Have a language expert improve your writing
Run a free plagiarism check in 10 minutes, generate accurate citations for free.
- Knowledge Base
Methodology
- Quasi-Experimental Design | Definition, Types & Examples

Quasi-Experimental Design | Definition, Types & Examples
Published on July 31, 2020 by Lauren Thomas . Revised on January 22, 2024.
Like a true experiment , a quasi-experimental design aims to establish a cause-and-effect relationship between an independent and dependent variable .
However, unlike a true experiment, a quasi-experiment does not rely on random assignment . Instead, subjects are assigned to groups based on non-random criteria.
Quasi-experimental design is a useful tool in situations where true experiments cannot be used for ethical or practical reasons.
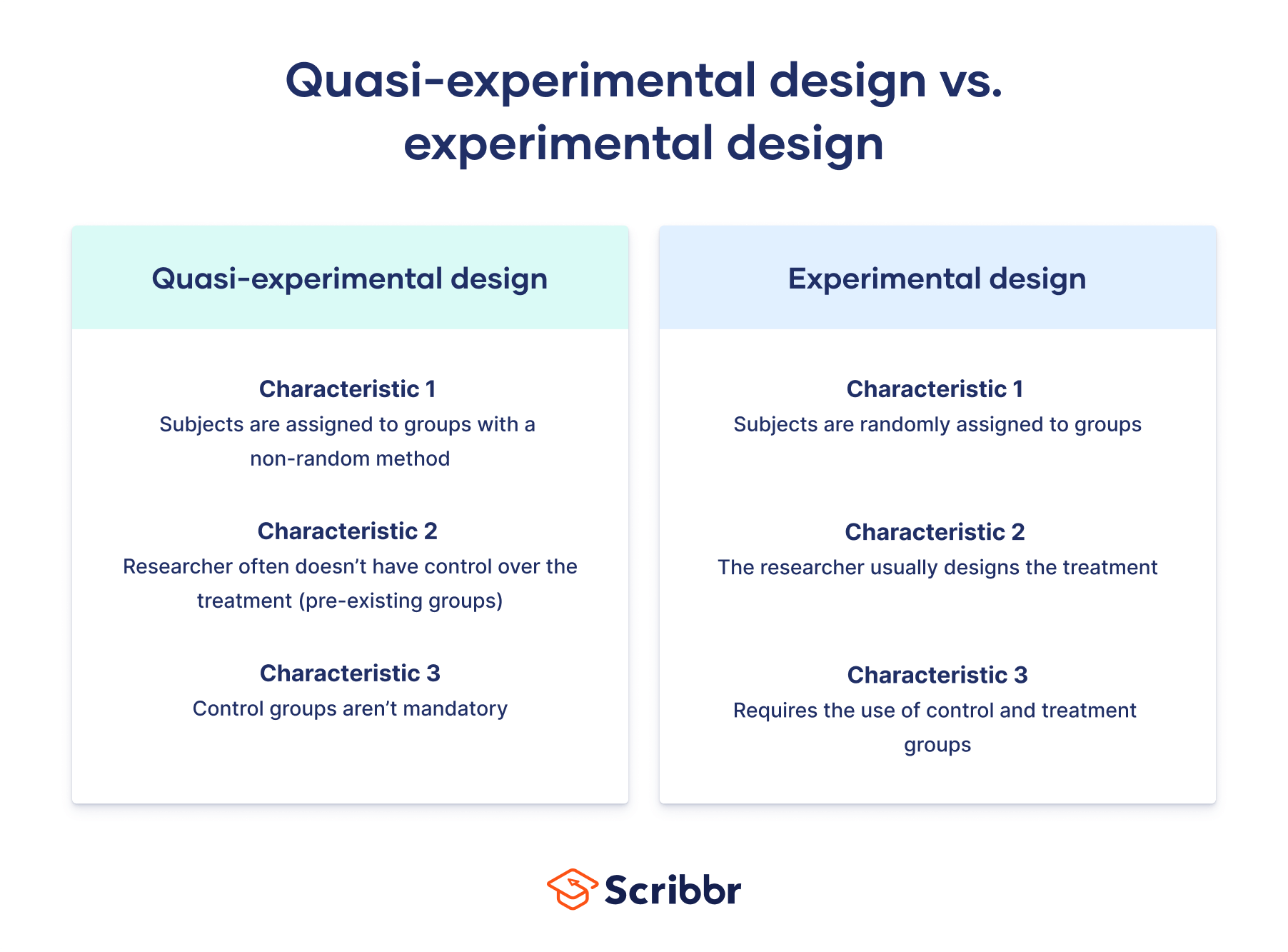
Table of contents
Differences between quasi-experiments and true experiments, types of quasi-experimental designs, when to use quasi-experimental design, advantages and disadvantages, other interesting articles, frequently asked questions about quasi-experimental designs.
There are several common differences between true and quasi-experimental designs.
True experimental design | Quasi-experimental design | |
---|---|---|
Assignment to treatment | The researcher subjects to control and treatment groups. | Some other, method is used to assign subjects to groups. |
Control over treatment | The researcher usually . | The researcher often , but instead studies pre-existing groups that received different treatments after the fact. |
Use of | Requires the use of . | Control groups are not required (although they are commonly used). |
Example of a true experiment vs a quasi-experiment
However, for ethical reasons, the directors of the mental health clinic may not give you permission to randomly assign their patients to treatments. In this case, you cannot run a true experiment.
Instead, you can use a quasi-experimental design.
You can use these pre-existing groups to study the symptom progression of the patients treated with the new therapy versus those receiving the standard course of treatment.
Receive feedback on language, structure, and formatting
Professional editors proofread and edit your paper by focusing on:
- Academic style
- Vague sentences
- Style consistency
See an example
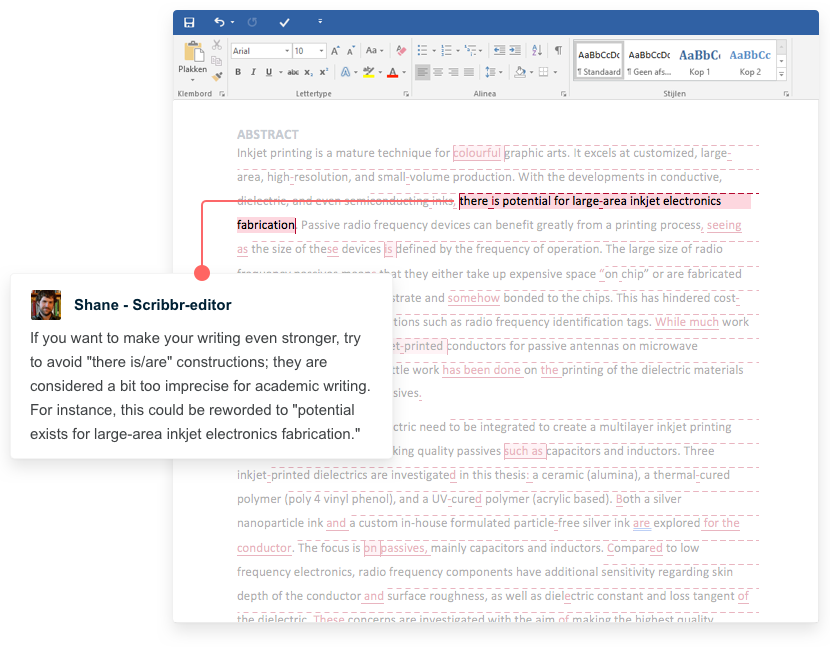
Many types of quasi-experimental designs exist. Here we explain three of the most common types: nonequivalent groups design, regression discontinuity, and natural experiments.
