed intraparticipant dose escalation and/or rational addition of binimetinib or cetuximab (for patients with CRC) after progression on PF-07799933 monotherapy or, for combination, with PF-07799933-related toxicity known to be ameliorated by combination (“Methods”; ref. 34 ).
No DLTs were observed, and steady-state C max /AUC tau MOEs for each of the first two dose levels (50 mg QD, 150 mg QD) were >40 in 2/3 treated patients ( Fig. 2B ). This enabled sequential threefold dose increases across the first three dose levels (50 mg QD → 150 mg QD → 225 mg BID) for a ninefold increase in total daily dose. PF-07799933 achieved drug concentrations in patients at 225 mg BID (dose level 3) that exceeded inhibitory concentrations for multiple BRAF mutants based on the cell-based pERK assay for the duration of the dosing interval, and approximated concentrations associated with 30 mg/kg QD dosing in mice ( Fig. 2C ).
Although no DLTs occurred at 225 mg BID, MOEs were <40 for all patients, limiting the next dose increase to twofold (i.e., 450 mg BID). At this dose, PF-07799933 showed high interpatient PK variability and lower exposures in 3/4 patients than for patients treated at 225 mg BID, likely due to dose-limited absorption (Supplementary Fig. S4). Therefore, this dose was not investigated further and a new formulation is being introduced (see Discussion).
Efficacy according to investigator assessments as of August 24, 2023, for 28 patients with measurable disease at baseline and ≥1 post-baseline imaging assessment who initiated PF-07799933 as monotherapy ( n = 16, 6 added binimetinib sequentially per protocol, see Methods) or in combination with binimetinib ( n = 8) or cetuximab ( n = 4, all CRC) is summarized in Fig. 3A and Supplementary Fig. S5A. All patients were refractory to standard systemic therapies, and all patients with BRAF V600E -mutant cancers previously received approved BRAF inhibitor treatments (Supplementary Table S3).
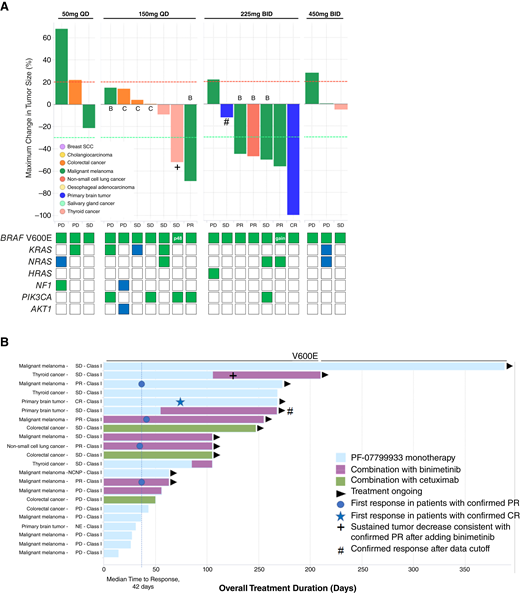
Efficacy in patients with BRAF V600E /Class I-mutant cancer. A, A waterfall plot of the maximum change in tumor size by treatment, dose, and tumor. Preliminary summary of select cooccurring mutations (known activating for oncogenes, known inactivating for tumor suppressor genes, green—tumor, blue—ctDNA) is shown at the bottom (see Supplementary Table S5). B, A swimmer plot representing the overall treatment duration. Two patients are not shown in A due to the absence of a target lesion ( n = 1) or the absence of postbaseline imaging assessment ( n = 1). + indicates patient with BRAF V600E + thyroid cancer who achieved sustained tumor decrease consistent with a confirmed PR with the addition of binimetinib, after progression on PF-07799933 monotherapy. # indicates patient with BRAF V600E + primary brain tumor who achieved a confirmed PR after the data cutoff. Note: response categories are per RECIST 1.1 except for primary brain tumor, which is categorized by RANO. B, binimetinib combination; C, cetuximab combination; CR, complete response; PR, partial response; SD, stable disease; PD, progressive disease; NE, not evaluable; NCNP, non-CR/non-PD; p48, BRAF p48 splice variant; gain, BRAF copy number gain.
For 20 patients with BRAF V600 -mutant cancers who initiated treatment with PF-07799933 monotherapy ( n = 12, 3 added binimetinib) or combination with binimetinib ( n = 5) or cetuximab ( n = 3, all CRC), overall response rate (ORR) increased with PF-07799933 starting dose from 50 mg QD to 225 mg BID: 0/3 responses by RECIST 1.1 or RANO at 50 mg QD, 1/7 at 150 mg QD, and 4/7 at 225 mg BID, including one complete response (CR; Fig. 3A ). A fifth patient (with BRAF inhibitor-refractory BRAF V600E + primary brain tumor) treated with 225 mg BID + binimetinib [latter added for PF-07799933 monotherapy-related adverse event (AE) per protocol] achieved an ongoing, confirmed partial response after the data cutoff. There were 0/3 responses at 450 mg BID, a dose limited by variable and low PK as described above. Efficacy was observed across tumor types. The median duration of treatment was 3.5 months (range 0.5–12.9). The median duration of response was not reached (range 2.8+, NE), and 4/5 responders remained on treatment and in response as of the data cutoff ( Fig. 3B ).
Two patients are summarized in detail in Fig. 4 . First, a patient with BRAF inhibitor-refractory BRAF V600E -mutant thyroid cancer with a BRAF p48 splice variant experienced ongoing, sustained tumor reduction up to −80% (consistent with a confirmed PR) upon the addition of binimetinib, after experiencing an unconfirmed partial response (PR) followed by PD on PF-07799933 150 mg QD monotherapy ( Fig. 4A–D ). Sustained efficacy in combination with binimetinib was consistent with preclinical in vivo efficacy experiments in a splice variant-containing model and may result from more sustained inhibition of MAPK signaling in the tumor cells [ Fig. 1D (right); see “Discussion”]. Second, a patient with a BRAF V600E -mutant primary brain tumor refractory to two lines of BRAF + MEK inhibitors experienced an ongoing confirmed CR with PF-07799933 225 mg BID monotherapy ( Fig. 4E–G ).
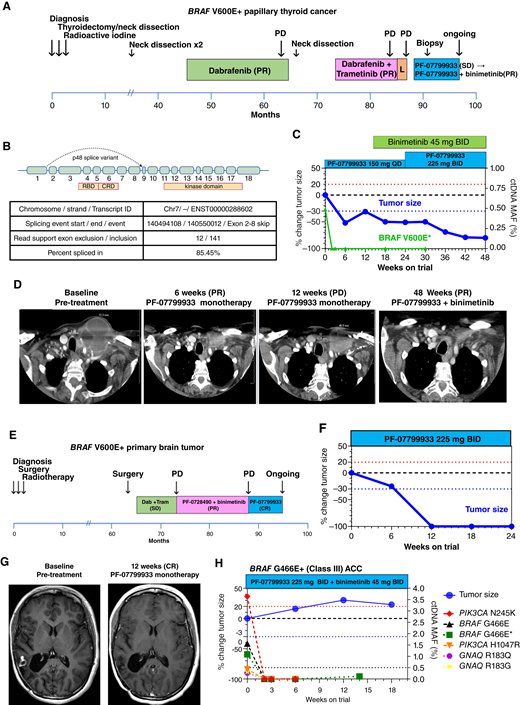
PF-07799933 overcomes de novo and acquired resistance and intracranial progression in patients with BRAF -mutant cancer. A, Previous therapies (best overall response to each systemic therapy shown in parenthesis) and timing of lymph node biopsy that showed a p48 splice variant for a patient with BRAF V600E + papillary thyroid cancer. B, Structure of the BRAF p48 in-frame BRAF splice-variant detected by tumor mRNA analysis. The table shows details of the spliced mRNA identified in 15% of all BRAF transcripts in a biopsy sample with 13% V600E MAF (Supplementary Table S5), likely representing the acquisition of the splice variant in cis in all V600E transcripts. C, Change in the sum of longest tumor diameters of target lesions (blue, normalized to baseline) and in BRAF V600E ctDNA (green). Note: ctDNA assay cannot detect splice variants in mRNA. Imaging for weeks 36 to 48 was obtained after the data cutoff. D, Images of a large left neck target lesion mass during treatment. E, Previous therapies for a patient with a BRAF V600E + primary brain tumor. PF-07284890 is an investigational, brain-penetrant BRAF monomer inhibitor. F, Change in the sum of target lesion diameters (no ctDNA was detected in this patient). G, Images of a right temporal lobe target lesion during treatment. H, Change in the sum of the longest tumor diameters and mutations detected in ctDNA for a patient with BRAF G466E -mutant ACC. * indicates BRAF V600E or BRAF G469A detected with BRAF gene-specific ctDNA assay. ACC, adenoid cystic carcinoma; CR, complete response; PR, partial response; SD, stable disease; PD, progressive; L, lenvatinib; MAF, mean allele frequency.
Among eight patients with BRAF Class II ( n = 4) or Class III ( n = 4) mutations treated with PF-07799933 monotherapy ( n = 4, 3 added binimetinib) or combination with binimetinib ( n = 3) or cetuximab ( n = 1, CRC), there were no responses (Supplementary Fig. S5A). However, only one patient received PF-07799933 treatment at a dose of 225 mg BID and in combination with binimetinib. This patient, with treatment-refractory, BRAF G466E (Class III)–mutant breast ACC, with previous progression on seven lines of systemic therapy and without acceptable alternative treatment options, experienced clinical improvement and a molecular CR in ctDNA despite radiographic PD, and remains on treatment beyond PD, as permitted by protocol ( Fig. 4H ; Supplementary Fig. S5B; see “Discussion”).
PF-07799933 was well-tolerated as monotherapy or combination. There were no DLTs and the MTD was not reached; ≥1 treatment-emergent AE (TEAE) with monotherapy and combination occurred in 94% and 100% of patients, respectively, and was grade ≥3 in 28% and 44%, respectively. TEAEs reported in ≥3 patients regardless of attribution are shown in Supplementary Table S4. The most common TEAEs for monotherapy were fatigue (any grade 44%/grade ≥3 0%), headache (28%/0%), vision blurred [22%/6% (a single patient with grade 3)], and lipase increased (16%/0%). The most common TEAEs for combinations were peripheral edema (33%/0%), acneiform rash, diarrhea, and fatigue (each 28%/0%). A single patient treated with PF-07799933 at 450 mg BID monotherapy had their dose reduced for AEs of vision blurred, peripheral sensory neuropathy, myalgia, fatigue, and decreased appetite. 6/8 AEs of blurred vision resolved without dose modification, and there were no abnormal findings reported on repeat ophthalmic examinations. No patient discontinued treatment for a study drug-related AE.
Although approved BRAF monomer inhibitors (in combination with MEK or EGFR inhibitors) have transformed treatment for patients with BRAF V600 -mutant cancer, long-term efficacy is limited by adaptive reactivation of ERK signaling, resistance from BRAF dimer formation in tumor cells, and poor brain penetration. Investigational pan-RAF dimer inhibitors overcome resistance preclinically, but many require intermittent dosing and dose modifications that may limit clinical activity and combinability ( 14 , 15 , 17 – 19 ). Recently, the pan-RAF dimer inhibitor tovorafenib dosed once weekly was associated with durable responses in pediatric patients with BRAF fusion+ or BRAF V600E + low-grade glioma ( 35 ). However, efficacy was lower in patients with recent prior MAPK inhibitor treatment and in patients with other tumor types, and treatment was still associated with frequent dose reductions and interruptions due to toxicities from pan-RAF inhibition ( 16 , 35 – 37 ). Although the selective BRAF dimer inhibitor plixorafenib overcomes some liabilities preclinically, it requires codosing with cobicistat, which may limit combinability with MEK inhibitors and other drugs that are also metabolized by CYP3A4 ( 21 – 23 ).
PF-07799933 was developed to address all of these liabilities. Here, we present preclinical experiments that confirmed significant inhibition of pERK in BRAF -mutant cells, significant sparing of pERK in BRAF wild-type cells, and combinability with a MEK inhibitor. PF-07799933 monotherapy demonstrated strong antitumor activity, systemically and in the brain, against de novo BRAF V600E (Class I) and non-V600E BRAF (Class II, III, or indel) mutant tumors and, in combination with binimetinib, against a de novo BRAF V600E- + acquired BRAF p61 splice variant mutant tumor.
Traditional phase 1 trials would have explored PF-07799933 monotherapy with a dose escalation design that only considered observed toxicity. This strategy was necessary in an era when most anticancer treatments were nonspecific, toxic, and often only modestly effective. However, for a highly specific agent against a credentialed oncogenic target, it risks exposing many patients to subtherapeutic doses early on and ineffective monotherapy later. Therefore, we implemented a novel, safety- and PK-guided dose escalation design that enabled rapid achievement of an efficacious dose, early intrapatient dose escalation, and early rational combination ( Fig. 2 ). The AE profile of PF-07799933 was generally similar, but of lower grade, to that of approved BRAF inhibitors, and the lack of DLTs allowed for two sequential threefold dose increases across the first three dose levels. In particular, AEs of blurred vision as seen with approved BRAF and MEK inhibitors were primarily of low grade, without findings on ophthalmic examinations, and most resolved without dose modification. This safety profile, along with contemporaneous PK profiling, accelerated dose escalation to quickly achieve evidence of clinical activity in patients with BRAF V600E –mutant solid tumor, systemically and in the brain, after failure of approved BRAF + MEK inhibitors, and in the presence of acquired resistance mutations. To our knowledge, this includes the first evidence of efficacy in a patient with a BRAF splice variant ( Fig. 4A–D ). Although the total number of patients is small, PF-07799933 at 225 mg BID ± binimetinib achieved an ORR of 57% (4/7, not including a fifth patient with confirmed response after data cutoff) for patients with BRAF inhibitor-refractory patients with BRAF V600E solid tumors. This response rate compares favorably to that of exarafenib [ORR = 17% (1/6), at its recommended dose for expansion of 300 mg BID], and of plixorafenib + cobicistat [ORR 18% (3/17)], in similar patient populations ( 14 , 23 ).
There are limitations to this study. The small number of patients and heterogeneous use of combination treatment may complicate comparisons to other RAF inhibitors. We have not yet observed tumor responses in patients with de novo BRAF Class II or III non-V600 mutations (no indel patients have been enrolled) despite clear monotherapy antitumor activity against BRAF Class II and indel-mutant mouse tumor xenografts in vivo at clinically achievable exposures of PF-07799933 [ Fig. 1D (left)]. However, few patients with BRAF non-V600 have been enrolled to date, with only one having received PF-07799933 at an efficacious dose of 225 mg BID and in combination with binimetinib. This patient achieved a molecular ctDNA CR despite radiographic PD and has continued ongoing treatment for clinical benefit beyond PD ( Fig. 4H ). For some patients, it is possible that co-occurring driver mutations in the setting of Class II and Class III BRAF mutations blunt antitumor efficacy [e.g., MYB-NUMB fusion, PIK3CA H1047R in the patients with G466E (Class III) + ACC with a molecular CR; Supplementary Fig. S5A; Supplementary Table S5]. For BRAF Class II/III expressing tumors, it is important to note that reports of the clinical activity for other investigational BRAF or pan-RAF inhibitors have been limited. For example, 17 patients with Class II BRAF fusion+ have been treated with plixorafenib, with a reported ORR of 8% (1/13 evaluable); similarly, only three Class II patients were reported to have been treated with exarafenib, yielding an ORR of 33% (1/3; refs. 14 , 23 ). Although PF-07799933 concentrations necessary for preclinical inhibitory were higher for BRAF non-V600- than BRAF V600 -mutant cancers, exposures achieved at 225 mg BID were consistent with significant pan- BRAF non-V600 target inhibition from preclinical data and provided higher pan-mutant BRAF target coverage than exarafenib or plixorafenib ( Fig. 2C ; Supplementary S3A and S3B). Upfront combination with a MEK inhibitor in additional patients and/or further dose escalation may provide even greater target coverage to address such Class II/III mutations clinically.
Increased exposures of PF-07799933 would take advantage of its favorable safety profile to drive enhanced target coverage and clinical efficacy. However, the decreased PK with high interpatient variability at a dose above 225 mg BID suggests that the initial formulation is likely affected by dose-limited absorption. A new formulation has been developed that preclinically overcomes this constraint, and preliminary clinical data indicate that this formulation may achieve higher exposures (Supplementary Fig. S4).
PF-07799933 monotherapy inhibited pERK and disrupted mutant- BRAF :wild-type- CRAF dimers in BRAF V600E + p61 splice variant+ melanoma cells in short-term assays in vitro ( Fig. 1A and B ). However, sustained tumor regression in the corresponding in vivo model and in a patient with BRAF V600E+ thyroid cancer harboring a BRAF p48 splice variant required combination with binimetinib [ Figs. 1D (right) and 4A–D ]. Efficacy across those tumors with distinct genotypes, RAF isoform levels, or resistance mechanisms, perhaps driving increased levels of RAF/MAPK signaling (including through additional RAF dimers) may require significant inhibition of RAF/MAPK signaling in the tumor cells. We have shown that this may be achieved at tolerable PF-07799933 doses in combination with binimetinib.
Despite permitting rational combination with binimetinib or cetuximab after PD on PF-07799933 monotherapy, some patients may not be able to receive sufficient combination treatment due to clinical deterioration.
Finally, current tumor response evaluation criteria do not account for sequential combination–dependent antitumor activity. This is exemplified by the patient with BRAF V600E + p48 splice variant+, who despite experiencing an ongoing −80% decrease in tumor size (relative to baseline imaging) after the addition of binimetinib, is considered to have a best overall response of SD by RECIST 1.1 due to prior PD on PF-07799933 monotherapy ( Fig. 4A–D ).
