Advertisement

A systematic review of Earthquake Early Warning (EEW) systems based on Artificial Intelligence
- Published: 24 February 2024
- Volume 17 , pages 957–984, ( 2024 )
Cite this article
- Pirhossein Kolivand 1 ,
- Peyman Saberian 2 ,
- Mozhgan Tanhapour 3 ,
- Fereshteh Karimi 4 ,
- Sharareh Rostam Niakan Kalhori 3 ,
- Zohreh Javanmard 3 ,
- Soroush Heydari 3 ,
- Seyed Saeid Hoseini Talari 3 ,
- Seyed Mohsen Laal Mousavi 3 ,
- Maryam Alidadi 5 ,
- Mahnaz Ahmadi 6 &
- Seyed Mohammad Ayyoubzadeh 3 , 4 , 7
456 Accesses
Explore all metrics
Early Earthquake Warning (EEW) systems alarm about ongoing earthquakes to reduce their devastating human and financial damages. In complicated tasks like earthquake forecasting, Artificial Intelligence (AI) solutions show promising results. The goal of this review is to investigate the AI-based EEW systems. Web of Science, Scopus, Embase, and PubMed databases were systematically searched from its beginning until April 18, 2023. Studies that used AI algorithms to develop EEWs and forecast earthquake magnitude were qualified. The quality assessment was conducted using the Mixed Methods Assessment Tool version 2018. Detailed analysis was performed on 26 of 2604 retrieved articles. Researchers predict earthquakes most often using neural network family models (21 studies). Among eight categorized groups of parameters for earthquake forecasting, it was often predicted utilizing seismic wave characteristics (65.38%) and seismic activity data (61.54%). AI models most often predicted earthquake magnitude (32.69%) and depth (15.38%). Logistic Model Tree and Bayesian Network had the highest sensitivity, accuracy, and F-measure efficiency (99.9%). Findings showed that AI algorithms can forecast earthquakes. However, additional study is needed to determine the efficacy of more data-driven AI algorithms in mining seismic data using more input variables. This review is helpful for seismologists and researchers developing EEW systems using AI.
This is a preview of subscription content, log in via an institution to check access.
Access this article
Subscribe and save.
- Get 10 units per month
- Download Article/Chapter or eBook
- 1 Unit = 1 Article or 1 Chapter
- Cancel anytime
Price includes VAT (Russian Federation)
Instant access to the full article PDF.
Rent this article via DeepDyve
Institutional subscriptions
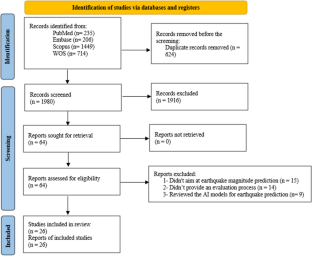
Similar content being viewed by others
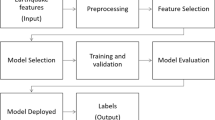
Machine learning for earthquake prediction: a review (2017–2021)
Medium–large earthquake magnitude prediction in tokyo with artificial neural networks.
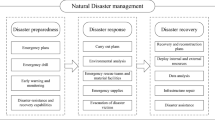
Can we detect trends in natural disaster management with artificial intelligence? A review of modeling practices
Explore related subjects.
- Artificial Intelligence
Data availability
No datasets were generated or analysed during the current study.
Abdalzaher MS, Krichen M, Yiltas-Kaplan D, Ben Dhaou I, Adoni WYH (2023) Early detection of earthquakes using IoT and cloud infrastructure: a survey. Sustainability 15(15):11713. https://doi.org/10.3390/su151511713
Article Google Scholar
Al Banna MH, Taher KA, Kaiser MS, Mahmud M, Rahman MS, Hosen AS, Cho GH (2020) Application of artificial intelligence in predicting earthquakes: state-of-the-art and future challenges. IEEE Access 8:192880–192923. https://doi.org/10.1109/ACCESS.2020.3029859
Allen CR (1976) Responsibilities in earthquake prediction: to the seismological society of America, delivered in Edmonton, Alberta, May 12, 1976. Bull Seismol Soc Am 66(6):2069–2074
Allen RM, Melgar D (2019) Earthquake early warning: advances, scientific challenges, and societal needs. Annual Rev Earth Planet Sci 47:361–388. https://doi.org/10.1146/annurev-earth-053018-060457
Article ADS CAS Google Scholar
Anders A (2013) Real-time decision support in the face of emerging natural hazard events [Doctoral dissertation, Technical University of Denmark]. Official research database of the Technical University of Denmark. https://orbit.dtu.dk/en/publications/real-time-decision-support-in-the-face-of-emerging-natural-hazard
Asim K, Martínez-Álvarez F, Basit A, Iqbal T (2017) Earthquake magnitude prediction in Hindukush region using machine learning techniques. Nat Hazards 85:471–486. https://doi.org/10.1007/s11069-016-2579-3
Bejani MM, Ghatee M (2021) A systematic review on overfitting control in shallow and deep neural networks. Artif Intell Rev 54:6391–6438. https://doi.org/10.1007/s10462-021-09975-1
Berhich A, Belouadha F-Z, Kabbaj MI (2021) LSTM-based earthquake prediction: enhanced time feature and data representation. Int J High Perform Syst Archit 10(1):1–11. https://doi.org/10.1504/IJHPSA.2021.115499
Biau G, Scornet E (2016) A random forest guided tour. TEST 25:197–227. https://doi.org/10.1007/s11749-016-0481-7
Article MathSciNet Google Scholar
Bilal MA, Ji Y, Wang Y, Akhter MP, Yaqub M (2022a) Early earthquake detection using batch normalization graph convolutional neural network (BNGCNN). Appl Sci 12(15):7548. https://doi.org/10.3390/app12157548
Article CAS Google Scholar
Bilal MA, Ji Y, Wang Y, Akhter MP, Yaqub M (2022b) An early warning system for earthquake prediction from seismic data using batch normalized graph convolutional neural network with attention mechanism (BNGCNNATT). Sensors 22(17):6482. https://doi.org/10.3390/s22176482
Article ADS PubMed PubMed Central Google Scholar
Burnett JW, Mothorpe C (2021) Human-induced earthquakes, risk salience, and housing values. Resour Energy Econ 63:101212. https://doi.org/10.1016/j.reseneeco.2020.101212
Chelidze T, Melikadze G, Kiria T, Jimsheladze T, Kobzev G (2020) Statistical and non-linear dynamics methods of Earthquake Forecast: application in the Caucasus. Front Earth Sci 8:194. https://doi.org/10.3389/feart.2020.00194
Article ADS Google Scholar
Cheng Z, Peng C, Chen M (2023) Real-time seismic intensity measurements prediction for earthquake early warning: a systematic literature review. Sensors 23(11):5052. https://doi.org/10.3390/s23115052
Chiang Y-J, Chin T-L, Chen D-Y (2022) Neural network-based strong motion prediction for on-site earthquake early warning. Sensors 22(3):704. https://doi.org/10.3390/s22030704
Chin T-L, Huang C-Y, Shen S-H, Tsai Y-C, Hu YH, Wu Y-M (2019) Learn to detect: improving the accuracy of earthquake detection. IEEE Trans Geoscience Remote Sens 57(11):8867–8878. https://doi.org/10.1109/TGRS.2019.2923453
Cochran ES, Kohler MD, Given DD, Guiwits S, Andrews J, Meier MA, Ahmad M, Henson I, Hartog R, Smith D (2018) Earthquake early warning ShakeAlert system: testing and certification platform. Seismol Res Lett 89(1):108–117. https://doi.org/10.1785/0220170138
Cremen G, Galasso C (2020) Earthquake early warning: recent advances and perspectives. Earth Sci Rev 205:103184. https://doi.org/10.1016/j.earscirev.2020.103184
Cremen G, Zuccolo E, Galasso C (2021) Accuracy and uncertainty analysis of selected methodological approaches to earthquake early warning in Europe. Seismological Soc Am 92(4):2321–2332
Google Scholar
Dallo I, Marti M, Clinton J, Böse M, Massin F, Zaugg S (2022) Earthquake early warning in countries where damaging earthquakes only occur every 50 to 150 years–the societal perspective. Int J Disaster Risk Reduct 83:103441. https://doi.org/10.1016/j.ijdrr.2022.103441
Debnath P, Chittora P, Chakrabarti T, Chakrabarti P, Leonowicz Z, Jasinski M, Gono R, Jasińska E (2021) Analysis of earthquake forecasting in India using supervised machine learning classifiers. Sustainability 13(2):971. https://doi.org/10.3390/su13020971
Department SR (2023) Number of earthquakes worldwide 2000–2021. Available at https://www.statista.com/statistics/263105/development-of-the-number-of-earthquakes-worldwide-since-2000/ . Accessed 13-09-2023
Dike HU, Zhou Y, Deveerasetty KK, Wu Q (2018) Unsupervised learning based on artificial neural network: a review. In: 2018 IEEE International Conference on Cyborg and Bionic Systems (CBS), IEEE
Dimililer K, Dindar H, Al-Turjman F (2021) Deep learning, machine learning and internet of things in geophysical engineering applications: an overview. Microprocessors Microsystems 80:103613. https://doi.org/10.1016/j.micpro.2020.103613
Essam Y, Kumar P, Ahmed AN, Murti MA, El-Shafie A (2021) Exploring the reliability of different artificial intelligence techniques in predicting earthquake for Malaysia. Soil Dyn Earthq Eng 147:106826. https://doi.org/10.1016/j.soildyn.2021.106826
Feng H, Miao Z, Hu Q (2022) Study on the uncertainty of machine learning model for earthquake-induced landslide susceptibility assessment. Remote Sens 14(13):2968. https://doi.org/10.3390/rs14132968
Galasso C, Zuccolo E, Aljawhari K, Cremen G, Melis NS (2023) Assessing the potential implementation of earthquake early warning for schools in the Patras region, Greece. Int J Disaster Risk Reduct 90:103610. https://doi.org/10.1016/j.ijdrr.2023.103610
Galkina A, Grafeeva N (2019) Machine learning methods for earthquake prediction: a survey. In: Proceedings of the Fourth Conference on Software Engineering and Information Management (SEIM-2019), Saint Petersburg, Russia
Gupta HK (2023) If a Magnitude∼ 8 earthquake occurs in India Today…. J Geol Soc India 99(3):299–302. https://doi.org/10.1007/s12594-023-2310-4
Gürsoy G, Varol A, Nasab A (2023) Importance of machine learning and deep learning algorithms in earthquake prediction: a review. In: 2023 11th International Symposium on Digital Forensics and Security (ISDFS), IEEE
Han Y, Zang Y, Meng L, Wang Y, Deng S, Ma Y, Xie M (2022) A summary of seismic activities in and around China in 2021. Earthq Res Adv 2(3):100157. https://doi.org/10.1016/j.eqrea.2022.100157
He C, Huang Q, Bai X, Robinson DT, Shi P, Dou Y, Zhao B, Yan J, Zhang Q, Xu F (2021) A global analysis of the relationship between urbanization and fatalities in earthquake-prone areas. Int J Disaster risk Sci 12:805–820. https://doi.org/10.1007/s13753-021-00385-z
Heaton TH (1985) A model for a seismic computerized alert network. Science 228(4702):987–990. https://doi.org/10.1126/science.228.4702.987
Article ADS CAS PubMed Google Scholar
Hong QN, Fàbregues S, Bartlett G et al (2018) The mixed methods appraisal tool (MMAT) version 2018 for information professionals and researchers. Educ Inf 34(4):285–291. https://doi.org/10.3233/EFI-180221
Jiao P, Alavi AH (2020) Artificial intelligence in seismology: advent, performance and future trends. Geosci Front 11(3):739–744. https://doi.org/10.1016/j.gsf.2019.10.004
Joshi A, Vishnu C, Mohan CK (2022) Early detection of earthquake magnitude based on stacked ensemble model. J Asian Earth Sciences: X 8:100122. https://doi.org/10.1016/j.jaesx.2022.100122
Kaftan I, Şalk M, Şenol Y (2017) Processing of earthquake catalog data of Western Turkey with artificial neural networks and adaptive neuro-fuzzy inference system. Arab J Geosci 10:1–9. https://doi.org/10.1007/s12517-017-3021-1
Kilb D, Bunn J, Saunders J, Cochran E, Minson S, Baltay A, O’Rourke C, Hoshiba M, Kodera Y (2021) The PLUM earthquake early warning algorithm: a retrospective case study of West Coast, USA, data. J Geophys Res: Solid Earth 126(7):e2020JB021053. https://doi.org/10.1029/2020JB021053
Liu Y, Yong S, He C, Wang X, Bao Z, Xie J, Zhang X (2022) An earthquake Forecast Model based on Multi-station PCA Algorithm. Appl Sci 12(7):3311. https://doi.org/10.3390/app12073311
Mahtta R, Fragkias M, Güneralp B, Mahendra A, Reba M, Wentz EA, Seto KC (2022) Urban land expansion: the role of population and economic growth for 300 + cities. Npj Urban Sustain 2(1):5. https://doi.org/10.1038/s42949-022-00048-y
Marhain S, Ahmed AN, Murti MA, Kumar P, El-Shafie A (2021) Investigating the application of artificial intelligence for earthquake prediction in Terengganu. Nat Hazards 108:977–999. https://doi.org/10.1007/s11069-021-04716-7
Meier MA, Kodera Y, Böse M, Chung A, Hoshiba M, Cochran E, Minson S, Hauksson E, Heaton T (2020) How often can earthquake early warning systems alert sites with high-intensity ground motion? J Geophys Res: Solid Earth 125(2):e2019JB017718. https://doi.org/10.1029/2019JB017718
Mignan A, Broccardo M (2020) Neural network applications in earthquake prediction (1994–2019): Meta-analytic and statistical insights on their limitations. Seismol Res Lett 91(4):2330–2342. https://doi.org/10.1785/0220200021
Mousavi SM, Beroza GC (2020) Bayesian-deep-learning estimation of Earthquake Location from single-station observations. IEEE Trans Geosci Remote Sens 58(11):8211–8224. https://doi.org/10.1109/TGRS.2020.2988770
Mousavi SM, Sheng Y, Zhu W, Beroza GC (2019a) STanford EArthquake dataset (STEAD): a global data set of seismic signals for AI. IEEE Access 7:179464–179476. https://doi.org/10.1109/ACCESS.2019.2947848
Mousavi SM, Zhu W, Ellsworth W, Beroza G (2019b) Unsupervised clustering of seismic signals using deep convolutional autoencoders. IEEE Geosci Remote Sens Lett 16(11):1693–1697. https://doi.org/10.1109/LGRS.2019.2909218
Murwantara IM, Yugopuspito P, Hermawan R (2020) Comparison of machine learning performance for earthquake prediction in Indonesia using 30 years historical data. TELKOMNIKA: Telecommunication, Computing, Electronics and Control 18(3):1331–1342. https://doi.org/10.12928/TELKOMNIKA.v18i3.14756
Nakano M, Sugiyama D, Hori T, Kuwatani T, Tsuboi S (2019) Discrimination of seismic signals from earthquakes and tectonic tremor by applying a convolutional neural network to running spectral images. Seismol Res Lett 90(2A):530–538. https://doi.org/10.1785/0220180279
Patterson J, Gibson A (2017) Deep learning: a practitioner’s approach. O’Reilly Media Inc.
Perol T, Gharbi M, Denolle M (2018) Convolutional neural network for earthquake detection and location. Sci Adv 4(2):e1700578. https://doi.org/10.1126/sciadv.1700578
Pirmagomedov R, Blinnikov M, Amelyanovich A, Glushakov R, Loskutov S, Koucheryavy A, Kirichek R, Bobrikova E (2018) IoT based earthquake prediction technology. In: Internet of Things, Smart Spaces, and Next Generation Networks and Systems: 18th International Conference, NEW2AN 2018, and 11th Conference, ruSMART 2018, St. Petersburg, Russia, August 27–29, 2018, Proceedings 18 Springer
Rahmani AM, Azhir E, Ali S, Mohammadi M, Ahmed OH, Ghafour MY, Ahmed SH, Hosseinzadeh M (2021) Artificial intelligence approaches and mechanisms for big data analytics: a systematic study. PeerJ Comput Sci 7:e488. https://doi.org/10.7717/peerj-cs.488
Article PubMed PubMed Central Google Scholar
Rajasekaran S, Pai GV (2017) Neural networks, fuzzy systems, and evolutionary algorithms: Synthesis and applications. PHI Learning Pvt. Ltd, New Delhi, pp 1–442
Rigatti SJ (2017) Random forest. J Insur Med 47(1):31–39. https://doi.org/10.17849/insm-47-01-31-39.1
Article PubMed Google Scholar
Roelofs R, Shankar V, Recht B, Fridovich-Keil S, Hardt M, Miller J, Schmidt L (2019) A meta-analysis of overfitting in machine learning. In: Proceedings of the 33rd conference on neural information processing systems (NeurIPS 2019), pp 1–10. Vancouver. Retrieved from https://proceedings.neurips.cc/paper_files/paper/2019/file/ee39e503b6bedf0c98c388b7e8589aca-Paper.pdf
Ross ZE, Meier MA, Hauksson E, Heaton TH (2018) Generalized seismic phase detection with deep learning. Bull Seismol Soc Am 108(5A):2894–2901. https://doi.org/10.1785/0120180080
Ross ZE, Yue Y, Meier MA, Hauksson E, Heaton TH (2019) PhaseLink: a deep learning approach to seismic phase association. J Geophys Res: Solid Earth 124(1):856–869. https://doi.org/10.1029/2018JB016674
Sadhukhan B, Chakraborty S, Mukherjee S (2023) Predicting the magnitude of an impending earthquake using deep learning techniques. Earth Sci Inf 16(1):803–823. https://doi.org/10.1007/s12145-022-00916-2
Salam MA, Ibrahim L, Abdelminaam DS (2021) Earthquake prediction using hybrid machine learning techniques. Int J Adv Comput Sci Appl 12(5):654–665. https://doi.org/10.14569/IJACSA.2021.0120578
Samui P, Kim D (2014) Applicability of artificial intelligence to reservoir induced earthquakes. Acta Geophys 62(3):608–619. https://doi.org/10.2478/s11600-014-0201-1
Satriano C, Wu Y-M, Zollo A, Kanamori H (2011) Earthquake early warning: concepts, methods and physical grounds. Soil Dyn Earthq Eng 31(2):106–118. https://doi.org/10.1016/j.soildyn.2010.07.007
Seto KC, Fragkias M, Güneralp B, Reilly MK (2011) A meta-analysis of global urban land expansion. PLoS ONE 6(8):e23777. https://doi.org/10.1371/journal.pone.0023777
Article ADS CAS PubMed PubMed Central Google Scholar
Thakur A, Konde A (2021) Fundamentals of neural networks. Int J Res Appl Sci Eng Technol 9:407–426
Thomas AM, Inbal A, Searcy J, Shelly DR, Bürgmann R (2021) Identification of low-frequency earthquakes on the San Andreas Fault with Deep Learning. Geophys Res Lett 48(13):e2021GL093157. https://doi.org/10.1029/2021GL093157
Vasti M, Dev A (2020) Classification and analysis of real-world earthquake data using various machine learning algorithms. In: Advances in Data Sciences, Security and Applications: Proceedings of ICDSSA 2019, Springer
Veroutsos E (2022) The World’s 10 Most Earthquake Prone Countries. Available at https://www.worldatlas.com/articles/the-world-s-10-most-earthquake-prone-countries.html . Accessed 17-09-2023
Wald DJ (2020) Practical limitations of earthquake early warning. Earthq Spectra 36(3):1412–1447. https://doi.org/10.1177/8755293020911388
Wang Q, Guo Y, Yu L, Li P (2020) Earthquakeprediction based on spatio-temporal data mining: an LSTM network approach. IEEE Trans Emerg Top Comput 8(1):148–158. https://doi.org/10.1109/TETC.2017.2699169
Wang Y, Li X, Wang Z, Liu J (2023) Deep learning for magnitude prediction in earthquake early warning. Gondwana Res 123:164–173
Yousefzadeh M, Hosseini SA, Farnaghi M (2021) Spatiotemporally explicit earthquake prediction using deep neural network. Soil Dyn Earthq Eng 144:106663. https://doi.org/10.1016/j.soildyn.2021.106663
Zhang X, Zhang M, Tian X (2021) Real-time earthquake early warning with deep learning: application to the 2016 M 6.0 Central Apennines, Italy earthquake. Geophys Res Lett 48(5):2020GL089394. https://doi.org/10.1029/2020GL089394
Zhang Y, Fung JF, Johnson KJ, Sattar S (2022) Review of seismic risk mitigation policies in earthquake-prone countries: lessons for earthquake resilience in the United States. J Earthquake Eng 26(12):6208–6235. https://doi.org/10.1080/13632469.2021.1911889
Zhao M, Xiao Z, Chen S, Fang L (2023) DiTing: a large-scale Chinese seismic benchmark dataset for artificial intelligence in seismology. Earthq Sci 36(2):84–94. https://doi.org/10.1016/j.eqs.2022.01.022
Zhu W, Mousavi SM, Beroza GC (2019) Seismic signal denoising and decomposition using deep neural networks. IEEE Trans Geosci Remote Sens 57(11):9476–9488. https://doi.org/10.1109/TGRS.2019.2926772
Zhu J, Li S, Song J (2022) Magnitude estimation for earthquake early warning with multiple parameter inputs and a support vector machine. Seismol Res Lett 93(1):126–136. https://doi.org/10.1785/0220210144
Zuccolo E, Cremen G, Galasso C (2021) Comparing the performance of regional earthquake early warning algorithms in Europe. Front Earth Sci 9:686272. https://doi.org/10.3389/feart.2021.686272
Download references
This study has been funded and supported by Iranian Red Crescent Society.
Author information
Authors and affiliations.
Department of Health Economics, Faculty of Medicine, Shahed University, Tehran, Iran
Pirhossein Kolivand
Department of Anesthesiology, Imam Khomeini Hospital Complex, Tehran University of Medical Sciences, Tehran, Iran
Peyman Saberian
Department of Health Information Management, School of Allied Medical Sciences, Tehran University of Medical Sciences, Tehran, Iran
Mozhgan Tanhapour, Sharareh Rostam Niakan Kalhori, Zohreh Javanmard, Soroush Heydari, Seyed Saeid Hoseini Talari, Seyed Mohsen Laal Mousavi & Seyed Mohammad Ayyoubzadeh
Research Center for Emergency and Disaster Resilience, Red Crescent Society of the Islamic Republic of Iran, Tehran, Iran
Fereshteh Karimi & Seyed Mohammad Ayyoubzadeh
Department of Health Information Technology and Management, School of Allied Medical Sciences, Shahid Beheshti University of Medical Sciences, Tehran, Iran
Maryam Alidadi
Medical Nanotechnology and Tissue Engineering Research Center, Shahid Beheshti University of Medical Sciences, Tehran, Iran
Mahnaz Ahmadi
Health Information Management Research Center, Tehran University of Medical Sciences, Tehran, Iran
Seyed Mohammad Ayyoubzadeh
You can also search for this author in PubMed Google Scholar
Contributions
Seyed Mohammad Ayyoubzadeh conceptualized the research. Pirhossein Kolivand, Sharareh Rostam Niakan Kalhori and Peyman Saberian supervised the manuscript. Mozhgan Tanhapour, Fereshteh Karimi, Zohreh Javanmard, Soroush Heydari, Seyed Saeid Hoseini Talari, Seyed Mohsen Laal Mousavi, and Maryam Alidadi wrote the original draft. Mahnaz Ahmadi reviewed the manuscript.
Corresponding author
Correspondence to Seyed Mohammad Ayyoubzadeh .
Ethics declarations
Competing interests.
The authors declare no competing interests.
Additional information
Communicated by: H. Babaie
Publisher’s Note
Springer Nature remains neutral with regard to jurisdictional claims in published maps and institutional affiliations.
Rights and permissions
Springer Nature or its licensor (e.g. a society or other partner) holds exclusive rights to this article under a publishing agreement with the author(s) or other rightsholder(s); author self-archiving of the accepted manuscript version of this article is solely governed by the terms of such publishing agreement and applicable law.
Reprints and permissions
About this article
Kolivand, P., Saberian, P., Tanhapour, M. et al. A systematic review of Earthquake Early Warning (EEW) systems based on Artificial Intelligence. Earth Sci Inform 17 , 957–984 (2024). https://doi.org/10.1007/s12145-024-01253-2
Download citation
Received : 14 November 2023
Accepted : 10 February 2024
Published : 24 February 2024
Issue Date : April 2024
DOI : https://doi.org/10.1007/s12145-024-01253-2
Share this article
Anyone you share the following link with will be able to read this content:
Sorry, a shareable link is not currently available for this article.
Provided by the Springer Nature SharedIt content-sharing initiative
- Earthquake forecasting
- Earthquake Early Warning system
- Seismic data mining
- Find a journal
- Publish with us
- Track your research
IEEE Account
- Change Username/Password
- Update Address
Purchase Details
- Payment Options
- Order History
- View Purchased Documents
Profile Information
- Communications Preferences
- Profession and Education
- Technical Interests
- US & Canada: +1 800 678 4333
- Worldwide: +1 732 981 0060
- Contact & Support
- About IEEE Xplore
- Accessibility
- Terms of Use
- Nondiscrimination Policy
- Privacy & Opting Out of Cookies
A not-for-profit organization, IEEE is the world's largest technical professional organization dedicated to advancing technology for the benefit of humanity. © Copyright 2024 IEEE - All rights reserved. Use of this web site signifies your agreement to the terms and conditions.
- A-Z Publications
Annual Review of Earth and Planetary Sciences
Volume 47, 2019, review article, earthquake early warning: advances, scientific challenges, and societal needs.
- Richard M. Allen 1 , and Diego Melgar 2
- View Affiliations Hide Affiliations Affiliations: 1 Department of Earth and Planetary Science, University of California, Berkeley, California 94720-4760, USA; email: [email protected] 2 Department of Earth Sciences, University of Oregon, Eugene, Oregon 97403-1272, USA; email: [email protected]
- Vol. 47:361-388 (Volume publication date May 2019) https://doi.org/10.1146/annurev-earth-053018-060457
- First published as a Review in Advance on January 30, 2019
- Copyright © 2019 by Annual Reviews. All rights reserved
- ▪ Earthquake early warning (EEW) is the rapid detection and characterization of earthquakes and delivery of an alert so that protective actions can be taken.
- ▪ EEW systems now provide public alerts in Mexico, Japan, South Korea, and Taiwan and alerts to select user groups in India, Turkey, Romania, and the United States.
- ▪ EEW methodologies fall into three categories, point source, finite fault, and ground motion models, and we review the advantages of each of these approaches.
- ▪ The wealth of information about EEW uses and user needs must be employed to focus future developments and improvements in EEW systems.
Article metrics loading...
Full text loading...
Literature Cited
- Allen RM 2011 . Earthquakes, early and strong motion warning. Encyclopedia of Solid Earth Geophysics HK Gupta 226– 33 Boston: Springer [Google Scholar]
- Allen RM , Cochran ES , Huggins T , Miles S , Otegui D 2017 . Quake warnings, seismic culture. Science 358 : 1111 [Google Scholar]
- Allen RM , Cochran ES , Huggins T , Miles S , Otegui D 2018 . Lessons from Mexico's earthquake early warning system. Eos Trans. AGU 99 [Google Scholar]
- Allen RM , Gasparini P , Kamigaichi O , Böse M 2009 . The status of earthquake early warning around the world: an introductory overview. Seismol . Res. Lett. 80 : 5 682– 93 [Google Scholar]
- Allen RM , Kanamori H 2003 . The potential for earthquake early warning in southern California. Science 300 : 786– 89 [Google Scholar]
- Allen RM , Ziv A 2011 . Application of real‐time GPS to earthquake early warning. Geophys. Res. Lett. 38 : 16 L16310 [Google Scholar]
- Asano K , Iwata T 2012 . Source model for strong ground motion generation in the frequency range 0.1–10 Hz during the 2011 Tohoku earthquake. Earth Planets Space 64 : 6 [Google Scholar]
- Atik LA , Abrahamson N , Bommer JJ , Scherbaum F , Cotton F , Kuehn N 2010 . The variability of ground-motion prediction models and its components. Seismol. Res. Lett. 81 : 5 794– 801 [Google Scholar]
- Barbour AJ , Crowell BW 2017 . Dynamic strains for earthquake source characterization. Seismol. Res. Lett. 88 : 2A 354– 70 [Google Scholar]
- Barnes CR , Best MM , Pautet L , Pirenne B 2011 . Understanding Earth–ocean processes using real-time data from NEPTUNE, Canada's widely distributed sensor networks, northeast Pacific. Geosci. Can. 38 : 1 21– 30 [Google Scholar]
- Bell SW , Forsyth DW , Ruan Y 2014 . Removing noise from the vertical component records of ocean‐bottom seismometers: results from year one of the Cascadia Initiative. Bull. Seismol. Soc. Am. 105 : 1 300– 13 [Google Scholar]
- Böse M , Felizardo C , Heaton TH 2015 . Finite-fault rupture detector (FinDer): going real-time in Californian ShakeAlert warning system. Seismol. Res. Lett. 86 : 6 1692– 704 [Google Scholar]
- Böse M , Hauksson E , Solanki K , Kanamori H , Heaton T 2009 . A new trigger criterion for improved real-time performance of onsite earthquake early warning in southern California. Bull. Seismol. Soc. Am. 99 : 897– 905 [Google Scholar]
- Böse M , Heaton TH , Hauksson E 2012 . Real-time finite fault rupture detector (FinDer) for large earthquakes. Geophys. J. Int. 191 : 2 803– 12 [Google Scholar]
- Böse M , Smith DE , Felizardo C , Meier MA , Heaton TH , Clinton JF 2017 . FinDer v. 2: improved real-time ground-motion predictions for M2–M9 with seismic finite-source characterization. Geophys. J. Int. 212 : 1 725– 42 [Google Scholar]
- Cauzzi C , Behr Y , Guenan TL , Douglas J , Auclair S et al. 2016 . Earthquake early warning and operational earthquake forecasting as real-time hazard information to mitigate seismic risk at nuclear facilities. Bull. Earthq. Eng. 14 : 2495– 512 [Google Scholar]
- Chamoli BP , Kumar A , Chen D-Y , Gairola A , Jakka RS et al. 2019 . A prototype earthquake early warning system for northern India. J. Earthq. Eng. In press [Google Scholar]
- Chen DY , Wu YM , Chin TL 2015 . Incorporating low-cost seismometers into the Central Weather Bureau seismic network for earthquake early warning in Taiwan. Terr. Atmos. Ocean. Sci. 26 : 5 503– 13 [Google Scholar]
- Chung AI , Cochran ES , Kaiser AE , Christensen CM , Yildirim B , Lawrence JF 2015 . Improved rapid magnitude estimation for a community‐based, low‐cost MEMS accelerometer network. Bull. Seismol. Soc. Am. 105 : 3 1314– 23 [Google Scholar]
- Chung AI , Henson I , Allen RM 2019 . Optimizing earthquake early warning performance: ElarmS-3. Seismol. Res. Lett . 90 : 2A 727– 43 [Google Scholar]
- Clayton RW , Heaton T , Kohler M , Chandy M , Guy R , Bunn J 2015 . Community seismic network: a dense array to sense earthquake strong motion. Seismol. Res. Lett. 86 : 5 1354– 63 [Google Scholar]
- Clinton J , Zollo A , Mărmureanu A , Zulfikar C , Parolai S 2016 . State-of-the art and future of earthquake early warning in the European region. Bull. Earthq. Eng. 14 : 9 2441– 58 [Google Scholar]
- Cochran ES , Kohler MD , Given DD , Guiwits S , Andrews J et al. 2017 . Earthquake early warning ShakeAlert system: testing and certification platform. Seismol. Res. Lett. 89 : 1 108– 17 [Google Scholar]
- Colombelli S , Allen RM , Zollo A 2013 . Application of real‐time GPS to earthquake early warning in subduction and strike‐slip environments. J. Geophys. Res. Solid Earth 118 : 7 3448– 61 [Google Scholar]
- Colombelli S , Zollo A 2015 . Fast determination of earthquake magnitude and fault extent from real-time P-wave recordings. Geophys. J. Int. 202 : 2 1158– 63 [Google Scholar]
- Colombelli S , Zollo A , Festa G , Picozzi M 2014 . Evidence for a difference in rupture initiation between small and large earthquakes. Nat. Commun. 5 : 3958 [Google Scholar]
- Cooper JD 1868 . Earthquake indicator San Franc. Bull. San Franc. Publ. Co San Francisco, CA: [Google Scholar]
- Crowell BW , Bock Y , Melgar D 2012 . Real‐time inversion of GPS data for finite fault modeling and rapid hazard assessment. Geophys. Res. Lett. 39 : 9 L09305 [Google Scholar]
- Crowell BW , Bock Y , Squibb MB 2009 . Demonstration of earthquake early warning using total displacement waveforms from real-time GPS networks. Seismol. Res. Lett. 80 : 5 772– 82 [Google Scholar]
- Crowell BW , Melgar D , Bock Y , Haase JS , Geng J 2013 . Earthquake magnitude scaling using seismogeodetic data. Geophys. Res. Lett. 40 : 23 6089– 94 [Google Scholar]
- Crowell BW , Melgar D , Geng J 2018a . Hypothetical real‐time GNSS modeling of the 2016 M w 7.8 Kaikōura earthquake: perspectives from ground motion and tsunami inundation prediction. Bull. Seismol. Soc. Am. 108 : 1736– 45 [Google Scholar]
- Crowell BW , Schmidt DA , Bodin P , Vidale JE , Baker B et al. 2018b . G‐FAST earthquake early warning potential for great earthquakes in Chile. Seismol. Res. Lett. 89 : 2A 542– 56 [Google Scholar]
- Crowell BW , Schmidt DA , Bodin P , Vidale JE , Gomberg J et al. 2016 . Demonstration of the Cascadia G‐FAST geodetic earthquake early warning system for the Nisqually, Washington, earthquake. Seismol. Res. Lett. 87 : 4 930– 43 [Google Scholar]
- Cua G , Heaton T 2007 . The virtual seismologist (VS) method: a Bayesian approach to earthquake early warning. Earthquake Early Warning Systems P Gasparini, G Manfredi, J Zschau 85– 132 Berlin: Springer [Google Scholar]
- Cuéllar A , Espinosa-Aranda JM , Suarez R , Ibarrola G , Uribe A et al. 2014 . The Mexican seismic alert system (SASMEX): its alert signals, broadcast results and performance during the M 7.4 Punta Maldonado earthquake of March 20th, 2012. Early Warning for Geological Disasters F Wenzel, Z Zschau 71– 87 Berlin: Springer-Verlag [Google Scholar]
- Cuéllar A , Suarez G , Espinosa-Aranda J 2018 . A fast earthquake early warning algorithm based on the first 3 s of the P-wave coda. Bull. Seismol. Soc. Am. 108 : 2068– 79 [Google Scholar]
- Ellsworth WL , Beroza GC 1995 . Seismic evidence for an earthquake nucleation phase. Science 268 : 5212 851– 55 [Google Scholar]
- Espinosa-Aranda JM , Jimenez A , Ibarrola G , Alcantar F , Aguilar A et al. 1995 . Mexico City seismic alert system. Seismol. Res. Lett. 66 : 42– 52 [Google Scholar]
- Evans JR , Allen RM , Chung AI , Cochran ES , Guy R et al. 2014 . Performance of several low‐cost accelerometers. Seismol. Res. Lett. 85 : 1 147– 58 [Google Scholar]
- Given DD , Cochran ES , Heaton T , Hauksson E , Allen RM et al. 2014 . Technical implementation plan for the ShakeAlert production system—an earthquake early warning system for the West Coast of the United States Open-File Rep. 2014-1097, US Geol. Surv Reston, VA: https://doi.org/10.3133/ofr20141097 [Crossref] [Google Scholar]
- Goldberg DE , Melgar D , Bock Y , Allen RM 2018 . Geodetic observations of weak determinism in rupture evolution of large earthquakes. J. Geophys. Res. Solid Earth 123 : 9950– 62 [Google Scholar]
- Grapenthin R , Johanson IA , Allen RM 2014a . Operational real‐time GPS‐enhanced earthquake early warning. J. Geophys. Res. Solid Earth 119 : 10 7944– 65 [Google Scholar]
- Grapenthin R , Johanson IA , Allen RM 2014b . The 2014 M w 6.0 Napa earthquake, California: observations from real‐time GPS‐enhanced earthquake early warning. Geophys. Res. Lett. 41 : 23 8269– 76 [Google Scholar]
- Hoshiba M 2013 . Real-time prediction of ground motion by Kirchhoff Fresnel boundary integral equation method: extended front detection method for earthquake early warning. J. Geophys. Res. Solid Earth 118 : 1038– 50 [Google Scholar]
- Hoshiba M 2014 . Review of the nationwide earthquake early warning in Japan during its first five years. Earthquake Hazard, Risk, and Disasters JF Shroder, M Wyss 505– 29 Waltham, MA: Academic [Google Scholar]
- Hoshiba M , Aoki S 2015 . Numerical shake prediction for earthquake early warning: data assimilation, real-time shake mapping, and simulation of wave propagation. Bull. Seismol. Soc. Am. 105 : 1324– 38 [Google Scholar]
- Hoshiba M , Iwakiri K 2011 . Initial 30 seconds of the 2011 off the Pacific coast of Tohoku earthquake ( M w 9.0)—amplitude and τ c for magnitude estimation for earthquake early warning. Earth Planets Space 63 : 553– 57 [Google Scholar]
- Hoshiba M , Ozaki T 2014 . Earthquake early warning and tsunami warning of the Japan Meteorological Agency, and their performance in the 2011 off the Pacific coast of Tohoku earthquake (M9.0). Early Warning for Geological Disasters F Wenzel, J Zschau 1– 28 Berlin: Springer-Verlag [Google Scholar]
- Hsu TY , Lin PY , Wang HH , Chiang HW , Chang YW et al. 2018 . Comparing the performance of the NEEWS earthquake early warning system against the CWB system during the 6 February 2018 M w 6.2 Hualien earthquake. Geophys. Res. Lett. 45 : 6001– 7 [Google Scholar]
- Hsu TY , Wang HH , Lin PY , Lin CM , Kuo CH , Wen KL 2016 . Performance of the NCREE's on-site warning system during the 5 February 2016 M w 6.53 Meinong earthquake. Geophys . Res. Lett 43 : 8954– 59 [Google Scholar]
- Johnson L , Rabinovici S , Kang G , Mahin SA 2016 . California earthquake early warning system benefit study CSSC Publ.16-04 PEER Rep. 2016/06, Pac Earthq. Eng. Res. Cent Berkeley, CA: [Google Scholar]
- Kanazawa T , Uehira K , Mochizuki M , Shinbo T , Fujimoto H et al. 2016 . S-NET project, cabled observation network for earthquakes and tsunamis Paper presented at the 9th Conference in the SubOptic Series, Dubai, Apr 18– 21 [Google Scholar]
- Kawamoto S , Hiyama Y , Ohta Y , Nishimura T 2016 . First result from the GEONET real-time analysis system (REGARD): the case of the 2016 Kumamoto earthquakes. Earth Planets Space 68 : 1 190 [Google Scholar]
- Kawamoto S , Ohta Y , Hiyama Y , Todoriki M , Nishimura T et al. 2017 . REGARD: a new GNSS‐based real‐time finite fault modeling system for GEONET. J. Geophys. Res. Solid Earth 122 : 2 1324– 49 [Google Scholar]
- Kodera Y 2018 . Real‐time detection of rupture development: earthquake early warning using P waves from growing ruptures. Geophys. Res. Lett. 45 : 1 156– 65 [Google Scholar]
- Kodera Y , Saitou J , Hayashimoto N , Adachi S , Morimoto M et al. 2016 . Earthquake early warning for the 2016 Kumamoto earthquake: performance evaluation of the current system and the next-generation methods of the Japan Meteorological Agency. Earth Planets Space 68 : 1 202 [Google Scholar]
- Kodera Y , Yamada Y , Hirano K , Tamaribuchi K , Adachi S et al. 2018 . The propagation of local undamped motion (PLUM) method: a simple and robust seismic wavefield estimation approach for earthquake early warning. Bull. Seismol. Soc. Am. 108 : 2 983– 1003 [Google Scholar]
- Kohler M , Cochran E , Given D , Guiwits S , Neuhauser D et al. 2017 . Earthquake early warning ShakeAlert system: West Coast wide production prototype. Seismol. Res. Lett. 89 : 1 99– 107 [Google Scholar]
- Kong Q , Allen RM , Schreier L 2016a . MyShake: initial observations from a global smartphone seismic network. Geophys. Res. Lett. 43 : 18 9588– 94 [Google Scholar]
- Kong Q , Allen RM , Schreier L , Kwon YW 2016b . MyShake: a smartphone seismic network for earthquake early warning and beyond. Sci. Adv. 2 : 2 e1501055 [Google Scholar]
- Kuyuk HS , Allen RM 2013 . Optimal seismic network density for earthquake early warning: a case study from California. Seismol. Res. Lett. 84 : 6 946– 54 [Google Scholar]
- Kuyuk HS , Allen RM , Brown H , Hellweg M , Henson I , Neuhauser D 2014 . Designing a network‐based earthquake early warning algorithm for California: ElarmS‐2. Bull. Seismol. Soc. Am. 104 : 162– 73 [Google Scholar]
- Li S 2018 . Approaching earthquake early-warning. Overv. Disaster Prev. 2 : 14– 24 [Google Scholar]
- Liu A , Yamada M 2014 . Bayesian approach for identification of multiple events in an early warning system. Bull. Seismol. Soc. Am. 104 : 3 1111– 21 [Google Scholar]
- Lu C , Zhou L , Zhang Z 2016 . Research and test on China high-speed railway earthquake early-warning system. Sci. Technol. Rev. 34 : 18 258– 64 [Google Scholar]
- Mărmureanu A , Ionescu C , Cioflan CO 2010 . Advanced real-time acquisition of the Vrancea earthquake early warning system. Soil Dyn. Earthq. Eng. 31 : 163– 69 [Google Scholar]
- Meier MA 2017 . How “good” are real‐time ground motion predictions from earthquake early warning systems?. J. Geophys. Res. Solid Earth 122 : 7 5561– 77 [Google Scholar]
- Meier MA , Ampuero JP , Heaton TH 2017 . The hidden simplicity of subduction megathrust earthquakes. Science 357 : 6357 1277– 81 [Google Scholar]
- Meier MA , Heaton T , Clinton J 2015 . The Gutenberg algorithm: evolutionary Bayesian magnitude estimates for earthquake early warning with a filter bank. Bull. Seismol. Soc. Am. 105 : 5 2774– 86 [Google Scholar]
- Meier MA , Heaton T , Clinton J 2016 . Evidence for universal earthquake rupture initiation behavior. Geophys. Res. Lett. 43 : 15 7991– 96 [Google Scholar]
- Melgar D , Bock Y , Crowell BW 2012 . Real-time centroid moment tensor determination for large earthquakes from local and regional displacement records. Geophys. J. Int. 188 : 2 703– 18 [Google Scholar]
- Melgar D , Crowell BW , Bock Y , Haase JS 2013 . Rapid modeling of the 2011 Mw 9.0 Tohoku‐Oki earthquake with seismogeodesy. Geophys. Res. Lett. 40 : 12 2963– 68 [Google Scholar]
- Melgar D , Crowell BW , Geng J , Allen RM , Bock Y et al. 2015 . Earthquake magnitude calculation without saturation from the scaling of peak ground displacement. Geophys. Res. Lett. 42 : 13 5197– 205 [Google Scholar]
- Melgar D , Hayes GP 2017 . Systematic observations of the slip pulse properties of large earthquake ruptures. Geophys. Res. Lett. 44 : 19 9691– 98 [Google Scholar]
- Melgar D , LeVeque RJ , Dreger DS , Allen RM 2016 . Kinematic rupture scenarios and synthetic displacement data: an example application to the Cascadia subduction zone. J. Geophys. Res. Solid Earth 121 : 9 6658– 74 [Google Scholar]
- Minson SE , Brooks BA , Glennie CL , Murray JR , Langbein JO et al. 2015 . Crowdsourced earthquake early warning. Sci. Adv. 1 : 3 e1500036 [Google Scholar]
- Minson SE , Meier MA , Baltay AS , Hanks TC , Cochran ES 2018 . The limits of earthquake early warning: timeliness of ground motion estimates. Sci. Adv. 4 : 3 eaaq0504 [Google Scholar]
- Minson SE , Murray JR , Langbein JO , Gomberg JS 2014 . Real‐time inversions for finite fault slip models and rupture geometry based on high‐rate GPS data. J. Geophys. Res. Solid Earth 119 : 4 3201– 31 [Google Scholar]
- Minson SE , Wu S , Beck JL , Heaton TH 2017 . Combining multiple earthquake models in real time for earthquake early warning. Bull. Seismol. Soc. Am. 107 : 4 1868– 82 [Google Scholar]
- Montagner JP , Juhel K , Barsuglia M , Ampuero JP , Chassande-Mottin E et al. 2016 . Prompt gravity signal induced by the 2011 Tohoku-Oki earthquake. Nat. Commun. 7 : 13349 [Google Scholar]
- Murray JR , Crowell BW , Grapenthin R , Hodgkinson K , Langbein JO et al. 2018 . Development of a geodetic component for the US West Coast earthquake early warning system. Seismol. Res. Lett. 89 2322– 36 [Google Scholar]
- Nakamura Y 1988 . On the urgent earthquake detection and alarm system (UrEDAS). Proceedings of the 9th World Conference on Earthquake Engineering Vol 7 673– 78 Tokyo-Kyoto, Japan: Jpn. Assoc. Earthq. Disaster Prev. [Google Scholar]
- Nakamura Y , Tucker B 1988 . Japan's earthquake early warning system: Should it be imported to California?. Calif. Geol. 41 : 33– 40 [Google Scholar]
- Noda S , Ellsworth WL 2016 . Scaling relation between earthquake magnitude and the departure time from P wave similar growth. Geophys. Res. Lett. 43 : 17 9053– 60 [Google Scholar]
- Noda S , Ellsworth WL 2017 . Determination of earthquake magnitude for early warning from the time dependence of P ‐wave amplitudes. Bull. Seismol. Soc. Am. 107 : 4 1860– 67 [Google Scholar]
- Noda S , Yamamoto S , Ellsworth WL 2016 . Rapid estimation of earthquake magnitude from the arrival time of the peak high‐frequency amplitude. Bull. Seismol. Soc. Am. 106 : 1 232– 41 [Google Scholar]
- Nof RN , Allen RM 2016 . Implementing the ElarmS earthquake early warning algorithm on the Israeli Seismic Network. Bull. Seismol. Soc. Am. 106 : 2332– 44 [Google Scholar]
- Ohta Y , Kobayashi T , Tsushima H , Miura S , Hino R et al. 2012 . Quasi real‐time fault model estimation for near‐field tsunami forecasting based on RTK‐GPS analysis: application to the 2011 Tohoku‐Oki earthquake ( M w 9.0). J. Geophys. Res. Solid Earth 117 : B2 B02311 [Google Scholar]
- Olson EL , Allen RM 2005 . The deterministic nature of earthquake rupture. Nature 438 : 7065 212– 15 [Google Scholar]
- Perol T , Gharbi M , Denolle M 2018 . Convolutional neural network for earthquake detection and location. Sci. Adv. 4 : 2 e1700578 [Google Scholar]
- Porter K , Shoaf K , Seligson H 2006 . Value of injuries in the Northridge earthquake. Earthq . Spectra 22 : 555– 63 [Google Scholar]
- Ross ZE , Meier MA , Hauksson E , Heaton TH 2018 . Generalized seismic phase detection with deep learning. Bull. Seismol. Soc. Am 108 : 2894– 901 [Google Scholar]
- Ruhl CJ , Melgar D , Chung AI , Grapenthin R , Allen RM 2019a . Quantifying the value of real-time geodetic constraints on earthquake early warning using a global seismic and geodetic dataset. arXiv:1901.11124 [physics.geo-ph]
- Ruhl CJ , Melgar D , Geng J , Goldberg DE , Crowell BW et al. 2019b . A global database of strong‐motion displacement GNSS recordings and an example application to PGD scaling. Seismol . Res. Lett 90 : 1 271– 79 [Google Scholar]
- Ruhl CJ , Melgar D , Grapenthin R , Allen RM 2017 . The value of real‐time GNSS to earthquake early warning. Geophys. Res. Lett. 44 : 16 8311– 19 [Google Scholar]
- Rydelek P , Horiuchi S 2006 . Earth science: Is earthquake rupture deterministic. ? Nature 442 : 7100 E5 [Google Scholar]
- Satriano C , Elia L , Martino C , Lancieri M , Zollo A , Iannaccone G 2010 . PRESTo, the earthquake early warning system for southern Italy: concepts, capabilities and future perspectives. Soil Dyn. Earthq. Eng. 31 : 137– 53 [Google Scholar]
- Saunders JK , Goldberg DE , Haase JS , Bock Y , Offield DG et al. 2016 . Seismogeodesy using GPS and low-cost MEMS accelerometers: perspectives for earthquake early warning and rapid response. Bull. Seismol. Soc. Am . 106 : 2469– 89 [Google Scholar]
- Seki T , Okada T , Ikeda M , Sugano T 2008 . Early warning “area mail. NTT Tech. Rev. 6 : 12 1– 6 [Google Scholar]
- Sheen D-H , Park J-H , Chi H-C , Hwang E-H , Lim I-S et al. 2017 . The first stage of an earthquake early warning system in South Korea. Seismol. Res. Lett. 88 : 6 1491– 98 [Google Scholar]
- Shoaf KI , Nguyen LH , Sareen HR , Bourque LB 1998 . Injuries as a result of California earthquakes in the past decade. Disasters 22 : 218– 35 [Google Scholar]
- Strauss JA , Allen RM 2016 . Benefits and costs of earthquake early warning. Seismol. Res. Lett. 87 : 3 765– 72 [Google Scholar]
- Tréhu AM , Wilcock WS , Hilmo R , Bodin P , Connolly J et al. 2018 . The role of the Ocean Observatories Initiative in monitoring the offshore earthquake activity of the Cascadia subduction zone. Oceanography 31 : 1 104– 13 [Google Scholar]
- Vallée M , Ampuero JP , Juhel K , Bernard P , Montagner JP , Barsuglia M 2017 . Observations and modeling of the elastogravity signals preceding direct seismic waves. Science 358 : 6367 1164– 68 [Google Scholar]
- Webb SC 1998 . Broadband seismology and noise under the ocean. Rev. Geophys. 36 : 1 105– 42 [Google Scholar]
- Wood MM , Mileti DS , Kano M , Kelley MM , Regan R , Bourque LB 2012 . Communicating actionable risk for terrorism and other hazards. Risk Anal 32 : 4 601– 15 [Google Scholar]
- Worden CB , Wald DJ , Allen TI , Lin K , Garcia D , Cua G 2010 . A revised ground-motion and intensity interpolation scheme for ShakeMap. Bull. Seismol. Soc. Am. 100 : 6 3083– 96 [Google Scholar]
- Wright TJ , Houlié N , Hildyard M , Iwabuchi T 2012 . Real‐time, reliable magnitudes for large earthquakes from 1 Hz GPS precise point positioning: the 2011 Tohoku‐Oki (Japan) earthquake. Geophys. Res. Lett. 39 : 12 L12302 [Google Scholar]
- Wu Y-M , Hsiao N-C , Chin T-L , Chen D-Y , Chan Y-T , Wang K-S 2014 . Earthquake early warning system in Taiwan. Encyclopedia of Earthquake Engineering M Beer, IA Kougioumtzoglou, E Patelli, S-K Au Berlin: Springer https://doi.org/10.1007/978-3-642-36197-5_99-1 [Crossref] [Google Scholar]
- Wu Y-M , Mittal H , Huang T-C , Yang BM , Jan J-C , Chen SK 2018 . Performance of a low-cost earthquake early warning system ( P -alert) and shake map production during the 2018 M w 6.4 Hualien, Taiwan, earthquake. Seismol. Res. Lett. 90 : 1 19– 29 [Google Scholar]
Data & Media loading...
- Article Type: Review Article
Most Read This Month
Most cited most cited rss feed, geologic evolution of the himalayan-tibetan orogen, chemical geodynamics, tectonic implications of the composition of volcanic arc magmas, global glacial isostasy and the surface of the ice-age earth: the ice-5g (vm2) model and grace, oxygen and hydrogen isotopes in the hydrologic cycle, laboratory-derived friction laws and their application to seismic faulting, arc assembly and continental collision in the neoproterozoic east african orogen: implications for the consolidation of gondwanaland, united plates of america, the birth of a craton: early proterozoic assembly and growth of laurentia, biogenic manganese oxides: properties and mechanisms of formation, a tale of amalgamation of three permo-triassic collage systems in central asia: oroclines, sutures, and terminal accretion.