Nonequivalent groups design
In nonequivalent group design, the researcher chooses existing groups that appear similar, but where only one of the groups experiences the treatment.
In a true experiment with random assignment , the control and treatment groups are considered equivalent in every way other than the treatment. But in a quasi-experiment where the groups are not random, they may differ in other ways—they are nonequivalent groups .
When using this kind of design, researchers try to account for any confounding variables by controlling for them in their analysis or by choosing groups that are as similar as possible.
This is the most common type of quasi-experimental design.
Regression discontinuity
Many potential treatments that researchers wish to study are designed around an essentially arbitrary cutoff, where those above the threshold receive the treatment and those below it do not.
Near this threshold, the differences between the two groups are often so minimal as to be nearly nonexistent. Therefore, researchers can use individuals just below the threshold as a control group and those just above as a treatment group.
However, since the exact cutoff score is arbitrary, the students near the threshold—those who just barely pass the exam and those who fail by a very small margin—tend to be very similar, with the small differences in their scores mostly due to random chance. You can therefore conclude that any outcome differences must come from the school they attended.
Natural experiments
In both laboratory and field experiments, researchers normally control which group the subjects are assigned to. In a natural experiment, an external event or situation (“nature”) results in the random or random-like assignment of subjects to the treatment group.
Even though some use random assignments, natural experiments are not considered to be true experiments because they are observational in nature.
Although the researchers have no control over the independent variable , they can exploit this event after the fact to study the effect of the treatment.
However, as they could not afford to cover everyone who they deemed eligible for the program, they instead allocated spots in the program based on a random lottery.
Although true experiments have higher internal validity , you might choose to use a quasi-experimental design for ethical or practical reasons.
Sometimes it would be unethical to provide or withhold a treatment on a random basis, so a true experiment is not feasible. In this case, a quasi-experiment can allow you to study the same causal relationship without the ethical issues.
The Oregon Health Study is a good example. It would be unethical to randomly provide some people with health insurance but purposely prevent others from receiving it solely for the purposes of research.
However, since the Oregon government faced financial constraints and decided to provide health insurance via lottery, studying this event after the fact is a much more ethical approach to studying the same problem.
True experimental design may be infeasible to implement or simply too expensive, particularly for researchers without access to large funding streams.
At other times, too much work is involved in recruiting and properly designing an experimental intervention for an adequate number of subjects to justify a true experiment.
In either case, quasi-experimental designs allow you to study the question by taking advantage of data that has previously been paid for or collected by others (often the government).
Quasi-experimental designs have various pros and cons compared to other types of studies.
- Higher external validity than most true experiments, because they often involve real-world interventions instead of artificial laboratory settings.
- Higher internal validity than other non-experimental types of research, because they allow you to better control for confounding variables than other types of studies do.
- Lower internal validity than true experiments—without randomization, it can be difficult to verify that all confounding variables have been accounted for.
- The use of retrospective data that has already been collected for other purposes can be inaccurate, incomplete or difficult to access.
If you want to know more about statistics , methodology , or research bias , make sure to check out some of our other articles with explanations and examples.
- Normal distribution
- Degrees of freedom
- Null hypothesis
- Discourse analysis
- Control groups
- Mixed methods research
- Non-probability sampling
- Quantitative research
- Ecological validity
Research bias
- Rosenthal effect
- Implicit bias
- Cognitive bias
- Selection bias
- Negativity bias
- Status quo bias
A quasi-experiment is a type of research design that attempts to establish a cause-and-effect relationship. The main difference with a true experiment is that the groups are not randomly assigned.
In experimental research, random assignment is a way of placing participants from your sample into different groups using randomization. With this method, every member of the sample has a known or equal chance of being placed in a control group or an experimental group.
Quasi-experimental design is most useful in situations where it would be unethical or impractical to run a true experiment .
Quasi-experiments have lower internal validity than true experiments, but they often have higher external validity as they can use real-world interventions instead of artificial laboratory settings.
Cite this Scribbr article
If you want to cite this source, you can copy and paste the citation or click the “Cite this Scribbr article” button to automatically add the citation to our free Citation Generator.
Thomas, L. (2024, January 22). Quasi-Experimental Design | Definition, Types & Examples. Scribbr. Retrieved August 14, 2024, from https://www.scribbr.com/methodology/quasi-experimental-design/
Is this article helpful?
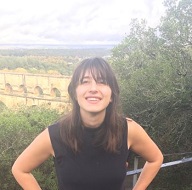
Lauren Thomas
Other students also liked, guide to experimental design | overview, steps, & examples, random assignment in experiments | introduction & examples, control variables | what are they & why do they matter, "i thought ai proofreading was useless but..".
I've been using Scribbr for years now and I know it's a service that won't disappoint. It does a good job spotting mistakes”
- Skip to secondary menu
- Skip to main content
- Skip to primary sidebar
Statistics By Jim
Making statistics intuitive
Quasi Experimental Design Overview & Examples
By Jim Frost Leave a Comment
What is a Quasi Experimental Design?
A quasi experimental design is a method for identifying causal relationships that does not randomly assign participants to the experimental groups. Instead, researchers use a non-random process. For example, they might use an eligibility cutoff score or preexisting groups to determine who receives the treatment.

Quasi-experimental research is a design that closely resembles experimental research but is different. The term “quasi” means “resembling,” so you can think of it as a cousin to actual experiments. In these studies, researchers can manipulate an independent variable — that is, they change one factor to see what effect it has. However, unlike true experimental research, participants are not randomly assigned to different groups.
Learn more about Experimental Designs: Definition & Types .
When to Use Quasi-Experimental Design
Researchers typically use a quasi-experimental design because they can’t randomize due to practical or ethical concerns. For example:
- Practical Constraints : A school interested in testing a new teaching method can only implement it in preexisting classes and cannot randomly assign students.
- Ethical Concerns : A medical study might not be able to randomly assign participants to a treatment group for an experimental medication when they are already taking a proven drug.