In summary, PF-07799933 demonstrated proof-of-concept antitumor activity, tolerability, and combinability in patients with BRAF -mutant cancers using a novel first-in-human phase 1 trial design that incorporated flexible dose escalation based on safety and rapid PK in patients. This included substantial tumor reductions in patients previously treated with approved BRAF inhibitor therapies (and other approved agents), with drug-acquired resistance mutations, and with brain involvement. Future development will more fully evaluate PF-07799933 as an upfront combination with a MEK inhibitor in patients with BRAF V600- and non-V600-mutant cancers with prior progression on approved targeted treatments.
Preclinical Characterization of PF-07799933 Activity
Cell lines, xenografts, and animal care.
All cells were obtained from ATCC except for A375-luciferase (Creative Biogene), WM3629 (Rockland), and MEL21514 (see below). All cell lines were cultured according to the recommended guidelines for the parental lines and used for experimentation within six passages. Efficacy studies with the CTG-1441 and CTG-0362 PDX models were performed at Champions Oncology. The MEL21514 PDX was generated from a biopsy provided by a female Hispanic patient with melanoma (51 years of age) following a relapse from Braftovi and Mektovi treatment (MT Group). BRAF V600E mutation and p61 splice variant expression in the MEL21514 PDX tumor were confirmed by whole-exome sequencing and RNA sequencing, respectively (Genewiz). For cell line development, MEL21514 PDX tumor samples were dissociated using the Miltenyi Biotec Human Tumor Dissociation Kit in combination with the gentleMACS Octo Dissociator according to the suggested hard tumor protocol. The dissociated cell suspension was then magnetically labeled with the Miltenyi Mouse Cell Depletion Kit and separated using the MACS Magnetic Separator for the enrichment of human tumor cells. Isolated MEL21514 tumor cells were pooled and established in culture using Renaissance Essential Tumor Medium/RETM (Rockland Inc.) supplemented with 10% fetal bovine serum/FBS (Hyclone), penicillin/streptomycin and cholera toxin. Following 10 passages in RETM, MEL21514 cells were grown in RPMI, supplemented with 10% FBS and 1-mmol/L sodium pyruvate. Cell lines and PDXs were authenticated by short tandem repeat profiling and regularly evaluated for Mycoplasma and murine viruses (MycoAlert, Lonza, Inc.; IDEXX; Charles River Research Animal Diagnostic Services).
All procedures performed on animals were in accordance with regulations and established guidelines and were reviewed and approved by Pfizer’s Institutional Animal Care and Use Committee. All mice were obtained at 6 to 8 weeks of age (Envigo), housed in groups of 5, and allowed a 1-week acclimation period before cancer cell inoculation. Food, water, temperature, and humidity were maintained per Pharmacology Testing Facility performance standards in accordance with the 2011 Guide for the Care and Use of Laboratory Animals (NRC) and AAALAC-International. For subcutaneous xenografts, each cell line (5 × 10 6 cells) or PDX (cell suspension prepared with Miltenyi gentleMACS) was injected subcutaneously into the right flank of female Foxn1 nu mice and allowed to grow to approximately 200 mm 3 prior to randomization by tumor size into dosing groups of eight animals. Body weight and subcutaneous tumor volume [determined by the formula (length × width 2 )/2] were recorded twice weekly. For intracranial xenografts, the A375-luciferase cell line (10,000 cells) was injected 2 mm lateral to the bregma at the bone suture line of female Foxn1 nu mice, with randomization after 7 days into dosing groups of ten animals based on tumor burden measured by total luminescence flux (photons/second) with an IVIS Spectrum In Vivo Imaging System (Perkin Elmer). Body weight and total flux were recorded twice weekly, with average total flux plotted against the day posttumor implantation.
Quantitative pERK Cell Analysis
Cells were seeded at 5 × 10 4 /well into clear, black-bottom, 96-well plates, incubated overnight at 37°C, and incubated for 1 hour with a nine-point dilution series of each inhibitor, followed by 3.7% formaldehyde fixation, methanol permeabilization, costaining with pERK and GAPDH antibodies, costaining with goat secondary antibodies conjugated to IRDye 800CW (for pERK) or IRDye 680RD (for GAPDH), and analysis of staining intensity by in-cell Western assay (LI-COR Odyssey). Signal intensity for pERK was normalized to GAPDH and the DMSO-treated control samples to generate percent of control (POC) data, which were then plotted versus compound concentration using GraphPad Prism 9 software to generate IC 50 data using a four-parameter curve fit. See Supplementary Table S6 for sources of primary antibodies.
Isothermal Stability Shift Dose Response Assays
Trypsinized A375 cell pellets were resuspended in PBS and treated with DMSO or PF-07799933 (concentrations ranging from 0.122 to 2,000 nmol/L) for 30 minutes at 37°C. Cells were heated to 50°C for 3 minutes in a PCR plate using a PTC-200 thermal cycler (MJ Research) and then promptly spun at 4,700 rpm in a swinging bucket centrifuge for 30 seconds at 4°C. Cells were lysed with three cycles of freeze–thawing in liquid nitrogen prior to adding nondenaturing buffer [10-mmol/L Na 2 PO 4 , 1.8-mmol/L KH 2 PO 4 (pH 7.4), 137-mmol/L NaCl, 2.7-mmol/L KCl, 1-mmol/L CaCl 2 , 10-mmol/L MgCl 2 , 0.02% n-dodecyl β-D-maltoside, 2× complete protease inhibitor (Sigma), and 2% phosphatase inhibitor cocktails 2 and 3 (Sigma)]. Samples were sonicated using the Bioruptor water bath sonicator (Diagenode) for 15 seconds with 30 seconds rest for 10 cycles. Proteins were denatured and cysteine residues reduced and alkylated at 95°C for 10 minutes in 50 mmol/L 4-(2-hydroxyethyl)-1-piperazineethanesulfonic acid (pH 8.0), 1% SDS, 1% Triton-X-100, 1% NP-40, 1% Tween 20, 1% sodium deoxycholate, 5-mmol/L EDTA, 50-mmol/L NaCl, 1% glycerol, 10-mmol/L tris(2-carboxyethyl)phosphine, and 40-mmol/L chloroacetamide. Protein sample clean-up was achieved using the SP3 bead method with Sera-Mag SpeedBeads (GE Healthcare), and proteins were digested with trypsin and Lys-C at 37°C for 15 hours. Individual peptide samples were labeled using isobaric Tandem Mass Tag labeling (TMTpro 16plex), combined, desalted (Waters Oasis HLB cartridge), and high pH reverse-phase fractionation was performed prior to liquid chromatography–mass spectrometry analysis.
Peptides were fractionated by direct injection on an Ionopticks Aurora Ultimate column (1.7 µm, 0.075 mm × 250 mm) at 0.2 µL/minute using an UltiMate 3000 RSLCnano System (Thermo Scientific). Mobile phases used were (A) 0.1% formic acid aqueous and (B) 0.1% formic acid acetonitrile. Peptides were gradient eluted from 2% to 5% B in 5 minutes, 5% to 30% B in 95 minutes, and 30% to 95% B in 4 minutes. Mass spectrometry analysis was performed using an Orbitrap Eclipse (Thermo Scientific). Samples were run in positive ion mode with a source spray of 1,800 V and an ion transfer tube temperature of 300°C. MS1 scans were performed in the Orbitrap mass analyzer set to a mass range of 350 to 1,800 m/z at a resolution of 120,000 and an automatic gain control target of 1 × 10 6 , normalized to 250%. MS2 fragmentation spectra were acquired using higher energy collision dissociation mode at 28% collision energy and a 1-m/z isolation window using the quadrupole. TMT 16plex reporter ions were quantified using the SPS MS3 method using higher energy collision dissociation fragmentation, multi-notch isolation, and a 2-m/z MS2 isolation window. MS3 spectra were acquired in the Orbitrap mass analyzer at 60,000 full width at half maximum resolution, a target of 1 × 10 5 , normalized to 300%, and 118 milliseconds max fill time. Protein quantification data were extracted from the ProteinGroups.txt file generated by MaxQuant (v2.1.1.0) processing of all raw files. MS2 spectra were searched using a Uniprot human database downloaded June 6, 2022, allowing for two missed cleavages with trypsin/P and LysC/P proteolytic specificity, and using variable modifications set as methionine oxidation and N-terminal protein acetylation, and fixed modifications set as cysteine carbamidomethylation. Search tolerances were set to 10 ppm for MS1 precursor ions and 20 ppm for MS2 fragment ions. Protein and peptide level false discovery rate thresholds were set to 1%, and at least one unique or razor peptide was required for protein identification, and unique plus razor peptides were used for protein quantification. Concentration–response curves were fit with R code adapted from the R package, TPP2D, ProteinGroups.txt table generated by MaxQuant.
Co-Immunoprecipitation and Immunoblotting
For co-immunoprecipitations of endogenous RAF proteins, MEL21514 or A375- NRAS Q61K cells were plated at 1.5 × 10 7 cells per 150-mm dish. Cells were incubated at 37°C, 5% CO 2 with DMSO vehicle control or indicated concentrations of encorafenib or PF-07799933 for 1 hour. Cell lysates were harvested in 1 mL per dish of magnesium lysis buffer [25-mmol/L 4-(2-hydroxyethyl)-1-piperazineethanesulfonic acid pH 7.5, 75-mmol/L NaCl, 5-mmol/L MgCl 2 , 5% glycerol, and 0.1% NP-40] supplemented with HALT protease/phosphatase inhibitor cocktail (Pierce). Lysates were clarified by centrifugation and normalized for protein concentration using the BCA assay kit (Pierce). Lysates were incubated with either BRAF (CST #53745) or CRAF (CST #14814) rabbit polyclonal antibodies at 1:100 dilution overnight at 4°C followed by incubation with protein-A conjugated magnetic beads (Dynabeads, Thermo Inc.) for 90 minutes at room temperature (22 °C). Protein immunoprecipitates were collected by magnetic separation and washed 3× with magnesium lysis buffer followed by resuspension in 1× LDS Sample Buffer (Thermo Inc.). Proteins were separated by SDS-PAGE using 4% to 20% NuPage gradient gels followed by transfer onto polyvinylidene difluoride membrane using the iBlot2 system. Membranes were incubated with indicated antibodies (see Supplementary Table S6) overnight at 4°C and imaged using enhanced chemiluminescence with West-Pico reagent (Pierce) on a Bio-Rad ChemiDoc XR imaging system. Immunoblot images were processed using Bio-Rad Image Lab and Adobe Illustrator software applications.
For co-immunoprecipitations of exogenous, wild-type RAF proteins, HEK293T cells grown in 100-mm dishes were transiently transfected with pcDNA3 vectors to enable cytomegalovirus promoter-driven expression of N-terminal fusions of GFP or V5-tagged human BRAF or CRAF; 48 hours posttransfection, cells were treated at indicated drug concentrations for 1 hour and processed as above.
BRAF Kinase Domain Protein Production, Crystallization, and Structure Determination
Long and short forms of N-terminal hexahistidine-tagged BRAF kinase domain BRAF-KD L (residues 432–726) and BRAF-KD S (residues 445–723 with three-point mutations F776A, R671A, and Y673A for solubility improvement) were expressed in insect cells. The proteins were purified by Talon affinity and Resource S ion exchange chromatography. Fractions containing BRAF were applied to Superdex 200 size exclusion chromatography columns preequilibrated with two column volumes of sizing buffer (25-mmol/L NaH 2 PO4/Na 2 HPO4 pH 7.0, 100-mmol/L NaCl, 1-mmol/L tris(2-carboxyethyl)phosphine, 0.25% CHAPS, and 15% glycerol). The elution fractions were assessed by 4% to 20% gradient SDS-PAGE, and fractions containing pure BRAF protein were pooled and concentrated to 11 mg/mL by Amicon Spin concentrator (MWCO: 10,000) before flash-freezing and storage at −80°C.
Cocrystallization was performed by mixing an equal volume of the Braf KD S : encorafenib complex and reservoir solution, which consists of 18% PEG3350, 0.2-mol/L Na 2 SO 4 , 0.1-mol/L sodium–potassium phosphate, pH 6.6 with the hanging-drop vapor diffusion method. For Braf-KD L : PF-07799933 complex crystallization, the reservoir solution contains 17% PEG5000MME, 1% PEG6000, sodium acetate, 0.2-mol/L NaCl, and 5% Tacsimate. Both crystals were harvested and flash-frozen in liquid nitrogen in a reservoir solution containing 20% (v/v) glycerol as a cryoprotectant.
Diffraction data were collected at the in-house Rigaku FRE or FRX rotating anode X-ray generators equipped with Pilatus 1-mol/L or Eiger 4-mol/L photon counting detector. Image processing and data reduction utilized iMosflm from the CCP4 package or Rigaku CrysPro software package. The initial phasing maps were computed by molecular replacement in PHASER ( 38 ) using the atomic coordinates from the isomorphous crystal of the BRAF kinase domain (PDB ID code 4MNE) as the search model. The initial atomic positions and B factors of compound-bound BRAF-KD structures were refined by successive rounds of rigid body, real-space, and B factor refinements using PHENIX ( 39 ). Encorafenib and PF-07799933 were located in SIGMA-A weighted difference mF o − DF c omit maps computed with phases from the refined models. Atomic models were then further refined by iterative manual model rebuilding into a sigma-weighted 2m|F o | − D|F c | map in COOT ( 40 ) and subsequent refinement cycles using PHENIX. Noncrystal symmetry refinement was used for the PF-07799933-bound BRAF-KD L structure. Water molecules were added progressively in the later stages of refinement. Diffraction and refinement statistics are summarized in Supplementary Table S2.
Trial Oversight
The first-in-human phase 1 trial of PF-07799933 (NCT05355701) was designed and is overseen by sponsor representatives and the investigators and is supported by the sponsor. The FDA and Institutional Review Boards from each site approved the trial, and each patient provided written informed consent. Part 1 monotherapy dose escalation and Part 2 combination dose escalation with binimetinib or cetuximab are reported here.
Key inclusion criteria were age ≥16, treatment-refractory advanced/metastatic solid tumors, BRAF V600 (Class I)/non-V600 (Class II/III) alteration in tumor and/or blood by local Clinical Laboratory Improvements Amendments laboratory test, Eastern Cooperative Oncology Group Performance Status 0 to 2, adequate end-organ function, and asymptomatic brain involvement if present. Symptomatic brain involvement was allowed once the dose of PF-07799933 achieved steady-state trough concentrations ( C trough ) consistent with BRAF mutant inhibition based on nonclinical data. Key exclusion criteria were other active malignancy, major surgery or whole-brain irradiation within 4 weeks, hemodynamically unstable thromboembolism within 12 weeks, history of retinal vein occlusion, positive pregnancy test, uncontrolled infection, significant cardiovascular disease, active condition affecting drug absorption, ongoing other anticancer or prohibited concomitant medication, and previous treatment with a BRAF dimer inhibitor.
Trial Procedures
Patients received continuous, oral, once (QD) or twice daily (BID) PF-07799933 in 21-day cycles. Dose escalation utilized a BLRM guided by the EWOC principle. A dose could only be used if the risk of excessive toxicity >0.33 was <25%; ≥2 DLT–evaluable participants were required per cohort.
No dose increase between dose levels could normally exceed more than twofold the current dose level. However, if exposures resulted in sufficient safety margins [i.e., the ratio of steady-state maximum concentration ( C max ) and area-under-the-curve-over-dosing-interval (AUC tau ) in the most sensitive nonclinical toxicology species (rat) at the severely toxic dose in 10% of animals (STD 10 ), to steady-state C max and AUC tau in ≥2/3 participants at a given dose level, was >40] and no DLTs were observed at the current and prior dose levels, the dose increase could be up to threefold over.
A participant could have PF-07799933 dose escalation or specific, rational combination therapy added [binimetinib for non-CRC or cetuximab for CRC] if (i) the dose to be escalated to or combined was allowed by BLRM/EWOC and he/she (ii) received ≥2 cycles of the current dose, (iii) experienced no AE meeting DLT criteria, and (iv) demonstrated disease progression by imaging, or (v) was experiencing a BRAF inhibitor AE ameliorated by the combination agent (e.g., skin rash, hyperkeratosis, and squamous cell carcinoma). Both intraparticipant dose escalation (including more than once) and combination addition were allowed, based on investigator preference, but each had to be done one at a time, with relevant criteria met each time.
Dose modifications and interruptions followed a prescribed algorithm. AEs were graded using Common Terminology Criteria for Adverse Events version 5.0. The response was evaluated by CT or MRI imaging using RECIST version 1.1 every 6 weeks (RANO for primary brain tumors).
Blood/plasma samples were collected prior to treatment and at defined intervals for PF-07799933 plasma concentration determination. ctDNA was prepared and analyzed using next-generation sequencing for the BRAF V600 mutation (Sysmex) or G360 73-gene panel (v 2.10, Guardant Health). DNA and RNA were extracted from formalin-fixed, paraffin-embedded tumor samples and analyzed by Tempus xT.V4 (for DNA) and Tempus RNA.v2 (for RNA).
See Supplementary Data for additional details on the rationale for starting dose and dose escalation approach, PK analysis, and molecular analysis.
The primary endpoints for Part 1 and Part 2 are first-cycle DLTs, AEs, lab, exam, and vital sign abnormalities, dose modifications, and recommended dose for expansion. Secondary endpoints include PK parameters, ORR by RECIST 1.1 (RANO for primary brain tumors), and duration of response. Exploratory outcomes include changes in ctDNA mutations.