An official website of the United States government
The .gov means it’s official. Federal government websites often end in .gov or .mil. Before sharing sensitive information, make sure you’re on a federal government site.
The site is secure. The https:// ensures that you are connecting to the official website and that any information you provide is encrypted and transmitted securely.
- Publications
- Account settings
Preview improvements coming to the PMC website in October 2024. Learn More or Try it out now .
- Advanced Search
- Journal List
- Sensors (Basel)

A Review on the Development of Earthquake Warning System Using Low-Cost Sensors in Taiwan
1 Department of Geosciences, National Taiwan University, Taipei 10617, Taiwan
2 Institute of Earth Sciences, Academia Sinica, Taipei 11529, Taiwan
3 Research Center for Future Earth, National Taiwan University, Taipei 10617, Taiwan
Himanshu Mittal
4 National Center for Seismology, Ministry of Earth Sciences, Government of India, New Delhi 110003, India; [email protected]
Associated Data
The strong motion waveform records from the P-Alert network used in this study can be downloaded at http://palert.earth.sinica.edu.tw/db/ (accessed on 15 September 2021).
Seismic instrumentation for earthquake early warnings (EEWs) has improved significantly in the last few years, considering the station coverage, data quality, and the related applications. The official EEW system in Taiwan is operated by the Central Weather Bureau (CWB) and is responsible for issuing the regional warning for moderate-to-large earthquakes occurring in and around Taiwan. The low-cost micro-electro-mechanical system (MEMS)-based P-Alert EEW system is operational in Taiwan for on-site warnings and for producing shakemaps. Since 2010, this P-Alert system, installed by the National Taiwan University (NTU), has shown its importance during various earthquakes that caused damage in Taiwan. Although the system is capable of acting as a regional as well as an on-site warning system, it is particularly useful for on-site warning. Using real-time seismic signals, each P-Alert system can provide a 2–8 s-long warning time for the locations situated in the blind zone of the CWB regional warning system. The shakemaps plotted using this instrumentation help to assess the damage pattern and rupture directivity, a key feature in the risk mitigation process. These shakemaps are delivered to the intended users, including the disaster mitigation authorities, for possible relief purposes. Earlier, the network provided only peak ground acceleration (PGA) shakemaps, but has now been updated to include peak ground velocity (PGV), spectral acceleration ( S a ) at different periods, and CWB intensity maps. The PGA and PGV shakemaps plotted using this network have proven helpful in establishing the fact that PGV is a better indicator of damage detection than PGA. This instrumentation is also useful in structural health-monitoring and estimating co-seismic deformations. Encouraged by the performance of the P-Alert network, more instruments are installed in Asia-Pacific countries.
1. Introduction
Every year, various natural hazards, including earthquakes, cause fatalities and property damage by affecting numerous people around the world. With advancements in technology and data processing speed, risk mitigation tools, such as earthquake early warnings (EEW), have emerged as life-saving guards in many earthquake-prone countries. The primary purpose of EEW is to detect an earthquake in the early stage, estimating the shaking intensity in the target regions, and to warn the users before experiencing the strong ground motion. Unlike other warning systems (typhoon, tsunami, volcano, flood, etc.), an hours- or minutes-long warning time is generally not possible. Despite this, the seconds-long warning achieved during EEW may be very helpful in saving the lives of human beings by allowing them to flee from buildings (if possible), or to take the proper shelter, or to move to a safer place within a building. Many countries, including Mexico [ 1 , 2 ], Japan [ 3 ], Taiwan [ 4 , 5 ], and South Korea [ 6 ], have developed EEW systems and are issuing warnings to the public and to authorities. Several other countries, namely, the United States [ 7 ], China [ 8 ], Turkey [ 9 ], Italy [ 10 ], and India [ 11 , 12 ], are in the process of developing and testing the EEW system [ 13 ].
The EEW system, in its principle form, takes information related to basic phases (P-wave and other phases) from the real-time seismic signals, performs the elementary calculations, and, if needed, issues a warning. The EEW system is not limited to major earthquakes only, but also targets smaller earthquakes, which may cause high shaking in local areas, as well. EEW systems are generally categorized as regional (network-based) and on-site (single station or network-based) systems. The regional system consists of several sensors placed around the fault/source, and seismic signals from these instruments are transferred continuously to the central station for processing [ 14 , 15 , 16 , 17 , 18 ]. The regional EEW system exploits the use of P-wave and some S-wave information [ 19 ] to estimate the location and magnitude of the earthquake and to predict the ground-shaking at farther distances using ground-motion prediction equations (GMPE). Once the earthquake is triggered using short-term averaging (STA), long-term averaging (LTA), or another algorithm, the initial few seconds of data (typically 3–5 s) after the P-wave’s arrival is used to perform the calculations. The regional EEW system generally takes about 10–15 s to detect an earthquake and issue a warning. By that time, the damaging S-waves reach some of the locations close to the epicenter and a warning is not possible. The areas without a warning are termed “blind zones” and may range around 40–60 km from the epicenter, depending upon how quickly an earthquake is located. The problem of the blind zone can be overcome by the on-site EEW system, under which a single station installed in the proximity of the target area will immediately sense the earthquake and issue the warning. This system will use the P-wave information and estimate the ground shaking using empirical scaling relationships. The on-site warning system is faster than the regional system and can provide early warnings to sites located in the near-source region. The accuracy of the estimation of earthquake parameters is moderate for an on-site EEW, as it is a single sensor-based or a small network-based system. The on-site EEW is functional in many countries, for example, in Japan [ 20 ], Bucharest, Romania [ 21 ], Istanbul [ 9 ], and Taiwan [ 22 ]. In Romania, a simple and effective EEW system is designed from the earthquakes in the Romanian Vranceazone, south-eastern Carpathians, and provides useful lead-times. The important parameter in EEW is the available time before the arrival of damaging S-waves’ or surface waves’ peak amplitude (called the lead-time). Based on the hypocentral distance between the source and target, the lead-time may be different using two approaches. Using the regional approach, a greater lead-time is achieved at larger distances; however, at smaller distances, on-site EEW systems are useful when the regional EEW fails.
Figure 1 depicts the difference in time taken for the issuance of the regional and on-site warnings. The left side shows the 13 s regional warning (lead-time) with intensity 4, issued by the Central Weather Bureau (CWB) network for Taipei City during the Hualien earthquake of 6 February 2018. The CWB network took 18 s to predict the intensity and issued the regional warning after the earthquake’s occurrence. By the time CWB issued the warning, the damaging S-waves reached the area encircled by the black line, where no warning was possible. However, the on-site warning network worked very well in this blind zone and issued the on-site warning (2–8 s). The working of the on-site EEW network during the Hualien earthquake is discussed by Wu et al. (2019) [ 15 ]. The right side of Figure 1 shows the peak amplitude of the vertical displacement, P d , estimated using the initial 3 s of P waves at one of the stations of the P-Alert network located at an epicentral distance of 19 km. Based on the previous works [ 23 , 24 ], P d is the recommended parameter for on-site EEWs, where shaking corresponding to P d > 0.5 cm may cause damage. The threshold value P d ≥ 0.35 cm for issuing the warning is obtained at 1.5 s after the P arrival. It could have several seconds of lead-time before peak ground motion, which shows the efficiency of on-site warnings in the blind zones of regional warnings.

A comparison of time taken for the regional and on-site warnings. The left side shows that the CWB network took 18 s to issue a 13 s regional warning (lead-time) for Taipei City during the Hualien earthquake of 6 February 2018. The right side of the figure shows the P d estimation using the initial 3 s of the waveform at one of the stations of the P-Alert network, where the threshold value was achieved in 1.5 s.
Building an efficient EEW system is a cost-effective process, as the stations should be densely distributed to detect and report the earthquake in minimal time. Generally, for a small area, EEW may be achieved by placing numerous instruments around seismogenic sources. A dense EEW system using traditional sensors is not feasible, especially in countries facing seismic hazards from a wide area. However, in recent years, low-cost sensors have emerged as an alternative to traditional sensors. The low-cost micro-electro-mechanical system (MEMS) sensors were introduced for EEW in 1990 [ 25 ] and are used by most of the countries working with EEW. Some countries have built their EEW network using these sensors only.
2. The EEW Systems in Taiwan
Taiwan is one of the countries that faces frequent seismic activities due to the ongoing subduction of the Philippine and Eurasian plates. Given the importance of property damage and economic loss, an EEW was conceptualized in Taiwan after the occurrence of the Hualien offshore M w 7.8 earthquake that caused extensive damage in the capital city Taipei in 1986, around 120 km away from the epicenter. S waves take more than 30 s to cover this distance. If a seismic system can detect an earthquake in the Hualien region within 20 s, then there could be a 10 s warning time for the Taipei metropolitan region. Thus, the implementation of the prototype EEW system by the CWB started in 1994 [ 26 ]. After several years of development, the CWB established a nationwide EEW system in 2002 [ 27 , 28 ]. To shorten the earthquake reporting time, the P-wave method is used in the CWB system [ 29 , 30 , 31 ]. Currently, the CWB system can regularly issue warnings within 20 s of an earthquake’s occurrence ( Figure 1 ). The CWB system is in charge of providing earthquake alerts in Taiwan via text message through mobile phones, TVs, and direct broadcasting systems in schools [ 4 , 32 ]. This is a regional network and issues warning to places that are around 40–60 km away from the epicenter.
The other EEW system, run by the National Center for Research on Earthquake Engineering (NCREE), comprises 90 instruments installed in elementary schools and acts as an on-site and hybrid network [ 22 ]. Both of the networks discussed above function very well and the CWB system is capable enough to detect earthquakes and issue the warning. However, to study the fault mechanism in detail, and to issue the warning in the blind zone using the on-site mechanism, the instruments should be placed densely around the fault zone. Taiwan has many faults, inland and offshore, so many instruments are required to instrument the fault zones densely. As the traditional instruments are not feasible to place closely together, the National Taiwan University (NTU) installed the low-cost sensors to minimize the cost.
3. The P-Alert Sensors and EEW
The research group at NTU worked in close association with a technology company in Taiwan for the development of a low-cost, MEMS-based, P-wave alert device named “P-Alert”. These MEMS-based sensors are embedded in a small housing and can record high-frequency, near-source ground-motion. The pilot project commenced in 2010 by installing 15 P-Alert devices in the Hualien part of the country [ 33 ]. The network showed its ability by detecting and recording earthquakes. Based on its performance in the Hualien region, the network was extended to other parts of the country. As of now, 761 P-Alert instruments have been installed under this network ( Figure 2 ). With a threshold parameters algorithm embedded inside, the P-Alert sensors are suitable for on-site, as well as for regional warnings. Considering the proper logistics (continuous power supply and dedicated internet connection for data transfer) for the P-Alert installation, most of these instruments are installed in elementary schools on the ground or first floor. Each P-Alert device can record three-component data, having 16-bit resolution and ±2 g of full dynamic range. The sampling rate of all the instruments is set to be 100 Hz and the real-time three-component continuous data is transferred and processed continuously at the central receiving station placed at NTU and Academia Sinica.

A map showing the distribution of P-Alert instruments in different parts of Taiwan. This dense array, consisting of 761 low-cost sensors, can act as an on-site, as well as a regional EEW system.
For on-site warning, as per the algorithm embedded in the P-Alert instruments, the data received by each of the field instruments is continuously monitored for STA/LTA ratios, peak ground acceleration (PGA), and P d obtained by double-integrating the real-time data. The records are high-pass filtered after taking the integration. Once an earthquake has been declared using STA/LTA algorithm, the software will look for P d and PGA, using the initial few seconds of data (usually 3–4 s) after the P-wave arrival [ 23 ] as the warning. Once the threshold parameters are exceeded ( P d ≥ 0.35 cm or PGA ≥ 80 gals), a warning is issued [ 34 ].
Numerous works have been carried out for on-site warnings using the initial portion of the P-waves. Wu and Kanamori (2005b) [ 35 ] suggested the prediction of earthquake magnitude by using the inverse of the predominant period, τ c , from the initial 3 s of P-wave waveforms. Considering the trade-off between cost and data quality, the dynamic range of P-Alert sensors is less than that of traditional sensors. Thus, the calculation of traditional frequency-based parameters proposed earlier for EEW, such as τ c , may not be accurate. The same authors [ 23 ] established a regression relationship between P d and Peak Ground Velocity (PGV), and proposed that earthquakes may be damaging whenever P d ≥ 0.5 cm . Wu and Kanamori (2008) [ 24 ] also worked to predict PGV with P d , using the various earthquakes recorded in Taiwan, Japan, and California ( Figure 3 ). Using the regression carried out from data from different parts of the world, the P d parameter is considered one of the pioneering parameters for estimating shaking intensity.

The relationship of P d with PGV by augmenting the 638 P-Alert records obtained during the 5 February 2016 Meinong earthquake and the 6 February 2018 Hualien earthquake with 780 records from Taiwan, Japan, and California via the strong motion instrument used by Wu and Kanamori (2008) [ 24 ].
To check the threshold value of P d in Taiwan, the relation of PGV and P d established by Wu et al. (2005a, 2008) [ 23 , 24 ] is validated using the P-Alert records. The 638 P-Alert records within the epicentral distance of 200 km, obtained during the 5 February 2016 M w 6.4 earthquake in Meinong and the 6 February 2018 M w 6.4 earthquake in Hualien, are augmented with 780 records from Taiwan, Japan, and California, via the strong motion instrument used by Wu and Kanamori (2008) [ 24 ]. By plotting the data recorded by the P-Alert instruments during the two earthquakes in Taiwan, the relationship is the same as the values from P-Alert records that lie within the original data of Wu and Kanamori (2008) [ 24 ], which shows that P-Alert records can be used successfully for P d calculation ( Figure 3 ).
In addition to the on-site warning data used at each station, the data from each field station, received at the central station, is processed continuously for the estimation of threshold parameters ( P d and PGA), as well as the magnitude and other parameters, using Earthworm software [ 4 ]. Figure 4 shows the setup of P-Alert instruments and the networking scheme of each of the field instruments to the central recording station. Each P-Alert instrument is equipped with the industrial protocol for connection and with two relays that it can switch the device on/off during emergency operations for on-site EEW purposes. The central recording station can also turn the instruments installed in the field on/off through the relay. The warning time in the regional warning is a function of data transmission and epicentral distance. Using a denser recording network, the warning time is maximized by recording the earthquakes promptly. For earthquakes occurring outside of or near the edge of the seismic network, a considerable error is reported in earthquake location and magnitude, and subsequently, the warning is delayed. As the P-Alert instruments are installed densely (5–10 km), the location and magnitude errors are minimized for in-land earthquakes. Even for the earthquakes occurring off the coast of Taiwan, the reported error is manageable. As the P-Alert instruments are installed in various elementary schools, the data flow is continuous from field stations.

The concept of the P-Alert networking system. Each P-Alert is equipped with the industrial protocol for connection and with two relays that can turn the device on/off for emergency operation. The central station also can turn remote devices on/off through P-Alert relays.
4. Shakemaps Using P-Alert Network
The NTU network can generate near-real-time shakemaps during earthquakes. A shakemap is a contour demonstrating the PGA, or any other ground-motion parameter distributions, recorded from different strong-motion stations. A seismic network with closely spaced instruments will deliver these shakemaps precisely, as no interpolation is required. Once five P-Alert instruments record a PGA ≥ 1.5 gal, the network starts plotting shakemaps [ 36 ]. These shakemaps are updated at regular intervals after 30 s and are delivered to the intended users, including the National Science and Technology Center for Disaster Reduction (NCDR), for damage assessment and possible rescue operations. The shakemaps are also posted on social media, including Facebook and Twitter. With P-Alert instruments distributed all over the country, the shakemaps produced using this instrumentation offer detailed shaking patterns, which are helpful for assessing the damage pattern. The ability to provide shakemaps and to determine rupture direction using this instrumentation is discussed previously in many studies [ 37 , 38 , 39 , 40 , 41 , 42 , 43 ].
Yang et al. (2021) [ 36 ] upgraded the NTU network to plot additional PGV, CWB Intensity Scale, and S a shakemaps at different periods as value-added products since 2018. The performance of the system with additional shakemaps was checked using the latest recorded earthquakes in the country. With the upgraded system, all shakemaps are now posted on social media after the occurrence of an earthquake. Sometimes, plotted PGV shakemaps have an advantage over PGA shakemaps. For example, Figure 5 shows the plotted PGA and PGV shakemaps during two earthquakes of 2018 and 2019 that occurred in the Hualien region. Mittal et al. (2021) [ 44 ] compared the performance of the P-Alert network using plotted shakemaps for these two earthquakes, which had an almost-similar magnitude ( M w 6.4 and M w 6.2). The performance was checked in terms of shakemaps. The instruments placed in the epicentral region recorded higher PGA values during both events. The 2018 earthquake had a magnitude M L 6.2 ( M L reported by the CWB) and caused destruction; however, the 2019 earthquake that had M L 6.3 did not cause any severe damage. From the analysis of PGV shakemaps, a different pattern was observed as compared to PGA. The higher PGV values (>17 cm/s) were observed during the 2018 earthquake, whereas this higher PGV value was recorded by only one instrument during the 2019 earthquake. The damaged areas (buildings suffering collapse and fatalities) during the 2018 earthquake were in the higher PGV areas. Based on the results, it was concluded that PGV may be a better indicator of damage distribution.

Due to the dense array in operation, real-time shakemaps are plotted. The plotted real-time PGA and PGV Shakemaps for two earthquakes that occurred in the Hualien region.
5. P-Alert Performance during Recent Damaging Events
The NTU network has shown its ability to work as an on-site EEW system and provide near-real-time shakemaps during recent events. Hsieh et al. (2014) [ 29 ] discussed the performance of the P-Alert network during the two earthquakes of 27 March ( M L 6.1) and 2 June ( M L 6.3) 2013 that occurred in central Taiwan. The working of this network during the Meinong earthquake of 5 February 2016 was discussed by Wu et al. (2016) [ 17 ]. The Meinong earthquake was an inland earthquake that occurred in Southern Taiwan and caused more than 117 fatalities. A detailed shakemap was generated by the NTU network within two minutes of the earthquake’s occurrence and the high shaking regions observed in the maps agreed with the damage locations. The individual instrument also provided 4–8 s of on-site warning time before PGA arrival ( Figure 6 ), which is crucial for the locations situated in the blind zone of regional warning. The instruments recorded high PGA values (497 gals) in the epicentral region. Using PGA and PGV shakemaps, it was observed that the highly damaged areas were in high PGV (>17 cm/s) regions.

The on-site method can give warnings in the epicentral region where no regional warning is possible. P-Alert records from two earthquakes in 2016 and 2018 show the same trend as Wu and Kanamori (2008) [ 24 ] in Figure 2 .
The Hualien earthquake of 6 February 2018 caused widespread damage in the eastern part of the country. The earthquake caused strong shaking and severe damage to many buildings in Hualien. Lead-times of 1.5–8 s before the arrival of PGA ( Figure 6 ) were obtained in the blind zone at different locations [ 18 ]. The PGA during this earthquake, recorded by the P-Alert network, reached around 600 gals, equivalent to a maximum intensity of VII. The PGV recorded by the P-Alert network reached around 125 cm/s without any interruptions [ 44 ], showing the robustness of the P-Alert network. By analyzing the P-Alert data of the 2018 Hualien earthquake, it was observed that the data recorded by this instrumentation could be used for surface-wave inversion [ 18 ]. Figure 6 shows the on-site warning time generated by different instruments during the 2016 Meinong earthquake and the 2018 Hualien earthquake. During both earthquakes, a useful lead-time is obtained in the blind zone of the CWB regional warning.
6. Applications of P-Alert Networks
Rupture direction is the key parameter and can cause severe destruction, as the ground motion is amplified because of a piling-up of ground motion from near- and far-end instruments. The dense shakemaps generated using P-Alert instrumentation can be used to assess the rupture direction, which is one of the key factors in studying the damage pattern after an earthquake. Wu et al. (2016) [ 17 ] found that the rupture direction evaluated using shakemaps during the Meinong earthquake of 2016 agreed with aftershock distribution, which is a usual way of assessing rupture direction. The timely information of rupture direction can help save a lot of lives. The rupture direction using shakemaps from this network during the 2018 Hualien earthquake correlated well with aftershock distribution and surface ruptures [ 18 ], which again emphasizes the ability of this instrumentation in estimating rupture direction. Using real-time shakemap interpolation and attenuation regression, Jan et al. (2018) [ 45 ] tested the feasibility of using rupture direction from the near-source P-Alert instruments for delivering a warning to the far areas. They used 16 moderate-to-large earthquakes to infer that directivity can be obtained precisely within 17 s of the occurrence of an earthquake, which in turn is very helpful for EEW. Figure 7 shows the rupture direction evaluated using the recorded PGA at selected stations during the Hualien M w 6.3 earthquake of 2013. From the figure, the rupture direction is northeast–southwest and agrees well with aftershock distribution [ 37 ].

Evaluated fault rupture direction using the recorded waveforms during the 2013 Hualien earthquake. The rupture direction agrees with the aftershock distribution.
The near-real-time detailed shakemaps can identify the direction of the source rupture. Yang et al. (2018) [ 43 ] proposed a nontraditional regional EEW system based on time-dependent anisotropic PGA attenuation relationships that are based on real-time P-Alert signals, named “ShakingAlarm”. This is a ground-motion-driven approach using observed data from the source region to establish time-dependent anisotropic PGA attenuation and accurately predict the PGA for the far region before the arrival of the observed PGA. Figure 8 shows the example of the 2016 Meinong earthquake. The stations outside the epicentral region could have had 5–10 s lead-time between the predicted and the observed PGA. The benefit of the ShakingAlarm approach is that it can reliably predict shaking intensity and avoid false alarms, unlike traditional regional EEW systems. However, it cannot provide as long a lead-time as traditional systems do. This is a trade-off problem with EEW, between reliable information and longer lead-times.

Predicted (solid) and observed (dash) PGA of three P-Alert stations of the 2016 Meinong earthquake. The predicted PGA by the ShakingAlarm approach. Stations W196, W21B, and L004 are 63, 27, and 35 km away from the epicenter, respectively.
Many multi-story buildings in different parts of the world need damage assessments post-earthquakes. The P-Alert instruments have proven efficient in structural health monitoring. Putting a minimum of only three of these instruments can accurately predict the structural health of any building after any earthquake. Hsu et al. (2018) [ 46 ] used P-Alert instruments and conducted several shake-table tests with incremental damage to check the performance of P-Alert for evaluating post-earthquake building safety. They found that acceptable damage detection for an entire building is possible using these instruments. The tests were conducted using three types of instruments. It was found that around 50% of P-Alert instruments were correct in identifying the damage level of each story of a building. However, when they aimed to detect the damage to the whole building, these devices displayed 100% correct results. It was concluded that, although P-Alert systems may be a poor indicator of damage location, they correctly depict the damage to an entire building.
Jan et al. (2017) [ 47 ] used real-time P-Alert data to determine the coseismic deformation ( C d ) in the epicentral region of earthquakes. The finite-fault model, a crucial component in seismic risk mitigation, can be directly derived from the C d values. They compared the P-Alert C d values with the C d values estimated using Global Navigation Satellite System (GNSS) data and strong-motion data from the Taiwan Strong Motion Instrumentation Program (TSMIP), and found that C d values estimated using the P-Alert network provided useful results, especially for earthquakes having PGA > 60 gals. High C d values ( C d > 2 cm) in the epicentral region can help authorities mitigate the damage and act promptly for rescue purposes. High C d values of the order of 60 cm were estimated using the records of the P-Alert network during the 2018 Hualien earthquake and agreed with C d values estimated using GNSS and TSMIP strong-motion data [ 47 ].
7. P-Alert Worldwide
In several countries, such as China, India, Nepal, Bhutan, Philippines, Indonesia, Greece, Vietnam, the Solomon Islands, and New Zealand, the P-Alert instruments are in-demand because of their low cost and their capability to capture and timely report an earthquake for EEW. All these countries have built EEW networks by either installing P-Alert instruments solely or including them in their existing network. The P-Alert instruments are also popular because they act as two-fold sensors, and separate sensors are not required for on-site and regional warning systems. P-Alert instruments are especially helpful for the countries sitting at the plate boundaries and facing seismic hazards from a large portion (India, China, and Indonesia, to name a few). The embedded algorithms in P-Alert and its effectiveness make it unique; thus, around 3500 P-Alert instruments are already installed around the world.
China established a prototype EEW system in the region of the 1976 Tangshan earthquake using 100 P-Alert sensors since 2010. They also tested the functioning of the EEW system in the Sichuan–Yunnan border region using 270 MEMS sensors, including 100 P-Alert sensors. The functioning of this system is reported by Peng et al. (2019) [ 48 ]. India developed its EEW in the Uttarakhand region of the northwest Himalayas by installing 200 P-Alert and P-Alert plus instruments [ 11 ] and is currently in the testing stage. This EEW system is planned to issue warnings to the plains regions, including the national capital city, Delhi, from earthquakes occurring around 250–300 km away. Since its installation, no bigger earthquake has been recorded by the network, for which warnings should be disseminated, so to date, no warnings have been issued. In the absence of data from this network, Mittal et al. (2019b) [ 49 ] tested the functioning of this EEW network by using the data recorded in Taiwan. They transformed the Taiwan P-Alert stations to match with the Indian stations, and recorded earthquakes within the Indian coordinates. The functioning was tested using the Indian velocity model, the global velocity model, and the Taiwan velocity model. Great accuracy in magnitude and earthquake location was reported using the Indian velocity model. Gujrat State, in India, is also planning to install the P-Alert EEW network for Ahmedabad and other big cities due to the earthquakes occurring in the Kachchh region. The feasibility of working on EEW in this region was put forward by Kumar et al. (2020) [ 12 ].
In New Zealand, the P-Alert instruments are distributed by the New Zealand company Jenlogix’s network and are used by several universities, councils, and power companies. The EEW network is specifically designed using these P-Alert instruments.