Quasi-experimental designs also come in handy when researchers want to study the effects of naturally occurring events, like policy changes or environmental shifts, where they can’t control who is exposed to the treatment.
Quasi-experimental designs occupy a unique position in the spectrum of research methodologies, sitting between observational studies and true experiments. This middle ground offers a blend of both worlds, addressing some limitations of purely observational studies while navigating the constraints often accompanying true experiments.
A significant advantage of quasi-experimental research over purely observational studies and correlational research is that it addresses the issue of directionality, determining which variable is the cause and which is the effect. In quasi-experiments, an intervention typically occurs during the investigation, and the researchers record outcomes before and after it, increasing the confidence that it causes the observed changes.
However, it’s crucial to recognize its limitations as well. Controlling confounding variables is a larger concern for a quasi-experimental design than a true experiment because it lacks random assignment.
In sum, quasi-experimental designs offer a valuable research approach when random assignment is not feasible, providing a more structured and controlled framework than observational studies while acknowledging and attempting to address potential confounders.
Types of Quasi-Experimental Designs and Examples
Quasi-experimental studies use various methods, depending on the scenario.
Natural Experiments
This design uses naturally occurring events or changes to create the treatment and control groups. Researchers compare outcomes between those whom the event affected and those it did not affect. Analysts use statistical controls to account for confounders that the researchers must also measure.
Natural experiments are related to observational studies, but they allow for a clearer causality inference because the external event or policy change provides both a form of quasi-random group assignment and a definite start date for the intervention.
For example, in a natural experiment utilizing a quasi-experimental design, researchers study the impact of a significant economic policy change on small business growth. The policy is implemented in one state but not in neighboring states. This scenario creates an unplanned experimental setup, where the state with the new policy serves as the treatment group, and the neighboring states act as the control group.
Researchers are primarily interested in small business growth rates but need to record various confounders that can impact growth rates. Hence, they record state economic indicators, investment levels, and employment figures. By recording these metrics across the states, they can include them in the model as covariates and control them statistically. This method allows researchers to estimate differences in small business growth due to the policy itself, separate from the various confounders.
Nonequivalent Groups Design
This method involves matching existing groups that are similar but not identical. Researchers attempt to find groups that are as equivalent as possible, particularly for factors likely to affect the outcome.
For instance, researchers use a nonequivalent groups quasi-experimental design to evaluate the effectiveness of a new teaching method in improving students’ mathematics performance. A school district considering the teaching method is planning the study. Students are already divided into schools, preventing random assignment.
The researchers matched two schools with similar demographics, baseline academic performance, and resources. The school using the traditional methodology is the control, while the other uses the new approach. Researchers are evaluating differences in educational outcomes between the two methods.
They perform a pretest to identify differences between the schools that might affect the outcome and include them as covariates to control for confounding. They also record outcomes before and after the intervention to have a larger context for the changes they observe.
Regression Discontinuity
This process assigns subjects to a treatment or control group based on a predetermined cutoff point (e.g., a test score). The analysis primarily focuses on participants near the cutoff point, as they are likely similar except for the treatment received. By comparing participants just above and below the cutoff, the design controls for confounders that vary smoothly around the cutoff.
For example, in a regression discontinuity quasi-experimental design focusing on a new medical treatment for depression, researchers use depression scores as the cutoff point. Individuals with depression scores just above a certain threshold are assigned to receive the latest treatment, while those just below the threshold do not receive it. This method creates two closely matched groups: one that barely qualifies for treatment and one that barely misses out.
By comparing the mental health outcomes of these two groups over time, researchers can assess the effectiveness of the new treatment. The assumption is that the only significant difference between the groups is whether they received the treatment, thereby isolating its impact on depression outcomes.
Controlling Confounders in a Quasi-Experimental Design
Accounting for confounding variables is a challenging but essential task for a quasi-experimental design.
In a true experiment, the random assignment process equalizes confounders across the groups to nullify their overall effect. It’s the gold standard because it works on all confounders, known and unknown.
Unfortunately, the lack of random assignment can allow differences between the groups to exist before the intervention. These confounding factors might ultimately explain the results rather than the intervention.
Consequently, researchers must use other methods to equalize the groups roughly using matching and cutoff values or statistically adjust for preexisting differences they measure to reduce the impact of confounders.
A key strength of quasi-experiments is their frequent use of “pre-post testing.” This approach involves conducting initial tests before collecting data to check for preexisting differences between groups that could impact the study’s outcome. By identifying these variables early on and including them as covariates, researchers can more effectively control potential confounders in their statistical analysis.
Additionally, researchers frequently track outcomes before and after the intervention to better understand the context for changes they observe.
Statisticians consider these methods to be less effective than randomization. Hence, quasi-experiments fall somewhere in the middle when it comes to internal validity , or how well the study can identify causal relationships versus mere correlation . They’re more conclusive than correlational studies but not as solid as true experiments.
In conclusion, quasi-experimental designs offer researchers a versatile and practical approach when random assignment is not feasible. This methodology bridges the gap between controlled experiments and observational studies, providing a valuable tool for investigating cause-and-effect relationships in real-world settings. Researchers can address ethical and logistical constraints by understanding and leveraging the different types of quasi-experimental designs while still obtaining insightful and meaningful results.
Cook, T. D., & Campbell, D. T. (1979). Quasi-experimentation: Design & analysis issues in field settings . Boston, MA: Houghton Mifflin
Share this:

Reader Interactions
Comments and questions cancel reply.
Experimental vs Quasi-Experimental Design: Which to Choose?
Here’s a table that summarizes the similarities and differences between an experimental and a quasi-experimental study design:
Experimental Study (a.k.a. Randomized Controlled Trial) | Quasi-Experimental Study | |
---|---|---|
Objective | Evaluate the effect of an intervention or a treatment | Evaluate the effect of an intervention or a treatment |
How participants get assigned to groups? | Random assignment | Non-random assignment (participants get assigned according to their choosing or that of the researcher) |
Is there a control group? | Yes | Not always (although, if present, a control group will provide better evidence for the study results) |
Is there any room for confounding? | No (although check for a detailed discussion on post-randomization confounding in randomized controlled trials) | Yes (however, statistical techniques can be used to study causal relationships in quasi-experiments) |
Level of evidence | A randomized trial is at the highest level in the hierarchy of evidence | A quasi-experiment is one level below the experimental study in the hierarchy of evidence [ ] |
Advantages | Minimizes bias and confounding | – Can be used in situations where an experiment is not ethically or practically feasible – Can work with smaller sample sizes than randomized trials |
Limitations | – High cost (as it generally requires a large sample size) – Ethical limitations – Generalizability issues – Sometimes practically infeasible | Lower ranking in the hierarchy of evidence as losing the power of randomization causes the study to be more susceptible to bias and confounding |
What is a quasi-experimental design?
A quasi-experimental design is a non-randomized study design used to evaluate the effect of an intervention. The intervention can be a training program, a policy change or a medical treatment.