Statistical Analysis
There is no formal hypothesis testing. Data are summarized descriptively.
Data Availability
Upon request, and subject to review, Pfizer, Inc. will provide the data that support the findings of this study. Subject to certain criteria, conditions, and exceptions, Pfizer, Inc. may also provide access to the related individual deidentified participant data. See https://www.pfizer.com/science/clinical-trials/trial-data-and-results for more information.
R. Yaeger reports grants and personal fees from Mirati Therapeutics; grants from Pfizer, Boehringer Ingelheim, and Daiichi Sankyo; personal fees from Zai Lab, Loxo@Lilly, and Revolution Medicine; grants from Boundless Bio; and personal fees from Amgen outside the submitted work. M.A. McKean reports grants from Aadi Biosciences, Alpine Immune Sciences, Arcus Biosciences, Arvinas, Ascentage Pharma Group, ASCO, Astellas, Aulos Bioscience, Bayer, Bicycle Therapeutics, BioMed Valley Discoveries, BioNTech, Boehringer Ingelheim, Bristol Myers Squibb, C4 Therapeutics, Daiichi Sankyo, Dragonfly Therapeutics, EMD Serono, Epizyme, Erasca, Exelixis, Foghorn Therapeutics, G1 Therapeutics, Genentech/Roche, Gilead Sciences, GlaxoSmithKline, IconoVir Bio, IDEAYA Biosciences, Ikena Oncology, ImmVira Pharma, Infinity Pharmaceuticals, Jacobio Pharmaceuticals, Jazz Pharmaceuticals, Kechow Pharma, Kezar Life Sciences, Kinnate BioPharma, Krystal Biotech, MedImmune, Mereo BioPharma, and Metabomed; grants and other support from Moderna; grants from NBE Therapeutics, Nektar, Novartis, NucMito Pharmaceuticals, OncoC4, Oncorus, OnKure, and PACT Pharma; grants and other support from Pfizer; grants from Plexxikon, Poseida, Prelude Therapeutics, Pyramid Biosciences, Regeneron, Remix Therapeutics, Sapience Therapeutics, Scholar Rock, Seattle Genetics, Synthrox, Takeda Pharmaceuticals, Teneobio, Tempest Therapeutics, Tizona Therapeutics, TMUNITY Therapeutics, TopAlliance Biosciences, and Xilio; and other support from Castle Biosciences, Iqvia, and Merck outside the submitted work. R. Haq reports grants and other support from Pfizer during the conduct of the study. J.T. Beck reports grants from Pfizer outside the submitted work. M.H. Taylor reports personal fees from BMS, Eisai, Blueprint Medicines, Array BioPharma, Merck, Exelixis, Genzyme, Incyte, and Regeneron outside the submitted work. J.E. Cohen reports personal fees from Roche, Medison Pharma, Merck, AstraZeneca, and Bristol Myers Squibb outside the submitted work. D.W. Bowles reports nonfinancial support from Pfizer during the conduct of the study and personal fees from Exelixis outside the submitted work. S.M. Gadgeel reports personal fees from AstraZeneca, Pfizer, Takeda, Mirati, Novartis, Bristol Myers Squibb, Genentech/Roche, Glaxo, Janssen, Merck, Esai, Arcus, Blueprint Medicines, Lilly, Regeneron, Gilead, Amgen, Bayer, Esai, and Boehringer Ingelheim outside the submitted work, as well as and AstraZeneca—Member of IDMC of a Phase III trial Merck—Travel support to attend and present at a medical conference and Mirati-Travel support to attend and present at a medical conference. C. Mihalcioiu reports grants from Pfizer and personal fees from Pfizer outside the submitted work. K.P. Papadopoulos reports other support from Pfizer during the conduct of the study, as well as other support from Abbvie, Amgen, Anheart Therapeutics, AstraZeneca, Bayer, Bicycle Therapeutics, Biontech, CytomX Therapeutics, Daiichi Sankyo, Debiopharm, F-Star, Linnaeus Therapeutics, Mirati Therapeutics, Bristol Myers Squibb, Tempest Therapeutics, Treadwell Therapeutics, Lilly, Kezar Life Sciences, Monte Rosa Therapeutics, PharmaMar, Revolution Medicine, Sensei Biotherapeutics, Storm Therapeutics, Regeneron, Incyte, and Merck outside the submitted work. E.L. Diamond reports nonfinancial support from Pfizer, Inc., during the conduct of the study and personal fees from Opna Bio outside the submitted work. K.B. Sturtz reports other support from Pfizer during the conduct of the study. G. Feng reports as an employee of and a shareholder in Pfizer, Inc. T.-C. Mou reports personal fees and other support from Pfizer during the conduct of the study and personal fees and other support from Pfizer outside the submitted work and is an employee of Pfizer. S. Gadal reports grants from Pfizer during the conduct of the study. N. Rosen reports grants from Pfizer-Array during the conduct of the study; personal fees and other support from Beigene; personal fees from MAPCure; and grants from Revolution Medicine, AstraZeneca, and Chugai outside the submitted work; in addition, N. Rosen has a patent for Biomarkers of ERK inhibition issued. J.J. Gaudino reports a patent for WO2021250521 issued. P.A. Lee reports personal fees from Pfizer, Inc., outside the submitted work. S.M. Rothenberg reports other support from Pfizer, Inc., during the conduct of the study and other support from Pfizer, Inc., outside the submitted work. No disclosures were reported by the other authors.
R. Yaeger: Data curation, supervision, investigation, writing–review and editing. M.A. McKean: Supervision, investigation, writing–review and editing. R. Haq: Supervision, investigation, writing–review and editing. J.T. Beck: Supervision, investigation, writing–review and editing. M.H. Taylor: Supervision, investigation, writing–review and editing. J.E. Cohen: Supervision, investigation, writing–review and editing. D.W. Bowles: Supervision, investigation, writing–review and editing. S.M. Gadgeel: Supervision, investigation, writing–review and editing. C. Mihalcioiu: Supervision, investigation, writing–review and editing. K.P. Papadopoulos: Supervision, investigation, writing–review and editing. E.L. Diamond: Data curation, formal analysis, investigation. K.B. Sturtz: Conceptualization, formal analysis, supervision, investigation, writing–review and editing. G. Feng: Data curation, formal analysis, investigation, project administration, writing–review and editing. S.K. Drescher: Formal analysis, investigation, writing–review and editing. M.B. Reddy: Conceptualization, supervision, writing–review and editing. B. Sengupta: Formal analysis, investigation. A.K. Maity: Formal analysis. S.A. Brown: Investigation. A. Singh: Formal analysis, supervision, investigation, writing–review and editing. E.N. Brown: Formal analysis, investigation. B.R. Baer: Formal analysis, investigation, writing–review and editing. J. Wong: Formal analysis, supervision, investigation, writing–review and editing. T.-C. Mou: Formal analysis, investigation, writing–review and editing. W.-I. Wu: Formal analysis, supervision, investigation, writing–review and editing. D.R. Kahn: Investigation. S. Gadal: Writing–review and editing. N. Rosen: Writing–review and editing. J.J. Gaudino: Supervision, investigation. P.A. Lee: Supervision, investigation, writing–review and editing. D.P. Hartley: Supervision, investigation, writing–review and editing. S.M. Rothenberg: Conceptualization, formal analysis, supervision, methodology, writing–original draft, project administration, writing–review and editing.
We thank Pfizer Boulder Research & Development, Array BioPharma, and Pfizer colleagues, including Nick Saccomano, Bin Li, Karyn Bouhana, Eric J. Hicken, Ellen R. Laird, Ray Li, William M. Old, Robb Van Gulick, and Jenn Winton. The study was funded by Pfizer, Inc. R. Yaeger was supported in part by the National Cancer Institute of the National Institutes of Health under award number P30 CA008748.
Note Supplementary data for this article are available at Cancer Discovery Online ( http://cancerdiscovery.aacrjournals.org/ ).
Supplementary data
Supplementary Methods, References
Table S1. Cell lines used for cell-based pERK assays in Figure 1A
Table S2. X-ray crystallography data collection and refinement statistics
Table S3. Demographic and clinical characteristics of the patients
Table S4. Treatment-Emergent Adverse Events (≥3 patients) with PF-07799933 monotherapy and combination therapy
Table S5. Preliminary mutation analysis
Table S6. Antibodies used for preclinical experiments
Figure S1. Preclinical characterization of PF-07799933 and comparison to other RAF inhibitors.
Figure S2. Crystal structures of BRAF kinase domain with PF-07799933 or encorafenib.
Figure S3. Mouse plasma PK profiles for exarafenib and plixorafenib.
Figure S4. Patient PK profiles for PF-07799933.
Figure S5. Efficacy for BRAF non-V600/Class II and Class III-mutant cancer patients.
Citing articles via
Email alerts.
- Online First
- Online ISSN 2159-8290
- Print ISSN 2159-8274
AACR Journals
- Blood Cancer Discovery
- Cancer Discovery
- Cancer Epidemiology, Biomarkers & Prevention
- Cancer Immunology Research
- Cancer Prevention Research
- Cancer Research
- Cancer Research Communications
- Clinical Cancer Research
- Molecular Cancer Research
- Molecular Cancer Therapeutics
- Information on Advertising & Reprints
- Information for Institutions/Librarians

- Privacy Policy
- Copyright © 2023 by the American Association for Cancer Research.
This Feature Is Available To Subscribers Only
Sign In or Create an Account
Thank you for visiting nature.com. You are using a browser version with limited support for CSS. To obtain the best experience, we recommend you use a more up to date browser (or turn off compatibility mode in Internet Explorer). In the meantime, to ensure continued support, we are displaying the site without styles and JavaScript.
- View all journals
- Explore content
- About the journal
- Publish with us
- Sign up for alerts
- Open access
- Published: 06 September 2024
ST-GEARS: Advancing 3D downstream research through accurate spatial information recovery
- Tianyi Xia 1 , 2 ,
- Luni Hu 1 , 2 ,
- Lulu Zuo 3 ,
- Lei Cao 1 , 2 ,
- Yunjia Zhang 1 , 2 ,
- Mengyang Xu ORCID: orcid.org/0000-0002-4487-7088 2 , 4 ,
- Lei Zhang 1 , 2 ,
- Taotao Pan 1 , 2 ,
- Bohan Zhang ORCID: orcid.org/0000-0001-6654-3567 1 , 2 ,
- Bowen Ma 1 , 2 ,
- Chuan Chen 1 , 2 ,
- Junfu Guo ORCID: orcid.org/0000-0002-4195-7031 3 ,
- Chang Shi 3 ,
- Mei Li ORCID: orcid.org/0000-0003-3310-2911 2 ,
- Chao Liu ORCID: orcid.org/0009-0008-6892-6754 1 , 2 ,
- Yuxiang Li ORCID: orcid.org/0000-0002-1575-3692 2 , 5 , 6 ,
- Yong Zhang ORCID: orcid.org/0000-0001-9950-1793 2 , 5 , 6 &
- Shuangsang Fang ORCID: orcid.org/0000-0002-4126-0074 1 , 2
Nature Communications volume 15 , Article number: 7806 ( 2024 ) Cite this article
1 Altmetric
Metrics details
- Bioinformatics
- Computational models
- Data processing
- Transcriptomics
Three-dimensional Spatial Transcriptomics has revolutionized our understanding of tissue regionalization, organogenesis, and development. However, existing approaches overlook either spatial information or experiment-induced distortions, leading to significant discrepancies between reconstruction results and in vivo cell locations, causing unreliable downstream analysis. To address these challenges, we propose ST-GEARS (Spatial Transcriptomics GEospatial profile recovery system through AnchoRS). By employing innovative Distributive Constraints into the Optimization scheme, ST-GEARS retrieves anchors with exceeding precision that connect closest spots across sections in vivo. Guided by the anchors, it first rigidly aligns sections, next solves and denoises Elastic Fields to counteract distortions. Through mathematically proved Bi-sectional Fields Application, it eventually recovers the original spatial profile. Studying ST-GEARS across number of sections, sectional distances and sequencing platforms, we observed its outstanding performance on tissue, cell, and gene levels. ST-GEARS provides precise and well-explainable ‘gears’ between in vivo situations and in vitro analysis, powerfully fueling potential of biological discoveries.
Similar content being viewed by others
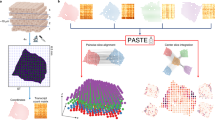
Alignment and integration of spatial transcriptomics data
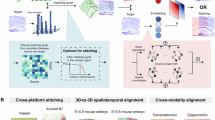
SANTO: a coarse-to-fine alignment and stitching method for spatial omics
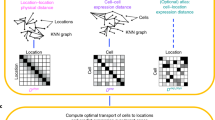
NovoSpaRc: flexible spatial reconstruction of single-cell gene expression with optimal transport
Introduction.
Spatial transcriptomics (ST) is an omics technology that fuels biological research based on measuring gene expression on each position-recorded spot across sliced tissues 1 , 2 , 3 . Notably, a range of methods has been developed. In vivo sequencing (ISS) 4 platforms such as Barcoded Anatomy Resolved by Sequencing (BARseq) 5 and Spatially-resolved Transcript Amplicon Readout Mapping (STARmap) 6 rely on amplification, hybridization and imaging process to capture gene expression information. Next Generation Sequencing (NGS) 7 platform such as Visium 1 , Stereo-seq 8 and Slide-Seq2 9 uses spatial barcoding and capturing in their implementations. These methods offer various sequencing resolutions ranging from 100 µm 10 , 11 to 500 nm 8 , and can measure thousands 5 to tens of thousands 8 of genes simultaneously.
Single-slice ST studies have unleashed discoveries, and facilitated our understanding in diverse biological and medical fields 9 , 12 , 13 , 14 , 15 . Consequently, numerous processing pipelines and analysis models have been developed for ST data on a single section 16 , 17 , 18 , 19 , 20 , 21 . However, to truly capture transcriptomics in the real-world context, three-dimensional (3D) ST was designed to recover biological states and processes in real-world dimensions, without restriction of the isolated planes in single sectional ST studies. Various research has utilized the power of 3D ST to uncover insights in homeostasis, development, and diseases. Among them, Wang et al. 22 uncovered spatial cell state dynamics of Drosophila larval testis and revealed potential regulons of transcription factors. Mohenska et al. 23 revealed complex spatial patterns in Murine heart and identified novel markers for cardiac subsections. And Vickovic et al. 24 explored cell type localizations in Human rheumatoid arthritis synovium. The vast and large variety of downstream 3D research has posted the need for a reliable and automatic recovery method of in vivo spatial profile.
However, the collection process of ST data casts significant challenges onto the accurate reconstruction of 3D ST and the situation has not been overcome by current explorations. Specifically, in 3D ST experiments, individual slices are cross sectioned in a consistent direction, then manually placed on different chips or slides 14 , 25 . This operation introduces varying geospatial reference systems of distinct sections, and coordinates are distorted compared to their in vivo states. The distortions occur due to squeezing and stretching effects during the picking, holding, and relocation of the sections. Such different geospatial systems and distortions complicates the recovery of in vivo 3D profile. Among current recovery approaches, STUtility 26 realizes multi-section alignment through the registration of histology images, without considering either geospatial or molecular profile of mRNA, which leads to compromised accuracies. Recently published method PASTE 27 , and its second version PASTE2 28 achieve alignment using both gene expression and coordinate information, through optimization of mapping between individual spots across sections. These methods cause inaccurate mappings and produces rotational misalignments due to the nonadaptive regularization factors, and their uniform sum of probability assigned to all spots upon presence of spots without actual anchors. All above approaches only consider rigid alignment, yet neglect the correction of shape distortions, resulting in shape inconsistency across registered sections. Published method Gaussian Process Spatial Alignment (GPSA) 29 considers shape distortions in its alignment, yet it doesn’t involve structural consistency in its loss function, which can cause the model to overfit to local gene expression similarities, leading to mistaken distortions of spatial information. Moreover, its hypothesis space involves readout prediction in addition to coordinates alignment, causing uncertainty in direction of gradient descent, and vulnerabilities to input noises. Another alignment approach, Spatial-linked alignment tool (SLAT) 30 also focuses on anchors construction between sections, yet it doesn’t provide a methodology to construct 3D transcriptomics profile. Other tools focus on analysis and visualization of 3d data, such as Spateo 31 , VT3D 32 and StereoPy 33 .
To address these limitations, we introduce ST-GEARS, a 3D geospatial profile recovery approach designed for ST experiments. By formulating the problem using the framework of Fused Gromov-Wasserstein (FGW) Optimal Transport (OT) 34 , ST-GEARS incorporates both gene expression and structural similarity into the Optimization process to retrieve cross-sectional mappings of spots with the same in vivo planar positions, also referred to as ‘anchors’. During this process, we introduce innovative Distributive Constraints that allow for different emphasis on distinct spot groups. The strategy addresses importance of expression consistent groups and suppresses inconsistent groups from imposing disturbances to optimization. Hence it increases anchor accuracy compared to current approaches. ST-GEARS utilizes the retrieved anchors to initially perform rigid alignment of sections. Subsequently, it introduces Elastic Field guided by the anchors to represent the deformation and knowledge to correct it according to each spot’s location. To enhance the quality of the field, Gaussian Smoothing is applied for denoising purposes. ST-GEARS then applies Bi-sectional Application to correction of each section’s spatial profile based on its denoised fields calculated with its neighboring sections. With validity proved mathematically, Bi-sectional Application eliminates distortions of sections, resulting in the successful recovery of a 3D in vivo spatial profile.