8. Summary and Recommendations
Due to a limited number of installed strong-motion accelerographs at larger distances, the biggest challenge in strong-motion recording is the spatial resolution. A dense network or enough network coverage is the backbone of an EEW system. Because of insufficient station coverage, the estimated earthquake location is error-prone, which, in turn, may cause problems for EEW in terms of estimating strong shaking for the affected areas. For earthquakes occurring at the edge of the network or outside of the network, the error locations may reach 50 km. The seismic arrays have been functional since the 1960s to monitor earthquake activities and to increase signal-to-noise ratios. Despite the advantage of these seismic arrays over regular networks, these are not common in many countries because of the huge cost of instruments, as the instruments are needed at intervals of very few kilometers. The increased spatial resolution in strong-motion recording and the employment of dense networks can be achieved by employing MEMS sensors.
With increased computation power and internet technology, MEMS sensors have proven useful for EEW and other applications. The applicability of MEMS-based sensors has been explored in various countries for EEW. The Quake-Catcher Network formed a seismological instrumentation network by using various MEMS accelerometers [ 50 ]. Kong et al. (2016) [ 51 ] used real-time MEMS records from smartphones to develop an application called MyShake for EEW. A machine-learning algorithm was used to differentiate between earthquakes and other sources and it was found that estimated earthquake locations and magnitudes were reasonable. Low-cost sensors have demonstrated value in nations, specifically China and India, that confront seismic hazards in a vast region [ 48 ]. Cascone et al. (2021) [ 52 ] tested the performance of a MEMS sensor prototype in Italy, designed by the Italian company ADEL Srl, to monitor small local events.
The MEMS-based P-Alert sensors have proven helpful in placing the sensors closely together and monitoring the seismic activities minutely. These sensors are cost-effective, low-power consuming, and easy to install. The recent version of P-Alert is promising in terms of dynamic range and storage, the two features that were missing in the earlier version of P-Alert. The main development in the P-Alert network is the continuous increase in the number of stations installed throughout the country. The density and coverage of the instruments are enough, except in the eastern part of the country. A continuous endeavor is being considered to find the proper logistics for the installation of these instruments there. Smartphone-based technology may be another option in Taiwan. In addition to EEW, the P-Alert sensors are used to study the directivity effect, structural health monitoring, and various seismological studies. The low cost of these P-Alert devices has attracted various countries to build their EEW network using these low-cost sensors, or by adding them to their existing network to increase density and network coverage. The results obtained using recorded or real-time data are encouraging and have shown their potential in various applications.
Acknowledgments
The authors gratefully acknowledge the Ministry of Science and Technology (MOST), Taiwan, for sponsoring the project, under which this study was carried out. Our work was also supported by the Research Center of Future Earth of the National Taiwan University (NTU). This work was also financially supported by the NTU Research Center for Future Earth from The Featured Areas Research Center Program, within the framework of the Higher Education Sprout Project by the Ministry of Education (MOE) in Taiwan. The GMT software from Wessel and Smith (1998) was used in plotting most of the figures and is gratefully acknowledged. The author (HM) is thankful to the Director of the National Centre for Seismology, Ministry of Earth Sciences, India, for providing the support to participate in this work. The constructive suggestions from two anonymous reviewers and an editor helped in refining the manuscript.
Author Contributions
Conceptualization, Y.-M.W.; methodology, Y.-M.W.; validation, Y.-M.W. and H.M.; formal analysis, Y.-M.W.; investigation, H.M. and Y.-M.W.; resources, Y.-M.W.; data curation, Y.-M.W.; writing—original draft preparation, H.M. and Y.-M.W.; writing—review and editing, H.M. and Y.-M.W.; visualization, Y.-M.W.; supervision, Y.-M.W.; project administration, Y.-M.W.; funding acquisition, Y.-M.W. All authors have read and agreed to the published version of the manuscript.
This research was funded by the Ministry of Science and Technology, Taiwan, grant number: MOST 108-2116-M-002-024-MY3 and MOST 109-2116-M-002-030-MY3.
Institutional Review Board Statement
Not applicable.
Informed Consent Statement
Data availability statement, conflicts of interest.
The authors declare no conflict of interest.
Publisher’s Note: MDPI stays neutral with regard to jurisdictional claims in published maps and institutional affiliations.
Information
- Author Services
Initiatives
You are accessing a machine-readable page. In order to be human-readable, please install an RSS reader.
All articles published by MDPI are made immediately available worldwide under an open access license. No special permission is required to reuse all or part of the article published by MDPI, including figures and tables. For articles published under an open access Creative Common CC BY license, any part of the article may be reused without permission provided that the original article is clearly cited. For more information, please refer to https://www.mdpi.com/openaccess .
Feature papers represent the most advanced research with significant potential for high impact in the field. A Feature Paper should be a substantial original Article that involves several techniques or approaches, provides an outlook for future research directions and describes possible research applications.
Feature papers are submitted upon individual invitation or recommendation by the scientific editors and must receive positive feedback from the reviewers.
Editor’s Choice articles are based on recommendations by the scientific editors of MDPI journals from around the world. Editors select a small number of articles recently published in the journal that they believe will be particularly interesting to readers, or important in the respective research area. The aim is to provide a snapshot of some of the most exciting work published in the various research areas of the journal.
Original Submission Date Received: .
- Active Journals
- Find a Journal
- Proceedings Series
- For Authors
- For Reviewers
- For Editors
- For Librarians
- For Publishers
- For Societies
- For Conference Organizers
- Open Access Policy
- Institutional Open Access Program
- Special Issues Guidelines
- Editorial Process
- Research and Publication Ethics
- Article Processing Charges
- Testimonials
- Preprints.org
- SciProfiles
- Encyclopedia
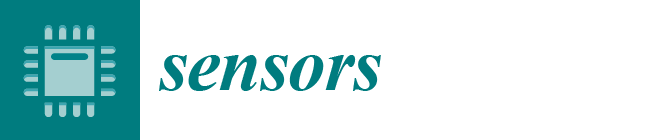
Article Menu
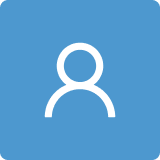
- Subscribe SciFeed
- Recommended Articles
- PubMed/Medline
- Google Scholar
- on Google Scholar
- Table of Contents
Find support for a specific problem in the support section of our website.
Please let us know what you think of our products and services.
Visit our dedicated information section to learn more about MDPI.
JSmol Viewer
Real-time seismic intensity measurements prediction for earthquake early warning: a systematic literature review.
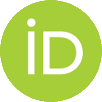
1. Introduction
2. theoretical study on the evolution of earthquake rupture, 3. network-based earthquake early warning, 3.1. source estimation method, 3.2. ground motion model based on m, r, vs30 with shakemap, 3.3. country-specific examples, 4. on-site warning method of earthquake early warning, 4.1. p wave parameters, 4.2. correlation between p wave warning parameters and ground motion model.
- Waveform processing: when an earthquake is detected, remove the mean value and linear trend of the waveform and pick up the P waveform. Calculate the signal-to-noise ratio to eliminate data that may be contaminated by the noise for data quality control;
- P wave parameter calculation: integrate the accelerometer records once and twice to obtain the P v and P d records; filter them with a Butterworth high-pass filter with a cutoff frequency of 0.075 Hz to remove the low-frequency drift after the second integration; and obtain the P d , P v , τ c and other parameters in the 3 s time window after the arrival of the P wave;
- Threshold setting: there is a good correlation between the seismic intensity parameter IMM and peak velocity and the early P wave peak displacement and IM parameter PGV [ 59 ]. By converting the intensity to the PGV, the threshold value of P d is calculated by determining the empirical correlation between the P d and PGV. Similarly, the threshold value of τ c is determined by the correlation between τ c and magnitude. For example, the P d threshold and τ c threshold are set to 0.2 cm and 0.6 s, respectively, for an earthquake with M > 6 and IMM ≥ 7 [ 15 ].
- Issue alert: judge whether the IM parameters exceed the set threshold, calculate the intensity level, determine the warning level and release the warning information.
Click here to enlarge figure
4.3. Ground Motion Model Based on Artificial Intelligence Technology
5. intensity measurements estimation based on finite fault model, intensity measurements estimation based on finite fault template matching, 6. intensity measurements prediction based on simulated seismic wave fields, 6.1. numerical shake prediction for eews, 6.2. intensity measurements prediction based on propagation of local undamped motion, 6.2.1. principle of plum method, 6.2.2. improvement and testing of the plum method.
- Grid definition: each station is connected to its six neighboring stations regardless of spacing. When a station monitors motion above the threshold, it sends its maximum predicted value to the surrounding six stations;
- Intensity modification: the intensity I J M A is changed to I M M I , and I M M I is calculated from the PGA and PGV;
- Dual station triggering algorithm: when both adjacent stations trigger the threshold, the station whose maximum value of ground vibration is triggered satisfies the primary threshold, and the adjacent triggered station meets the secondary threshold to solve the noise-spike false-alarm problem.
7. Intensity Measurements Evaluation Methodology
7.1. evaluation of intensity measurements accuracy based on different algorithms, 7.2. impact of alert costs on the intensity measurements accuracy, 8. discussion, 9. conclusions, author contributions, institutional review board statement, informed consent statement, data availability statement, acknowledgments, conflicts of interest.
- Allen, R.M.; Melgar, D. Earthquake early warning: Advances, scientific challenges, and societal needs. Annu. Rev. Earth Planet. Sci. 2019 , 47 , 361–388. [ Google Scholar ] [ CrossRef ]
- Cremen, G.; Galasso, C. Earthquake early warning: Recent advances and perspectives. Earth Sci. Rev. 2020 , 205 , 103184. [ Google Scholar ] [ CrossRef ]
- Allen, R.M.; Stogaitis, M. Global growth of earthquake early warning. Science 2022 , 375 , 717–718. [ Google Scholar ] [ CrossRef ] [ PubMed ]
- Yamamoto, S.; Tomori, M. Earthquake early warning system for railways and its performance. J. JSCE 2013 , 1 , 322–328. [ Google Scholar ] [ CrossRef ] [ PubMed ]
- Suárez, G. The seismic early warning system of Mexico (SASMEX): A retrospective view and future challenges. Front. Earth Sci. 2022 , 10 , 196. [ Google Scholar ] [ CrossRef ]
- Kodera, Y.; Hayashimoto, N.; Tamaribuchi, K.; Noguchi, K.; Moriwaki, K.; Takahashi, R.; Morimoto, M.; Okamoto, K.; Hoshiba, M. Developments of the nationwide earthquake early warning system in Japan after the 2011 M w 9.0 Tohoku-Oki earthquake. Front. Earth Sci. 2021 , 9 , 726045. [ Google Scholar ] [ CrossRef ]
- Wu, Y.M.; Mittal, H.; Chen, D.-Y.; Hsu, T.Y.; Lin, P.Y. Earthquake early warning systems in Taiwan: Current status. J. Geol. Soc. India 2021 , 97 , 1525–1532. [ Google Scholar ] [ CrossRef ]
- Sheen, D.H.; Park, J.H.; Chi, H.C.; Hwang, E.H.; Lim, I.S.; Seong, Y.J.; Pak, J. The first stage of an earthquake early warning system in South Korea. Seismol. Res. Lett. 2017 , 88 , 1491–1498. [ Google Scholar ] [ CrossRef ]
- Goltz, J.D.; Wald, D.J.; McBride, S.K.; DeGroot, R.; Breeden, J.K.; Bostrom, A. Development of a companion questionnaire for “Did You Feel It?”: Assessing response in earthquakes where an earthquake early warning may have been received. Earthq. Spectra 2023 , 39 , 434–453. [ Google Scholar ] [ CrossRef ]
- Kumar, R.; Mittal, H.; Sharma, B. Earthquake Genesis and Earthquake Early Warning Systems: Challenges and a Way Forward. Surv. Geophys. 2022 , 43 , 1143–1168. [ Google Scholar ] [ CrossRef ]
- Chamoli, B.P.; Kumar, A.; Chen, D.Y.; Gairola, A.; Jakka, R.S.; Pandey, B.; Kumar, P.; Rathore, G. A prototype earthquake early warning system for northern India. J. Earthq. Eng. 2021 , 25 , 2455–2473. [ Google Scholar ] [ CrossRef ]
- Cremen, G.; Galasso, C.; Zuccolo, E. Investigating the potential effectiveness of earthquake early warning across Europe. Nat. Commun. 2022 , 13 , 639. [ Google Scholar ] [ CrossRef ]
- Bracale, M.; Colombelli, S.; Elia, L.; Karakostas, V.; Zollo, A. Design, implementation and testing of a network-based Earthquake Early Warning System in Greece. Front. Earth Sci. 2021 , 9 , 667160. [ Google Scholar ] [ CrossRef ]
- Peng, C.; Jiang, P.; Ma, Q.; Su, J.; Cai, Y.; Zheng, Y. Chinese Nationwide Earthquake Early Warning System and Its Performance in the 2022 Lushan M6.1 Earthquake. Remote Sens. 2022 , 14 , 4269. [ Google Scholar ] [ CrossRef ]
- Zollo, A.; Amoroso, O.; Lancieri, M.; Wu, Y.M.; Kanamori, H. A threshold-based earthquake early warning using dense accelerometer networks. Geophys. J. Int. 2010 , 183 , 963–974. [ Google Scholar ] [ CrossRef ]
- Satriano, C.; Wu, Y.M.; Zollo, A.; Kanamori, H. Earthquake early warning: Concepts, methods and physical grounds. Soil Dyn. Earthq. Eng. 2011 , 31 , 106–118. [ Google Scholar ] [ CrossRef ]
- Hoshiba, M.; Iwakiri, K.; Hayashimoto, N.; Shimoyama, T.; Hirano, K.; Yamada, Y.; Ishigaki, Y.; Kikuta, H. Outline of the 2011 off the Pacific coast of Tohoku earthquake (Mw 9.0)—Earthquake early warning and observed seismic intensity. Earth Planets Space 2011 , 63 , 547–551. [ Google Scholar ] [ CrossRef ]
- Hoshiba, M.; Ozaki, T. Earthquake Early Warning and Tsunami Warning of the Japan Meteorological Agency, and Their Performance in the 2011 off the Pacific Coast of Tohoku Earthquake (9.0). In Early Warning for Geological Disasters: Scientific Methods and Current Practice ; Friedemann, W., Jochen, Z., Eds.; Springer: Berlin/Heidelberg, Germany, 2014; pp. 1–28. ISBN 978-3-642-12233-0. [ Google Scholar ]
- Aoi, S.; Asano, Y.; Kunugi, T.; Kimura, T.; Uehira, K.; Takahashi, N.; Ueda, H.; Shiomi, K.; Matsumoto, T.; Fujiwara, H. MOWLAS: NIED observation network for earthquake, tsunami and volcano. Earth Planets Space 2020 , 72 , 1–31. [ Google Scholar ] [ CrossRef ]
- Böse, M.; Smith, D.E.; Felizardo, C.; Meier, M.A.; Heaton, T.H.; Clinton, J.F. FinDer v.2: Improved real-time ground-motion predictions for M2–M9 with seismic finite-source characterization. Geophys. J. Int. 2018 , 212 , 725–742. [ Google Scholar ] [ CrossRef ]
- Kodera, Y.; Yamada, Y.; Hirano, K.; Tamaribuchi, K.; Adachi, S.; Hayashimoto, N.; Morimoto, M.; Nakamura, M.; Hoshiba, M. The Propagation of Local Undamped Motion (PLUM) Method: A Simple and Robust Seismic Wavefield Estimation Approach for Earthquake Early Warning. Bull. Seismol. Soc. Am. 2018 , 108 , 983–1003. [ Google Scholar ] [ CrossRef ]
- Rafiei, M.H.; Adeli, H. NEEWS: A novel earthquake early warning model using neural dynamic classification and neural dynamic optimization. Soil Dyn. Earthq. Eng. 2017 , 100 , 417–427. [ Google Scholar ] [ CrossRef ]
- Chiang, Y.J.; Chin, T.L.; Chen, D.Y. Neural Network-Based Strong Motion Prediction for On-Site Earthquake Early Warning. Sensors 2022 , 22 , 704. [ Google Scholar ] [ CrossRef ] [ PubMed ]
- Song, J.; Zhu, J.; Wang, Y.; Li, S. On-site alert-level earthquake early warning using machine-learning-based prediction equations. Geophys. J. Int. 2022 , 231 , 786–800. [ Google Scholar ] [ CrossRef ]
- Olson, E.L.; Allen, R.M. The deterministic nature of earthquake rupture. Nature 2005 , 438 , 212–215. [ Google Scholar ] [ CrossRef ] [ PubMed ]
- Böse, M.; Hauksson, E.; Solanki, K.; Kanamori, H.; Heaton, T.H. Real-time testing of the on-site warning algorithm in southern California and its performance during the July 29 2008 Mw5.4 Chino Hills earthquake. Geophys. Res. Lett. 2009 , 36 , L00B03. [ Google Scholar ] [ CrossRef ]
- Colombelli, S.; Zollo, A.; Festa, G.; Picozzi, M. Evidence for a difference in rupture initiation between small and large earthquakes. Nat. Commun. 2014 , 5 , 4958. [ Google Scholar ] [ CrossRef ]
- Colombelli, S.; Festa, G.; Zollo, A. Early rupture signals predict the final earthquake size. Geophys. J. Int. 2020 , 223 , 692–706. [ Google Scholar ] [ CrossRef ]
- Rydelek, P.; Horiuchi, S. Is earthquake rupture deterministic? Nature 2006 , 442 , E5–E6. [ Google Scholar ] [ CrossRef ]
- Meier, M.A.; Heaton, T.; Clinton, J. Evidence for universal earthquake rupture initiation behavior. Geophys. Res. Lett. 2016 , 43 , 7991–7996. [ Google Scholar ] [ CrossRef ]
- Trugman, D.T.; Page, M.T.; Minson, S.E.; Cochran, E.S. Peak Ground Displacement Saturates Exactly When Expected: Implications for Earthquake Early Warning. J. Geophys. Res. Solid Earth 2019 , 124 , 4642–4653. [ Google Scholar ] [ CrossRef ]
- Melgar, D.; Hayes, G.P. Systematic Observations of the Slip Pulse Properties of Large Earthquake Ruptures. Geophys. Res. Lett. 2017 , 44 , 9691–9698. [ Google Scholar ] [ CrossRef ]
- Goldberg, D.E.; Melgar, D.; Bock, Y.; Allen, R.M. Geodetic Observations of Weak Determinism in Rupture Evolution of Large Earthquakes. J. Geophys. Res. Solid Earth 2018 , 123 , 9950–9962. [ Google Scholar ] [ CrossRef ]
- Meier, M.A.; Ampuero, J.; Heaton, T.H. The hidden simplicity of subduction megathrust earthquakes. Science 2017 , 357 , 1277–1281. [ Google Scholar ] [ CrossRef ]
- Zollo, A.; Lancieri, M.; Nielsen, S. Earthquake magnitude estimation from peak amplitudes of very early seismic signals on strong motion records. Geophys. Res. Lett. 2006 , 33 , L23312. [ Google Scholar ] [ CrossRef ]
- Hutchison, A.A.; Böse, M.; Manighetti, I. Improving Early Estimates of Large Earthquake’s Final Fault Lengths and Magnitudes Leveraging Source Fault Structural Maturity Information. Geophys. Res. Lett. 2020 , 47 , e2020GL087539. [ Google Scholar ] [ CrossRef ]
- Melgar, D.; Bock, Y.; Sanchez, D.; Crowell, B.W. On robust and reliable automated baseline corrections for strong motion seismology. J. Geophys. Res. Solid Earth 2013 , 118 , 1177–1187. [ Google Scholar ] [ CrossRef ]
- Wu, Y.M.; Zhao, L. Magnitude estimation using the first three seconds P-wave amplitude in earthquake early warning. Geophys. Res. Lett. 2006 , 33 , e2006GL026871. [ Google Scholar ] [ CrossRef ]
- Nakamura, Y. On the urgent earthquake detection and alarm system (UrEDAS). In Proceedings of the Ninth World Conference on Earthquake Engineering, Tokyo-Kyoto, Japan, 2–9 August 1988; pp. 673–678. [ Google Scholar ]
- Huang, P.L.; Lin, T.L.; Wu, Y.M. Application of τc* Pd in earthquake early warning. Geophys. Res. Lett. 2015 , 42 , 1403–1410. [ Google Scholar ] [ CrossRef ]
- Cua, G.; Heaton, T. The Virtual Seismologist (VS) method: A Bayesian approach to earthquake early warning. In Earthquake Early Warning Systems ; Paolo, G., Gaetano, M., Jochen, Z., Eds.; Springer: Berlin/Heidelberg, Germany, 2007; ISBN 978-3-540-72241-0. [ Google Scholar ]
- Mousavi, S.M.; Ellsworth, W.L.; Zhu, W.; Chuang, L.Y.; Beroza, G.C. Earthquake transformer—An attentive deep-learning model for simultaneous earthquake detection and phase picking. Nat. Commun. 2020 , 11 , 3952. [ Google Scholar ] [ CrossRef ]
- Zhang, X.; Zhang, M.; Tian, X. Real-time earthquake early warning with deep learning: Application to the 2016 M 6.0 Central Apennines, Italy earthquake. Geophys. Res. Lett. 2021 , 48 , e2020GL089394. [ Google Scholar ] [ CrossRef ]
- Chiou, B.; Darragh, R.; Gregor, N.; Silva, W. NGA project strong-motion database. Earthq. Spectra 2008 , 24 , 23–44. [ Google Scholar ] [ CrossRef ]
- Abrahamson, N.; Silva, W. Summary of the Abrahamson & Silva NGA ground-motion relations. Earthq. Spectra 2008 , 24 , 67–97. [ Google Scholar ] [ CrossRef ]
- Goulet, C.A.; Kishida, T.; Ancheta, T.D.; Cramer, C.H.; Darragh, R.B.; Silva, W.J.; Hashash, Y.M.; Harmon, J.; Parker, G.A.; Stewart, J.P.; et al. PEER NGA-east database. Earthq. Spectra 2021 , 37 , 1331–1353. [ Google Scholar ] [ CrossRef ]
- Mazzoni, S.; Kishida, T.; Stewart, J.P.; Contreras, V.; Darragh, R.B.; Ancheta, T.D.; Ancheta, T.D.; Chiou, B.S.; Silva, W.J.; Bozorgnia, Y. Relational database used for ground-motion model development in the NGA-Sub project. Earthq. Spectra 2022 , 38 , 1529–1548. [ Google Scholar ] [ CrossRef ]
- Parker, G.A.; Stewart, J.P.; Boore, D.M.; Atkinson, G.M.; Hassani, B. NGA-subduction global ground motion models with regional adjustment factors. Earthq. Spectra 2022 , 38 , 456–493. [ Google Scholar ] [ CrossRef ]
- Kuyuk, H.S.; Allen, R.M. Optimal seismic network density for earthquake early warning: A case study from California. Seismol. Res. Lett. 2013 , 84 , 946–954. [ Google Scholar ] [ CrossRef ]
- Kuyuk, H.S.; Allen, R.M.; Brown, H.; Hellweg, M.; Henson, I.; Neuhauser, D. Designing a network-based earthquake early warning algorithm for California: ElarmS-2. Bull. Seismol. Soc. Am. 2014 , 104 , 162–173. [ Google Scholar ] [ CrossRef ]
- Chung, A.I.; Henson, I.; Allen, R.M. Optimizing Earthquake Early Warning Performance: ElarmS-3. Seismol. Res. Lett. 2019 , 90 , 727–743. [ Google Scholar ] [ CrossRef ]
- Thakoor, K.; Andrews, J.; Hauksson, E.; Heaton, T. From earthquake source parameters to ground-motion warnings near you: The ShakeAlert earthquake information to ground-motion (eqInfo2GM) method. Seismol. Res. Lett. 2019 , 90 , 1243–1257. [ Google Scholar ] [ CrossRef ]
- Caruso, A.; Colombelli, S.; Elia, L.; Picozzi, M.; Zollo, A. An on-site alert level early warning system for Italy. J. Geophys. Res. Solid Earth 2017 , 122 , 2106–2118. [ Google Scholar ] [ CrossRef ]
- Wurman, G.; Allen, R.M.; Lombard, P. Toward earthquake early warning in northern California. Geophys. Res. Solid Earth 2007 , 112 , B08311. [ Google Scholar ] [ CrossRef ]
- Odaka, T.; Ashiya, K.; Tsukada, S.Y.; Sato, S.; Ohtake, K.; Nozaka, D. A new method of quickly estimating epicentral distance and magnitude from a single seismic record. Bull. Seismol. Soc. Am. 2003 , 93 , 526–532. [ Google Scholar ] [ CrossRef ]
- Festa, G.; Zollo, A.; Lancieri, M. Earthquake magnitude estimation from early radiated energy. Geophys. Res. Lett. 2008 , 35 , L22307. [ Google Scholar ] [ CrossRef ]
- Alcik, H.; Ozel, O.; Apaydin, N.; Erdik, M. A study on warning algorithms for Istanbul earthquake early warning system. Geophys. Res. Lett. 2009 , 36 , L00B05. [ Google Scholar ] [ CrossRef ]
- Wang, Z.; Zhao, B. A new M w estimation parameter for use in earthquake early warning systems. J. Seismol. 2018 , 22 , 325–335. [ Google Scholar ] [ CrossRef ]
- Wu, Y.M.; Kanamori, H. Rapid assessment of damage potential of earthquakes in Taiwan from the beginning of P waves. Bull. Seismol. Soc. Am. 2005 , 95 , 1181–1185. [ Google Scholar ] [ CrossRef ]
- Peng, C.; Yang, J.; Chen, Y.; Zhu, X.; Xu, Z.; Zheng, Y.; Jiang, X. Application of a Threshold-Based Earthquake Early Warning Method to the Mw 6.6 Lushan Earthquake, Sichuan, China. Seismol. Res. Lett. 2015 , 86 , 841–847. [ Google Scholar ] [ CrossRef ]
- Colombelli, S.; Zollo, A. Fast determination of earthquake magnitude and fault extent from real-timeP-wave recordings. Geophys. J. Int. 2015 , 202 , 1158–1163. [ Google Scholar ] [ CrossRef ]
- Song, J.; Li, S.; Wang, Y.; Bai, L. Application of a threshold-based earthquake early warning to ltaly Mw 6.2 earthquake on 24 August 2016. Earthq. Eng. Eng. dyn. 2017 , 37 , 15–22. (In Chinese) [ Google Scholar ]
- Wang, Y.; Li, S.; Song, J. Threshold-based evolutionary magnitude estimation for an earthquake early warning system in the Sichuan-Yunnan region, China. Sci. Rep. 2020 , 10 , 1–12. [ Google Scholar ] [ CrossRef ]
- Colombelli, S.; Caruso, A.; Zollo, A.; Festa, G.; Kanamori, H. A P wave-based, on-site method for earthquake early warning. Geophys. Res. Lett. 2015 , 42 , 1390–1398. [ Google Scholar ] [ CrossRef ]
- Meier, M.A.; Heaton, T.; Clinton, J. The Gutenberg algorithm: Evolutionary Bayesian magnitude estimates for earthquake early warning with a filter bank. Bull. Seismol. Soc. Am. 2015 , 105 , 2774–2786. [ Google Scholar ] [ CrossRef ]
- Peng, C.; Jiang, P.; Ma, Q.; Wu, P.; Su, J.; Zheng, Y.; Yang, J. Performance Evaluation of an Earthquake Early Warning System in the 2019–2020 M6.0 Changning, Sichuan, China, Seismic Sequence. Front. Earth Sci. 2021 , 9 , 699941. [ Google Scholar ] [ CrossRef ]
- Wang, Z.; Zhao, B. Applicability of Accurate Ground Motion Estimation Using Initial P Wave for Earthquake Early Warning. Front. Earth Sci. 2021 , 9 , 718216. [ Google Scholar ] [ CrossRef ]
- Hsu, T.Y.; Huang, S.K.; Chang, Y.W.; Kuo, C.H.; Lin, C.M.; Chang, T.M.; Wen, K.L.; Loh, C.H. Rapid on-site peak ground acceleration estimation based on support vector regression and P-wave features in Taiwan. Soil Dyn. Earthq. Eng. 2013 , 49 , 210–217. [ Google Scholar ] [ CrossRef ]
- Song, J.; Yu, C.; Li, S. Continuous prediction of onsite PGV for earthquake early warning basedon least squares support vector machine. Chin. J. Geophys. 2021 , 64 , 555–568. [ Google Scholar ] [ CrossRef ]
- Hsu, T.Y.; Wu, R.T.; Liang, C.W.; Kuo, C.H.; Lin, C.M. Peak ground acceleration estimation using P-wave parameters and horizontal-to-vertical spectral ratios. Terrest. Atmos. Ocean. Sci. 2020 , 31 , 1–8. [ Google Scholar ] [ CrossRef ]
- Hsu, T.Y.; Huang, C.W. Onsite Early Prediction of PGA Using CNN With Multi-Scale and Multi-Domain P-Waves as Input. Front. Earth Sci. 2021 , 9 , 626908. [ Google Scholar ] [ CrossRef ]
- Jozinović, D.; Lomax, A.; Štajduhar, I.; Michelini, A. Rapid prediction of earthquake ground shaking intensity using raw waveform data and a convolutional neural network. Geophys. J. Int. 2020 , 222 , 1379–1389. [ Google Scholar ] [ CrossRef ]
- Jozinović, D.; Lomax, A.; Štajduhar, I.; Michelini, A. Transfer learning: Improving neural network based prediction of earthquake ground shaking for an area with insufficient training data. Geophys. J. Int. 2022 , 229 , 704–718. [ Google Scholar ] [ CrossRef ]
- Brown, H.M.; Allen, R.M.; Grasso, V.F. Testing elarms in Japan. Seismol. Res. Lett. 2009 , 80 , 727–739. [ Google Scholar ] [ CrossRef ]
- Kurahashi, S.; Irikura, K. Source model for generating strong ground motions during the 2011 off the Pacific coast of Tohoku Earthquake. Earth Planets Space 2011 , 63 , 571–576. [ Google Scholar ] [ CrossRef ]
- Böse, M.; Heaton, T.H.; Hauksson, E. Real-time finite fault rupture detector (FinDer) for large earthquakes. Geophys. J. Int. 2012 , 191 , 803–812. [ Google Scholar ] [ CrossRef ]
- Lu, J.; Li, S. Detailed analysis and preliminary performance evaluation of the FinDer: A real-time finite faultrupture detector for earthquake early warning. World Earthq. Eng. 2021 , 37 , 152–164. (In Chinese) [ Google Scholar ]
- Li, J.; Böse, M.; Feng, Y.; Yang, C. Real-Time Characterization of Finite Rupture and Its Implication for Earthquake Early Warning: Application of FinDer to Existing and Planned Stations in Southwest China. Front. Earth Sci. 2021 , 9 , 699560. [ Google Scholar ] [ CrossRef ]
- Böse, M.; Felizardo, C.; Heaton, T.H. Finite-Fault Rupture Detector (FinDer): Going Real-Time in Californian ShakeAlert Warning System. Seismol. Res. Lett. 2015 , 86 , 1692–1704. [ Google Scholar ] [ CrossRef ]
- Böse, M.; Hutchison, A.A.; Manighetti, I.; Li, J.; Massin, F.; Clinton, J.F. FinDerS(+): Real-Time Earthquake Slip Profiles and Magnitudes Estimated from Back projected Displacement with Consideration of Fault Source Maturity Gradient. Front. Earth Sci. 2021 , 9 , 685879. [ Google Scholar ] [ CrossRef ]
- Hoshiba, M. Real-time prediction of impending ground shaking: Review of wavefield-based (Ground-Motion-Based) method for earthquake early warning. Front. Earth. Sci. 2021 , 9 , 722784. [ Google Scholar ] [ CrossRef ]
- Hoshiba, M. Real-time prediction of ground motion by Kirchhoff-Fresnel boundary integral equation method: Extended front detection method for Earthquake Early Warning. J. Geophys. Res. Solid Earth 2013 , 118 , 1038–1050. [ Google Scholar ] [ CrossRef ]
- Hoshiba, M.; Aoki, S. Numerical Shake Prediction for Earthquake Early Warning: Data Assimilation, Real-Time Shake Mapping, and Simulation of Wave Propagation. Bull. Seismol. Soc. Am. 2015 , 105 , 324–1338. [ Google Scholar ] [ CrossRef ]
- Ogiso, M.; Hoshiba, M.; Shito, A.; Matsumoto, S. Numerical Shake Prediction for Earthquake Early Warning Incorporating Heterogeneous Attenuation Structure: The Case of the 2016 Kumamoto Earthquake. Bull. Seismol. Soc. Am. 2018 , 108 , 3457–3468. [ Google Scholar ] [ CrossRef ]
- Kagawa, T. Application of the Modified PLUM Method to a Dense Seismic Intensity Network of a Local Government in Japan: A Case Study on Tottori Prefecture. Front. Earth Sci. 2021 , 9 , 672613. [ Google Scholar ] [ CrossRef ]
- Kodera, Y. An Earthquake Early Warning Method Based on Huygens Principle: Robust Ground Motion Prediction Using Various Localized Distance-Attenuation Models. J. Geophys. Res. Solid Earth 2019 , 124 , 12981–12996. [ Google Scholar ] [ CrossRef ]
- Kodera, Y.; Saitou, J.; Hayashimoto, N.; Adachi, S.; Morimoto, M.; Nishimae, Y.; Hoshiba, M. Earthquake early warning for the 2016 Kumamoto earthquake: Performance evaluation of the current system and the next-generation methods of the Japan Meteorological Agency. Earth Planets Space 2016 , 68 , 1–14. [ Google Scholar ] [ CrossRef ]
- Kodera, Y.; Hayashimoto, N.; Moriwaki, K.; Noguchi, K.; Saito, J.; Akutagawa, J.; Adachi, S.; Morimoto, M.; Okamoto, K.; Honda, S.; et al. First-Year Performance of a Nationwide Earthquake Early Warning System Using a Wavefield-Based Ground-Motion Prediction Algorithm in Japan. Seismol. Res. Lett. 2020 , 91 , 826–834. [ Google Scholar ] [ CrossRef ]
- Cochran, E.S.; Bunn, J.; Minson, S.E.; Baltay, A.S.; Kilb, D.L.; Kodera, Y.; Hoshiba, M. Event Detection Performance of the PLUM Earthquake Early Warning Algorithm in Southern California. Bull. Seismol. Soc. Am. 2019 , 109 , 1524–1541. [ Google Scholar ] [ CrossRef ]
- Minson, S.E.; Saunders, J.K.; Bunn, J.J.; Cochran, E.S.; Baltay, A.S.; Kilb, D.L.; Hoshiba, M.; Kodera, Y. Real-Time Performance of the PLUM Earthquake Early Warning Method during the 2019 M 6.4 and 7.1 Ridgecrest, California, Earthquakes. Bull. Seismol. Soc. Am. 2020 , 110 , 1887–1903. [ Google Scholar ] [ CrossRef ]
- Kilb, D.; Bunn, J.J.; Saunders, J.K.; Cochran, E.S.; Minson, S.E.; Baltay, A.; O’Rourke, C.T.; Hoshiba, M.; Kodera, Y. The PLUM Earthquake Early Warning Algorithm: A Retrospective Case Study of West Coast, USA, Data. J. Geophys. Res. Solid Earth 2021 , 126 , e2020JB021053. [ Google Scholar ] [ CrossRef ]
- Cochran, E.S.; Saunders, J.K.; Minson, S.E.; Bunn, J.; Baltay, A.; Kilb, D.; O’Rourke, C.; Hoshiba, M.; Kodera, Y. Alert Optimization of the PLUM Earthquake Early Warning Algorithm for the Western United States. Bull. Seismol. Soc. Am. 2022 , 112 , 803–819. [ Google Scholar ] [ CrossRef ]
- Cochran, E.S.; Kohler, M.D.; Given, D.D.; Guiwits, S.; Andrews, J.; Meier, M.A.; Ahmad, M.; Henson, I.; Hartog, R.; Smith, D. Earthquake Early Warning ShakeAlert System: Testing and Certification Platform. Seismol. Res. Lett. 2017 , 89 , 108–117. [ Google Scholar ] [ CrossRef ]