Unlike a true experiment, in a quasi-experimental study the choice of who gets the intervention and who doesn’t is not randomized. Instead, the intervention can be assigned to participants according to their choosing or that of the researcher, or by using any method other than randomness.
Having a control group is not required, but if present, it provides a higher level of evidence for the relationship between the intervention and the outcome.
(for more information, I recommend my other article: Understand Quasi-Experimental Design Through an Example ) .
Examples of quasi-experimental designs include:
- One-Group Posttest Only Design
- Static-Group Comparison Design
- One-Group Pretest-Posttest Design
- Separate-Sample Pretest-Posttest Design
What is an experimental design?
An experimental design is a randomized study design used to evaluate the effect of an intervention. In its simplest form, the participants will be randomly divided into 2 groups:
- A treatment group: where participants receive the new intervention which effect we want to study.
- A control or comparison group: where participants do not receive any intervention at all (or receive some standard intervention).
Randomization ensures that each participant has the same chance of receiving the intervention. Its objective is to equalize the 2 groups, and therefore, any observed difference in the study outcome afterwards will only be attributed to the intervention – i.e. it removes confounding.
(for more information, I recommend my other article: Purpose and Limitations of Random Assignment ).
Examples of experimental designs include:
- Posttest-Only Control Group Design
- Pretest-Posttest Control Group Design
- Solomon Four-Group Design
- Matched Pairs Design
- Randomized Block Design
When to choose an experimental design over a quasi-experimental design?
Although many statistical techniques can be used to deal with confounding in a quasi-experimental study, in practice, randomization is still the best tool we have to study causal relationships.
Another problem with quasi-experiments is the natural progression of the disease or the condition under study — When studying the effect of an intervention over time, one should consider natural changes because these can be mistaken with changes in outcome that are caused by the intervention. Having a well-chosen control group helps dealing with this issue.
So, if losing the element of randomness seems like an unwise step down in the hierarchy of evidence, why would we ever want to do it?
This is what we’re going to discuss next.
When to choose a quasi-experimental design over a true experiment?
The issue with randomness is that it cannot be always achievable.
So here are some cases where using a quasi-experimental design makes more sense than using an experimental one:
- If being in one group is believed to be harmful for the participants , either because the intervention is harmful (ex. randomizing people to smoking), or the intervention has a questionable efficacy, or on the contrary it is believed to be so beneficial that it would be malevolent to put people in the control group (ex. randomizing people to receiving an operation).
- In cases where interventions act on a group of people in a given location , it becomes difficult to adequately randomize subjects (ex. an intervention that reduces pollution in a given area).
- When working with small sample sizes , as randomized controlled trials require a large sample size to account for heterogeneity among subjects (i.e. to evenly distribute confounding variables between the intervention and control groups).
Further reading
- Statistical Software Popularity in 40,582 Research Papers
- Checking the Popularity of 125 Statistical Tests and Models
- Objectives of Epidemiology (With Examples)
- 12 Famous Epidemiologists and Why
Research Methodologies Guide
- Action Research
- Bibliometrics
- Case Studies
- Content Analysis
- Digital Scholarship This link opens in a new window
- Documentary
- Ethnography
- Focus Groups
- Grounded Theory
- Life Histories/Autobiographies
- Longitudinal
- Participant Observation
- Qualitative Research (General)
Quasi-Experimental Design
- Usability Studies
Quasi-Experimental Design is a unique research methodology because it is characterized by what is lacks. For example, Abraham & MacDonald (2011) state:
" Quasi-experimental research is similar to experimental research in that there is manipulation of an independent variable. It differs from experimental research because either there is no control group, no random selection, no random assignment, and/or no active manipulation. "
This type of research is often performed in cases where a control group cannot be created or random selection cannot be performed. This is often the case in certain medical and psychological studies.
For more information on quasi-experimental design, review the resources below:
Where to Start
Below are listed a few tools and online guides that can help you start your Quasi-experimental research. These include free online resources and resources available only through ISU Library.
- Quasi-Experimental Research Designs by Bruce A. Thyer This pocket guide describes the logic, design, and conduct of the range of quasi-experimental designs, encompassing pre-experiments, quasi-experiments making use of a control or comparison group, and time-series designs. An introductory chapter describes the valuable role these types of studies have played in social work, from the 1930s to the present. Subsequent chapters delve into each design type's major features, the kinds of questions it is capable of answering, and its strengths and limitations.
- Experimental and Quasi-Experimental Designs for Research by Donald T. Campbell; Julian C. Stanley. Call Number: Q175 C152e Written 1967 but still used heavily today, this book examines research designs for experimental and quasi-experimental research, with examples and judgments about each design's validity.
Online Resources
- Quasi-Experimental Design From the Web Center for Social Research Methods, this is a very good overview of quasi-experimental design.
- Experimental and Quasi-Experimental Research From Colorado State University.
- Quasi-experimental design--Wikipedia, the free encyclopedia Wikipedia can be a useful place to start your research- check the citations at the bottom of the article for more information.
- << Previous: Qualitative Research (General)
- Next: Sampling >>
- Last Updated: Aug 12, 2024 4:07 PM
- URL: https://instr.iastate.libguides.com/researchmethods
- Privacy Policy

Home » Quasi-Experimental Research Design – Types, Methods
Quasi-Experimental Research Design – Types, Methods
Table of Contents

Quasi-Experimental Design
Quasi-experimental design is a research method that seeks to evaluate the causal relationships between variables, but without the full control over the independent variable(s) that is available in a true experimental design.
In a quasi-experimental design, the researcher uses an existing group of participants that is not randomly assigned to the experimental and control groups. Instead, the groups are selected based on pre-existing characteristics or conditions, such as age, gender, or the presence of a certain medical condition.
Types of Quasi-Experimental Design
There are several types of quasi-experimental designs that researchers use to study causal relationships between variables. Here are some of the most common types:
Non-Equivalent Control Group Design
This design involves selecting two groups of participants that are similar in every way except for the independent variable(s) that the researcher is testing. One group receives the treatment or intervention being studied, while the other group does not. The two groups are then compared to see if there are any significant differences in the outcomes.
Interrupted Time-Series Design
This design involves collecting data on the dependent variable(s) over a period of time, both before and after an intervention or event. The researcher can then determine whether there was a significant change in the dependent variable(s) following the intervention or event.
Pretest-Posttest Design
This design involves measuring the dependent variable(s) before and after an intervention or event, but without a control group. This design can be useful for determining whether the intervention or event had an effect, but it does not allow for control over other factors that may have influenced the outcomes.
Regression Discontinuity Design
This design involves selecting participants based on a specific cutoff point on a continuous variable, such as a test score. Participants on either side of the cutoff point are then compared to determine whether the intervention or event had an effect.