To understand effects of ST-GEARS, we first studied its counterparts with innovations including anchors retrieval and elastic registration, respectively on Human dorsolateral prefrontal cortex (DLPFC) 35 , and Drosophila larva 22 . We found an advanced anchors accuracy of ST-GEARS compared to other available methods involving anchor’s concept and unveiled Distributive Constraints as reason behind the advancement. We validated the effectiveness of elastic registration process of ST-GEARS on both tissue shape smoothness and cross-sectional consistency. Then, we studied output of ST-GEARS and other methods on their reconstruction of Mouse hippocampus tissues 36 , Drosophila embryo individual 22 and a complete Mouse brain 37 . The result was studied on morphological, cell and gene levels. ST-GEARS was found to be the only method that correctly reconstruct on all cases despite of cross-sectioning distance, number of sections, and sequencing platforms, and it was found to output the most accurate spatial information under both annotation type or clustering information, and hybridization evidence.
ST-GEARS algorithm
ST-GEARS uses ST data as its inputs, including mRNA expression, spatial coordinates as well as approximate grouping information such as clustering or annotation of each observation. Then it recovers 3D geospatial profile in following steps (Fig. 1 ).
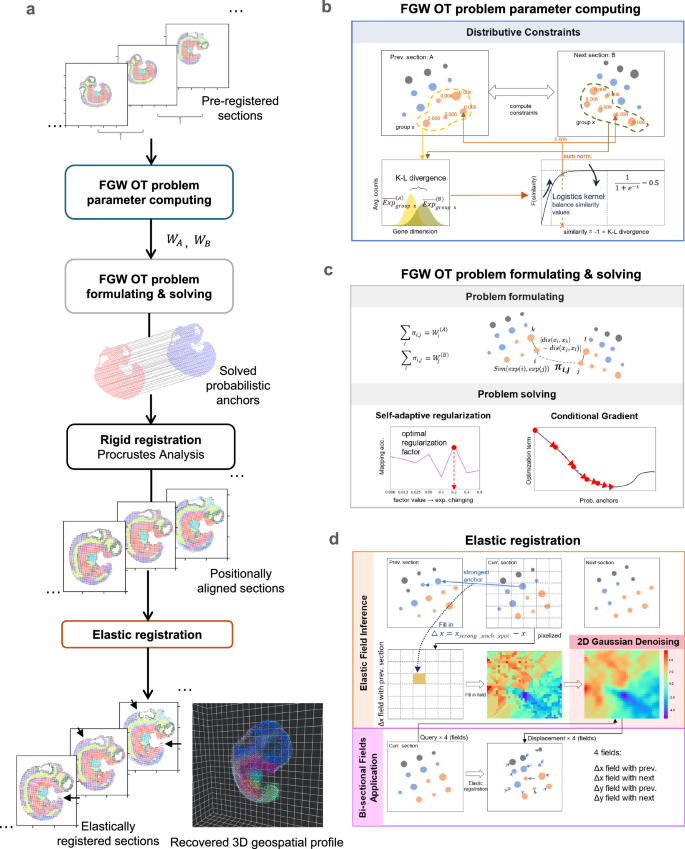
a The automatic pipeline of ST-GEARS which recovers ST-GEARS 3D in vivo spatial information by ordered steps including Fused Gromov Wasserstein (FGW) Optimal Transport (OT) problem parameter computing, problem formulating and solving which outputs probabilistic anchors across sections, rigid registration through Procrustes Analysis which solves optimal positional alignment using the anchors, and finally elastic registration. The input of the method is Unique molecular identifier (UMI) counts and location of each spot measured by ST technology, along with their annotations or cross-section clustering result. And the output of the method is recovered 3D in vivo spatial information of the experimented tissue, or sample. b FGW OT problem parameter computing, which assigns nonuniform weights to spots in preparation for future problem formulating, based on cross-sectional similarity of annotation types or clusters. c FGW OT problem formulating, whose setting aims to solve probabilistic anchors joining spots with highest in vivo proximity, through optimizing the combination of gene expression and structural similarity 34 . FGW OT problem solving, which is implemented based on Conditional Gradient (CG) method, leading to retrieved probabilistic anchors. d Elastic registration, which utilizes the anchors again to compute and denoise distortion fields which guides the elimination of distortions, then applies the fields bi-sectionally to positionally aligned sections, leading to the recovered 3D in vivo spatial information.
(1) Optimization problem formulation under scheme of FGW OT with enhancement of Distributive Constraints. FGW OT formulation is established to enable solving of ‘anchors’, which are the joining of pair of spots with same in vivo planar positions. Noticeably, each solved anchor is equipped with a probability that describes its strength of connection, and each spot is solved to have zero to multiple anchors. Among each two sections, section-specific groups of spots, and genes are initially excluded from the formulation to avoid causing disturbances to anchors computing. Considering that connected spots are more spatially approximate, and more similar in gene expression because of shared cell identity 38 , 39 , FGW was adopted to combine the gene expression and structural terms in optimization, enabling highest gene expression similarity between mapped spots, at the same time keeping similar spot positions relative to their sections. Moreover, an innovative Distributive Constraints setting is designed and integrated into FGW OT’s formulation, to assign higher emphasis on spots or cells whose annotation or cluster express high similarity across section, and vice versa. Distributive Constraints leads registration to rely more on expression-consistent regions of sections, hence largely enhancing both accuracy of anchors and precision of following rigid and elastic registration.
(2) Optimization problem solving utilizing self-adaptive regularization and conditional gradient descent. Our designed Self-adaptive Regularization strategy automatically determines the relative importance between gene expression and structural terms in the optimization problem. This strategy leads to an optimal regularization factor across different section distances, spot sizes, extent of distortions, and data quality such as level of diffusion. Conditional Gradient 34 is adopted as optimizer, which updates anchors iteratively towards higher expression and structural similarity with each iteration. The efficacy of Conditional Gradient has been demonstrated through its convergence to a local optimal point 40 , thereby ensuring the robustness and effectiveness of our approach.
(3) Rigid registration by Procrustes Analysis 41 . After filtering out anchors with relatively low probabilities, the optimal transformation and rotation of each section are analytically solved through Procrustes Analysis, which minimizes summed spatial distances of spots anchored to each other. With the transformation and rotation applied, sections are positionally aligned.
(4) Elastic registration guided by anchors. Based on rigid registration result and anchors solved by FGW OT, elastic registration is implemented through the process including elastic field inference, 2D Gaussian denoising, and bi-sectional fields application. Based on each rigidly registered section, elastic fields is inferred leveraging the location difference between its own spots and its anchored spots on anterior and posterior neighbor sections. An elastic field is a 2D displacement distribution, describing how displacement values are distributed across different locations. Making use of continuity of deformation at local scales, 2D Gaussian Denoising convolutes all over the fields to reduce noises. With denoised fields, our designed Bi-sectional Fields Application corrects each section’s deformation according to its fields calculated with anterior and posterior neighbor sections. The bi-sectional correction method is mathematically proved to approximately recover each section’s spatial profile to its original state.
Enhancement of anchor retrieval accuracy through distributive constraints
As was unfolded, ST-GEARS is an algorithm flow jointly constituted of probabilistic anchor computation and spatial information recovery. Hence, to validate the effectiveness of our method and demonstrate its underlying design philosophy, we conducted comprehensive studies on the two counterparts using real-world data. To begin, we utilized the DLPFC dataset 35 to study our anchors retrieving accuracy with emphasis on the effect of Distributive Constraints design.
To assess the effects of Distributive Constraints on anchor accuracy, we compared ST-GEARS with and without this setting, and with other constraints involving methods including PASTE, PASTE2 and SLAT. We investigated constraint values assigned by these methods, as well as their solved number of anchors and maximum anchor probability of each spot. Furthermore, we examined the annotation types that were considered connected based on the computed anchors to assess accuracy of anchors. Among the methods we compared, ST-GEARS with Distributive Constraints was found to assign different constraint values to spots within different neuron layers, while the others assigned uniform constraints to all layers (Fig. 2a , Supplementary Fig. 1 ). The results of ST-GEARS showed that both number of anchors and the anchors’ maximum probabilities for each spot were lower in Layer 2 and Layer 4 compared to the thicker layers. However, this pattern was not observed in methods without Distributive Constraints setting (Fig. 2a , Supplementary Fig. 1 ). To illustrate the impact of this strategy on anchor accuracy, we tagged each spot with annotation of its connected spot by anchor with highest probability. We then compared this result to the tagged spot’s original annotation (Fig. 2a , Supplementary Fig. 1 ). Under Distributive Constraints, ST-GEARS achieved a significantly higher proximity between annotations compared to PASTE and our method without Distributive Constraints. PASTE2 also led to approximate annotations, but it anchored multiple spots to spots from neighboring layers, particularly those near layer boundaries. SLAT also mapped multiple spots to spots from different tissue layers, particularly of spots located on layer 2, 4 and 6.
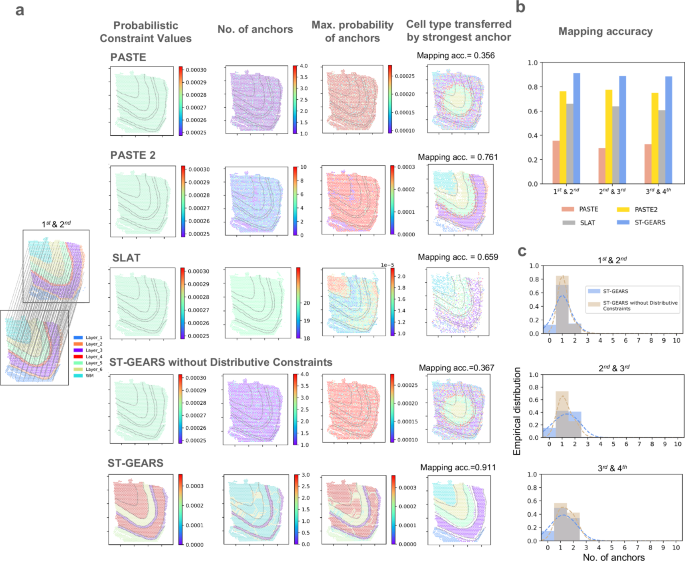
a (from left to right) 1st and 2nd human dorsolateral prefrontal cortex (DLPFC) section of patient #3 by Maynard et al. 35 with their provided annotations and our anchors showcase, (of the same section pair) probabilistic constraints settings in Optimal Transport (OT) problem formulating, no. of anchors computed on each spot, max. anchor probability value computed of each spot, and annotation type mapped back to spots through computed anchors; (from top to bottom) respectively by PASTE, PASTE2, SLAT, ours without distributive constraints setting, and ours. The distinction of different annotation types on the 1st section is marked by dotted lines. Mapping accuracy is used to measure accuracy of anchors and is marked alongside respective annotation type mapping visualizations. b Mapping accuracy measured on anchors of sections pairs used in ( b ) by PASTE, PASTE2, SLAT, and ST-GEARS. c Comparison of no. of anchors histograms between ST-GEARS and ST-GEARS without distributive constraints, of sections pairs of 1st and 2nd, 2nd and 3rd, and 3rd and 4th sections. The Probability Density Function (PDF) estimated by Gaussian kernel was plotted in dotted lines with the same color of histograms, to highlight the distribution differences. Source data are provided as a Source Data file.
To evaluate the precision of anchors, we conducted a comparison with the Mapping accuracy index introduced by PASTE 27 . This index measures the weighted percentage \({\sum}_{i,j,l(i)=l(j)}{\pi }_{{ij}}\) of anchors that connect spots with same annotation. As a result, ST-GEARS outperformed PASTE2 and SLAT, and reached a score that was over 0.5 (out of 1) higher than both PASTE and our method without Distributive Constraints (Fig. 2a , b , Supplementary Fig. 1 ).
To uncover the reasons behind the aforementioned phenomena, as the functional area in between thicker neocortical layers, thinner neocortical layers have comparable transcriptomic similarity with their adjacent layers in gene expression, than with its own annotation type 1 , 35 . This implies that, in contrast to thicker layers, thinner layers tend to introduce more disturbances during anchor computation. However, the Distributive Constraints imposed suppression on these annotation types by assigning a smaller sum of probability to each of their spots. The suppression was reflected in above results where each spot in Layer 2 and Layer 4 has fewer assigned anchors and a lower maximum probability (Fig. 2a , Supplementary Fig. 1 ). Further analysis on all spots in the DLPFC reveals that a certain percentage of spots were suppressed in anchor generation due to the Distributive Constraints (Fig. 2c , Supplementary Fig. 2 ).
Recovery of in vivo shape profile through elastic registration
We then utilized Drosophila larva data to investigate the spatial profile recovery effect of ST-GEARS, with an emphasis on our innovated elastic registration. We first applied rigid registration to Drosophila larva sections and observed a visually aligned configuration of individual sections (Supplementary Fig. 3 ). By further mapping cell annotations back to their previous sections, according to the strongest anchors of each spot, the projected annotations are visually in match with original ones (Supplementary Fig. 4 ). The accuracy of the mapping matching between annotations was quantified by Mapping accuracy (Supplementary Fig. 5 ). The above findings validated that ST-GEARS produced reliable anchors and accurately aligned sections through rigid registration. However, when stacking the sections together, we observed an inconsistency on the edge of lateral cross-section of the rigid result (Supplementary Fig. 6 ). This inconsistency doesn’t conform to the knowledge of intra-tissue and overall structural continuity of Drosophila larvae.
After applying elastic registration to the rigidly-aligned larva, we observed a notable improvement in the continuity of the cross section above, indicating a closer-to-real spatial information being retrieved. To further understand the effect of elastic operation on the dataset, we compared the changes in area of the complete body and three individual tissues (trachea, central nervous system (CNS), and fat body) on all sections. We observed an enhanced smoothness in the curves of elastically registered sections, which aligns with the continuous morphology of the larva as expected by theoretical knowledge. To quantify the smoothing effect, we calculated Scale-independent Standard Deviation of Differences ( \({SI}-{STD}-{DI}={STD}(\{{s}_{i}-{s}_{i-1}:i\in [{\mathrm{1,2}},...,I-1]\})/{|mean}(\{{s}_{i}-{s}_{i-1}:i\in [{\mathrm{1,2}},...,I-1]\})|\) ) onto the curves, which measures the smoothness of area changes along the sectioning direction (Fig. 3a and Methods). A decrease of SI-STD-DI on all tissues and the body provided empirical evidence for the improved smoothness. To further investigate the recovery of internal structures, we introduced Mean Structural Similarity (MSSIM). MSSIM takes structurally consistent sections as input, and measures pairwise internal similarity of reconstructed result using annotations or clustering information (Supplementary Fig. 7 ). (See Methods for details). An improved MSSIM was noticed on all 4 sections, indicating that elastic registration further recovers internal geospatial continuity on basis of rigid operation(Fig. 3b ). By comparing registration effect of individual sections, we also observed that the elastic process successfully rectified a bending flaw along the edge of the third section, (Fig. 3c ). The shape fixing highlighted that ST-GEARS not only yielded a more structurally consistent 3D volume, but also provided a more accurate morphology for single sections. The improved smoothness, the recovered structural continuity, and the shape fixing collectively demonstrate that elastic registration effectively recovers geospatial profile.
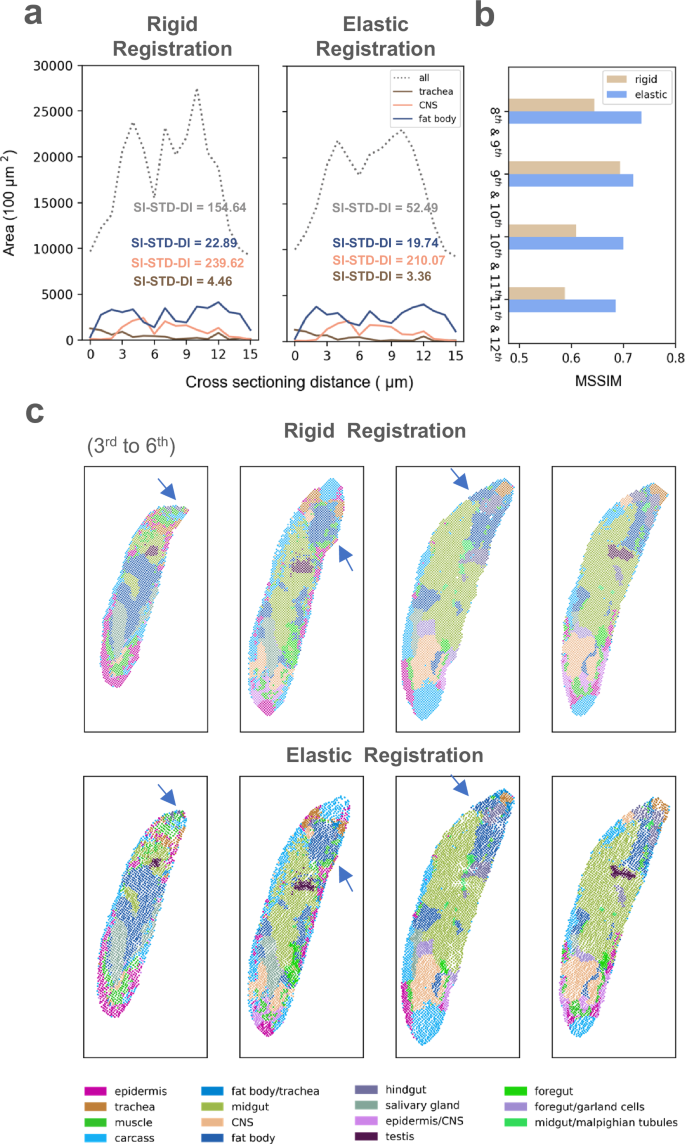
a A comparison of area changes of 3 tissues and complete body of Drosophila Larva, between result of rigid registration and result of elastic registration appended to rigid registration. The areas are calculated based on recovered spot position of different tissues along cross-sectioning direction. Standard Deviation of Differences (SI-STD-DI) quantifying the smoothness is marked alongside each curve. b A comparison of structural accuracy, measured by Mean Structural Similarity (MSSIM), of selected section pairs from Drosophila Larva (L3), between result of rigid registration only and result of elastic registration appended to rigid registration. The chosen section pairs are the structurally consistent ones. c Comparison of individual sections recovered by rigid registration only and by elastic registration appended to rigid registration, of 1st to 5th section of Drosophila Larva (L3). Shape correction of bended area in the 3 rd section, and increased cross-sectional consistency on the 4th and 5th section were highlighted by blue arrows. Source data are provided as a Source Data file.