- Böse, M.; Heaton, T.; Hauksson, E. Rapid Estimation of Earthquake Source and Ground-Motion Parameters for Earthquake Early Warning Using Data from a Single Three-Component Broadband or Strong-Motion Sensor. Bull. Seismol. Soc. Am. 2012 , 102 , 738–750. [ Google Scholar ] [ CrossRef ]
- Meier, M.-A. How “good” are real-time ground motion predictions from Earthquake Early Warning systems? J. Geophys. Res. Solid Earth 2017 , 122 , 5561–5577. [ Google Scholar ] [ CrossRef ]
- Meier, M.A.; Kodera, Y.; Böse, M.; Chung, A.; Hoshiba, M.; Cochran, E.; Minson, S.; Hauksson, E.; Heaton, T. How Often Can Earthquake Early Warning Systems Alert Sites With High-Intensity Ground Motion? J. Geophys. Res. Solid Earth 2020 , 125 , e2019JB017718. [ Google Scholar ] [ CrossRef ]
- Minson, S.E.; Meier, M.A.; Baltay, A.S.; Hanks, T.C.; Cochran, E.S.J.S.A. The limits of earthquake early warning: Timeliness of ground motion estimates. Sci. Adv. 2018 , 4 , eaaq0504. [ Google Scholar ] [ CrossRef ]
- Minson, S.E.; Baltay, A.S.; Cochran, E.S.; Hanks, T.C.; Page, M.T.; McBride, S.K.; Milner, K.R.; Meier, M.A. The Limits of Earthquake Early Warning Accuracy and Best Alerting Strategy. Sci. Rep. 2019 , 9 , 2478. [ Google Scholar ] [ CrossRef ]
- Minson, S.E.; Cochran, E.S.; Wu, S.; Noda, S. A framework for evaluating earthquake early warning for an infrastructure network: An idealized case study of a northern California rail system. Front. Earth Sci. 2021 , 9 , 620467. [ Google Scholar ] [ CrossRef ]
- Wu, Y.M.; Mittal, H. A Review on the Development of Earthquake Warning System Using Low-Cost Sensors in Taiwan. Sensors 2021 , 21 , 7649. [ Google Scholar ] [ CrossRef ]
- Mittal, H.; Yang, B.M.; Tseng, T.L.; Wu, Y.M. Importance of real-time PGV in terms of lead-time and shakemaps: Results using 2018 ML 6.2 & 2019 ML 6.3 Hualien, Taiwan earthquakes. J. Asian Earth Sci. 2021 , 220 , 104936. [ Google Scholar ] [ CrossRef ]
- Chen, M.; Peng, C.; Cheng, Z. Earthquake event recognition on smartphones based on neural network models. Sensors 2022 , 22 , 8769. [ Google Scholar ] [ CrossRef ]
- Finazzi, F. The earthquake network project: Toward a crowdsourced smartphone-based earthquake early warning system. Bull. Seismol. Soc. Am. 2016 , 106 , 1088–1099. [ Google Scholar ] [ CrossRef ]
- Colombelli, S.; Carotenuto, F.; Elia, L.; Zollo, A. Design and implementation of a mobile device app for network-based earthquake early warning systems (EEWSs): Application to the PRESTo EEWS in southern Italy. Nat. Hazards Earth Syst. Sci. 2020 , 20 , 921–931. [ Google Scholar ] [ CrossRef ]
- Kong, Q.; Allen, R.M.; Schreier, L.; Kwon, Y.W. MyShake: A smartphone seismic network for earthquake early warning and beyond. Sci. Adv. 2016 , 2 , e1501055. [ Google Scholar ] [ CrossRef ] [ PubMed ]
- Esposito, M.; Palma, L.; Belli, A.; Sabbatini, L.; Pierleoni, P. Recent advances in internet of things solutions for early warning systems: A review. Sensors 2022 , 22 , 2124. [ Google Scholar ] [ CrossRef ] [ PubMed ]
- Alphonsa, A.; Ravi, G. Earthquake early warning system by IOT using Wireless sensor networks. In Proceedings of the 2016 IEEE International Conference on Wireless Communications, Signal Processing and Networking, WiSPNET 2016, Chennai, India, 23–25 March 2016; pp. 1201–1205. [ Google Scholar ]
- Wang, C.Y.; Huang, T.C.; Wu, Y.M. Using LSTM Neural Networks for Onsite Earthquake Early Warning. Seismol. Res. Lett. 2022 , 93 , 814–826. [ Google Scholar ] [ CrossRef ]
- Li, Z.; Meier, M.A.; Hauksson, E.; Zhan, Z.; Andrews, J. Machine learning seismic wave discrimination: Application to earthquake early warning. Geophys. Res. Lett. 2018 , 45 , 4773–4779. [ Google Scholar ] [ CrossRef ]
Article | Category | Research Methods | Opinions |
---|---|---|---|
[ ] | deterministic assumptions | Using extensive global seismic data, measured the period of seismic waves and calculated the scalar relationship between τ and Mw on a log–linear scale. | Information on the final magnitude of the earthquake was available within the first few seconds of the earthquake source rupture. |
[ ] | deterministic assumptions | The relationship that earthquake rupture initiation behavior has with earthquake magnitude was investigated using the early strong motion records of the near-source P and S signals, which demonstrated a statistically significant scale. | At the early stage of earthquake rupture, there was a proportional relationship between stress drop and/or active slip surface and seismic moment. |
[ ] | deterministic assumptions | Analyzed a high-quality seismic database to measure peak displacement (P ) amplitudes with progressively expanding time windows. | The evolution of P with time was related to the early stages of the rupture process and could be used as an indicator of the final size of the rupture. |
[ ] | deterministic assumptions | The early P wave signals of earthquakes of different magnitudes were analyzed, and an amplitude parameter quantifying the initial peak amplitude was introduced to explore the possible differences in their early rupture. | Small and large earthquakes rupture at different initiation stages, and the final rupture extent of the seismic event was statistically controlled by its initial behavior. |
[ ] | no correlation assumption | Studied the proportional relationship between τ and Mw, as well as the effect of this relationship on whether the earthquake rupture was deterministic. | No evidence that the earthquake magnitude could be estimated before the rupture had been completed. |
[ ] | weak deterministic assumptions | Using a large amount of seismic data, examined how peak absolute vertical displacements evolve over time for different magnitudes. | Small and large ruptures started in indistinguishable ways. |
[ ] | weak deterministic assumptions | Before the arrival of the S wave, the vertical component P measured in the time window was gradually extended and a linear relationship was assumed between log10 (P ) and the Mw. | The evolution of P over time suggested a general initial growth pattern that was inconsistent with deterministic models of earthquake rupture. |
[ ] | weak deterministic assumptions | From a finite fault model database of strong seismic events of magnitude Mw 7.0–9.0, the average rise time and rupture speeds of each seismic event were analyzed. | They proposed weak determinism, which held that the magnitude of an earthquake could be predicted after it had been nucleated for some time. |
[ ] | weak deterministic assumptions | Seismic and geodetic data were used to study early rupture indicators to determine if the observations supported deterministic rupture behavior. | Although the initial few seconds were not sufficient to infer the final earthquake magnitude, an accurate estimate could be made before the rupture was complete, which indicated a weak certainty. |
[ ] | weak deterministic assumptions | The typical temporal rupture behavior of large shallow subduction zone earthquakes was studied using three extensive source–time function catalogs. | The final magnitude could not be accurately predicted until the rupture had developed to a certain size. |
Reference | Methods | Research Methods | Method Performance |
---|---|---|---|
[ ] | Boundary integral equation | A simple wavefield estimation method that predicted earthquake intensity directly from the real-time seismic intensity observed near the target location. | The method was computationally inexpensive, overcame some disadvantages in terms of point sources and was a powerful method for wavefield estimation that could improve the performance of EEWS. |
[ ] | Boundary integral equation | Based on Huygens’ principle and the Kirchhoff–Fresnel boundary integral equation, the prediction of subsequent wave fields directly from the observed seismic wave field was proposed. | The method compensated for the shortcomings of the PSA but required a dense observation network; additionally, the warning time was short. |
[ ] | Radiative transfer theory | A method was proposed to accurately estimate the current wavefield distribution in real time using data assimilation techniques, and then the time evolution of future wavefields was predicted through seismic wave propagation simulations. | The method might mostly reflect the current actual observations, and the assimilation technique minimized the difference between the estimated current state and the actual observations. |
[ ] | Radiative transfer theory | The path term was incorporated into the numerical shake prediction scheme to predict future wave fields with heterogeneous attenuation structures. | Careful treatment of heterogeneous attenuation structures in numerical shake prediction could help improve ground motion forecasts, especially those with long lead times. |
[ ] | Radiative transfer theory | A modified Propagation of Local Undamped Motion (PLUM) was proposed by introducing an attenuation factor to the wave propagation. | Improved accuracy and rapidity of seismic intensity distribution compared to the original method. |
[ ] | Radiative transfer theory | The ALPHA algorithm was proposed; it is based on the Huygens principle, assumes multiple point source models below each observatory and establishes various attenuation relationships to predict intensity. | Compared to existing algorithms, ALPHA enables EEWS to provide accurate warnings to a wider area at an earlier stage. |
Alarm Category | Abbreviations | Description |
---|---|---|
True Positive | TP | GM exceeds the threshold and alerts before it arrives |
False Positive | FP | GM does not exceed the threshold, but the alarm is issued |
True Negatives | TN | GM arrives without exceeding the threshold, and no alarm is issued |
False Negative | FN | GM is above the threshold, but no alarm is issued |
The statements, opinions and data contained in all publications are solely those of the individual author(s) and contributor(s) and not of MDPI and/or the editor(s). MDPI and/or the editor(s) disclaim responsibility for any injury to people or property resulting from any ideas, methods, instructions or products referred to in the content. |
Share and Cite
Cheng, Z.; Peng, C.; Chen, M. Real-Time Seismic Intensity Measurements Prediction for Earthquake Early Warning: A Systematic Literature Review. Sensors 2023 , 23 , 5052. https://doi.org/10.3390/s23115052
Cheng Z, Peng C, Chen M. Real-Time Seismic Intensity Measurements Prediction for Earthquake Early Warning: A Systematic Literature Review. Sensors . 2023; 23(11):5052. https://doi.org/10.3390/s23115052
Cheng, Zhenpeng, Chaoyong Peng, and Meirong Chen. 2023. "Real-Time Seismic Intensity Measurements Prediction for Earthquake Early Warning: A Systematic Literature Review" Sensors 23, no. 11: 5052. https://doi.org/10.3390/s23115052
Article Metrics
Article access statistics, further information, mdpi initiatives, follow mdpi.
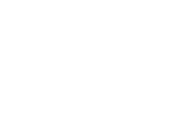
Subscribe to receive issue release notifications and newsletters from MDPI journals
ORIGINAL RESEARCH article
Feasibility study of an earthquake early warning system in eastern central italy.
- 1 Istituto Nazionale di Geofisica e Vulcanologia, Osservatorio Nazionale Terremoti, Ancona, Italy
- 2 Istituto Nazionale di Geofisica e Vulcanologia, Osservatorio Nazionale Terremoti, Roma, Italy
An earthquake early warning system (EEWS) is a monitoring infrastructure that allows alerting strategic points (targets) before the arrival of strong shaking waves during an earthquake. In a region like Central Italy, struck by recent and historical destructive earthquakes, the assessment of implementation of an EEWS is a significant challenge due to the proximity of seismic sources to many potential targets, such as historical towns, industrial plants, and hospitals. In order to understand the feasibility of an EEWS in such an area, we developed an original method of event declaration simulation (EDS), a tool for assessing the effectiveness of an EEWS for existing seismic networks, improving them with new stations, and designing new networks for EEW applications. Values of the time first alert (TFA), blind zone radius (BZ), and lead time (LT) have been estimated with respect to selected targets for different network configurations in the study region. Starting from virtual sources homogeneously arranged on regular mesh grids, the alert response was evaluated for actual and improved seismic networks operating in the area, taking into account the effects of the transmission and acquisition systems. In the procedure, the arrival times of the P wave picks, the association binder, the transmission latencies, and the computation times were used to simulate the configuration of PRESTo EEWS, simulating both real-time and playback elaborations of real earthquakes. The NLLOC software was used to estimate P and S arrival times, with a local velocity model also implemented in the PRESTo EEWS. Our results show that, although Italy’s main seismic sources are located close to urban areas, the lead times calculated with the EDS procedure, applied to actual and to improved seismic networks, encourage the implementation of EEWS in the study area. Considering actual delays due to data transmission and computation time, lead times of 5–10 s were obtained simulating real historical events striking some important targets of the region. We conclude that EEWSs are useful tools that can contribute to protecting people from the harmful effects of earthquakes in Italy.
1 Introduction
In the past twenty years, EEWSs have been implemented in different regions of the world and are considered a useful tool to reduce seismic risk ( Satriano et al., 2011b ). EEWSs were developed with different approaches, methodologies, and combining new experiences. At present, many countries have operational or prototype EEWSs. Allen et al. (2009b) described the status of EEW in the world and the principal operating systems at that time. Other examples include EEWS in Japan ( Odaka et al., 2003 ), Taiwan ( Wu and Teng, 2002 ; Hsiao et al., 2009 ), Mexico ( Suarez et al., 2009 ), Turkey ( Erdik et al., 2003 ; Alcik et al., 2009 ), and Romania ( Böse et al., 2007 ). The principal active systems are based on the software ElarmS ( Allen and Kanamori, 2003 ; Allen et al., 2009a ) and ShakeAlert ( Kohler et al., 2020 ) in California, on Virtual Seismologist in California and Switzerland ( Cua et al., 2009 ), in Europe ( Clinton et al., 2016 ), and in particular PRESTo in Italy ( Iannaccone et al., 2010 ; Satriano et al., 2011a ).
Major developments have led to two main types of systems: a regional alert system and an on-site system ( Satriano et al., 2011b ; Zollo et al., 2014 ). The regional system, based on the use of a regional network that records seismic events, aims to detect, locate, and determine the magnitude of an event starting from the analysis of a few seconds of the first arrivals of the P waves recorded at the stations ( Picozzi, 2012 ). The on-site system consists of a single sensor or more sensors near or inside the target structure to be alerted. In this system the P-wave recordings to the sensor are used to predict the peak ground motion at the site ( Colombelli et al., 2015 ). This approach could be considered useful for sites located within the BZ of a regional EEW system, allowing for a useful warning before the arrival of strong shaking waves. Caruso et al. (2017) proposed a P-wave-based EEW approach called on-site alert level (SAVE). Many studies combined the two EEW approaches ( Zollo et al., 2010 ; Colombelli et al., 2012a ); these systems combine local parameters and predicted ground motions at a regional scale to provide reliable and rapid estimates of the seismic source and the expected damage zone ( Colombelli et al., 2015 ).
The approaches for regional EEW can be classified as the “point-source” (simply the source as a concentrated volume) or “finite fault” (a more sophisticated and realistic characterization of the source, considering the entire fault area). Most studies have used the “point-source” demonstrating the reliability of this approach for the magnitude estimation of small to moderate events. However, it has been shown that this approach is not always accurate for strong earthquakes (magnitude> 6.5–7), due to the saturation of the P-wave parameters. Several authors (for example, Colombelli et al., 2012b ) estimated the magnitude over time windows longer than the recorded P-wave and/or the S-wave signal to obtain more accurate final values. These magnitude calculations are reliable at the cost of requiring more data and time ( Velazquez et al., 2020 ). In our study, the selected earthquakes have a moderate magnitude (≤ 6.5) and were considered as point sources.
Potentially, an EEWS can produce and transmit alert messages to different end-users to allow them to adopt several types of safety measures in a few seconds. The main benefits of an EEWS include public warning, first responder mobilization, and safety of health care and utility infrastructures, transit systems, and workplaces ( Allen and Melgar, 2019 ). Whereas in most cases evacuation of buildings is unrealistic, due to the short time available to act; a portion of the affected population can receive the alert and take safety measures in certain types of structures and infrastructures ( Iervolino et al., 2008 ).
Receiving an alert message increases personal situational awareness and yields a more rapid response, especially in well-trained people who can take precautionary and protective actions like “Drop-Cover-Hold on”, suspending delicate medical procedures, or slowing down a train ride. In shaking areas, a time of 10 s allows people to protect themselves and prepare for evacuation ( Fujinawa and Noda, 2013 ). A time interval of 5–7 s could be enough to trigger automatic mitigation actions ( Cauzzi et al., 2016 ) at power plants, energy sector grids, and utilities infrastructures to prevent explosions, combustions, loss of water, flooding, fatal collisions, and elevator interruptions. Social studies have demonstrated that receiving alert messages even a few seconds before the shaking occurs help people to prepare and react in the proper way ( Dunn et al., 2016 ; Becker et al., 2020a ).
The elongated shape of the Italian peninsula, combined with the small damage area for moderate, but often destructing Apenninic earthquakes (M6-7), determine small distances between sources and potential EEW targets. For this reason, in many cases the time to start safety actions may be too short. Therefore, an evaluation of the feasibility of an EEW implementation is needed in this area. A first theoretical evaluation was performed by Olivieri et al. (2008) with RSN (National Seismic Network, IV, INGV Seismological Data Center, 2006) and by Picozzi et al. (2015) using the RAN seismic network (Italian strong motion network) managed by the National Civil Protection ( Gorini et al., 2010 ), whose stations are mostly not connected in real-time.
Our study area extends for about 200 × 200 km in eastern central Italy and is characterized by the following two main seismic zones: 1) a NNW-SSE seismic zone elongated in the Appennines, where several moderate to strong earthquakes have occurred in the past and 2) a coastline-offshore seismic zone ( Figure 1a ), with less frequent and on average weaker seismicity. Figure 1b shows the target points chosen in the study compared to the individual and composite seismogenic sources from the DISS catalog ( DISS Working Group, 2018 ) . An individual seismogenic source (ISS) is a simplified, three-dimensional representation of a rectangular fault plane, whereas a composite seismogenic source (CSS) is a simplified, three-dimensional representation of a crustal fault containing an unspecified number of seismogenic sources that cannot be singled out. The area analyzed in our study is affected by different fault systems. We select as targets the cities with at least 40,000 inhabitants or with a significant cultural value. The selected cities are Ancona, Pesaro, Macerata, Ascoli Piceno, Fermo, Fabriano, Urbino, San Benedetto del Tronto, Civitanova Marche, Senigallia, Jesi, Perugia, Foligno, and Terni ( Table 1 ).
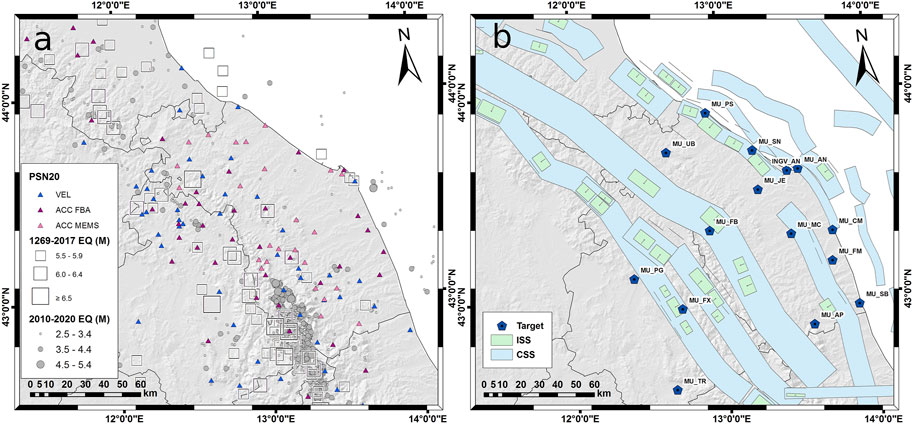
FIGURE 1 . Seismicity, seismic network, different fault zones, and selected targets of the study area. a) PSN20: Permanent Seismic Network in the year 2020. Blue triangles: velocimetric sensors. Purple triangles: FBA high performance accelerometer sensors. Pink triangles: MEMS accelerometer. Black squares: 1219–2019 EQ, moderate to strong earthquakes (M ≥ 5.5) extracted from CPTI15 database ( Rovida et al., 2021 ). Grey circles: earthquakes recorded by the seismic network from 2010 to 2020 in the magnitude range 2.5 ≤ M ≤ 5.4. b) Pentagon: Targets. ISS: individual seismogenic source. CSS: composite seismogenic source (see text for explanation, DISS Working Group (2018)) .
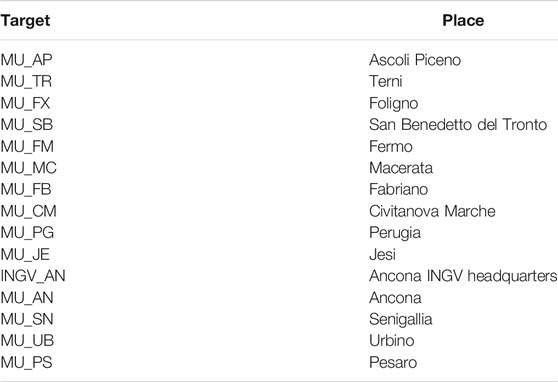
TABLE 1 . Target: city code. Place: extended name of cities.
In the study area, an EEWS based on PRESTo software has been operating since 2015. The system was based on permanent seismic networks managed by the INGV (National Institute of Geophysics and Vulcanology) composed by different sensors: velocimeters (short period and broad-band), high performance accelerometers, and MEMS. The seismic network in this area includes the RSN, a more dense local network (namely, the Alto Tiberina Near Fault Observatory—Taboo ( Chiaraluce et al., 2014 )), and some seismic stations installed for regional monitoring ( Cattaneo et al., 2017 ). A first evaluation of the performance of the EEWS was made analyzing the seismic sequence of 2016–2017 ( Festa et al., 2018 ). The system has been continuously operating over the years, without changing configuration, with some temporary interruptions.
In this work, the feasibility of a regional EEWS was evaluated by developing a procedure of event declaration simulation (EDS) for estimating the time useful to activate safety actions. The EDS procedure can be used for different applications: 1) to assess the feasibility of an EEWS in a specific area with an operating seismic network; 2) to plan the integration of new stations into an existing network; 3) to design a new network for an EEWS; and finally, 4) to assess the feasibility of an EEWS varying network density and trigger parameters. Regarding 3) and 4), the EDS can create virtual networks for the areas of interest and allow to plan investments and installations in advance.
The study was mainly based on the calculation of the time first alert (TFA)—the instant in which the event is declared starting from the coincidence of P phases at the stations, the blind zone radius (BZ)—the area in which no safety action can be carried out, and the lead time (LT)—the useful time to initiate safety actions on the targets. In the paper, we first propose a description of the method, of the parameters setting to obtain realistic simulations, and of the EDS validation with PRESTo EEWS. Then, an EDS application in eastern central Italy is showed, discussing the results of the TFA, BZ, and LT mapping in terms of feasibility and limits of the EEW implementation.
2 Event Declaration Simulation Method
The developed EDS is composed by a chain of subroutines including NonLinLoc modules (nonlinear location, or NLLoc; Lomax et al., 2009 ) in the preparatory phase and homemade python scripts in the core of the simulation that emulates some parameters similar to the PRESTo software. The NLLOC package is a well-known and widely used nonlinear inversion code, consisting of a set of programs and where it is possible to integrate an existing velocity model, travel-time calculation and probabilistic solution, for visualization of 3D volume data and location results ( http://alomax.free.fr/nlloc/ ).
The procedure of event declaration simulation needs the following inputs: arrival times of the P phases to the seismic stations, arrival time of the S phases to the targets, a binding configuration and latencies of the real-time data transmission vectors ( Figure 2 ). To obtain the arrival time of P and S phases, a velocity model, a seismic network, a seismic source, and target locations are required.
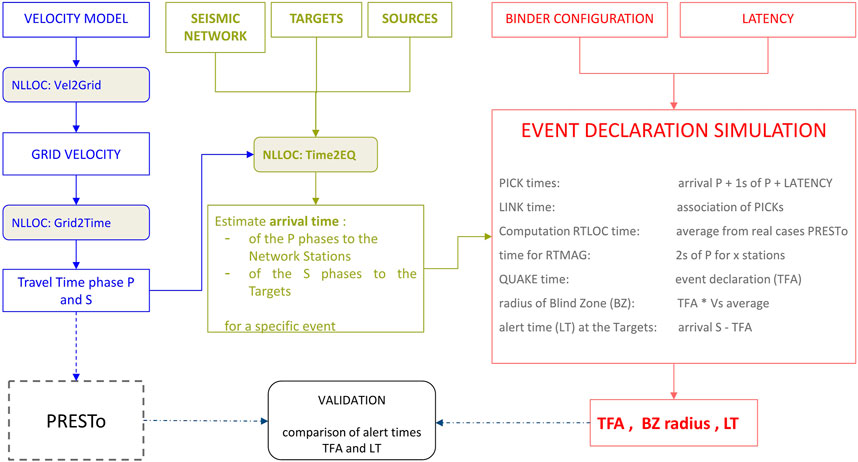
FIGURE 2 . Flow chart of the EDS method. Left (blue): P and S travel time calculation with NonLinLoc software modules ( Lomax et al., 2009 ). Middle (green): P and S arrival time relative at seismic stations and targets respectively. Right (red): input parameters and elements of the EDS core. Bottom : terms of the validation.
Figure 2 shows the most important steps of the simulation procedure, exemplifying the main blocks of the procedure from the inputs to the three outputs: TFA, BZ, and LT.
Starting from a velocity model and using the NLLoc Vel2Grid module, it is possible to create a defined grid of velocities, covering the volume of the study area. Then, the NLLoc Grid2Time module calculates the travel times from node points of the 3D velocity grid to the location of the seismic stations. The so obtained travel times are the same in use in the PRESTo system to locate the real seismic events.
The procedure estimates the arrival times of the P phases to the seismic stations and arrival times of the S phases to the targets, taking advantage of the NLLoc Time2EQ module, given the locations of seismic stations, seismic source and targets.
In the core of the simulation, the expected TFA, BZ, and LT are calculated starting from P and S arrival times, binder configuration (coincidences), and data latencies ( Figure 2 ).
The simulated parameters conceptually emulate some parameters of the PRESTo software.
“PICK time” is defined as the time of the P phase trigger at each seismic station, the sum of the estimated P phase arrival, the accumulation of 1 s of P waveform for the phase picker and the latency of the data packet. “LINK time” signs the moment when a certain number of PICKs are included in a relative small space and time interval and the binder declares an association. The simulation considers a “computation time” useful to locate the supposed event and the accumulation of 2 s of P waveform to compute the magnitude. The “computation time” and the time to compute the magnitude are inferred from real-time application of the PRESTo system implemented in the study area, collecting the log outputs relative to RTLOC and RTMAG modules ( Zollo et al., 2010 ; Satriano et al., 2011b ).
The integration of simulated parameters allows to calculate the “QUAKE time” (TFA) as the needed time to declare the event, summed to the “LINK time”. The BZ is estimated multiplying the TFA times the average of the S velocity in the travelled volume. Finally, the useful time (LT) to initiate actions to secure the targets is obtained from the difference between the arrival time of S phase at the targets and the TFA.
3 Event Declaration Simulation Configuration
The aim of this work is to assess the feasibility of an EEWS in eastern central Italy where a dense seismic network operates, and the main characteristics of the seismicity are well-known ( Figure 1a, b ). The study area is inhabited by about 2.5 million people, distributed in some main cities with population ranging from tens of thousands up to one hundred thousand inhabitants, and in several small historical villages where few hundred people live. Moreover, in most of the target towns and villages, both along the coasts and in the inner Umbria and Marche regions, residents increase dramatically during the summer and other vacation periods.
One of the starting points of the analysis is the velocity model of the volume crossed by seismic waves. A grid of 300 km × 300 km, 67 km thick, starting from 3 km above sea level was created, that could include all the seismicity of the region. A step of 1 km divides nodes of the grid and the central origin geographic point is 43.25 N – 13.00 E. To make the simulation more reliable, we chose a modified version of a 1D velocity model calculated for the region from an instrumental earthquakes catalog ( De Luca et al., 2009 ), preserving the Vp/Vs ratio equal to 1.85 and inserting a gradient between velocity layers ( Supplementary Table 1 ).
The configuration of the INGV seismic network has been evolving over the years. From 2015, when PRESTo software was installed for real-time monitoring and EEW testing, the number of stations has been increasing. Moreover, during the seismic sequence of the year 2016 ( Chiaraluce et al., 2017 ) an emergency temporary seismic network was installed to densify the permanent network ( Moretti et al., 2016 ; SISMIKO, 2020 ). Therefore, we set four seismic network configurations for EDS:
- PSN15: Permanent Seismic Network of the year 2015
- TSN16: Temporary Seismic Network of the 2016 seismic emergency (added to PSN15)
- PSN20: Permanent Seismic Network of the year 2020 (including PSN15)
- ASN20: Accelerometric Seismic Network of the year 2020.
The PSN15 is the same network configuration implemented in the real time PRESTo instance and contributed to validate EDS, to estimate data latencies and test performance of the PRESTo system in Festa et al. (2018) . TSN16 was used to test the response of the network with a significant increase of the density of the PSN15 up to a station inter-distance of about 5 km in the epicentral area of the 2016–2017 seismic sequence. PSN20 contributed to augment dataset of the EDS validation comparing results with a playback instance of the PRESTo software. The ASN20 helped to estimate the network response if we consider only accelerometric components, corresponding to a reduction of the network density, balanced by the certainty of unclipped records. A list of the seismic stations belonging to each network is inserted in Supplementary Table 2 .
The EDS is also able to manage arbitrary virtual networks, composed by scattered or equally spaced grid of stations to design network response in uncovered areas.
Also for the sources, we can input a single seismic source or a set of sources, scattered or equally spaced. The location of a single source, for example, is useful to reproduce the response of the seismic network in terms of TFA for an historical or a recent significant event. Furthermore, for the same event, the EDS returns LT relative to the main cities of the region. Extending the principle to a grid of equally spaced sources, the EDS can map the three output parameters (TFA, BZ, and LT) over the whole region. This approach allows classifying areas characterized by small or large TFA and BZ relative to the events’ epicenters. In this work, for a first mapping of TFA and BZ, we choose a grid of sources 5 km spaced at depth of 10 km. The LT was estimated for all the selected cities ( Table 1 ).
The number of triggered stations and the time interval for association are the main parameters for the set of the binder, the module that allows to declare an event. In the EDS, the binder configuration emulates the “Binder” of PRESTo software parameters ( PRESTo, 2013 ). We adopt the setup used in the real-time PRESTo instance for choosing the values of binder parameters in the EDS ( Table 2 ). The number of at least six stations in coincidence (STA_CO) inside a time window of 3 s (SEC_CO) is a good compromise between the heterogeneous density of the seismic network and the requirement of a rapid response of the system considered the distances of targets from the sources. The SEC_AS parameter is set to 10 s, a value that avoids the effect of the shift of a good location during grid search in the playback PRESTo instance with respect to the results of the real-time instance with SEC_AS = 15 s. The AVEL_MIN, AVEL_MAX, VEL_SPA, VEL_DIST parameters design a cone inside which the coincidence picks must fall.