Natural Experiments
This design involves studying the effects of an intervention or event that occurs naturally, without the researcher’s intervention. For example, a researcher might study the effects of a new law or policy that affects certain groups of people. This design is useful when true experiments are not feasible or ethical.
Data Analysis Methods
Here are some data analysis methods that are commonly used in quasi-experimental designs:
Descriptive Statistics
This method involves summarizing the data collected during a study using measures such as mean, median, mode, range, and standard deviation. Descriptive statistics can help researchers identify trends or patterns in the data, and can also be useful for identifying outliers or anomalies.
Inferential Statistics
This method involves using statistical tests to determine whether the results of a study are statistically significant. Inferential statistics can help researchers make generalizations about a population based on the sample data collected during the study. Common statistical tests used in quasi-experimental designs include t-tests, ANOVA, and regression analysis.
Propensity Score Matching
This method is used to reduce bias in quasi-experimental designs by matching participants in the intervention group with participants in the control group who have similar characteristics. This can help to reduce the impact of confounding variables that may affect the study’s results.
Difference-in-differences Analysis
This method is used to compare the difference in outcomes between two groups over time. Researchers can use this method to determine whether a particular intervention has had an impact on the target population over time.
Interrupted Time Series Analysis
This method is used to examine the impact of an intervention or treatment over time by comparing data collected before and after the intervention or treatment. This method can help researchers determine whether an intervention had a significant impact on the target population.
Regression Discontinuity Analysis
This method is used to compare the outcomes of participants who fall on either side of a predetermined cutoff point. This method can help researchers determine whether an intervention had a significant impact on the target population.
Steps in Quasi-Experimental Design
Here are the general steps involved in conducting a quasi-experimental design:
- Identify the research question: Determine the research question and the variables that will be investigated.
- Choose the design: Choose the appropriate quasi-experimental design to address the research question. Examples include the pretest-posttest design, non-equivalent control group design, regression discontinuity design, and interrupted time series design.
- Select the participants: Select the participants who will be included in the study. Participants should be selected based on specific criteria relevant to the research question.
- Measure the variables: Measure the variables that are relevant to the research question. This may involve using surveys, questionnaires, tests, or other measures.
- Implement the intervention or treatment: Implement the intervention or treatment to the participants in the intervention group. This may involve training, education, counseling, or other interventions.
- Collect data: Collect data on the dependent variable(s) before and after the intervention. Data collection may also include collecting data on other variables that may impact the dependent variable(s).
- Analyze the data: Analyze the data collected to determine whether the intervention had a significant impact on the dependent variable(s).
- Draw conclusions: Draw conclusions about the relationship between the independent and dependent variables. If the results suggest a causal relationship, then appropriate recommendations may be made based on the findings.
Quasi-Experimental Design Examples
Here are some examples of real-time quasi-experimental designs:
- Evaluating the impact of a new teaching method: In this study, a group of students are taught using a new teaching method, while another group is taught using the traditional method. The test scores of both groups are compared before and after the intervention to determine whether the new teaching method had a significant impact on student performance.
- Assessing the effectiveness of a public health campaign: In this study, a public health campaign is launched to promote healthy eating habits among a targeted population. The behavior of the population is compared before and after the campaign to determine whether the intervention had a significant impact on the target behavior.
- Examining the impact of a new medication: In this study, a group of patients is given a new medication, while another group is given a placebo. The outcomes of both groups are compared to determine whether the new medication had a significant impact on the targeted health condition.
- Evaluating the effectiveness of a job training program : In this study, a group of unemployed individuals is enrolled in a job training program, while another group is not enrolled in any program. The employment rates of both groups are compared before and after the intervention to determine whether the training program had a significant impact on the employment rates of the participants.
- Assessing the impact of a new policy : In this study, a new policy is implemented in a particular area, while another area does not have the new policy. The outcomes of both areas are compared before and after the intervention to determine whether the new policy had a significant impact on the targeted behavior or outcome.
Applications of Quasi-Experimental Design
Here are some applications of quasi-experimental design:
- Educational research: Quasi-experimental designs are used to evaluate the effectiveness of educational interventions, such as new teaching methods, technology-based learning, or educational policies.
- Health research: Quasi-experimental designs are used to evaluate the effectiveness of health interventions, such as new medications, public health campaigns, or health policies.
- Social science research: Quasi-experimental designs are used to investigate the impact of social interventions, such as job training programs, welfare policies, or criminal justice programs.
- Business research: Quasi-experimental designs are used to evaluate the impact of business interventions, such as marketing campaigns, new products, or pricing strategies.
- Environmental research: Quasi-experimental designs are used to evaluate the impact of environmental interventions, such as conservation programs, pollution control policies, or renewable energy initiatives.
When to use Quasi-Experimental Design
Here are some situations where quasi-experimental designs may be appropriate:
- When the research question involves investigating the effectiveness of an intervention, policy, or program : In situations where it is not feasible or ethical to randomly assign participants to intervention and control groups, quasi-experimental designs can be used to evaluate the impact of the intervention on the targeted outcome.
- When the sample size is small: In situations where the sample size is small, it may be difficult to randomly assign participants to intervention and control groups. Quasi-experimental designs can be used to investigate the impact of an intervention without requiring a large sample size.
- When the research question involves investigating a naturally occurring event : In some situations, researchers may be interested in investigating the impact of a naturally occurring event, such as a natural disaster or a major policy change. Quasi-experimental designs can be used to evaluate the impact of the event on the targeted outcome.
- When the research question involves investigating a long-term intervention: In situations where the intervention or program is long-term, it may be difficult to randomly assign participants to intervention and control groups for the entire duration of the intervention. Quasi-experimental designs can be used to evaluate the impact of the intervention over time.
- When the research question involves investigating the impact of a variable that cannot be manipulated : In some situations, it may not be possible or ethical to manipulate a variable of interest. Quasi-experimental designs can be used to investigate the relationship between the variable and the targeted outcome.
Purpose of Quasi-Experimental Design
The purpose of quasi-experimental design is to investigate the causal relationship between two or more variables when it is not feasible or ethical to conduct a randomized controlled trial (RCT). Quasi-experimental designs attempt to emulate the randomized control trial by mimicking the control group and the intervention group as much as possible.
The key purpose of quasi-experimental design is to evaluate the impact of an intervention, policy, or program on a targeted outcome while controlling for potential confounding factors that may affect the outcome. Quasi-experimental designs aim to answer questions such as: Did the intervention cause the change in the outcome? Would the outcome have changed without the intervention? And was the intervention effective in achieving its intended goals?
Quasi-experimental designs are useful in situations where randomized controlled trials are not feasible or ethical. They provide researchers with an alternative method to evaluate the effectiveness of interventions, policies, and programs in real-life settings. Quasi-experimental designs can also help inform policy and practice by providing valuable insights into the causal relationships between variables.