With elastic process validated and applied onto rigid registration result, the recovery of spatial information was completed. Stacking individual sections of the elastic result, a complete geospatial profile of the larva was generated (Supplementary Fig. 8 ), visualizing the ST-GEARS’ ability of in vivo spatial information recovery.
Application to sagittal sections of Mouse hippocampus
After validating the component phases of ST-GEARS, we proceeded to apply the method to multiple real-world problems to recover geospatial profiles. We first focused on two sagittal sections of Mouse hippocampus 36 (Supplementary Fig. 9 ) that were 10 μm apart, accounting for 1–2 layers of Cornu Ammonis (CA) 1 neurons 42 . Considering the proximity of these sections, we assumed no structural differences between them.
To compare the differences of registration effect among methods, we extracted CA fields and dentate gyrus (DG) beads (Supplementary Fig. 10 ), then stacked the two sections for a more obvious contrast (Fig. 4a ). PASTE2 failed in performing the registration, leaving the sections unaligned. By GPSA, the sections’ positions were aligned, yet the 2nd section were squeezed into a narrower region than first one, leading to a contradiction of region’s location. The ‘narrowing’ phenomena may be caused by the overfitting of GPSA model on gene expression similarity, since it doesn’t involve structural similarity between registered sections in loss function. The scale on horizontal and vertical axis was distorted due to the equal scale range strategy adopted in GPSA’s preprocessing. STalign also misaligned the sections, leaving an obvious angle between two slices in registration result. This may be due to the method’s processing of ST data into images which completely relies on gene expression abundance to decide pixel intensities. On the sagittal section of Mouse hippocampus, the abundance difference between regions may not provide sufficient structural information required by registration. In the comparison between PASTE and ST-GEARS, our method demonstrates a more accurate centerline overlapping of CA fields and DG compared to PASTE. This indicated an enhanced recovery of spatial structure consistency and an improved registration effect. To quantitatively evaluate these findings, we utilized the MSSIM index as a measure of structural consistency and compared it among PASTE, PASTE2, GPSA, STalign and ST-GEARS (Fig. 4b ). Consistent with the results of centerline, ST-GEARS achieved a higher MSSIM score than GPSA and PASTE, surpassing PASTE2 and STalign by >0.2 out of 1. By comparing memory efficiency across all methods, ST-GEARS and PASTE used ~1 GB less memory than PASTE2, GPSA and STalign, and the peak memory across ST-GEARS and PASTE was almost the same (Supplementary Fig. 11 ). In perspective of time efficiency, registration utilizing ST-GEARS, STalign, GPSA and PASTE was much faster than PASTE2.
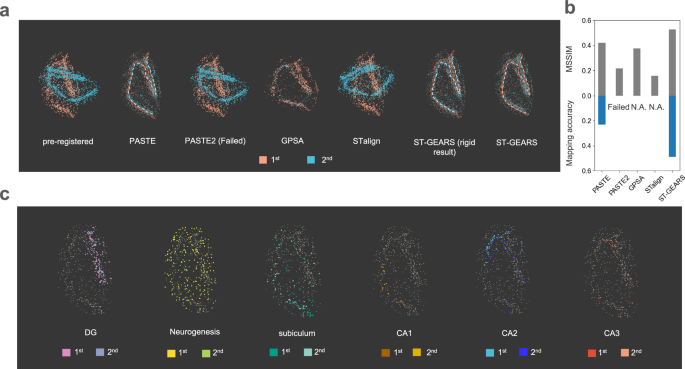
a Stacked projections of Cornu Ammonis (CA) fields and dentate gyrus (DG), of pre-registered and registered result of Mouse hippocampus sagittal sections with 10 µm distance, respectively by PASTE, PASTE2, GPSA, STalign and ST-GEARS. b A comparison of both MSSIM measuring structural accuracy and Mapping accuracy measuring anchor accuracy of the 2 registered sections, across PASTE, PASTE2, GPSA, STalign and ST-GEARS. c Stacked projections of region-specific annotation types including DG, Neurogenesis, subiculum, CA1, CA2 and CA3, registered by ST-GEARS. Each column highlights the stacked projection of a single annotation type. Source data are provided as a Source Data file.
To understand reasons behind our enhancement, we thoroughly examined the anchors generated by PASTE, PASTE2 and ST-GEARS, as well as the effects of our elastic registration. By mapping cluster information of the 2nd section to the 1st, and the 1st to the 2nd through anchors, we found correspondences between the projected and original annotations (Supplementary Fig. 12 ). Accordingly, our Mapping accuracy was over 0.25 higher than PASTE and over 0.45 than PASTE2 (Fig. 4a ), indicating our exceptional anchor accuracy. To understand and further substantiate this advantage, we visualized the probabilistic constraints and its resulted anchors probabilities (Supplementary Fig. 13a ). It is worth noting that ST-GEARS implemented Distributive Constraints, in contrast to the uniform distributions used by PASTE. As a result, a certain percentage of spots were found to be suppressed in anchors connection by ST-GEARS (Supplementary Fig. 13b ) compared to PASTE, leaving the registration to rely more on spots with higher cross-sectional similarity and less computational disturbances, and hence lead to a higher anchor accuracy. We excluded Distributive Constraints from ST-GEARS, and noticed an obvious decrease of mapping accuracy on the hippocampus dataset (Supplementary Fig. 14 ), indicating the contribution of Distributive Constraints on anchors accuracy. In the study of elastic effect, we found an increased overlapping of centerlines by elastic registration than by rigid operation only when overlapping CA fields and DG (Fig. 4b ). Quantitively by MSSIM, the cross-sectional similarity was found to be increased by elastic registration (Supplementary Fig. 15 ). These findings suggest that the combination of Distributive Constraints and elastic process contributed to the enhanced registration of the Mouse hippocampus.
To explore the potential effect of impact of our registration on downstream analysis, we extracted region-specific annotation types from the sections, and analyzed their overlapping through stacking registered sections together (Fig. 4c ). In all annotation types including DG, Neurogenesis, subiculum, CA1, CA2 and CA3, the distribution regions from both sections were nearly identical. The overlapping result unveils that ST-GEARS integrated the spatial profile of same cell subpopulations, enabling a convenient and accurate downstream analysis of multiple sections.
Application to 3D reconstruction of Drosophila embryo
Besides tissue level registration of Mouse hippocampus, to evaluate the performance of ST-GEARS in reconstructing individual with multiple sections, we further tested it on a Drosophila embryo. The transcriptomics of embryo was measured by Stereo-seq, with 7 μm cross-sectioning distance 22 . By quantifying the registration effect of spatial information recovery and comparing it to PASTE, PASTE2, GPSA and STalign, we found that ST-GEARS achieved the highest MSSIM in five out of the six structurally consistent pairs (Fig. 5a ). On the pair where ST-GEARS did not result in highest MSSIM, it surpassed PASTE, and achieved a similar score to PASTE2. By comparing area changes with SI-STD-DI quantification of the complete section, and three individual tissues including epidermis, midgut and foregut, ST-GEARS yielded higher smoothness on all regions than all other approaches, both visually and quantitatively (Fig. 5b ).
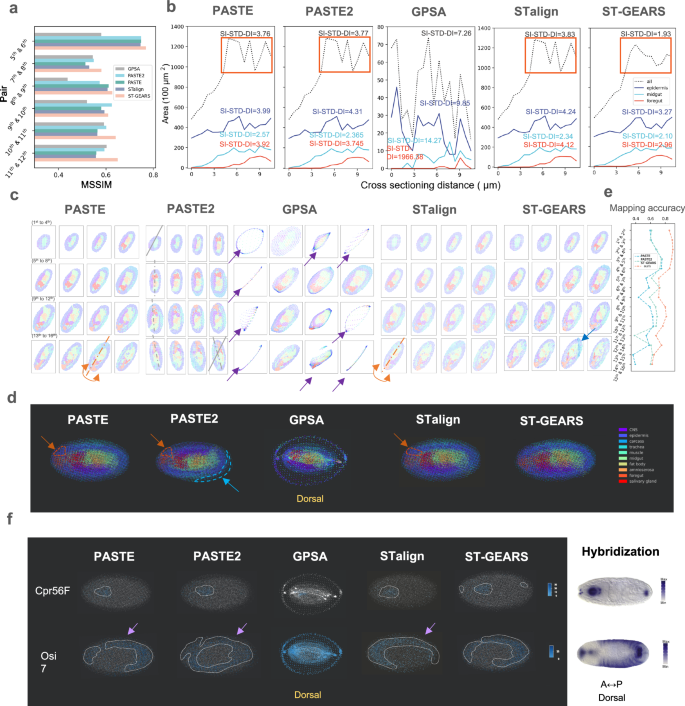
a A comparison of Mean Structural Similarity (MSSIM) measuring structural similarity, of section pairs that are structurally consistent from Drosophila Embryo (E14-16h), between reconstruction results of PASTE, PASTE2, GPSA, STalign and ST-GEARS. b A comparison of area changes of 3 tissues and complete body of Drosophila Embryo, along cross-sectioning direction, between reconstruction result of PASTE, PASTE2, GPSA, STalign and ST-GEARS. Standard Deviation of Differences (SI-STD-DI) which measures structural consistency is marked alongside each curve to quantify the smoothness. The smoothness difference of ST-GEARS compared to PASTE, PASTE2 and STalign are highlighted by orange rectangles. c Reconstructed individual sections with recovered spatial location of each spot. In result of PASTE, the incorrect flipping on the 15th section was highlighted in orange. In result of PASTE2, gradual rotations were marked by the 1st, 5th, 9th, 13th and 16th sections’ approximate symmetry axis whereas symmetry axis of the 1st section was replicated onto the 16th for angle comparison. In result of GPSA, mistakenly distorted sections were marked by purple arrows. In result of STalign, the incorrect flipping on the 13th section was highlighted in orange. In result of ST-GEARS, the fix of dissecting area on the 15th section was marked by a blue arrow. d Dorsal view of 3D reconstructed Drosophila embryo by PASTE, PASTE2, GPSA, STalign and ST-GEARS. The inaccurate regionalization of midgut was circled and pointed with arrow in orange. The resulted extruding part of single section by PASTE2 was circled and pointed in blue. e Mapping accuracy of all section pairs by PASTE, PASTE2 and ST-GEARS. f By dorsal view, regionalization of marker gene Cpr56F and Osi7 by PASTE, PASTE2, GPSA, STalign and ST-GEARS, and their comparison with hybridization result from Berkeley Drosophila Genome Project (BDGP) database. The gathering expression regions were highlighted by dotted lines. Source data are provided as a Source Data file.
To compare the reconstruction effect, we studied both registered individual section, and reconstructed 3D volume. Among the methods compared, PASTE produced a wrong flipping on the 15 th section along A-P axis (Fig. 5c ). Stacking sections back to 3D and investigating on dorsal view, the wrong flipping caused a false regionalization of foregut circled in orange (Fig. 5d ). Along the first to last section registered by PASTE2, a gradual rotation was witnessed (Fig. 5c ), leading to over 20 degrees of angular misalignment between the first and the last section. Similar to PASTE, this misalignment also caused the wrong regionalization of foregut in 3D map (Fig. 4d ). Equally induced by the rotation, sections were found to extrude in the 3D result circled in blue, breaking the round overall morphology of the embryo. GPSA caused false distortion of 8 out of 16 sections as pointed by purple arrows (Fig. 5c ) and the stacked sections formed a dorsal view of an isolated circle and an inner region (Fig. 5d ). The phenomena may be due to its overfitting onto expressions, which is caused by the contradiction between its hypothesis of consistent readout across sections, and the large readout variation across 16 sections in this application. Similar to PASTE, STalign also produced a wrong flipping, on the 13 th section along A-P axis (Fig. 5c ). Stacking the projections back to 3D, a mistaken regionalization of foregut, caused by the wrong flipping, was circled in orange (Fig. 5d ). In contrast, ST-GEARS avoided all of these mistakes in its results (Fig. 5c ). From the perspective of individual section profiles, noticeably in the 15 th section, we observed a significant reduction in the dissecting region between two parallel lines, indicating the successful fixation of flaws in the session. By comparing time usage across all methods, ST-GEARS achieved the 2nd lowest time consumption in registration (Supplementary Fig. 11 ). In terms of memory consumption, ST-GEARS, PASTE and STalign used much less memory than PASTE2 and GPSA. The three most memory efficient methods used almost identity peak memory, with the value fluctuation of <7%.
To comprehend the rationale behind our improvement, we analyzed the anchors generated by the three methods and the impact of our elastic registration. In the investigation of anchor accuracy, we discovered that ST-GEARS achieves the highest mapping accuracy among all section pairs (Fig. 5e ), suggesting its advanced ability to generate precise anchors, which forms the basis for precise spatial profile recovery. To understand this advancement, probabilistic constraints and its resulted anchors distributions (Supplementary Fig. 16 , Supplementary Fig. 17 ) were studied. With Distributive Constraints (Supplementary Fig. 16a ), ST-GEARS generated different maximum probabilities on different annotation types (Supplementary Fig. 16b ), which indicates that annotation types with higher cross-sectional consistency were prioritized in anchor generation. This selection led to reduced computational disturbances, and hence higher accuracy of anchors. We also compared anchor accuracy with and without Distributive Constraints adopted, and noticed an increase of mapping accuracy on each pair of sections (Supplementary Fig. 18 ). In final registration result, ST-GEARS without Distributive Constraints failed to fix the experimental flaw on the 15 th section (Supplementary Fig. 19 ), in contrast to effect upon the setting adopted (Fig. 5c ). Above findings validate the contributive effect of Distributive Constraints in our method. In study of elastic registration in shape smoothness, we witnessed an increased level of smoothness of tissue epidermis, foregut, and midgut, as well as the complete section, through area changes quantified by SI-STD-DI index (Supplementary Fig. 20 ). In internal structure aspect, an increased MSSIM of structural consistent pairs were noticed (Supplementary Fig. 21 ). An experimental flaw on the 15 th section was also fixed by elastic registration (Supplementary Fig. 22 ). Above findings point that the enhancement of registration accuracy on Drosophila embryo was induced by Distributive Constraints and elastic process.
By mapping spots back to 3D space, we further investigated the effect of different method on downstream analysis, in the perspective of genes expression (Fig. 5f ). Cpr56F and Osi7 were selected as marker genes, which were found to respectively highly express in foregut, and foregut plus epidermis region 22 . Investigating Cpr56F expression by ST-GEARS from dorsal view, we noticed three highly expressing regions, at anterior end, front region, and posterior end of the embryo. The finding matches the hybridization result of stage 13-16 Drosophila embryo extracted from Berkeley Drosophila Genome Project (BDGP) database. In contrast, none of PASTE, PASTE2, GPSA and STalign presented high expression at all three locations. When analyzing the distribution of Osi7 by PASTE, PASTE2 and STalign, we noticed a sharp decrease in expression from inner region to the outer layer marked by purple arrows, contradicting the prior knowledge of high expression in the epidermis. This is probably because PASTE and PASTE2 do not consider distortion correction as part of their methods, leaving section edges un-coincided and marker genes not obviously highly expressed on the outermost region. Though involving distortion correction, STalign lost certain amount of structural information by transforming ST data to image utilizing only information of regional gene expression abundance. The registration did not adequately correct distortion without support of enough structural messages. Similarly, PASTE2 failed to capture expression in outer layers and instead revealed a high expression in one inter-connected area, which did not correspond to the separate expression regions observed in hybridization result. No spatial pattern was witnessed when analyzing distribution of Osi7 by GPSA, which forms an obvious contrast to its hybridization evidence. Comparably, none of the violations was shown in the result of ST-GEARS. The comparison of spatial distribution indicated our potential capability to better enhance the process of downstream gene-related analysis.
Application to Mouse brain reconstruction
The design of 3D experiments involves various levels of sectioning distances 22 , 36 , 37 . To further investigate the applicability of ST-GEARS on ST data with larger slice intervals, we applied the method to a complete Mouse brain hemisphere dataset, which consists of 40 coronal sections (Supplementary Fig. 23a ), with a sectioning distance of 200 μm 37 . The transcriptomics data was measured by BARseq, which includes sequencing data and its cross-modal histology images. Each observation represents captured transcriptomics surrounded by the boundary of a cell.