TABLE 2 . Configuration Parameters of the EDS binder.
A key factor to take into account for an EEWS is the data latency due to the communication protocol ( Satriano et al., 2011a ). The latency affects the alert times of the EEWS which can only be activated when a good part of the data is available in nearly true real-time. The seismic data of the stations in eastern central Italy are transmitted by different types of transmission vectors: TCP/IP, WiFi, GPRS/UMTS, and Satellite (SAT-LIBRA and KA-SAT). At the time of the analysis, LTE routers were not available. Starting from the PRESTo log files, the real latency data for 86 seismic stations for the period 2015–2019 were collected. The long time window of the analysis allowed in some cases to assess the improved performance of the stations after the change of the transmission protocol. An average value of the latency for each type of transmission vectors was calculated by the geometric mean to exclude outliers, that is, values significantly out of the trend, referred to a malfunction of the station. The results of the latency classification are listed in Table 3 with the average values calculated and the number of stations used. Considering the main transmission vectors used in the network of this study, it is possible to make some considerations. As expected, the stations with a satellite time division multiple access carrier system (i.e., SAT-LIBRA) are those with the greatest latency and are not good for EEW application in small areas, but in our case few stations are equipped with this type of satellite transmission. Instead, another satellite system (i.e., KA-SAT) returns an average of 2.64 s, an acceptable value for EEW applications. The TCP/IP, WiFi, and GSM/UMTS latencies range between 1.86 and 2.83 s. The best value is for direct connection TCP/IP from remote stations. TCP/IP connections from other acquisition centers connected with a mixed copper-fiber line return a slight worsening. The transmission by WiFi backbone gives back a latency of 2.07 s, confirming suitable use for EEW systems. The WiFi system is not a public system, but a system dedicated to civil protection services, available thanks to the Regione Marche authority through an agreement with INGV. Each system used for data transmission (TCP/IP, WiFi, and GSM/UMTS, Satellite) could suffer temporary blackout of the communication lines. In case of a strong earthquake the redundancy of the transmission lines used in the network should reduce the risk of data blackout.
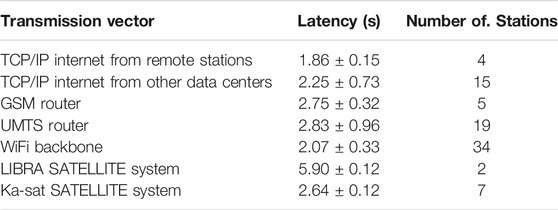
TABLE 3 . Transmission vector, mean and standard deviation of data latency, and number of stations used for each type.
Besides transmission times, another important parameter for an efficient EEWS is the computing time, defined as the difference between the association time of the stations and the computed TFA.
During this time, in the real-time system, the location and magnitude are estimated and the event is declared. The EDS does not simulate the earthquake location process and the magnitude estimate. So, the computing times inserted in our simulation are extracted from a statistics of the real-time PRESTo instance. The time difference between “QUAKE time” and “LINK time” was calculated and compared on a dataset of 91 events ( Supplementary Table 3 ) detected in real-time by the PRESTo system and re-simulated by EDS. The events, belonging to the INGV bulletin, were selected from August 2016 to May 2020, with magnitude 3.0 ≤ Mw ≤ 6.0. These events were detected by the system in real-time for the same period in the study area. The calculation times, taken from the system files in real-time, were distributed according to a lognormal curve ( Figure 3 ). The calculated values are mode equal to 0.29 s, median equal to 0.42 s, and average 0.51 s. Following these results, a value of 0.30 s was chosen as the average computation time for the simulation.
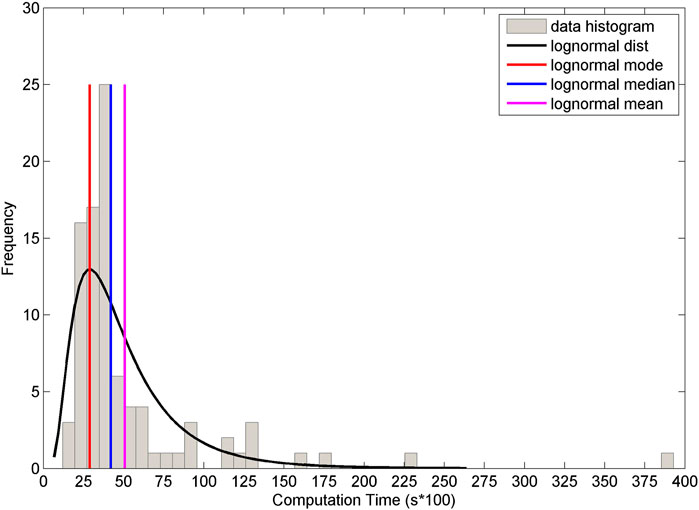
FIGURE 3 . Statistic of the computation time values.
4 Event Declaration Simulation Validation
The simulation procedure was validated comparing the first time of the alerts (TFA) of the EDS with first “QUAKE time” of the PRESTo instances. In the comparison with real time outputs of the PRESTo system, the values of mean latencies belonging to different transmission vectors are inserted in the EDS. Differently, for the comparison with PRESTo playback outputs, a zero latency was set in EDS. These two approaches help to increase the reliability of the validation, excluding that results are affected by a bad estimate of the latencies.
Figure 4 shows a detail of the processing of Mw 5.4 October 26, 2016 event and the comparison of arrival times relative to P phases of the triggered stations, the TFA and the arrival of S phases useful to calculate the LT at the target INGV_AN in the city of Ancona (epicentral distance 88 km). The black marks represent an operator review of the P phases at the triggered stations and the S phase at the target station (cT0). The black point represents the origin time of the located event, taken into account for the EDS test. The red marks are relative to PRESTo instances (cT1, cT3), while the blue marks are relative to EDS (cT2, cT4, cT5).
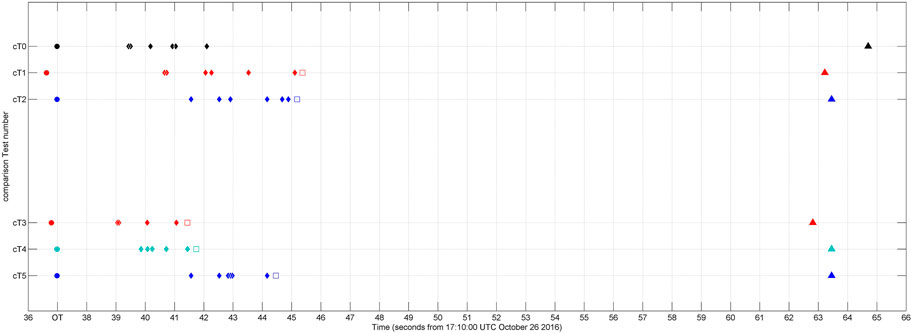
FIGURE 4 . Comparison of arrival times relative to Mw 5.4 October 26, 2016 earthquake. Circles: Origin Time. Diamonds: P phase arrival times of the triggered stations. Squares: TFA. Triangles: S phase arrival times at INGV_AN target in city of Ancona (88 km epicentral distance). cT0: arrival times of human event revision. cT1: PRESTo real time instance with PSN15. cT2: EDS with real latency and with PSN15. cT3: PRESTo playback instance with PSN15 and TSN16. cT4: EDS with zero latency and PSN15 + TSN16. cT5: EDS with real latency with PSN15 + TSN16.
The arrival times of operator reviewed P phases are lesser than the PRESTo and EDS real time tests where the data latencies are present. The reviewed arrival time is more similar for the tests in playback where zero latency is setting up but a 1 s of P waveform analysis remains.
The comparison of the TFA between PRESTo and EDS is very good for the two approaches, that is, real-time (cT1 vs cT2) and playback (cT3 vs cT4). The effect of the data latencies in the real time case imply a delay of about 3 s of the TFA with respect to the last P phase arrival time of the human reviewed case. This result is coherent with the values of the mean latencies calculated for the different transmission protocol ranging between 2 and 3 s inserted in the test, and 1 s needed to process the P waveform. The playback version of the test return a little early TFA respect to the last reviewed P phase, both for PRESTo and EDS, but the difference is small, 0.7 and 0.4 s respectively. Finally, the EDS allows to estimate a theoretical TFA adding emergency temporary seismic stations, active in epicentral area at the time of the earthquake, and stations data latencies (cT5). The effect on TFA is about 1 s of the advance respect the PRESTo real-time case (cT1), simply for the early achievement of the station coincidence determined by an augmented network density in the epicentral area. The values of S phase arrival times of PRESTo and EDS are coherent thanks to the same modeling of velocity volume used for travel time computation. From the good simulation of the TFA and S phase arrival at the target by EDS, a good estimate of the LT follows.
For a general validation of the TFA estimated with EDS, we selected 20 seismic events with 3.0 ≤ Mw ≤ 6.5, all elaborated also by PRESTo playback instance and 16 events by real-time instance. The selected events are scattered over the study area and over time, with the aim to insert in these tests different and unfavorable states of the network in terms of station inter-distances. The events belong to a time window from August 24, 2016, to January 28, 2020, with the hypocentral depth between 6.8 and 33.3 km ( Supplementary Table 4 ). The time window includes some major events of the 2016 Central Italy sequence, located in the southern sector of the region, while the other events were chosen in order to perform tests in the northern part of the region.
Table 4 summarizes the results of EDS estimates of TFA compared with real-time and playback PRESTo instances obtained by setting up the PSN15 configuration network. Moreover, the playback configuration (zero latency) was used to return a TFA mean difference, adding PSN16 emergency temporary station data relative to 2016–2017 events and the PSN20 network configuration for more recent events.