Overall, the purpose of quasi-experimental design is to provide a rigorous method for evaluating the impact of interventions, policies, and programs while controlling for potential confounding factors that may affect the outcome.
Advantages of Quasi-Experimental Design
Quasi-experimental designs have several advantages over other research designs, such as:
- Greater external validity : Quasi-experimental designs are more likely to have greater external validity than laboratory experiments because they are conducted in naturalistic settings. This means that the results are more likely to generalize to real-world situations.
- Ethical considerations: Quasi-experimental designs often involve naturally occurring events, such as natural disasters or policy changes. This means that researchers do not need to manipulate variables, which can raise ethical concerns.
- More practical: Quasi-experimental designs are often more practical than experimental designs because they are less expensive and easier to conduct. They can also be used to evaluate programs or policies that have already been implemented, which can save time and resources.
- No random assignment: Quasi-experimental designs do not require random assignment, which can be difficult or impossible in some cases, such as when studying the effects of a natural disaster. This means that researchers can still make causal inferences, although they must use statistical techniques to control for potential confounding variables.
- Greater generalizability : Quasi-experimental designs are often more generalizable than experimental designs because they include a wider range of participants and conditions. This can make the results more applicable to different populations and settings.
Limitations of Quasi-Experimental Design
There are several limitations associated with quasi-experimental designs, which include:
- Lack of Randomization: Quasi-experimental designs do not involve randomization of participants into groups, which means that the groups being studied may differ in important ways that could affect the outcome of the study. This can lead to problems with internal validity and limit the ability to make causal inferences.
- Selection Bias: Quasi-experimental designs may suffer from selection bias because participants are not randomly assigned to groups. Participants may self-select into groups or be assigned based on pre-existing characteristics, which may introduce bias into the study.
- History and Maturation: Quasi-experimental designs are susceptible to history and maturation effects, where the passage of time or other events may influence the outcome of the study.
- Lack of Control: Quasi-experimental designs may lack control over extraneous variables that could influence the outcome of the study. This can limit the ability to draw causal inferences from the study.
- Limited Generalizability: Quasi-experimental designs may have limited generalizability because the results may only apply to the specific population and context being studied.
About the author
Muhammad Hassan
Researcher, Academic Writer, Web developer
You may also like

Experimental Design – Types, Methods, Guide

Descriptive Research Design – Types, Methods and...

Explanatory Research – Types, Methods, Guide

Quantitative Research – Methods, Types and...

Ethnographic Research -Types, Methods and Guide

Qualitative Research Methods
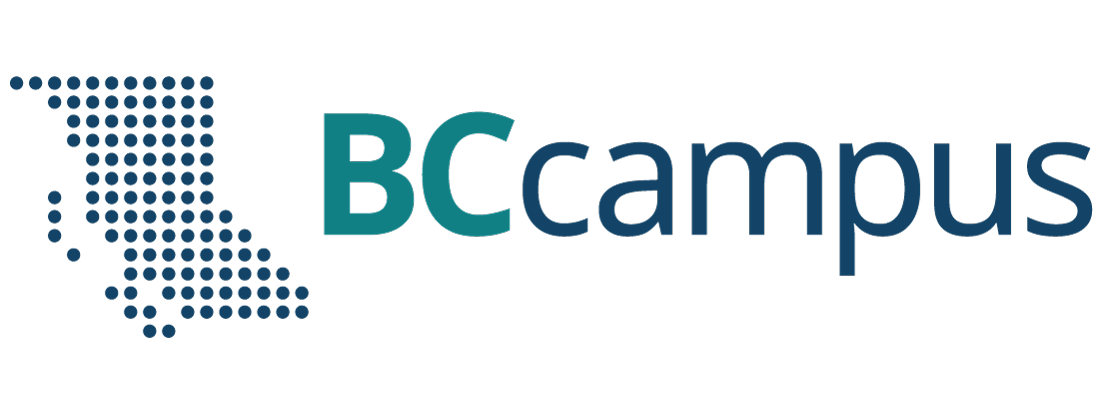
Want to create or adapt books like this? Learn more about how Pressbooks supports open publishing practices.
Chapter 7: Nonexperimental Research
Quasi-Experimental Research
Learning Objectives
- Explain what quasi-experimental research is and distinguish it clearly from both experimental and correlational research.
- Describe three different types of quasi-experimental research designs (nonequivalent groups, pretest-posttest, and interrupted time series) and identify examples of each one.
The prefix quasi means “resembling.” Thus quasi-experimental research is research that resembles experimental research but is not true experimental research. Although the independent variable is manipulated, participants are not randomly assigned to conditions or orders of conditions (Cook & Campbell, 1979). [1] Because the independent variable is manipulated before the dependent variable is measured, quasi-experimental research eliminates the directionality problem. But because participants are not randomly assigned—making it likely that there are other differences between conditions—quasi-experimental research does not eliminate the problem of confounding variables. In terms of internal validity, therefore, quasi-experiments are generally somewhere between correlational studies and true experiments.
Quasi-experiments are most likely to be conducted in field settings in which random assignment is difficult or impossible. They are often conducted to evaluate the effectiveness of a treatment—perhaps a type of psychotherapy or an educational intervention. There are many different kinds of quasi-experiments, but we will discuss just a few of the most common ones here.
Nonequivalent Groups Design
Recall that when participants in a between-subjects experiment are randomly assigned to conditions, the resulting groups are likely to be quite similar. In fact, researchers consider them to be equivalent. When participants are not randomly assigned to conditions, however, the resulting groups are likely to be dissimilar in some ways. For this reason, researchers consider them to be nonequivalent. A nonequivalent groups design , then, is a between-subjects design in which participants have not been randomly assigned to conditions.
Imagine, for example, a researcher who wants to evaluate a new method of teaching fractions to third graders. One way would be to conduct a study with a treatment group consisting of one class of third-grade students and a control group consisting of another class of third-grade students. This design would be a nonequivalent groups design because the students are not randomly assigned to classes by the researcher, which means there could be important differences between them. For example, the parents of higher achieving or more motivated students might have been more likely to request that their children be assigned to Ms. Williams’s class. Or the principal might have assigned the “troublemakers” to Mr. Jones’s class because he is a stronger disciplinarian. Of course, the teachers’ styles, and even the classroom environments, might be very different and might cause different levels of achievement or motivation among the students. If at the end of the study there was a difference in the two classes’ knowledge of fractions, it might have been caused by the difference between the teaching methods—but it might have been caused by any of these confounding variables.
Of course, researchers using a nonequivalent groups design can take steps to ensure that their groups are as similar as possible. In the present example, the researcher could try to select two classes at the same school, where the students in the two classes have similar scores on a standardized math test and the teachers are the same sex, are close in age, and have similar teaching styles. Taking such steps would increase the internal validity of the study because it would eliminate some of the most important confounding variables. But without true random assignment of the students to conditions, there remains the possibility of other important confounding variables that the researcher was not able to control.