Through respectively applying PASTE, PASTE2, GPSA, STalign and ST-GEARS onto the dataset, we observed multiple misaligned sections produced by approaches including PASTE, PASTE2, GPSA and STalign (Supplementary Fig. 23b , Supplementary Fig. 23c , Supplementary Fig. 23d , Fig. 6a ). In PASTE, these misalignments include 2 sections with ~ 180° angular misalignment (Supplementary Fig. 23b ). By PASTE2, 4 rotational misalignments and 8 positional misalignments were noticed (Supplementary Fig. 23d ). By GPSA, 12 sections were observed to be rotationally misaligned, and 3 sections were mistakenly distorted (Supplementary Fig. 23b ), probably due to its overfitting onto expressions discussed in analysis of Drosophila embryo. The scale on horizontal and vertical axis was distorted maybe due to the similar reason analyzed in Mouse hippocampus. And by STalign, 7 rotational misalignments were generated (Supplementary Fig. 23e ). As a clear contrast, our algorithm correctly aligned all 40 sections with 200 μm intervals (Supplementary Fig. 23f ). To more accurately assess the result of our registration, we employed the direction of the cutting lines induced during tissue processing 37 , and compared the consistency of tilt angles of these lines in the 20th, 25th, 26th, 27th, 33rd, 34th and 37th slices where these lines are visible. Notably, neither visual angle differences nor cutting line curving were observed, indicating that the sections were properly aligned by ST-GEARS (Fig. 6a , Supplementary Fig. 23f ). To quantify the registration accuracy in aspect of structural continuity, we calculated MSSIM scores of 11 section pairs that are structural consistent (Fig. 6b ). Consistent with the visual observations, PASTE2 presented a much larger score range than other methods, which reflects its instability across sections in this dataset, and GPSA exhibited the lowest median MSSIM score indicating its suboptimal average performance. By comparison, PASTE yielded a higher median score and a smaller variation, while ST-GEARS resulted in the highest median score and the smallest variation among all methods. In terms of computational efficiency, ST-GEARS achieved the 2nd lowest time consumption and lowest peak memory consumption across all methods (Supplementary Fig. 11 ).
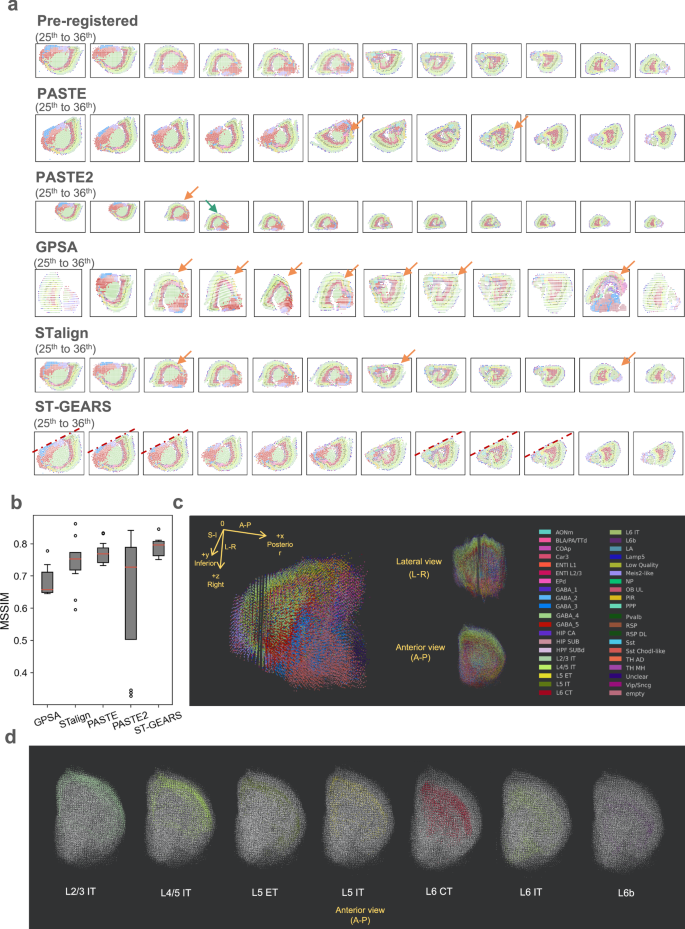
a Reconstructed individual sections with recovered spatial location of each spot from the 25th to 36th section. Positional misalignments are marked by arrows of green, and angular misalignments are marked by arrows of orange. Visible cutting lines by ST-GEARS are marked by dotted lines. b A comparison of Mean Structural Similarity (MSSIM) score of 11 section pairs that are structurally consistent, between result of PASTE, PASTE2, GPSA, STalign and our method. The 11 biological replicates were studied, which were derived from different closest section pairs with each section pair representing smallest unit of study. Non control group was used as a MSSIM close to 1 is assumed to the idealized similarity value of the structurally similar pairs, hence a higher MSSIM value indicates higher reconstruction accuracy. The red lines positions show median score; the box extends from the first quartile (Q1) to the third quartile (Q3) of scores; the lower whisker is at the lowest datum above Q1 − 0.5 * (Q3-Q1), and the upper whisker is at the highest datum below Q3 + 0.5*(Q3-Q1); scores out of whiskers range are marked by circles. c Perspective, Lateral and Anterior view of reconstructed Mouse brain hemisphere. d Anterior view of layer annotation types distribution of reconstructed Mouse brain hemisphere. Source data are provided as a Source Data file.
To understand the reasons behind our progress, we examined anchor accuracy changes with regularization factors during ST-GEARS computation (Supplementary Fig. 24 ). Out of 39 section pairs, we observed a change in mapping accuracy >0.1 (out of 1) in 12 pairs. By Self-adaptive Regularization which was designed to face varying data characteristics which also includes varying section distances, regularization factor that leads to optimal mapping accuracy was selected, leading to an increased anchors accuracy in the 12 section pairs. Notably, among these 12 pairs, pairs 29th & 30th, 31st & 32nd and 32nd & 33rd were correctly aligned by ST-GEARS but misaligned by PASTE, which doesn’t adopt any self-adaptive regularization strategy.
After validating the registration result, we investigated the recovered cell-types’ distribution in the 3D space to assess the effectiveness of the reconstruction and its impact on further analysis. We observed that the complete morphology of hemisphere was recovered by ST-GEARS, with clear distinction of different tissues on perspective, lateral and anterior views (Fig. 6c ). We further studied the distribution of separate annotation types within cortex layers and found that 3D regionalization of each annotation type was recovered by ST-GEARS (Fig. 6d ). The reconstructed result indicated the adaptability of ST-GEARS across various scales of sectioning intervals, and its applicability on both bin-level, and cell-level datasets on which histology information is incorporated.
We introduce ST-GEARS, a 3D geospatial profile recovery approach for ST experiments. Leveraging the formulation of FGW OT, ST-GEARS utilizes both gene expression and structural similarities to retrieve cross-sectional mappings of spots with same in vivo planar coordinates, referred to as ‘anchors’. To further enhance accuracy, it uses our innovated Distributive Constraints to enhance the accuracy. Then it rigidly aligns sections utilizing the anchors, before finally eliminating section distortions using Gaussian-denoised Elastic Fields and its Bi-sectional Application.
We validate counterpart of ST-GEARS including anchors retrieval and elastic registration, respectively on DLPFC and Drosophila larva dataset. In the validation of anchors retrieval, through Mapping accuracy evaluation of retrieved anchors, ST-GEARS consistently outperformed PASTE and PASTE2 across all section pairs. We show Distributive Constraints as reasons behind its distinguished performance, which effectively suppressed the generation of anchors between spot groups with low cross-sectional similarity while enhances their generation among groups with higher similarity. To investigate the effectiveness of the elastic registration process, we evaluate the effects of tissue area changes and cross-sectional similarity using the Drosophila larvae dataset. Both smoother tissue area curves and higher similarity observed between structurally consistent sections confirm the efficacy of the elastic process of ST-GEARS.
We demonstrate ST-GEARS’s advanced accuracy of reconstruction compared to current approaches including PASTE, PASTE2 and GPSA, and its positive impact on downstream analysis compared to existing approaches. Our evaluation encompasses diverse application cases, including registration of two adjacent sections of Mouse hippocampus tissue measured by Slide-seq, reconstruction of 16 sections of Drosophila embryo individual measured by Stereo-seq, and reconstruction of a complete Mouse brain measured by BARseq, including 40 sections with sectioning interval as far as 200 μm. Among the methods, registered result by ST-GEARS exhibited the highest intra-structural consistency measured by MSSIM for two hippocampus sections separated by a single layer of neurons. On 16 sections of a Drosophila embryo individual, our method’s outstanding accuracy is indicated by both MSSIM and smoothness of tissue area changes. Importantly, ST-GEARS provides more reliable embryo morphology, precise tissue regionalization, and accurate marker gene distribution under hybridization evidence compared to existing approaches. This suggests that ST-GEARS provides higher quality tissues, cells, and genes information. On Mouse brain sections with large intervals of 200 μm, ST-GEARS avoided positional and angular misalignments that occur in result of PASTE and PASTE2. The improvement was quantified by a higher MSSIM. Both hemisphere morphology and cortex layer regionalization were reflected in the result of 3D reconstruction by ST-GEARS. The successful representation of important structural and functional features in the aforementioned studies collectively underscores ST-GEARS’ reliability and capability for advancing 3D downstream research, enabling more comprehensive and insightful analysis of complex biological systems.
To further enhance and extend our method, opportunities in various aspects are anticipated to be explored. Firstly, algorithm aspects including hyperparameter sensitivity and scalability can be further explored for a more enhanced method performance. Though recommended values are provided for two of its hyperparameters, method performance is still affected by parameter values, raising the potential issue of overfitting and sensitivity which can be further studied. In scalability aspect, ST-GEARS introduces obvious computational cost increasement when dealing with large-scale datasets. Though strategy of Granularity adjusting is innovated to down-grade complexity, opportunity of improving robustness on increasing scale of data is expected to be further explored. Secondly, tasks aimed at improving data preprocessing, including but not limited to batch effect removal and diffusion correction, are expected to be integrated into our method, considering their coupling property with registration task itself: inaccuracies in input data introduce perturbations to anchors optimization, while recovered spatial information of our method may assist data quality enhancement by providing registered sections. Thirdly, the ST-GEARS’ Distributive Constraint takes rough grouping information as its input, which may potentially introduce computational burden during the reconstruction process. To address this, an automatic step is expected to be developed to reliably cluster spots while maintaining computational efficiency of the overall process. This step can be integrated into our method either as preprocessing, or as a coupling task, similarly to our expectation of data quality enhancement. Finally, we envision incorporating a wider scope of anchors applications into our existing framework. such as information integration of sections across time, across modalities and even across species. With interpretability, robustness and accuracy provided by ST-GEARS, we anticipate its applications and extension in various areas of biological and medical research. We believe that our method can help address a multitude of questions regarding growth and development, disease mechanisms, and evolutionary processes.
FGW OT description
Fused Gromov Wasserstein (FGW) Optimal Transport (OT) is the modeling of spot-wise or cell-wise similarity between two sections, with the purpose of solving optimal mappings between the spots or cells, with mappings also called ‘anchors’. By FGW OT, the optimal group of mappings enables highest gene expression similarity between mapped spots, at the same time keeping similar positions relative to their located sections.
The required input of FGW OT includes genes expression, spot or cell locations before registration, and constraint values which assigns different weight to the optimization on different spots or cells. For gene expression, we introduce \({{\bf{A}}}\in {R}^{{n}_{A},m}\) for section A, to describe normalized count of unique molecular identifiers (UMIs) of different genes of each cell or spot, thereinto n A denotes number of spots in slice A, and m denotes number of genes that are captured in both sections. Similarly, we describe gene expression on section B as \({{\bf{B}}}\in {R}^{{n}_{B},m}\) , with genes arranged in the same order as in A . For spot or cell locations, we introduce \({{{\bf{X}}}}_{{{\bf{A}}}}\in {R}^{{n}_{A},2}\) to describe spots locations of section A, with the 1st column storing horizontal coordinates and the 2nd storing vertical coordinates. Similarly, we have \({{{\bf{X}}}}_{{{\bf{B}}}}\in {R}^{{n}_{B},2}\) to describe spots locations in section B. Spots are arranged in the same order in gene expression and location matrices. Constraint values are discussed in section of Distributive Constraints.
FGW OT solves:
Thereinto, \({{{\bf{M}}}}_{{{\bf{AB}}}}\in {R}^{{n}_{A},{n}_{B}}\) describes the similarity of each pair of spots respectively on section A and B, formulated as \({{{\bf{M}}}}_{{{\bf{i}}},{{\bf{j}}}}^{({{\bf{AB}}})}={KL}({A}_{i,:},{B}_{j,:})\) . Be noted that \({{{\rm{M}}}}_{{{\rm{i}}},{{\rm{j}}}}^{({{\rm{AB}}})}\) still indicates spot-wise similarity M AB , with section code AB being moved to superscript and added parenthesis for clarity, since subscript location are taken by spot index i, j . KL denotes Kullback-Leibler (KL) divergence 43 . \({{{\bf{C}}}}_{{{\bf{A}}}}\in {R}^{{n}_{A},{n}_{A}}\) describes spot-wise distance within section A, with \({{{\bf{C}}}}_{{{\bf{i}}}{{,}}{{\bf{j}}}}^{({{\bf{A}}})}={dis}({{{\bf{X}}}}_{{{\bf{i}}}{{,}}{{:}}}^{({{\bf{A}}})}{{,}}{{{\bf{X}}}}_{{{\bf{j}}},{{:}}}^{({{\bf{A}}})})\) , and dis denoting Euclidean distance measure. Be noted that \({{{\rm{X}}}}_{{{\rm{i}}},:}^{({{\rm{A}}})}\) and \({{{\rm{X}}}}_{{{\rm{j}}},:}^{({{\rm{A}}})}\) still indicate spot locations X A , with section code A being moved to superscript and added parenthesis for clarity, since subscript location are taken by spot index i and j . \({{{\rm{C}}}}_{{{\rm{i}}},{{\rm{j}}}}^{({{\rm{A}}})}\) refers to spot-wise distance C A for the same reason. Similarly, \({{{\bf{C}}}}_{{{\bf{B}}}}\in {R}^{{n}_{B},{n}_{B}}\) describes spot-wise distance of section B. \({{\bf{L}}}\in {R}^{{n}_{A},{n}_{B},{n}_{A},{n}_{B}}\) defines the difference between all spot pair distance respectively on section A and B, with \({{{\rm{L}}}}_{{{\rm{i}}},{{\rm{j}}},{{\rm{k}}},{{\rm{l}}}}=|{{{\rm{C}}}}_{{{\rm{i}}},{{\rm{k}}}}^{({{\rm{A}}})}-{{{\rm{C}}}}_{{{\rm{j}}},{{\rm{l}}}}^{({{\rm{B}}})}|\) . ⊗ denotes Kronecker product of two matrices; 〈,〉 denotes matrix multiplication.
Adjacency matrix \({{\mathbf{\pi }}}\in {R}^{({n}_{A},{n}_{B})}\) to be optimized stores strength of anchors between spots from the two sections, with row index representing spots on section A, and column index representing spots on section B. Sum of elements of π is 1. With \({{\langle }}{{{\bf{M}}}}_{{{\bf{AB}}}}^{{{\bf{2}}}}{{,}}{{\mathbf{\pi }}}{{\rangle }}\) , the similarity of mapped spots are measured. With \({{\langle }}{{{\bf{L}}}}^{{{\bf{2}}}}({{{\bf{C}}}}_{{{\bf{A}}}}{{,}}{{{\bf{C}}}}_{{{\bf{B}}}}){{\times}}{{\mathbf{\pi }}}{{,}}{{\mathbf{\pi }}}{{\rangle }}\) , similarity between distance of spot pairs on section A, with its anchored spot pairs on section B, is measured. \(\langle {{{\bf{L}}}}^{{{\bf{2}}}}({{{\bf{C}}}}_{{{\bf{A}}}}{l{{,}}}{{{\bf{C}}}}_{{{\bf{B}}}})\otimes {{\mathbf{\pi }}}{{,}}{{\mathbf{\pi }}}\rangle\) describes similarity between spatial structures under the anchors’ connection. α ∈ [0,1] denotes regularization factor, which specifies the relative importance of structure similarity compared to expression similarity. W A and W B are constraint values that are introduced in section of Distributive Constraints.
With the formulation above, FGW OT solves optimal anchors between the spots, or cells, which enables maximum weighted combination of gene expression similarity and position similarity of mapped spots or cells.
Distributive constraints
As adopted by constraint values in FGW OT, we introduce Distributive Constraints, to assign different emphasis to spots or cells in the optimization. Distributive Constraints utilizes cell type component information to differentiate the emphasis: if an annotation or cluster express high similarity across sections, its corresponding spots or cells will be placed relatively high sum of probability, and vice versa. With higher sum of probability, more anchors and anchors with higher strength are generated, while less anchors are produced on spots with lower sum of probability. This operation leads registration to rely more on expression-consistent regions of sections, hence largely enhancing both accuracy of anchors and precision of following rigid and elastic registration.
The required inputs of Distributive Constraints include \({{{\bf{G}}}}_{{{\bf{A}}}}\in {R}^{{n}_{A}}\) and \({{{\bf{G}}}}_{{{\bf{B}}}}\in {R}^{{n}_{B}}\) , which store the grouping information such as annotation type or cluster of each spot in section A and B. We then summarize the repeated annotations or clusters from G A and G B , and put the unique values in \({{\bf{g}}}\in {R}^{{n}_{{group}}}\) . n group is the number of unique annotation type or clusters. Then implemented in ST-GEARS, for each annotation type or cluster g i , we calculate the average gene expression across spots:
Be noted that \({{{\bf{G}}}}_{{{{\bf{i}}}}^{{\prime} }}^{({{\bf{A}}})}\) and \({{{\bf{G}}}}_{{{{\bf{i}}}}^{{\prime} }}^{({{\bf{B}}})}\) still indicate grouping information G A and G B , with section code A and B being moved to superscript and added parenthesis for clarity, since subscript location are taken by spot index i ′ and j ′. And \({{\bf{1}}}_{{{{\bf{n}}}}_{{{\bf{A}}}}}\) and \({{\bf{1}}}_{{{{\bf{n}}}}_{{{\bf{B}}}}}\) are both row vectors of ones.