TABLE 4 . Results of the TFA comparison relative to real time and in playback PRESTo instances.
The TFA validation results return mean values lower than 1.0 s. The negative sign indicates an early TFA of the EDS, ascribable to non-simulation of the recursive recalculation of the location and magnitude that occurs in some real cases (PRESTo system) with the arrival of new data. The results of PSN15 real-time and playback cases (validation test 1 and 2) are similar, confirming a reliable simulation for both data latencies and station triggering, respectively. The last case (validation test 3) takes advantage of using 2016 temporary stations and latest installed stations, therefore the network density augmented in a part of analysis. The third test shows a better result, reducing the mean and the uncertainty of TFA difference.
The success of the EDS validation tests allows to perform simulations useful to quantify the EEW response of the actual seismic networks operating in eastern central Italy and to estimate the LT for the main cities in the region.
5 Event Declaration Simulation Applications, Results and Discussion
The EDS was developed to evaluate the EEW response of INGV seismic network in eastern central Italy in terms of TFA, BZ, and LD, but it is useful also to reproduce and design the feasibility of the system in different or not yet monitored areas.
The first possible application allowed by EDS is the response of a seismic network relative to one single seismic event with the aim of assessing LT for a set of targets.
Considering the hypocentral location of the Mw 6.0 August 24, 2016, event, we have simulated the response of four network configurations ( Table 5 ), assessing the LT for the main cities of the study area ( Figure 5 ). During the analysis, the calculation of the BZ is performed by multiplying the TFA by the average Vs estimated at the last station useful for coincidence. The TFA value is calculated as the arrival time of the P wave at the last station, added to the latency value and the estimated computing time of the system. Vs is calculated as the product of mean Vp at the last station for coincidence by the ratio Vs/Vp ( De Luca et al., 2009 , reported in Supplementary Table 1 ). In the first test (SST1), we used the PSN15 seismic network, the existing network at the time of the event. The second test (SST2) was performed inserting all stations of the TSN16, simulating the presence of the whole emergency network. This temporary seismic network was installed after the earthquake of August 24, 2016. The third test (SST3) represents the unfavorable case of a network consisting of only the current accelerometric INGV stations (ASN20). The last test (SST4) is an example of the design of a virtual arbitrary seismic network with the station inter-distance of 5 km. The calculated mean latency was associated to the PSN15 stations used for the latency statistics, a 2.75 s GSM/UMTS mean latency was associated to the TSN16 stations, and the mean latency was associated at new stations not present in PSN15, depending on their transmission vector. The virtual network with the inter-distance of 5 km was designed like a GSM/UMTS network and a mean latency of 2.75 s was applied to all virtual stations.