Pretest-Posttest Design
In a pretest-posttest design , the dependent variable is measured once before the treatment is implemented and once after it is implemented. Imagine, for example, a researcher who is interested in the effectiveness of an antidrug education program on elementary school students’ attitudes toward illegal drugs. The researcher could measure the attitudes of students at a particular elementary school during one week, implement the antidrug program during the next week, and finally, measure their attitudes again the following week. The pretest-posttest design is much like a within-subjects experiment in which each participant is tested first under the control condition and then under the treatment condition. It is unlike a within-subjects experiment, however, in that the order of conditions is not counterbalanced because it typically is not possible for a participant to be tested in the treatment condition first and then in an “untreated” control condition.
If the average posttest score is better than the average pretest score, then it makes sense to conclude that the treatment might be responsible for the improvement. Unfortunately, one often cannot conclude this with a high degree of certainty because there may be other explanations for why the posttest scores are better. One category of alternative explanations goes under the name of history . Other things might have happened between the pretest and the posttest. Perhaps an antidrug program aired on television and many of the students watched it, or perhaps a celebrity died of a drug overdose and many of the students heard about it. Another category of alternative explanations goes under the name of maturation . Participants might have changed between the pretest and the posttest in ways that they were going to anyway because they are growing and learning. If it were a yearlong program, participants might become less impulsive or better reasoners and this might be responsible for the change.
Another alternative explanation for a change in the dependent variable in a pretest-posttest design is regression to the mean . This refers to the statistical fact that an individual who scores extremely on a variable on one occasion will tend to score less extremely on the next occasion. For example, a bowler with a long-term average of 150 who suddenly bowls a 220 will almost certainly score lower in the next game. Her score will “regress” toward her mean score of 150. Regression to the mean can be a problem when participants are selected for further study because of their extreme scores. Imagine, for example, that only students who scored especially low on a test of fractions are given a special training program and then retested. Regression to the mean all but guarantees that their scores will be higher even if the training program has no effect. A closely related concept—and an extremely important one in psychological research—is spontaneous remission . This is the tendency for many medical and psychological problems to improve over time without any form of treatment. The common cold is a good example. If one were to measure symptom severity in 100 common cold sufferers today, give them a bowl of chicken soup every day, and then measure their symptom severity again in a week, they would probably be much improved. This does not mean that the chicken soup was responsible for the improvement, however, because they would have been much improved without any treatment at all. The same is true of many psychological problems. A group of severely depressed people today is likely to be less depressed on average in 6 months. In reviewing the results of several studies of treatments for depression, researchers Michael Posternak and Ivan Miller found that participants in waitlist control conditions improved an average of 10 to 15% before they received any treatment at all (Posternak & Miller, 2001) [2] . Thus one must generally be very cautious about inferring causality from pretest-posttest designs.
Does Psychotherapy Work?
Early studies on the effectiveness of psychotherapy tended to use pretest-posttest designs. In a classic 1952 article, researcher Hans Eysenck summarized the results of 24 such studies showing that about two thirds of patients improved between the pretest and the posttest (Eysenck, 1952) [3] . But Eysenck also compared these results with archival data from state hospital and insurance company records showing that similar patients recovered at about the same rate without receiving psychotherapy. This parallel suggested to Eysenck that the improvement that patients showed in the pretest-posttest studies might be no more than spontaneous remission. Note that Eysenck did not conclude that psychotherapy was ineffective. He merely concluded that there was no evidence that it was, and he wrote of “the necessity of properly planned and executed experimental studies into this important field” (p. 323). You can read the entire article here: Classics in the History of Psychology .
Fortunately, many other researchers took up Eysenck’s challenge, and by 1980 hundreds of experiments had been conducted in which participants were randomly assigned to treatment and control conditions, and the results were summarized in a classic book by Mary Lee Smith, Gene Glass, and Thomas Miller (Smith, Glass, & Miller, 1980) [4] . They found that overall psychotherapy was quite effective, with about 80% of treatment participants improving more than the average control participant. Subsequent research has focused more on the conditions under which different types of psychotherapy are more or less effective.
Interrupted Time Series Design
A variant of the pretest-posttest design is the interrupted time-series design . A time series is a set of measurements taken at intervals over a period of time. For example, a manufacturing company might measure its workers’ productivity each week for a year. In an interrupted time series-design, a time series like this one is “interrupted” by a treatment. In one classic example, the treatment was the reduction of the work shifts in a factory from 10 hours to 8 hours (Cook & Campbell, 1979) [5] . Because productivity increased rather quickly after the shortening of the work shifts, and because it remained elevated for many months afterward, the researcher concluded that the shortening of the shifts caused the increase in productivity. Notice that the interrupted time-series design is like a pretest-posttest design in that it includes measurements of the dependent variable both before and after the treatment. It is unlike the pretest-posttest design, however, in that it includes multiple pretest and posttest measurements.
Figure 7.3 shows data from a hypothetical interrupted time-series study. The dependent variable is the number of student absences per week in a research methods course. The treatment is that the instructor begins publicly taking attendance each day so that students know that the instructor is aware of who is present and who is absent. The top panel of Figure 7.3 shows how the data might look if this treatment worked. There is a consistently high number of absences before the treatment, and there is an immediate and sustained drop in absences after the treatment. The bottom panel of Figure 7.3 shows how the data might look if this treatment did not work. On average, the number of absences after the treatment is about the same as the number before. This figure also illustrates an advantage of the interrupted time-series design over a simpler pretest-posttest design. If there had been only one measurement of absences before the treatment at Week 7 and one afterward at Week 8, then it would have looked as though the treatment were responsible for the reduction. The multiple measurements both before and after the treatment suggest that the reduction between Weeks 7 and 8 is nothing more than normal week-to-week variation.