With average gene expression of each annotation type or cluster, with the form of distribution, we measure its difference across sections by KL divergence. Then the calculated distance is mapped by logistic kernel, to further emphasize differences between relatively consistent annotations or clusters.
\({di}{s}_{{map}}={f}_{{logistic}}({dis})\) , where \({f}_{{logistic}}\left(x\right)=\frac{1}{1+{e}^{-x}}-0.5\) . Putting scaler value dis of each annotation or cluster together, we have a vector \({{\bf{DI}}}{{{\bf{S}}}}_{{{\bf{map}}}}\in {R}^{{n}_{{celltype}}}\) . Finally, we transform the distance to similarity, map the similarity result back to each spot:
We further apply normalization on the result:
W A and W B are constraints values applied in (1). Since the values are computed based on similarity measure using cell composition information, weight of FGW OT is automatically redistributed, with higher emphasis on more consistent regions across sections, and less emphasis on less consistent area. Enhanced anchor accuracy hence registration accuracy is then achieved.
Self-adaptive regularization
In FGW OT formulation, a regularization factor is included to specify the relative importance of structural similarity compared to expression similarity during optimization. ST-GEARS includes a self-adaptive regularization method that determines the factor value, that induces highest overall accuracy of anchors despite of varying situations. Situations include but are not limited to section distances, spot sizes, extent of distortions, and data quality such as level of diffusion.
By practice, our method respectively adopts factors on multiple scales including 0.8, 0.4, 0.2, 0.1, 0.05, 0.025, 0.013, and 0.006. The candidate values vary exponentially, for ST-GEARS to find the optimal term regardless of scale differences between expression and structural term in (1). The accuracy of each set of optimized anchors by every regularization factor was evaluated, by measuring weighted percentage \({\sum}_{{{{\bf{G}}}}_{{{\bf{i}}}}^{{{(}}{{\bf{A}}}{{)}}}{{=}}{{{\bf{G}}}}_{{{\bf{j}}}}^{{{(}}{{\bf{B}}}{{)}}}}{{{\boldsymbol{\pi }}}}_{{{\bf{i}}}{{,}}{{\bf{j}}}}\) of anchors that join spots with same annotation types or clusters. Be noted that \({{{\rm{G}}}}_{{{\rm{i}}}}^{({{\rm{A}}})}\) and \({{{\rm{G}}}}_{{{\rm{j}}}}^{({{\rm{B}}})}\) still indicate grouping information G A and G B , respectively, with section code A and B being moved to superscript and added parenthesis for clarity, since subscript location are taken by spot index i and j . The regularization factor value that achieves highest accuracy is then adopted by our method.
Elastic field inference
Finding spots with highest probability.
After rigid registration, elastic fields are inferred based on the anchors with the highest probability for each spot or cell. For elastic field to be applied on each section, it is calculated using its anchors with closest sections, as well as spatial coordinates of sections after rigid registration. Along cross-sectioning order, each section in the middle has two closest sections, respectively on its anterior and posterior sides. Exceptionally, if a section is on anterior or posterior end, it has only one closest section.
Specifically for a section in the middle with N spots, we calculate \({{{\bf{I}}}}_{{{\bf{pre}}}}\epsilon {Z}^{N}\) and \({{{\bf{I}}}}_{{{\bf{next}}}}\epsilon {Z}^{N}\) which stores the mapped spots on anterior and posterior neighbor section for each of its spots. The calculation takes as input adjacency matrix π pre , which stores anchors with the anterior neighbor section output by FGW OT, and π next storing anchors with posterior section.
Be noted that \({{{\rm{\pi }}}}_{:,{{\rm{n}}}}^{\left({{\rm{pre}}}\right)}\) and \(\,{{{\rm{\pi }}}}_{{{\rm{n}}},:}^{({{\rm{next}}})}\) still indicate adjacency matrix π pre and π next , with direction code pre and next being moved to superscript and added parenthesis for clarity, since subscript location are taken by spot index n .
Notably, not every spot in a selected section has its own anchored spot, due to multiple strategies including distributive constraint and anchors filtration, hence their corresponding element in I pre and I next are null. For section located on posterior end, only I next is applicable; and for section located on anterior end, only \({{{\bf{I}}}}_{{{\rm{pre}}}}^{{{\rm{n}}}}\) is applicable.
Elastic field establishment
After specifying spots with highest probability, ST-GEARS calculates location displacements between the spots, then establishes elastic fields for each section. An elastic field is a 2D displacement distribution, describing how displacement values are distributed across different locations. And it is established to enable ST-GEARS to benefit from further denoising functions to reduce elastic operation outliers and improve elastic effect consistency across regions.
For each section located in the middle, 4 elastic fields are generated. Two of those represent the section’s horizontal and vertical displacement distribution compared to anterior neighbor section, denoted as 2D matrix F (x_pre) and F (y_pre) , while the other two represent its horizontal and vertical displacement distribution compared to posterior neighbor, denoted as F (x_next) and F (y_next) . To initialize F (x_pre) , F (y_pre) , F (x_next) and F (y_next) for the section, the shape of the matrix is first decided. Its height denoted by Height and width denoted by Width are calculated by gridding the spot locations using a fixed step. Height and Width are shared across the 4 matrices:
For its input, \({{\bf{X}}}\in {R}^{N,2}\) denotes spots location of current section after rigid registration. For a single section, we prepare \({{{\bf{X}}}}^{{{(}}{{\bf{pre}}}{{)}}}\epsilon {R}^{{N\_pre},2}\) and \({{{\bf{X}}}}^{{{(}}{{\bf{next}}}{{)}}}\epsilon {R}^{{N\_next},2}\) as spots location of its anterior and posterior section after rigid alignment, respectively. psize represents average distance between closest spot or cell centers, and it is to be input by users. The matrix has no filled values to this step.
To fill in the fields, we first transform spot locations into the coordinate system of field. With \({{\bf{X}}}\_{{\bf{shifted}}}\,\epsilon {R}^{N,2}\) and \({{\bf{X}}}\_{{\bf{pixel}}}\,\epsilon {R}^{N,2}\) :
We then calculate location displacements between each of its spots and their anchored spots with highest probability, on both anterior and posterior neighbors. With \({{\bf{X}}}\_{{\bf{corres}}}\,\epsilon {R}^{N,2}\) and \({{\bf{X}}}\_{{\bf{delta}}}\epsilon {R}^{N,2}\) :
With the spot locations in field coordinates and the displacement values above, we fill in corresponding elements of the elastic field:
By the end of Eqs. ( 2 ), 4 elastic fields for each section in the middle is established. However, some elements in the matrix are still empty, because of absence of spots or cells located in the grid of location. To address this problem, 2d nearest interpolation method 44 was adopted, which fills in every empty element, with the displacement value of its neighboring elements:
thereinto \({{\bf{mes}}}{{{\bf{h}}}}_{{{\bf{trans}}}}\epsilon {N}^{{n}_{{grids}}\times 2}\) denotes grid coordinates of the designed field, with \({n}_{{grids}}={Height}\times {Width}\) . And f interp_grid denotes the nearest interpolation method.
For section located on posterior end, only F (x_next) and F (y_next) are applicable; and for section located on anterior end, only F (x_pre) and F (y_pre) are applicable.
2D Gaussian denoising
As caused by exerted force, the displacement or elastic field is expected to have static or smoothly changing values across different locations 45 , 46 , 47 . ST-GEARS makes use of this property, to smoothen the field and to reduce errors in the field caused by any upper stream process, such as raw data noises and inaccuracy in anchor computation. Gaussian filtering 48 , 49 is adopted to implement the denoising, similarly to image denoising processes 50 , 51 . Denoised elastic fields are then generated.
It calculates weighted average across the neighboring region of each element to replace its value:
where f gaussian_filter denotes the method of Gaussian filtering.
Bi-sectional fields application
Bi-sectional fields application plan.
With elastic fields generated and denoised, ST-GEARS uses the fields as a guidance to correct distortion for each section. Through querying the elastic fields with spatial location of each spot, the displacement to be implemented is returned. For a section in the middle, its elastic fields calculated with both anterior and posterior neighbor sections are queried, and guidance provided by both anterior and posterior sections are applied on the rigid aligned result, called ‘Bi-sectional Fields Application’. After the application, the distortion of the section is corrected, and the elastic registration result is generated.
Specifically, the denoised elastic fields are first queried, returning the displacement to be implemented:
Next, average displacement returned by both anterior and posterior sections are applied on the rigid registration result, leading to final elastic registration result \({{\bf{X}}}\_{{\bf{final}}}\in {R}^{N,2}\!\!:\)
For section located on posterior end,
For section located on anterior end,
The validity of this plan is proved in the section: Proof of validity of Bi-sectional Fields Application.
Proof of validity of Bi-sectional fields application
Bi-sectional Fields Application accurately recovers the spatial profile before distortion, by averaging and applying displacement value guided by both anterior and posterior neighbor section. The effect is approved mathematically as following:
Take section A, B, and C as an example of a sequence of sections, with X A , X B and X C denoting their spots’ spatial information after rigid alignment, and X A_insitu , X B_insitu and X C_insitu denoting their in vivo spatial information. The distortion occurred to the slices during experiments are denoted as X A_dis , X B_dis and X C_dis .
According to Bi-sectional Fields Application, the corrected spatial information is:
Based on the in vivo morphological consistency across sections, spatial information of section B can be approximated by an average of information of A and C, written as
Given that X A_dis and X C_dis can be seen as independent and identically distributed sets of variables,
where μ ABC is the universal mean, and Σ ABC is the variance of the 2d displacement information.
Inserting the terms (4) and (5) back to Eq. ( 3 ) gives
indicating the proximity of corrected spatial information to in vivo spatial information.
Evaluation metrix
We evaluated the accuracy of anchors by index of Mapping Accuracy, and measured the reconstruction effect by MSSIM and SI-STD-DI, in both elastic effect study and overall methodology comparison.
Mapping accuracy
Designed and adopted by PASTE 27 , Mapping Accuracy calculates the weighted percentage of anchors joining spots with same annotation.
MSSIM index
MSSIM measures the accuracy of registration, based on the assumption that in some sectioning positions, tissue morphology remains almost consistent across slices. The method quantifies the accuracy, by measuring the similarity of annotation type distribution of such section pairs.
To implement the quantification, first, structurally consistent section pairs are selected among all sections arranged in sequence.
Next, on each section from the pair, transformation from individual spots to a complete image is implemented, by gridding the rectangular area that surrounds the tissue, and assigning each grid of a value that represents the annotation type which occurs most frequently in the grid. The resulted image describes the annotation type distribution of the section.
Finally, similarity between each pair of images is measured, by index of MSSIM 52 . The method generates a window with fixed size, slides the window simultaneously on both images, and compares the two framed parts by windows on their intensity, contrast, and structures. Among those, the intensity difference is measured by difference of average pixel values, the contrast difference is measured by comparing variance of the two sets of framed pixel values, and the structure difference is measured by comparing their covariances. A Structural Similarity of Images (SSIM) index is calculated for each position of the window using \({SSIM}(X,Y)=\frac{(2{\mu }_{x}{\mu }_{y})(2{\sigma }_{{xy}}+{c}_{2})}{({\mu }_{x}^{2}+{\mu }_{y}^{2}+{c}_{1})({\sigma }_{x}^{2}+{\sigma }_{y}^{2}+{c}_{2})}\) , where μ x and μ y denote average pixel values of the frames, σ x and σ y denote variances of the frames, and σ xy denotes covariances of the two frames. c 1 and c 2 are constants to avoid 0 value of the divisor. Averaging the SSIM value across all windows gives the final MSSIM result of the two sections.
SI-STD-DI measures smoothness of area changing across sections along a fixed axis, by calculating the standard deviation of area changes on each pair of adjacent sections and scale the result by dividing it by average area.
Software and code
Data analysis.
All software used to analyze data in this study are open-sourced Python packages, including anndata = 0.9.2, numpy = 1.22.4, pandas = 1.4.3, scipy = 1.10.1, matplotlib = 3.5.2, k3d = 2.15.3.
Statistics and reproducibility
No statistical method was used to predetermine sample size. No data were excluded from the analyses. The experiments were not randomized. The Investigators were not blinded to allocation during experiments and outcome assessment.
Reporting summary
Further information on research design is available in the Nature Portfolio Reporting Summary linked to this article.
Data availability
All data used in this research were collected from published sources. DLPFC data was obtained from the research: Transcriptome-scale Spatial Gene Expression in the Human Dorsolateral Prefrontal Cortex, with data downloading link of http://research.libd.org/spatialLIBD/index.html ; Drosophila embryo and Drosophila larva data were collected from High-resolution 3d Spatiotemporal Transcriptomic Maps of Developing Drosophila Embryos and Larvae, with the dataset link of https://db.cngb.org/stomics/datasets/STDS0000060 . Mouse brain data was collected from research: Modular cell type organization of cortical areas revealed by in vivo sequencing. The download link is: https://data.mendeley.com/datasets/8bhhk7c5n9/1 . All datasets were generated on Spatial Transcriptomics platform, with DLPFC data generated by Visium technology of 10x Genomics, Mouse brain data generated by BARseq of Cold Spring Harbor Laboratory, while Drosophila embryo and larva generated by Stereo-seq technology of BGI. Source data are provided with this paper.
Code availability
The methods of ST-GEARS is packaged, and distributed as an open-source, publicly available repository at https://github.com/STOmics/ST-GEARS 53 .
Marx, V. Method of the year: spatially resolved transcriptomics. Nat. Methods 18 , 9–14 (2021).
Article CAS PubMed Google Scholar
Yue, L. et al. A guidebook of spatial transcriptomic technologies, data resources and analysis approaches. Comput. Struct. Biotechnol . J. 21 , 940–955 (2023)
Park, H.-E. et al. Spatial transcriptomics: technical aspects of recent developments and their applications in neuroscience and cancer research. Adv. Sci. 10 , 2206939 (2023).
Article CAS Google Scholar
Gyllborg, D. et al. Hybridization-based in vivo sequencing (hybiss) for spatially resolved transcriptomics in human and mouse brain tissue. Nucleic acids Res. 48 , 112–112 (2020).
Article Google Scholar
Chen, X. et al. High-throughput mapping of long-range neuronal projection using in vivo sequencing. Cell 179 , 772–786 (2019).
Article CAS PubMed PubMed Central Google Scholar
Wang, X. et al. Three-dimensional intact-tissue sequencing of single-cell transcriptional states. Science 361 , 5691 (2018).
Qin, D. Next-generation sequencing and its clinical application. Cancer Biol. Med. 16 , 4 (2019).
Article PubMed PubMed Central Google Scholar
Chen, A. et al. Large field of view-spatially resolved transcriptomics at nanoscale resolution. BioRxiv https://doi.org/10.1101/2021.01.17.427004 (2021).
Stickels, R. R. et al. Highly sensitive spatial transcriptomics at near-cellular resolution with slide-seqv2. Nat. Biotechnol. 39 , 313–319 (2021).
Moses, L. & Pachter, L. Museum of spatial transcriptomics. Nat. Methods 19 , 534–546 (2022).
Moor, A. E. & Itzkovitz, S. Spatial transcriptomics: paving the way for tissue-level systems biology. Curr. Opin. Biotechnol. 46 , 126–133 (2017).
Zhou, R., Yang, G., Zhang, Y. & Wang, Y. Spatial transcriptomics in development and disease. Mol. Biomed. 4 , 32 (2023).
Li, Z. & Peng, G. Spatial transcriptomics: New dimension of understanding biological complexity. Biophys. Rep. 8 , 119 (2022).
Williams, C. G., Lee, H. J., Asatsuma, T., Vento-Tormo, R. & Haque, A. An introduction to spatial transcriptomics for biomedical research. Genome Med. 14 , 1–18 (2022).
Walker, B. L., Cang, Z., Ren, H., Bourgain-Chang, E. & Nie, Q. Deciphering tissue structure and function using spatial transcriptomics. Commun. Biol. 5 , 220 (2022).
Atta, L. & Fan, J. Computational challenges and opportunities in spatially resolved transcriptomic data analysis. Nat. Commun. 12 , 5283 (2021).
Article ADS CAS PubMed PubMed Central Google Scholar
Velten, B. et al. Identifying temporal and spatial patterns of variation from multimodal data using Mefisto. Nat. Methods 19 , 179–186 (2022).
Townes, F. W. & Engelhardt, B. E. Nonnegative spatial factorization applied to spatial genomics. Nat. Methods 20 , 229–238 (2023).
Verma, A. & Engelhardt, B. A Bayesian nonparametric semi-supervised model for integration of multiple single-cell experiments. bioRxiv https://doi.org/10.1101/2020.01.14.906313 (2020).
Svensson, V., Teichmann, S. A. & Stegle, O. Spatialde: identification of spatially variable genes. Nat. Methods 15 , 343–346 (2018).
Dries, R. et al. Giotto: a toolbox for integrative analysis and visualization of spatial expression data. Genome Biol. 22 , 1–31 (2021).
Wang, M. et al. High-resolution 3d spatiotemporal transcriptomic maps of developing drosophila embryos and larvae. Dev. Cell 57 , 1271–1283 (2022).
Mohenska, M. et al. 3d-cardiomics: a spatial transcriptional atlas of the mammalian heart. J. Mol. Cell. Cardiol. 163 , 20–32 (2022).
Vickovic, S. et al. Three-dimensional spatial transcriptomics uncovers cell type localizations in the human rheumatoid arthritis synovium. Commun. Biol. 5 , 129 (2022).