TABLE 5 . Results of tests with single source and different network configurations.
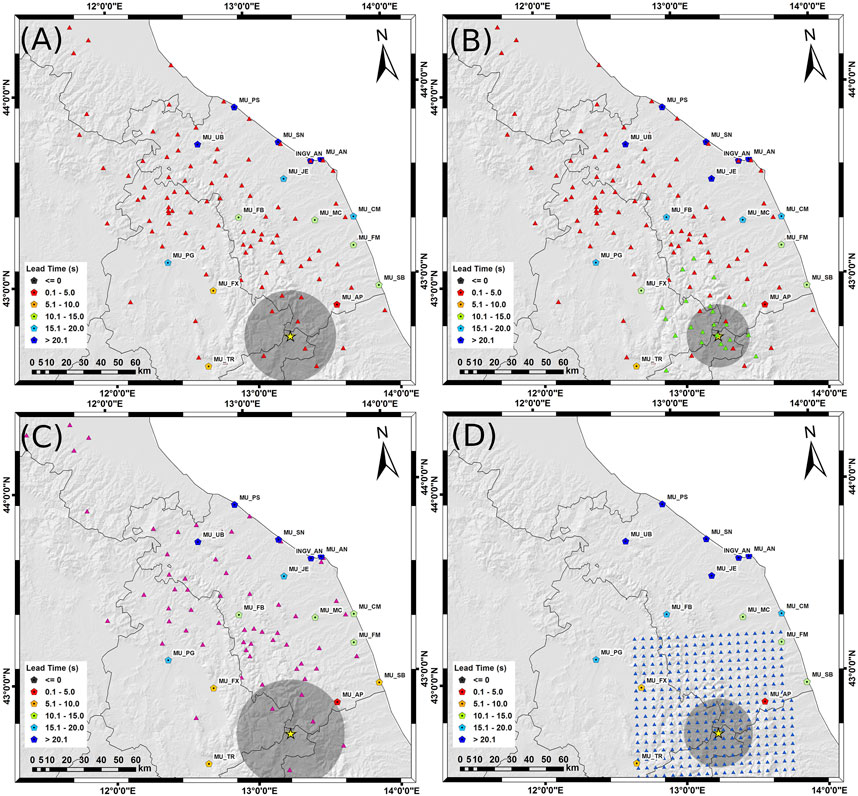
FIGURE 5 . EDS of the EEW response relative to a single source. Yellow star: location of the Mw 6.0 August 24, 2016 earthquake. Gray circle: Blind Zone. Pentagon: Targets. Legend: color map of the LT (s) linked to the targets. (A) SST1 test. Red triangles: PSN15 network. (B) SST2 test. Red triangles: PSN15 network; green triangles: TSN16. (C) SST3 test. Purple triangles: ASN20. (D) SST4 test. Blue triangles: 5 km inter-distance virtual network.
The network response in terms of TFA varies in dependence of the seismic station distribution and of network density around the epicentral area ( Figure 5 ). The addition of TSN16 to PSN15 around the seismic event causes a reduction of TFA from 8.7 to 6.3 s (gain of 2.4 s) and a reduction of the blind zone (BZ) radius from 26.5 to 18.3 km ( Table 5 ). In the third case, the sparse distribution of the accelerometric stations around the epicentral area causes a worsening of the network response with respect to the other two tests and TFA and BZ jump to 9.8 s and 31.4 km respectively. In the SST3 test, the MU_AP target has not safely time for activate protection actions, being on the BZ border, and MU_SB, MU_FX, and MU_TR targets have less than 10 s of LT ( Figure 5C and Table 6 ). The sample SST4 returns similar values of the SST2 test ( Table 5 and Table 6 ), strengthening the idea that the EDS can represent a tool for design an improvement of the EEWS starting from an existent seismic network or a new EEWS imaging an entirely new network in uncovered areas.
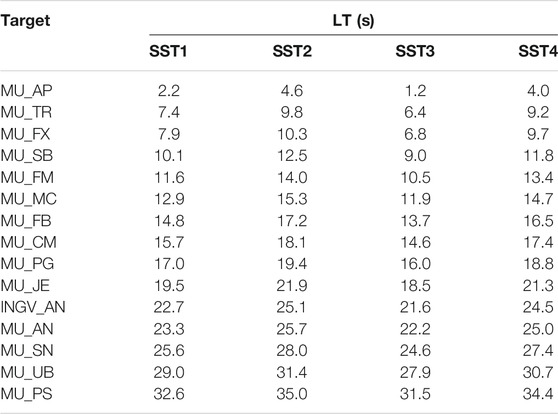
TABLE 6 . Results of LT for selected targets. Target: city code. Place: extended name of cities. LT: Values of LT in seconds referred to the test in Table 4 .
The EDS is also a tool to map the TFA, BZ, and LT over the territory. Indeed, it is possible to configure the procedure including a set of sources, every combined with a TFA and a BZ value. Moreover, it is possible to obtain LT linked to each epicentral location referred to a single target.
The example to explain the use of EDS to mapping EEW parameters is showed in Figure 6 , where the PSN20 is applied. In the left column, a set of locations referred to the main historical seismic events of Mw ≥ 5.5 from 1269 to 2017 AD that hit the region is presented ( Figure 6A ), extracted from CPTI15 catalog ( Rovida et al., 2021 ). In the right column, a grid of sources with inter-distance of 5 km, depth of 10 km and covering the whole region is depicted ( Figure 6B ). The example of historical events answers the question of which TFA and BZ would occur with the actual seismic network if seismic events repeat in the same locations. Each historical epicentral location is mapped in terms of TFA and BZ ( Figures 6C, E ). Smaller TFA (red points) are mapped where the network is denser in inland areas at the center of the network. For southern inland events and coastal events (yellow dots), the EEWS provides less protection which is significantly reduced for northern events (green dots) where TFA greater than 10 s results in BZ with a radius greater than 30 km.
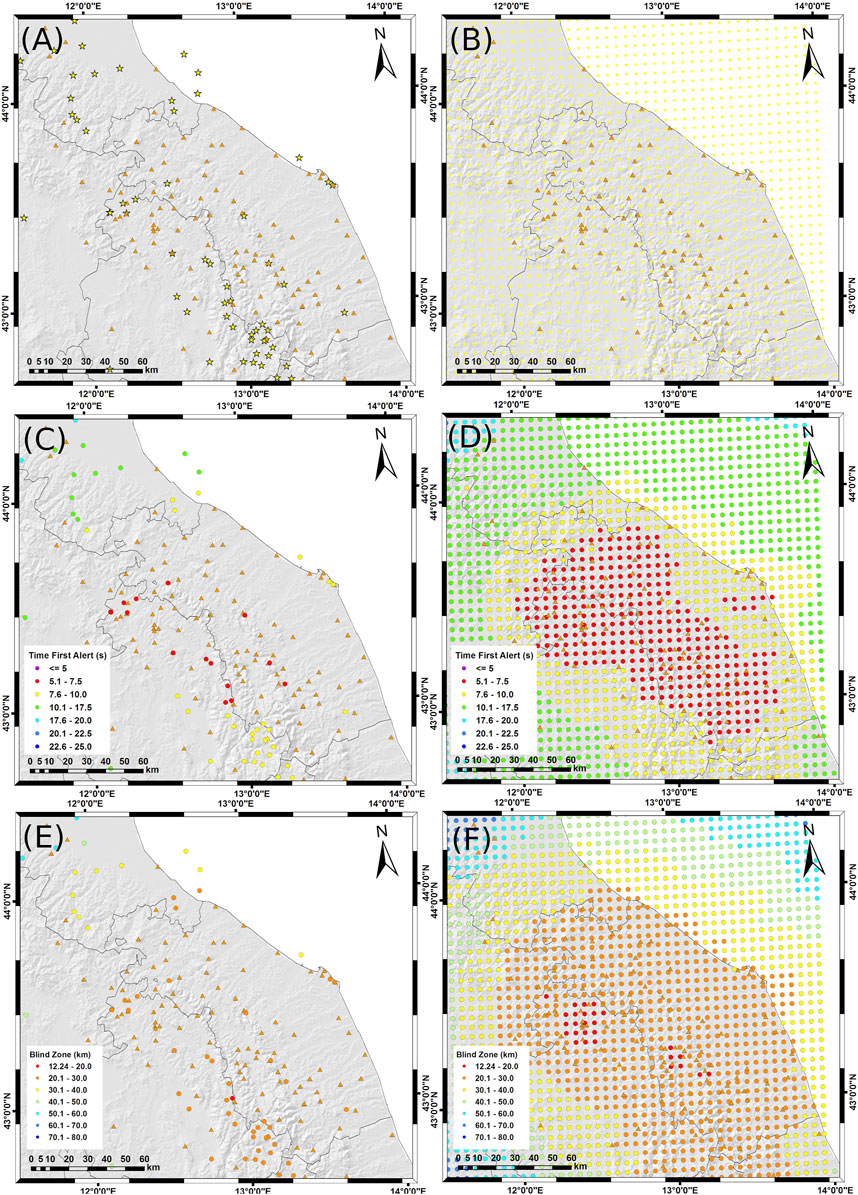
FIGURE 6 . TFA and BZ mapping. (A) : historical events from CPTI15 v3.0 Mw ≥ 5.5 from1269 to 2017 AD, depth 10 km before 1997. (B) : virtual grid of sources distant of 5 km and depth of 10 km. (C) : mapping of TFA with historical events. (D) : mapping of TFA with virtual grid. (E) : mapping of BZ with historical events. (F) : mapping of BZ with virtual grid.
The same principle can be extended to the whole territory, mapping TFA ( Figure 6D ), and BZ ( Figure 6F ) with the grid of sources. The map of the TFA depicts the edges of the areas within which, if a seismic event occurs, the EEWS responds with a TFA threshold. In particular, for the eastern part of central Italy, with the actual INGV network, an EEWS could produce TFA less than 7.5 s for a large inland area that includes part of the most active seismic zones. Around the Adriatic coast, the seismic network is less dense and an earthquake that occurs off-shore is out of the network. Therefore, the coastal and off-shore TFAs are shorter than those in the inland zones. Besides, the elongated distribution of the seismic stations next to the coast could cause bad locations and estimate of the magnitude by the EEWS, worsening even more the protection provided by the alarm. Also, the northern part of the region is lacking stations and the resulting TFA are similar to the off-shore values. For the largest part of the region, a BZ ranging between 20 and 30 km from the epicenter would not be protected by a warning. The BZ radius increases up to over 30–40 km for the off-shore locations and the EEW system could not produce a TFA for coastal cities. To overcome this problem, offshore seismometers would be extremely useful.
The EDS allows exporting results of the TFA values to map LT referred to a single target. The LT map helps to link places of hypothetical epicenters and the time available for safety actions at the target. For example, the map in Figure 7 shows the LT available for the city of Fabriano (in particular for the location of the city Hall, MU_FB target). The color map shows the values of LT in equally spaced sources, located at 10 km depth. The black dots limit the epicenters for which the city of Fabriano falls in the BZ. Indeed, in this zone the LT is negative (≤0 s). The area marked with red dots corresponds to a 0 s < LT ≤ 5 s where an alarm could be provided but security actions are unlikely. In the orange area (5 s < LT ≤ 10 s) triggering automatic actions would be possible ( Cauzzi et al., 2016 ). Besides the orange area (LT > 10 s), trained people can take precautionary and protective actions ( Fujinawa and Noda, 2013 ) and the probability of a successful alert increases significantly ( Becker et al., 2020b ).
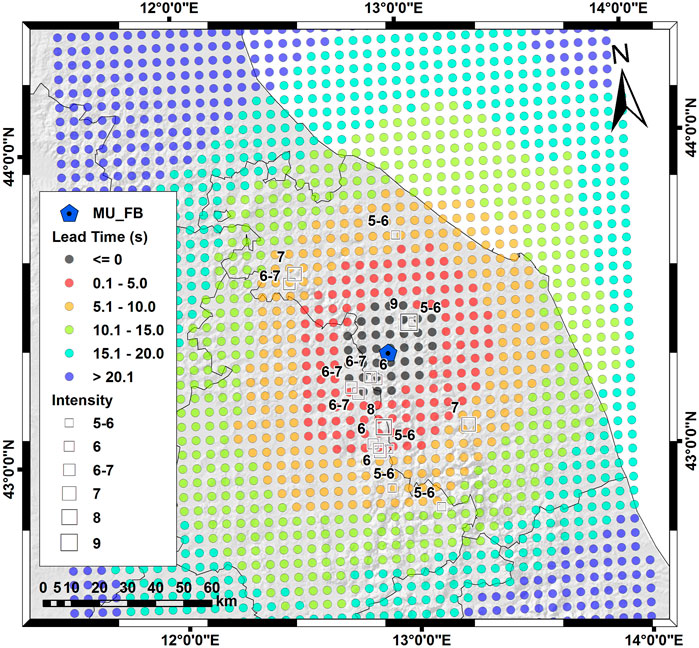
FIGURE 7 . LT mapping for city of Fabriano and historical destructive earthquake. Blue pentagon: place of city of Fabriano (MU_FB target). Colored circles: values of LT in seconds. Squares: CPTI15 v3.0 historical earthquakes and macroseismic intensity (DBMI15 v3.0, Locati et al., 2021 ) estimated for the city of Fabriano.
This argument makes sense in particular for those earthquakes that could damage the target. Therefore, the destructive earthquakes for the city of Fabriano, extracted from CPTI15 catalog ( Rovida et al., 2021 ), overlap the LT map in Figure 7 . Clearly, most of the events fall in black and red areas, since the MU_FB target is placed close to the inland active seismic zone ( Figure 1 ) and the EEWS would be almost useless. However, some historical events that caused damages with a macroseismic intensity from five to six to 7, fall in the orange area where automatic actions are possible. For the southernmost event (Mw 6.5 on October 30, 2016), an EEWS could have provided an alert 10 s before the arrival of the first S seismic waves to the city of Fabriano, a good LT to take several safety actions.
6 Conclusion
The feasibility of an EEWS derives from the design of a seismic network with respect to the seismic sources located within or around it. EEWSs are today an effective contribution to the problem of seismic risk mitigation, but few countries have operational systems ( Satriano et al., 2011b ). Implementation of EEWSs in Italy is a challenge. Italy is an elongated peninsula, with its central part crossed by a mountain chain that is seismically active and runs very close to urbanized areas. Therefore, the simulations of seismic networks contribute in the field of territorial safety by identifying the time in which some types of protection actions could be activated.
In this work, we have developed a simulation procedure (EDS), useful to estimate the feasibility of an EEWS and showed some applications in eastern central Italy, where INGV manages a dense seismic network. A validation process was performed by comparing results of simulations with real-time and playback instances of the EEW PRESTo system implemented in the same region ( Festa et al., 2018 ).
EDS is a tool to simulate different seismic network responses reproducing the physical contest of a specific historical or recent earthquake; moreover, EDS is a tool to map EEW parameters (TFA, BZ, and LT) to classify the whole territory in terms of areas where it is possible to activate safety actions. With EDS, it is possible to model real seismic networks inserting actual or theoretical data latencies due to different transmission vectors and to design new seismic networks in uncovered areas.
As expected, the results of the EDS application in eastern central Italy highlights short, but still useful, alert times for innermost land and coastal areas overlooking the active seismic zones. However, we have shown that most of the currently used transmission vectors have latencies between 2 and 3 s, an acceptable value for seismic early warnings. The quantitative estimate of TFA, BZ, and LT supplies useful information to project an improvement of the EEWS with the aim to reduce as much as possible the TFA. Modern approaches include the use of low-cost sensors for a greater diffusion of the seismic monitoring throughout the territory, and the development of networks oriented toward smart cities using fast protocols and connectivity ( Ladina et al., 2016 ; Pierleoni et al., 2018 ; D’Alessandro et al., 2019 ). In the area of the 2016 Central Italy sequence, we have estimated a gain of about 2.5 s of LT, adding the 2016 emergency temporary stations (station inter-distance about 5 km) over the permanent network, with a data latency similar to GPRS connections. The result was repeated setting a virtual network in a station grid with a constant inter-distance of 5 km and a mean data latency of 2.6 s to demonstrate the ability to project an EEWS in uncovered areas and to obtain realistic times of the first alert.
Although the Italian territory is mostly characterized by seismic sources next to urbanized areas, the lead times calculated with EDS procedure encourage the opportunity of implementing an EEWS for several interesting targets. Simulating historical events, a LT between 5 and 10 s was reproduced for some of these targets. We conclude that automatic safety actions, situational awareness and a more rapid response by well-trained people are a realistic goal in eastern central Italy.
We are aware that the study presented here has some limitations, first of all the system performance is evaluated analyzing mainly the rapidity of an EWS, whereas no evaluation assessment is made on the reliability of the earthquake impact prediction. This implies that the evaluation of the shaking could be inaccurate or even lead to false or missed alerts. However, the goal of our analysis was to determine whether an EWS could be a viable solution to reduce seismic exposure in certain regions of Italy, and in which conditions (network geometry, transmission times, etc.). Our results are encouraging provided that some technological issues are considered and that people’s awareness of seismic risk is increased. Further studies will be dedicated to a more thorough assessment of EWS.
The development of a widespread monitoring infrastructure near the main seismic sources, the massive training of citizens, and the collaboration with civil protection authorities could improve the scenarios simulated in this work, making an EEWS really effective in protecting people from the harmful effects of earthquakes in Italy.
Data Availability Statement
The raw data supporting the conclusions of this article will be made available by the authors, without undue reservation.
Author Contributions
Conceptualization; methodology; validation; data analysis and curation: CL, SM; data analysis supervision: AA, MC; writing-original draft preparation; writing-review and editing: CL, SM, AA, MC. All authors contributed to manuscript, read and approved the submitted version.
This work has been carried out within the Project ART-IT (Allerta Rapida Terremoti in Italia), funded by the Italian Ministry of University and Research (Progetto Premiale 2015, DM. 850/2017), and supported also by the Agreement between Civil Protection Department of the Regione Marche and INGV.
Conflict of Interest
The authors declare that the research was conducted in the absence of any commercial or financial relationships that could be construed as a potential conflict of interest.
Publisher’s Note
All claims expressed in this article are solely those of the authors and do not necessarily represent those of their affiliated organizations, or those of the publisher, the editors and the reviewers. Any product that may be evaluated in this article, or claim that may be made by its manufacturer, is not guaranteed or endorsed by the publisher.
Acknowledgments
The authors thank Mario Locati for support with DBMI15 v2.0, Luca Elia for suggestion and explanations with PRESTo configuration, and Simona Colombelli for the suggestions about the details of the work.
Supplementary Material
The Supplementary Material for this article can be found online at: https://www.frontiersin.org/articles/10.3389/feart.2021.685751/full#supplementary-material
Alcik, H., Ozel, O., Apaydin, N., and Erdik, M. (2009). A Study on Warning Algorithms for Istanbul Earthquake Early Warning System. Geophys. Res. Lett. 36, L00B05. doi:10.1029/2008GL036659
CrossRef Full Text | Google Scholar
Allen, R. M., Brown, H., Hellweg, M., Khainovski, O., Lombard, P., and Neuhauser, D. (2009a). Real-time Earthquake Detection and hazard Assessment by ElarmS across California. Geophys. Res. Lett. 36, L00B08. doi:10.1029/2008GL036766
Allen, R. M., Gasparini, P., Kamigaichi, O., and Böse, M. (2009b). The Status of Earthquake Early Warning Around the World: An Introductory Overview. Seismological Res. Lett. 80 (5), 682–693. doi:10.1785/gssrl.80.5.682
Allen, R. M., and Kanamori, H. (2003). The Potential for Earthquake Early Warning in Southern California. Science 300, 786–789. doi:10.1126/science.1080912
PubMed Abstract | CrossRef Full Text | Google Scholar
Allen, R. M., and Melgar, D. (2019). Earthquake Early Warning: Advances, Scientific Challenges, and Societal Needs. Annu. Rev. Earth Planet. Sci. 47, 361–388. doi:10.1146/annurev-earth-053018-060457
Becker, J. S., Potter, S. H., Prasanna, R., Tan, M. L., Payne, B. A., Holden, C., et al. (2020b). Scoping the Potential for Earthquake Early Warning in Aotearoa New Zealand: A Sectoral Analysis of Perceived Benefits and Challenges. Int. J. Disaster Risk Reduction 51, 101765. doi:10.1016/j.ijdrr.2020.101765
Becker, J. S., Potter, S. H., Vinnell, L. J., Nakayachi, K., McBride, S. K., and Johnston, D. M. (2020a). Earthquake Early Warning in Aotearoa New Zealand: a Survey of Public Perspectives to Guide Warning System Development. Humanit Soc. Sci. Commun. 7. doi:10.1057/s41599-020-00613-9
Böse, M., Ionescu, C., and Wenzel, F. (2007). Earthquake Early Warning for Bucharest, Romania: Novel and Revised Scaling Relations. Geophys. Res. Lett. 34, L07302. doi:10.1029/2007GL029396
Caruso, A., Colombelli, S., Elia, L., Picozzi, M., and Zollo, A. (2017). An On-Site Alert Level Early Warning System for Italy. J. Geophys. Res. Solid Earth 122. doi:10.1002/2016JB013403
Cattaneo, M., Frapiccini, M., Ladina, C., Marzorati, S., and Monachesi, G. (2017). A Mixed Automatic-Manual Seismic Catalog for Central-Eastern Italy: Analysis of Homogeneity. Ann. Geophys. 60, 6. doi:10.4401/ag-7333
Cauzzi, C., Behr, Y., Le Guenan, T., Douglas, J., Auclair, S., Woessner, J., et al. (2016). Earthquake Early Warning and Operational Earthquake Forecasting as Real-Time hazard Information to Mitigate Seismic Risk at Nuclear Facilities. Bull. Earthquake Eng. 14, 2495–2512. doi:10.1007/s10518-016-9864-0
Chiaraluce, L., Amato, A., Carannante, S., Castelli, V., Cattaneo, M., Cocco, M., et al. (2014). The Alto Tiberina Near Fault Observatory (Northern Apennines, Italy). Ann. Geophys. 57, 3. doi:10.4401/ag-6426
Chiaraluce, L., Di Stefano, R., Tinti, E., Scognamiglio, L., Michele, M., Casarotti, E., et al. (2017). The 2016 Central Italy Seismic Sequence: A First Look at the Mainshocks, Aftershocks, and Source Models. Seismological Res. Lett. 88 (3), 757–771. doi:10.1785/0220160221
Clinton, J., Zollo, A., Marmureanu, A., Zulfikar, C., and Parolai, S. (2016). State-of-the Art and Future of Earthquake Early Warning in the European Region. Bull. Earthquake Eng. 14, 2441–2458. doi:10.1007/s10518-016-9922-7
Colombelli, S., Amoroso, O., Zollo, A., and Kanamori, H. (2012a). Test of a Threshold-Based Earthquake Early-Warning Method Using Japanese Data. Bull. Seismological Soc. America 102, 1266–1275. doi:10.1785/0120110149
Colombelli, S., Caruso, A., Zollo, A., Festa, G., and Kanamori, H. (2015). A P Wave‐based, On‐site Method for Earthquake Early Warning. Geophys. Res. Lett. 42, 1390–1398. doi:10.1002/2014GL063002
Colombelli, S., Zollo, A., Festa, G., and Kanamori, H. (2012b). Early Magnitude and Potential Damage Zone Estimates for the Great Mw 9 Tohoku-Oki Earthquake. Geophys. Res. Lett. 39, a–n. doi:10.1029/2012gl053923
Cua, G., Fischer, M., Heaton, T., and Wiemer, S. (2009). Real-time Performance of the Virtual Seismologist Earthquake Early Warning Algorithm in Southern California. Seismological Res. Lett. 80, 740–747. Number 5. doi:10.1785/gssrl.80.5.740
D’Alessandro, A., Costanzo, A., Ladina, C., Buongiorno, F., Cattaneo, M., Falcone, S., et al. (2019). Urban Seismic Networks, Structural Health and Cultural Heritage Monitoring: The National Earthquakes Observatory (INGV Ltaly) Experience. Front. Built Environ. doi:10.3389/fbuil.2019.00127
De Luca, G., Cattaneo, M., Monachesi, G., and Amato, A. (2009). Seismicity in Central and Northern Apennines Integrating the Italian National and Regional Networks. Tectonophysics 476, 121–135. doi:10.1016/j.tecto.2008.11.032
DISS Working Group (2018). Database of Individual Seismogenic Sources (DISS), Version 3.2.1: A Compilation of Potential Sources for Earthquakes Larger Than M 5.5 in Italy and Surrounding Areas. Istituto Nazionale di Geofisica e Vulcanologia. Available at: http://diss.rm.ingv.it/diss/ . doi:10.6092/INGV.IT-DISS3.2.1
Dunn, P. T., Ahn, A. Y. E., Bostrom, A., and Vidale, J. E. (2016). Perceptions of Earthquake Early Warnings on the U.S. West Coast. Int. J. Disaster Risk Reduction 20, 112–122. doi:10.1016/j.ijdrr.2016.10.019
Erdik, M., Fahjan, Y., Ozel, O., Alcik, H., Mert, A., and Gul, M. (2003). Instabul Earthquake Rapid Response and the Early Warning System. Bull. Earthquake Eng. 1 (1), 157–163. doi:10.1023/A:1024813612271
Festa, G., Picozzi, M., Caruso, A., Colombelli, S., Cattaneo, M., Chiaraluce, L., et al. (2018). Performance of Earthquake Early Warning Systems during the 2016-2017 Mw 5-6.5 Central Italy Sequence. Seismol. Res. Lett. 89, 1–12. doi:10.1785/0220170150
Fujinawa, Y., and Noda, Y. (2013). Japan's Earthquake Early Warning System on 11 March 2011: Performance, Shortcomings, and Changes. Earthquake Spectra 29 (S1), S341–S368. doi:10.1193/1.4000127
Gorini, A., Nicoletti, M., Marsan, P., Bianconi, R., De Nardis, R., Filippi, L., et al. (2010). The Italian strong Motion Network. Bull. Earthquake Eng. 8, 1075–1090. doi:10.1007/s10518-009-9141-6
Hsiao, N.-C., Wu, Y.-M., Shin, T.-C., Zhao, L., and Teng, T.-L. (2009). Development of Earthquake Early Warning System in Taiwan. Geophys. Res. Lett. 36, L00B02. doi:10.1029/2008GL036596
Iannaccone, G., Zollo, A., Elia, L., Convertito, V., Satriano, C., Martino, C., et al. (2010). A Prototype System for Earthquake Early-Warning and Alert Management in Southern Italy. Bull. Earthquake Eng. 8, 1105–1129. doi:10.1007/s10518-009-9131-8
Iervolino, I., Galasso, C., and Manfredi, G. (2008). “Information-dependent lead-time Maps for Earthquake Early Warning in the Campania Region,” in The 14th World Conference on Earthquake Engineering , Beijing, China , October 12-17, 2008 .
Google Scholar
INGV Seismological Data Centre (2006). Rete Sismica Nazionale (RSN) . Italy: Istituto Nazionale di Geofisica e Vulcanologia (INGV) . doi:10.13127/sd/x0fxnh7qfy
CrossRef Full Text
Kohler, M. D., Smith, D. E., Andrews, J., Chung, A. I., Hartog, R., Henson, I., et al. (2020). Earthquake Early Warning ShakeAlert 2.0: Public Rollout. Seismol. Res. Lett. 91 (3), 1763–1775. doi:10.1785/0220190245
Ladina, C., Marzorati, S., Monachesi, G., Cattaneo, M., Frapiccini, M., and Castelli, V. (2016). Strong-motion Observations Recorded in Strategic Public Buildings during the 24 August 2016 Mw 6.0 Amatrice (central Italy) Earthquake. Ann. Geophys. 59. Fast Track 5 (2016): The Amatrice seismic sequence: preliminary data and results. doi:10.4401/ag-7194
Locati, M., Camassi, R., Rovida, A., Ercolani, E., Bernardini, F., Castelli, V., et al. (2021). Database Macrosismico Italiano (DBMI15), versione 3.0 . Istituto Nazionale di Geofisica e Vulcanologia (INGV) . doi:10.13127/DBMI/DBMI15.3
Lomax, A., Michelini, A., and Curtis, A. (2009). “Earthquake LocationEarthquake Location, Direct, Global-Search Methods,” in Encyclopedia of Complexity and System Science (New York: Springer ), 2449–2473. doi:10.1007/978-0-387-30440-3_150
Moretti, M., Pondrelli, S., Margheriti, L., Abruzzese, L., Anselmi, M., Arroucau, P., et al. (2016). SISMIKO: Emergency Network Deployment and Data Sharing for the 2016 central Italy Seismic Sequence. Ann. Geophys. 59.Fast Track 5. doi:10.4401/ag-7212
Odaka, T., Ashiya, K., Tsukada, S., Sato, S., Ohtake, K., and Nozaka, D. (2003). A New Method of Quickly Estimating Epicentral Distance and Magnitude from a Single Seismic Record. Bull. Seismological Soc. America 93 (1), 526–532. doi:10.1785/0120020008
Olivieri, M., Allen, R. M., and Wurman, G. (2008). The potential for earthquake early warning in Italy using ElarmS. Bull. Seismol. Soc. Am. 98 (1), 495–503. doi:10.1785/0120070054
Picozzi, M. (2012). An Attempt of Real-Time Structural Response Assessment by an Interferometric Approach: A Tailor-Made Earthquake Early Warning for Buildings. Soil Dyn. Earthquake Eng. 38, 109–118. doi:10.1016/j.soildyn.2012.02.003
Picozzi, M., Zollo, A., Brondi, P., Colombelli, S., Elia, L., and Martino, C. (2015). Exploring the Feasibility of a Nationwide Earthquake Early Warning System in Italy. J. Geophys. Res. Solid Earth 120, 2446–2465. doi:10.1002/2014JB011669
Pierleoni, P., Marzorati, S., Ladina, C., Raggiunto, S., Belli, A., Palma, L., et al. (2018). Perfarmance Evaluation of a Low-Cost Sensing Unit for Seismic Applications: Field Testing during Seismic Events of 2016-2017 in Central Ltaly. IEEE Sensor Joumal 18, 16. doi:10.11O9/JSEN.2018.285006510.1109/jsen.2018.2850065
PRESTo, (2013). Probabilistic and Evolutionary Early Warning SysTem. Available at: http://www.prestoews.org (Accessed March 25, 2021).
Rovida, A., Locati, M., Camassi, R., Lolli, B., Gasperini, P., and Antonucci, A. (2021). Catalogo Parametrico dei Terremoti Italiani (CPTI15), versione 3.0 . Istituto Nazionale di Geofisica e Vulcanologia (INGV) . doi:10.13127/CPTI/CPTI15.3
Satriano, C., Elia, L., Martino, C., Lancieri, M., Zollo, A., and Iannaccone, G. (2011a). PRESTo, the Earthquake Early Warning System for Southern Italy: Concepts, Capabilities and Future Perspectives. Soil Dyn. Earthquake Eng. 31, 137–153. doi:10.1016/j.soildyn.2010.06.008
Satriano, C., Wu, Y.-M., Zollo, A., and Kanamori, H. (2011b). Earthquake Early Warning: Concepts, Methods and Physical Grounds. Soil Dyn. Earthquake Eng. 31, 106–118. doi:10.1016/j.soildyn.2010.07.007
SISMIKO (2020). Coordinamento Delle Reti Sismiche mobile in Emergenza. Available at: http://sismiko.ingv.it (Accessed March 25, 2021).
Suarez, G., Novelo, D., and Mansilla, E. (2009). Performance Evaluation of the Seismic Alert System (SAS) in Mexico City: A Seismological and a Social Perspective. Seismological Res. Lett. 80 (5), 707–716. doi:10.1785/gssrl.80.5.707
Velazquez, O., Pescaroli, G., Cremen, G., and Galasso, C. (2020). A Review of the Technical and Socio-Organizational Components of Earthquake Early Warning Systems. Front. Earth Sci. 8, 533498. doi:10.3389/feart.2020.533498
Wu, Y.-M., and Teng, T. L. (2002). A Virtual Subnetwork Approach to Earthquake Early Warning. Bull. Seismological Soc. America 92 (5), 2008–2018. doi:10.1785/0120010217
Zollo, A., Amoroso, O., Lancieri, M., Wu, Y.-M., and Kanamori, H. (2010). A Threshold-Based Earthquake Early Warning Using Dense Accelerometer Networks. Geophys. J. Int. 183, 963–974. doi:10.1111/j.1365-246X.2010.04765.x
Zollo, A., Colombelli, S., Elia, L., Emolo, A., Festa, G., Iannaccone, G., et al. (2014). “An Integrated Regional and On-Site Earthquake Early Warning System for Southern Italy: Concepts, Methodologies and Performances,” in Book: Early Warning for Geological Disasters, Advanced Technologies in Earth Sciences , 117–137. doi:10.1007/978-3-642-12233-0_7
Keywords: earthquakes, early warning, seismic networks, seismic risk reduction, simulation
Citation: Ladina C, Marzorati S, Amato A and Cattaneo M (2021) Feasibility Study of an Earthquake Early Warning System in Eastern Central Italy. Front. Earth Sci. 9:685751. doi: 10.3389/feart.2021.685751
Received: 25 March 2021; Accepted: 05 July 2021; Published: 20 August 2021.
Reviewed by:
Copyright © 2021 Ladina, Marzorati, Amato and Cattaneo. This is an open-access article distributed under the terms of the Creative Commons Attribution License (CC BY). The use, distribution or reproduction in other forums is permitted, provided the original author(s) and the copyright owner(s) are credited and that the original publication in this journal is cited, in accordance with accepted academic practice. No use, distribution or reproduction is permitted which does not comply with these terms.
*Correspondence: Chiara Ladina, [email protected]
Disclaimer: All claims expressed in this article are solely those of the authors and do not necessarily represent those of their affiliated organizations, or those of the publisher, the editors and the reviewers. Any product that may be evaluated in this article or claim that may be made by its manufacturer is not guaranteed or endorsed by the publisher.
Academia.edu no longer supports Internet Explorer.
To browse Academia.edu and the wider internet faster and more securely, please take a few seconds to upgrade your browser .
Enter the email address you signed up with and we'll email you a reset link.
- We're Hiring!
- Help Center
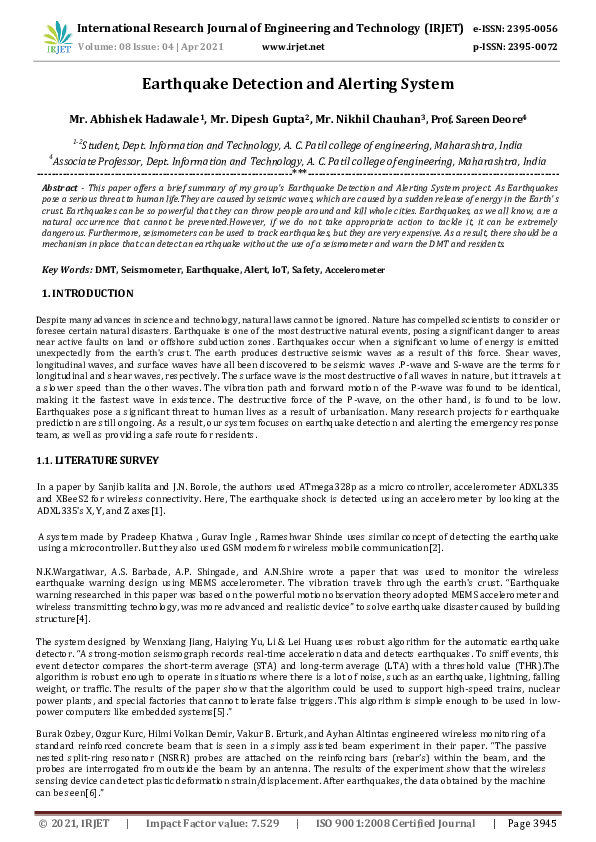
Earthquake Detection and Alerting System

Related Papers
IRJET Journal
This paper offers a brief summary of my group's Earthquake Detection and Alerting System project. As Earthquakes pose a serious threat to human life.They are caused by seismic waves, which are caused by a sudden release of energy in the Earth's crust. Earthquakes can be so powerful that they can throw people around and kill whole cities. Earthquakes, as we all know, are a natural occurrence that cannot be prevented.However, if we do not take appropriate action to tackle it, it can be extremely dangerous. Furthermore, seismometers can be used to track earthquakes, but they are very expensive. As a result, there should be a mechanism in place that can detect an earthquake without the use of a seismometer and warn the DMT and residents.
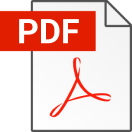
Internets of Things-enabled Intelligent Transportation Systems (ITS) are gaining significant attention in academic literature and industry, and are seen as an answer to enhancing road safety in smart cities. Due to the ever increasing number of vehicles, a big rise within the number of road accidents has been observed. Vehicles embedded with a plethora of sensors enable us to not only monitor the present situation of the vehicle and its surroundings but also facilitates the detection of incidents. Significant research, for instance, has been conducted on accident rescue, particularly on the utilization of data and Communication Technologies (ICT) for efficient and prompt rescue operations. The majority of such works provide sophisticated solutions that specialise in reducing response times. However, such solutions are often expensive and aren't available altogether sorts of vehicles. Given this, we present a completely unique Internet of Things-based accident detection and reporting system for a sensible city environment. The proposed approach aims to require advantage of advanced specifications of smartphones to style and develop a low-cost solution for enhanced transportation systems that's deployable in legacy vehicles. In this context, a customized Android application is developed to collect information regarding speed, gravity, pressure, sound, and site. The speed is a factor that is used to help improve the identification of accidents. It arises due to clear differences in environmental conditions (e.g., noise, deceleration rate) that arise in low speed collisions, versus higher speed collisions).The information acquired is further processed to detect road incidents. Furthermore, a navigation system is additionally developed to report the incident to the closest hospital. The proposed approach is validated through simulations and comparison with a true data set of road accidents acquired from Road Safety Open Repository, and shows promising leads to terms of accuracy.
Natural disasters such as earth quake and flood etc have very harmful effects lively hood and even loss of life. There are lot of incident took place in past decades one of such incident which as took place in recent time in India Up flood due over flow of Ganga river which has effected severely and many people lost their life the incident of Jharkhand earth quake also took place in recent times so to avoid such situation we implemented a system where the location is displayed on Google map and data is recorded in blynk app and alerting is done through buzzer here we can see demonstration of both earth quake and flood when the sensor observes or senses the vibrations the earth quake is detected notification is sent to blynk app and water level rises from the dam the sensor senses up to the level the water level has been increased which is recorded in graph form in blynk app which we can demonstrate using glass by pouring water level at different level hence the alerting is done prior to the event so there is less chances of risk to the life and whole system works on Wi-Fi model. The proposed system which we have implemented in this course of time consist of the two sensors MEMS and ultrasonic sensors most commonly called as flood sensors, GPS, Wi-Fi module, LCD display and Arduino
One of the prime reasons for vehicular accidents is due to undetected potholes and road humps. Well maintained roads contribute a major portion to the country's economy. The importance of road infrastructure society could be compared with importance of blood vessels for humans. To ensure road surface quality it should be monitored continuously and repaired as necessary In this paper ultrasonic sensor are used to identify potholes and humps to measure their depth and height respectively. This provides a prototype of an IoT based potholes and hump detection system that can be integrated with the vehicle and provide timely information to maintenance authorities so that necessary steps can be taken for safety of drivers.
In the present time internet becomes an essential need and without it we can't imagine our lifestyle. This paper talks about a smart military base which is design to upgrade the defence security of our military. It collects data from various sensors fitted inside it and send it to the cloud storage having IP address 184.106.153.149. We use ESP8266 WIFI module for uploading the data on the cloud in real time and Arduino uno microcontroller board to control all the sensors and modules. We can observe the movement ,temperature, flammable or toxic gases present in the air and humidity inside the base from any corner of the world. This project is based on the concept of internet of things(IoT).
The objective of the smart helmet is to provide a means and apparatus for detecting and reporting accidents. Sensors, and cloud computing infrastructures are utilised for building the system. The accident detection system communicates the accelerometer values to the processor which continuously monitors for erratic variations. When an accident occurs, the related details are sent to the emergency contacts by utilizing a cloud based service. The vehicle location is obtained by making use of the global positioning system. The system promises a reliable and quick delivery of information relating to the accident in real time and up dated to cloud which are accessed by IOT. Thus, by making use of the ubiquitous connectivity which is a salient feature for the smart cities, a smart helmet for accident detection is built.
The Internet of Things (IoT) is assuming an imperative part in our day by day lives all together to accomplish assignments by in corporating the utilization of sensor networks including our current circumstance. The frameworks created to notice the actual inclination that makes information furthermore, the made information is put away in the cloud. The put away data is then used for planning applications for controlling important activities. This paper describes the application and experimentation of a framework made out of sensors for checking temperature and stickiness of the zone encompassing. This noticed data is used to perform transient activities, for example, controlling the electronic contraptions for warming or cooling that takes additional time. The recorded information is stacked to cloud for capacity and further accessed through an Android application and showcases the results to the versatile clients. The system introduced in this paper utilized Arduino UNO board, DHT11 sensor, soil moisture sensor, ESP8266 Wi-Fi module, which makes data to open IoT based API organization Thing Speak through which it is examined and kept aside. An Android application is made which gets to the cloud also, shows results for end-customers through REST API Web organization. The exploratory outcomes shown demonstrate the adequacy of the framework.
The loss of properties and living population is getting enhanced by every year due to the dynamic alterations in weather conditions which results in heavy floods. Therefore, implementation of an intelligent analysis of flood risk is necessitated for the field of research in Disaster management. This project implements an intelligent IoT-based flood monitoring and alerting system using Raspberry Pi model, where water sensors and rain sensors are utilized to alert the authorities regarding the heaviness of rain and monitoring of water level in a lake or river. This system alerts the people in nearby villages since it utilizes IoT system for notifying the village people.
Healthcare is given the major importance now a-days in all the countries with the advent of the chronic diseases. This work provides better solution for remote health monitoring with affordable cost using latest technologies. IoT is the fast-growing technology in every sector. With the integration of IoT and sensors healthcare monitoring can be brought to such a pace. Sensor readout are carried out using LabVIEW; a virtual instrumentation software. The results will be copied in the IoT server and then it will be analyzed. If it is in normal condition the report will be forwarded to the patient or if it is critical the results will be sent to the healthcare immediately, also ambulance can be called automatically without human intervention, if necessary. This ensures that proper treatment is provided to the patient at the early stage. The health will be monitored continuously and properly diagnosed of the state of patient health. It reduces the complexity, the cost, the waiting time and the death rates. These devices can be installed at any remote location, even at work environment, and can be operated without the help of medical personal. This work focuses on fast-track generation of report and reaching the doctor even before the patient arrive.
There are a couple of spots that are more disposed to flooding than various spots; the execution of flood-prepared systems near any critical water an area or stream gives fundamental data that can ensure property and save lives. Clearly, the best flood alerted techniques are extreme and need high help, and require incredibly qualified delegates to work it. These days, there is no thought regarding when floods will happen, so there is a need to caution individuals who are close to the overwhelmed region. Hence, we are arranging this system to enlighten people about the approaching flood through notice and prepared messages. Sending real time data to the users who have installed the app and also providing a direction details to the users within the app. For that reason, we will utilize a few sensors that will assist with giving data about the flood. This framework gives genuine execution to affiliations, associations, and people excited about creating and working flood seeing and cautioning structures. This undertaking likewise incorporates a Sensor-Based Water Quality Monitoring System, which is used for assessing physical and substance boundaries of the water. The boundaries, for instance, Temperature, pH, flex sensor and water level, and nature of the water, can be assessed. This sensor data is essential for quality monitoring of the flood alerting system
Loading Preview
Sorry, preview is currently unavailable. You can download the paper by clicking the button above.
RELATED PAPERS
Rinku Bhagat
- We're Hiring!
- Help Center
- Find new research papers in:
- Health Sciences
- Earth Sciences
- Cognitive Science
- Mathematics
- Computer Science
- Academia ©2024
New horizon in the statistical physics of earthquakes: Dragon-king theory and dragon-king earthquakes
- Sornette, Didier
- Wu, Zhongliang
- Li, Hangwei
A systematic quantitative investigation into whether the mechanisms of large earthquakes are unique could significantly deepen our understanding of fault rupture and seismicity patterns. This research holds the potential to advance our ability to predict large earthquakes and enhance the effectiveness of disaster prevention and mitigation strategies. In 2009, one of us introduced the dragon-king theory, offering a quantitative framework for identifying and testing extreme outliers-referred to as dragon-king events-that are endogenously generated. This theory provides valuable tools for explaining, predicting, and managing the risks associated with these rare but highly impactful events. The present paper discusses the feasibility of applying this theory to seismology, proposing that dragon-king earthquake events can be identified as outliers to the Gutenberg-Richter law. It also examines several seismological mechanisms that may contribute to the occurrence of these extraordinary events. Although applying the dragon-king theory to seismology presents practical challenges, it offers the potential to significantly enrich statistical seismology. By reexamining the classification of earthquake rupture types through a statistical testing lens and integrating these insights with underlying physical mechanisms, this approach can greatly enhance the analytical tools and depth of research in the field of statistical seismology.
- Physics - Geophysics;
- Physics - Data Analysis;
- Statistics and Probability

IMAGES
COMMENTS
1 Introduction. Earthquake early warning systems (EEWSs) are shifting earthquake science toward real-time event detection and data processing [Kuyuk and Allen, 2013a, 2013b; Kuyuk et al., 2014; Satriano et al., 2010].These systems are minimizing the time needed to calculate source parameters of earthquakes (essentially, location, and magnitude) to within a few seconds of their occurrence.
This paper is supported by the European Union 's Horizon 2020 research and innovation programme under grant agreement No 821046, project TURNkey (Towards more Earthquake-resilient Urban Societies through a Multi-sensor-based Information System enabling Earthquake Forecasting, Early Warning and Rapid Response actions).
Early Earthquake Warning (EEW) systems alarm about ongoing earthquakes to reduce their devastating human and financial damages. In complicated tasks like earthquake forecasting, Artificial Intelligence (AI) solutions show promising results. The goal of this review is to investigate the AI-based EEW systems. Web of Science, Scopus, Embase, and PubMed databases were systematically searched from ...
Earthquake Monitoring and Early Warning Systems, Figure 2. Location of the 4 m ost deadly earthquakes of the 21st century (up to the end of 2007) o n a map showing the l ocation of the deadl y ...
Recently, mobile devices such as smartphones and IoT devices have been successfully used for detecting earthquakes using their built-in accelerometers. To utilize such devices as a seismic sensor, there are two approaches including a standalone or a client-server approach. The client-server approach is more accurate than the stand-alone approach but requires high-performance servers and ...
1 Joint Centre for Disaster Research, Massey University, Wellington, New Zealand; 2 Department of Civil and Environmental Engineering, The University of Auckland, Auckland, New Zealand; Earthquake early warning system (EEWS) plays an important role in detecting ground shaking during an earthquake and alerting the public and authorities to take appropriate safety measures, reducing possible ...
Earthquake early warning (EEW) is the delivery of ground shaking alerts or warnings. It is distinguished from earthquake prediction in that the earthquake has nucleated to provide detectable ground motion when an EEW is issued. Here we review progress in the field in the last 10 years. We begin with EEW users, synthesizing what we now know about who uses EEW and what information they need and ...
Earthquake early warning systems (EEWS) are crucial for saving lives in earthquake-prone areas. In this study, we explore the potential of IoT and cloud infrastructure in realizing a sustainable EEWS that is capable of providing early warning to people and coordinating disaster response efforts. To achieve this goal, we provide an overview of the fundamental concepts of seismic waves and ...
The P-Alert Sensors and EEW. The research group at NTU worked in close association with a technology company in Taiwan for the development of a low-cost, MEMS-based, P-wave alert device named "P-Alert". These MEMS-based sensors are embedded in a small housing and can record high-frequency, near-source ground-motion.
Earthquakes are disasters that threaten the operational safety of high-speed railways. To obtain reliable alerts for the earthquake monitoring and early warning systems of high-speed railways, based on magnitude and peak ground acceleration (PGA) thresholds (M = 5.5 and PGA = 40 cm/s 2), an earthquake early warning (EEW) method for high-speed railways using deep learning is proposed.
With the gradual development of and improvement in earthquake early warning systems (EEWS), more accurate real-time seismic intensity measurements (IMs) methods are needed to assess the impact range of earthquake intensities. Although traditional point source warning systems have made some progress in terms of predicting earthquake source parameters, they are still inadequate at assessing the ...
An earthquake early warning system (EEWS) is a monitoring infrastructure that allows alerting strategic points (targets) before the arrival of strong shaking waves during an earthquake. In a region like Central Italy, struck by recent and historical destructive earthquakes, the assessment of implementation of an EEWS is a significant challenge ...
Advance Development of Warning Alarm System for Earthquake Using MEMS Sensor. December 2018. Indian Journal of Public Health Research and Development 9 (12):1553. DOI: 10.5958/0976-5506.2018.02077 ...
This paper presents a new copula-based earthquake early warning (EEW) decision-making strategy, aiming to characterize missed-alarm and false-alarm probabilities for an on-site EEW and determine ...
The aims of review paper are: i). Review the vary keywords used in research. ii). Studies the different types of earthquakes detector that are appropriate. iii). Review the effectiveness of earthquake detectors and the effects of installation on environment. iv). Examination the output produced by the earthquake detectors through the created or ...
IJSDR2308020 International Journal of Scientific Development and Research (IJSDR) www.ijsdr.org 136 Development of wireless earthquake alarm system for early warning 1Er. Abrar Ahmad Nath, 2Dushyanth V Babu R, 3Dr Dasarathy A K 1M.Tech. Student, 2Assistant Professor, 3Head of Department Department of Civil Engineering
The paper presents the results of an ongoing research project on the performance of the SASMEX system following the two earthquakes that hit Mexico City in 2017, (a) the 7 September, and (b) the ...
HISTORY OF EARTHQUAKE ALARM SYSTEMS Fig. 1 shows a history of research and development concerning earthquake disaster prevention systems in Japan railways. The first main system is an automatic train control system developed when Tokaido Shinkansen began operating. In this system, mechanical alarm seismographs, which were newly developed, were ...
ElarmS, or Earthquake Alarm Systems, can provide warning of ground shaking during an earthquake. The objective is to rapidly detect the initiation of an earthquake, estimate the level of ground shaking to be expected, and issue a warning before significant ground shaking starts. This can be done by detecting the first energy to radiate from an ...
IRJET Journal. This paper offers a brief summary of my group's Earthquake Detection and Alerting System project. As Earthquakes pose a serious threat to human life.They are caused by seismic waves, which are caused by a sudden release of energy in the Earth's crust. Earthquakes can be so powerful that they can throw people around and kill whole ...
Earthquake Alarm Detector Microcontroller based Circuit for issuing Warning for vibration in Steel Foundations October 2017 International Journal of Mechanical & Mechatronics Engineering 7(26 ...
IRE 1700159 ICONIC RESEARCH AND ENGINEERING JOURNALS 124 Earthquake Alarm System Based on Advanced Wireless GSM Modem PROF. PRADEEP KHATWA 1, GAURAV INGLE 2, RAMESHHWAR SHINDE 3, AJAY SANE4 1,2,3,4 Dr. D.Y.Patil School Of Engineering, Pune Abstract -- Many life and properties have been lost due to the earthquake.
A systematic quantitative investigation into whether the mechanisms of large earthquakes are unique could significantly deepen our understanding of fault rupture and seismicity patterns. This research holds the potential to advance our ability to predict large earthquakes and enhance the effectiveness of disaster prevention and mitigation strategies. In 2009, one of us introduced the dragon ...
This study shows the development of an earthquake detector unit system using Arduino Mega and ADXL335 accelerometer. The alarm system will be triggered and will give a sound when the ...