Rao, A., Barkley, D., França, G. S. & Yanai, I. Exploring tissue architecture using spatial transcriptomics. Nature 596 , 211–220 (2021).
Bergenstråhle, J., Larsson, L. & Lundeberg, J. Seamless integration of image and molecular analysis for spatial transcriptomics workflows. BMC Genom. 21 , 1–7 (2020).
Zeira, R., Land, M., Strzalkowski, A. & Raphael, B. J. Alignment and integration of spatial transcriptomics data. Nat. Methods 19 , 567–575 (2022).
Liu, X., Zeira, R. & Raphael, B. Paste2: Partial alignment of multi-slice spatially resolved transcriptomics data. In Research in Computational Molecular Biology: 27th Annual International Conference , 210 (Springer Nature, 2023)
Jones, A., Townes, F. W., Li, D. & Engelhardt, B. E. Alignment of spatial genomics data using deep gaussian processes. Nat. Methods 20 , 1379–1387 (2023).
Xia, C.-R., Cao, Z.-J., Tu, X.-M. & Gao, G. Spatial-linked alignment tool (slat) for aligning heterogenous slices properly. bioRxiv https://doi.org/10.1101/2023.04.07.535976 (2023).
Qiu, X., et al. Spateo: multidimensional spatiotemporal modeling of single-cell spatial transcriptomics. BioRxiv https://doi.org/10.1101/2022.12.07.519417 (2022).
Guo, L. et al. Vt3d: a visualization toolbox for 3d transcriptomic data. J. Genetics Genom. 50, 713–719 (2023).
Fang, S. et al. Stereopy: modeling comparative and spatiotemporal cellular heterogeneity via multi-sample spatial transcriptomics. bioRxiv https://doi.org/10.1101/2023.12.04.569485 (2023).
Titouan, V., Courty, N., Tavenard, R. & Flamary, R. Optimal transport for structured data with application on graphs. Int. Conf. Mach. Learn. 91 , 6275–6284 (2019).
Maynard, K. R. et al. Transcriptome-scale spatial gene expression in the human dorsolateral prefrontal cortex. Nat. Neurosci. 24 , 425–436 (2021).
Rodriques, S. G. et al. Slide-seq: A scalable technology for measuring genome-wide expression at high spatial resolution. Science 363 , 1463–1467 (2019).
Chen, X., Fischer, S., Zhang, A., Gillis, J. & Zador, A. Modular cell type organization of cortical areas revealed by in vivo sequencing. BioRxiv https://doi.org/10.1101/2022.11.06.515380 (2022).
Abdolhosseini, F. et al. Cell identity codes: understanding cell identity from gene expression profiles using deep neural networks. Sci. Rep. 9 , 2342 (2019).
Article ADS PubMed PubMed Central Google Scholar
Efroni, I., Ip, P.-L., Nawy, T., Mello, A. & Birnbaum, K. D. Quantification of cell identity from single-cell gene expression profiles. Genome Biol. 16 , 1–12 (2015).
Lacoste-Julien, S. Convergence rate of frank-wolfe for non-convex objectives. arXiv https://doi.org/10.48550/arXiv.1607.00345 (2016).
Wahba, G. A least squares estimate of satellite attitude. SIAM Rev. 7 , 409–409 (1965).
Article ADS Google Scholar
Lanjakornsiripan, D. et al. Layer-specific morphological and molecular differences in neocortical astrocytes and their dependence on neuronal layers. Nat. Commun. 9 , 1623 (2018).
Csisz ́ar, I: I-divergence geometry of probability distributions and minimization problems. Ann. Probab . 3 , 146–158 (1975).
Schoenberg, I. J. Contributions to the problem of approximation of equidistant data by analytic functions. In I. J. Schoenberg Selected Papers. Contemporary Mathematicia ns. (ed. de Boor, C.) 3–57 (Birkhäuser, Boston, 1988).
Zhou, H. & Jayender, J. Smooth deformation field-based mismatch removal in real-time. arXiv https://doi.org/10.1101/7.08553 (2020).
Li, X. & Hu, Z. Rejecting mismatches by correspondence function. Int. J. Comput. Vis. 89 , 1–17 (2010).
Li, X., Larson, M. & Hanjalic, A. Pairwise geometric matching for large-scale object retrieval. In: Proc. IEEE Conference on Computer Vision and Pattern Recognition , 5153–5161 (IEEE, 2015)
Bergholm, F. Edge focusing. In IEEE Transactions on Pattern Analysis and Machine Intelligence, 726–741 (IEEE, 1987).
Marr, D. & Hildreth, E. Theory of edge detection. Proc. R. Soc. Lond. Ser. B. Biol. Sci. 207 , 187–217 (1980).
ADS CAS Google Scholar
Mafi, M. et al. A comprehensive survey on impulse and gaussian denoising filters for digital images. Signal Process. 157 , 236–260 (2019).
Saxena, C. & Kourav, D. Noises and image denoising techniques: a brief survey. Int. J. Emerg. Technol. Adv. Eng. 4 , 14878–14885 (2014).
Google Scholar
Wang, Z., Bovik, A. C., Sheikh, H. R. & Simoncelli, E. P. Image quality assessment: from error visibility to structural similarity. IEEE Trans. image Process. 13 , 600–612 (2004).
Article ADS PubMed Google Scholar
Xia, T. et al. ST-GEARS: Advancing 3d downstream research through accurate spatial information recovery. GitHub . https://doi.org/10.5281/zenodo.13131713 (2024).
Download references
Acknowledgements
This work is part of the “SpatioTemporal Omics Consortium” (STOC) paper package. A list of STOC members is available at: http://sto-consortium.org . We acknowledge the Stomics Cloud platform ( https://cloud.stomics.tech/ ) for providing convenient ways for analyzing spatial omics datasets. We acknowledge the CNGB Nucleotide Sequence Archive (CNSA) of China National GeneBank DataBase (CNGBdb) for maintaining the Drosophila database. This work is supported by National Natural Science Foundation of China (32300526 to S. F., 32100514 to M. X.). We thank Weizhen Xue for the inspirational discussion towards design of Distributive Constraints. We thank Yating Ren for her advice towards a more efficient code implementation. We thank Dr. Xiaojie Qiu and Dr. Yinqi Bai for the discussion on the registration topic and their advice on our work.
Author information
Authors and affiliations.
BGI Research, Beijing, 102601, China
Tianyi Xia, Luni Hu, Lei Cao, Yunjia Zhang, Lei Zhang, Taotao Pan, Bohan Zhang, Bowen Ma, Chuan Chen, Chao Liu & Shuangsang Fang
BGI Research, Shenzhen, 518083, China
Tianyi Xia, Luni Hu, Lei Cao, Yunjia Zhang, Mengyang Xu, Qin Lu, Lei Zhang, Taotao Pan, Bohan Zhang, Bowen Ma, Chuan Chen, Mei Li, Chao Liu, Yuxiang Li, Yong Zhang & Shuangsang Fang
BGI, Shenzhen, 518083, China
Lulu Zuo, Junfu Guo & Chang Shi
BGI Research, Qingdao, 266555, China
Mengyang Xu
BGI Research, Wuhan, 430074, China
Yuxiang Li & Yong Zhang
Guangdong Bigdata Engineering Technology Research Center for Life Sciences, BGI research, Shenzhen, 518083, China
You can also search for this author in PubMed Google Scholar
Contributions
Tianyi Xia was responsible of method design, analysis design and implementation, as well as drafting of this manuscript. Dr. Luni Hu participated in structure design of the applications. Lulu Zuo was in part of 3D visualizations design, and she helps maintain our online repository. Tianyi Xia, Lei Cao, Lulu Zuo and Dr. Luni Hu conducted experiments and analysis for reply to peer review. Dr. Yunjia Zhang provided insights in anchors results interpretation of DLPFC dataset, and in accuracy analysis of mouse brain dataset. Dr. Mengyang Xu revised this article. Lei Zhang and Bowen Ma offered numerous suggestions to enhance computational efficiency, in both memory and time. Taotao Pan and Chuan Chen provided suggestions in data preprocessing. Qin Lu, Bohan Zhang, Junfu Guo, Chang Shi and Mei Li provided suggestions for this study. Dr. Shuangsang Fang supervised this study in structure and analysis design, and she revised this article. Chao Liu, Yuxiang Li and Yong Zhang supervised this study.
Corresponding authors
Correspondence to Chao Liu , Yuxiang Li , Yong Zhang or Shuangsang Fang .
Ethics declarations
Competing interests.
The authors declare no competing interests.
Peer review
Peer review information.
Nature Communications thanks Jun Ding, Xiangyu Luo and the other, anonymous, reviewer(s) for their contribution to the peer review of this work. A peer review file is available.
Additional information
Publisher’s note Springer Nature remains neutral with regard to jurisdictional claims in published maps and institutional affiliations.
Supplementary information
Supplementary information, peer review file, reporting summary, source data, source data, rights and permissions.
Open Access This article is licensed under a Creative Commons Attribution-NonCommercial-NoDerivatives 4.0 International License, which permits any non-commercial use, sharing, distribution and reproduction in any medium or format, as long as you give appropriate credit to the original author(s) and the source, provide a link to the Creative Commons licence, and indicate if you modified the licensed material. You do not have permission under this licence to share adapted material derived from this article or parts of it. The images or other third party material in this article are included in the article’s Creative Commons licence, unless indicated otherwise in a credit line to the material. If material is not included in the article’s Creative Commons licence and your intended use is not permitted by statutory regulation or exceeds the permitted use, you will need to obtain permission directly from the copyright holder. To view a copy of this licence, visit http://creativecommons.org/licenses/by-nc-nd/4.0/ .
Reprints and permissions
About this article
Cite this article.
Xia, T., Hu, L., Zuo, L. et al. ST-GEARS: Advancing 3D downstream research through accurate spatial information recovery. Nat Commun 15 , 7806 (2024). https://doi.org/10.1038/s41467-024-51935-0
Download citation
Received : 04 January 2024
Accepted : 20 August 2024
Published : 06 September 2024
DOI : https://doi.org/10.1038/s41467-024-51935-0
Share this article
Anyone you share the following link with will be able to read this content:
Sorry, a shareable link is not currently available for this article.
Provided by the Springer Nature SharedIt content-sharing initiative
By submitting a comment you agree to abide by our Terms and Community Guidelines . If you find something abusive or that does not comply with our terms or guidelines please flag it as inappropriate.
Quick links
- Explore articles by subject
- Guide to authors
- Editorial policies
Sign up for the Nature Briefing: AI and Robotics newsletter — what matters in AI and robotics research, free to your inbox weekly.


Maintenance work is planned from 22:00 BST on Monday 16th September 2024 to 22:00 BST on Tuesday 17th September 2024.
During this time the performance of our website may be affected - searches may run slowly, some pages may be temporarily unavailable, and you may be unable to access content. If this happens, please try refreshing your web browser or try waiting two to three minutes before trying again.
We apologise for any inconvenience this might cause and thank you for your patience.
Lab on a Chip
Development of in vitro model of exosome transport in microfluidic gut-brain axis-on-a-chip.

* Corresponding authors
a Department of Chemical Engineering, Hongik University, Seoul, Republic of Korea E-mail: [email protected]
b School of Electrical and Electronic Engineering, Yonsei University, Seoul, Korea 03722
The gut communicates with the brain in a variety of ways known as the gut–brain axis (GBA), which is known to affect neurophysiological functions as well as neuronal disorders. Exosomes capable of passing through the blood–brain-barrier (BBB) have received attention as a mediator of gut–brain signaling and drug delivery vehicles. In conventional well plate-based experiments, it is difficult to observe the exosome movement in real time. Here, we developed a microfluidic-based GBA chip for co-culturing gut epithelial cells and neuronal cells and simultaneously observing exosome transport. The GBA-chip is aimed to mimic the in vivo situation of convective flow in blood vessels and convective and diffusive transport in the tissue interstitium. Here, fluorescence-labeled exosome was produced by transfection of HEK-293T cells with CD63-GFP plasmid. We observed in real time the secretion of CD63-GFP-exosomes by the transfected HEK-293T cells in the chip, and transport of the exosomes to neuronal cells and analyzed the dynamics of GFP-exosome movement. Our model is expected to enhance understanding of the roles of exosome in GBA.
- This article is part of the themed collection: Lab on a Chip HOT Articles 2024
Article information
Download citation, permissions.

G. M. Seo, H. Lee, Y. J. Kang, D. Kim and J. H. Sung, Lab Chip , 2024, Advance Article , DOI: 10.1039/D4LC00490F
To request permission to reproduce material from this article, please go to the Copyright Clearance Center request page .
If you are an author contributing to an RSC publication, you do not need to request permission provided correct acknowledgement is given.
If you are the author of this article, you do not need to request permission to reproduce figures and diagrams provided correct acknowledgement is given. If you want to reproduce the whole article in a third-party publication (excluding your thesis/dissertation for which permission is not required) please go to the Copyright Clearance Center request page .
Read more about how to correctly acknowledge RSC content .
Social activity
Search articles by author.
This article has not yet been cited.
Advertisements

IMAGES
VIDEO
COMMENTS
Definition. The term in vivo refers to a type of experiment that is carried out within a whole, living organism, such as a plant or animal.. In vivo means "within the living" in Latin, which aptly fits its modern definition.. Overview. In vivo refers to a specific type of experimentation that involves living animals. Living animals are very complex, which provides both benefits and ...
In Vivo vs. In Vitro: Definition, Examples, and More
In Vivo vs. In Vitro: What Are the Differences?
In vivo - Wikipedia ... In vivo
Colloquially, these experiments are commonly called "test tube experiments". In contrast, in vivo work is that which is conducted with living organisms in their normal, intact state, while ex vivo studies are conducted on functional organs that have been removed from the intact organism. Common examples of in vitro experiments include work that ...
In vivo experiments also provide insights into the complex interplay between different organ systems, the influence of the immune system, and the overall physiological response. In vivo experiments also offer the advantage of studying long-term effects and dynamic processes. By observing the progression of a disease or the response to a ...
In Vivo model involves the internal environment of a living being, results of in vivo studies are considered more reliable or more relevant than those of in vitro studies. While the terms in vivo and in vitro sound very similar, their meanings are not. In vivo is Latin for "within the living.". It is a study model used for a process or ...
Ethically, organotypic brain slice cultures offer a potentially alternative system to severe in vivo animal studies. In vivo animal experiments can cause significant pain or stress. Moreover, organotypic brain slice cultures reduce the number of animals required for an in vivo experiment since multiple slices can be obtained from one animal .
As a consequence, the majority of in vivo experiments were equivalent to testing a treatment on 30-year-old identical twin brothers who all live in the same village, followed the same career path ...
This document is intended to provide guidance for the design, development and statistical validation of in vivo assays residing in flow schemes of discovery projects. It provides statistical methodology for pre-study, cross-study (lab-to-lab transfers and protocol changes), and in-study (quality control monitoring) validation. Application of the enclosed methods will increase confidence in ...
What is in vivo. In vivo refers to a phenomenon in which experiments are performed using a whole, living organism. The two forms of in vivo experiments are animal studies and clinical trials during drug development. The overall effect of the experiment on a living organism can be observed in in vivo techniques. Thus, in vivo experiments are more precise than in vitro experiments.
In vivo, in vitro and in silico: an open space for the ...
Differences between in vitro, in vivo, and in silico studies
Ex vivo refers to experiments or studies conducted outside of a living organism, typically using isolated cells, tissues, or organs. In contrast, in vivo studies involve experiments conducted within a living organism, such as a mouse, rat, or human. Both approaches are essential for understanding biological processes and developing new ...
In Vivo vs In Vitro: Definition, Pros and Cons
Over the past 30 years researchers have developed a global picture of bacterial evolution by using both laboratory-basedin vivo evolution experiments and in silicoevolution of digital organisms.
In Vitro vs In Vivo Preclinical Studies
An in vivo experiment is a principle-testing experiment run in the context of an academic course. It is a laboratory-style multi-condition experiment conducted in the natural setting of student course work including the classroom, computer lab, study hall, dorm room, home, etc. The conditions in an in vivo experiment manipulate a small but ...
However, animal experiments represent intact organisms necessary to simulate the complex interplay of different process which is crucial for studying intestinal drug absorption. Thus, in vivo methodologies are widely used despite being expensive, time consuming and poorly correlating with humans [15], [16], [17].
The term in vivo refers to a medical test, experiment or procedure that is done on (or in) a living organism, such as a laboratory animal or human. The term in vitro, in contrast to in vivo, refers to a medical study or experiment which is done in the laboratory within the confines of a test tube or laboratory dish.
In Vivo vs. In Situ Experiments | Definition & Examples
the National Science Foundation. In vivo two-photon imaging experiments were performed at Stanford Wu Tsai Neuroscience Microscopy Service, with help from G. Wang. We gratefully acknowledge M. Goodman and A. Baghdasaryan at Stanford University for useful discussions and assistance. Funding: This work was supported by National Institutes of ...
In vivo antitumor activity was assessed in mouse xenografts harboring representative BRAF mutations and treated orally with different agents ... Sustained efficacy in combination with binimetinib was consistent with preclinical in vivo efficacy experiments in a splice variant-containing model and may result from more sustained inhibition of ...
Existing 3D Spatial Transcriptomics reconstruction approaches often overlook spatial information or experiment-induced distortions. Here, authors propose ST-GEARS to bridge the gap between in vivo ...
In conventional well plate-based experiments, it is difficult to observe the exosome movement in real time. Here, we developed a microfluidic-based GBA chip for co-culturing gut epithelial cells and neuronal cells and simultaneously observing exosome transport. ... The GBA-chip is aimed to mimic the in vivo situation of convective flow in blood ...