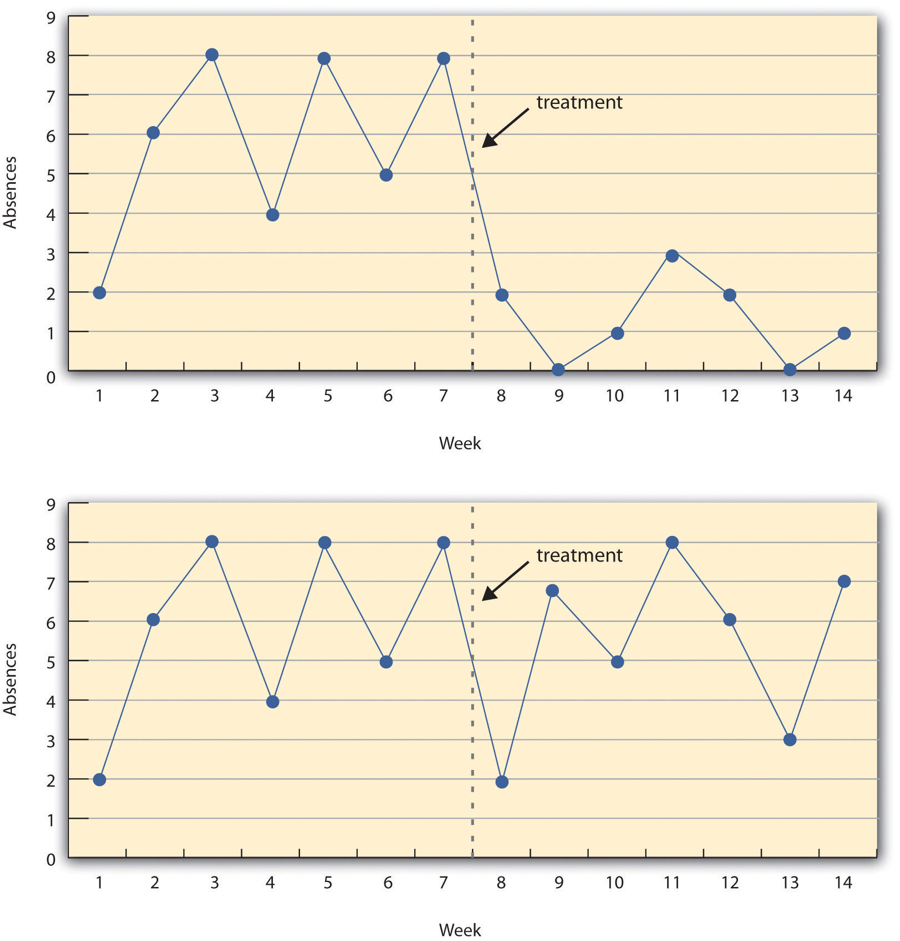
Combination Designs
A type of quasi-experimental design that is generally better than either the nonequivalent groups design or the pretest-posttest design is one that combines elements of both. There is a treatment group that is given a pretest, receives a treatment, and then is given a posttest. But at the same time there is a control group that is given a pretest, does not receive the treatment, and then is given a posttest. The question, then, is not simply whether participants who receive the treatment improve but whether they improve more than participants who do not receive the treatment.
Imagine, for example, that students in one school are given a pretest on their attitudes toward drugs, then are exposed to an antidrug program, and finally are given a posttest. Students in a similar school are given the pretest, not exposed to an antidrug program, and finally are given a posttest. Again, if students in the treatment condition become more negative toward drugs, this change in attitude could be an effect of the treatment, but it could also be a matter of history or maturation. If it really is an effect of the treatment, then students in the treatment condition should become more negative than students in the control condition. But if it is a matter of history (e.g., news of a celebrity drug overdose) or maturation (e.g., improved reasoning), then students in the two conditions would be likely to show similar amounts of change. This type of design does not completely eliminate the possibility of confounding variables, however. Something could occur at one of the schools but not the other (e.g., a student drug overdose), so students at the first school would be affected by it while students at the other school would not.
Finally, if participants in this kind of design are randomly assigned to conditions, it becomes a true experiment rather than a quasi experiment. In fact, it is the kind of experiment that Eysenck called for—and that has now been conducted many times—to demonstrate the effectiveness of psychotherapy.
Key Takeaways
- Quasi-experimental research involves the manipulation of an independent variable without the random assignment of participants to conditions or orders of conditions. Among the important types are nonequivalent groups designs, pretest-posttest, and interrupted time-series designs.
- Quasi-experimental research eliminates the directionality problem because it involves the manipulation of the independent variable. It does not eliminate the problem of confounding variables, however, because it does not involve random assignment to conditions. For these reasons, quasi-experimental research is generally higher in internal validity than correlational studies but lower than true experiments.
- Practice: Imagine that two professors decide to test the effect of giving daily quizzes on student performance in a statistics course. They decide that Professor A will give quizzes but Professor B will not. They will then compare the performance of students in their two sections on a common final exam. List five other variables that might differ between the two sections that could affect the results.
- regression to the mean
- spontaneous remission
Image Descriptions
Figure 7.3 image description: Two line graphs charting the number of absences per week over 14 weeks. The first 7 weeks are without treatment and the last 7 weeks are with treatment. In the first line graph, there are between 4 to 8 absences each week. After the treatment, the absences drop to 0 to 3 each week, which suggests the treatment worked. In the second line graph, there is no noticeable change in the number of absences per week after the treatment, which suggests the treatment did not work. [Return to Figure 7.3]
- Cook, T. D., & Campbell, D. T. (1979). Quasi-experimentation: Design & analysis issues in field settings . Boston, MA: Houghton Mifflin. ↵
- Posternak, M. A., & Miller, I. (2001). Untreated short-term course of major depression: A meta-analysis of studies using outcomes from studies using wait-list control groups. Journal of Affective Disorders, 66 , 139–146. ↵
- Eysenck, H. J. (1952). The effects of psychotherapy: An evaluation. Journal of Consulting Psychology, 16 , 319–324. ↵
- Smith, M. L., Glass, G. V., & Miller, T. I. (1980). The benefits of psychotherapy . Baltimore, MD: Johns Hopkins University Press. ↵
A between-subjects design in which participants have not been randomly assigned to conditions.
The dependent variable is measured once before the treatment is implemented and once after it is implemented.
A category of alternative explanations for differences between scores such as events that happened between the pretest and posttest, unrelated to the study.
An alternative explanation that refers to how the participants might have changed between the pretest and posttest in ways that they were going to anyway because they are growing and learning.
The statistical fact that an individual who scores extremely on a variable on one occasion will tend to score less extremely on the next occasion.
The tendency for many medical and psychological problems to improve over time without any form of treatment.
A set of measurements taken at intervals over a period of time that are interrupted by a treatment.
Research Methods in Psychology - 2nd Canadian Edition Copyright © 2015 by Paul C. Price, Rajiv Jhangiani, & I-Chant A. Chiang is licensed under a Creative Commons Attribution-NonCommercial-ShareAlike 4.0 International License , except where otherwise noted.
Share This Book
The use and interpretation of quasi-experimental design
Last updated
6 February 2023
Reviewed by
Miroslav Damyanov
Short on time? Get an AI generated summary of this article instead
- What is a quasi-experimental design?
Commonly used in medical informatics (a field that uses digital information to ensure better patient care), researchers generally use this design to evaluate the effectiveness of a treatment – perhaps a type of antibiotic or psychotherapy, or an educational or policy intervention.
Even though quasi-experimental design has been used for some time, relatively little is known about it. Read on to learn the ins and outs of this research design.
Make research less tedious
Dovetail streamlines research to help you uncover and share actionable insights
- When to use a quasi-experimental design
A quasi-experimental design is used when it's not logistically feasible or ethical to conduct randomized, controlled trials. As its name suggests, a quasi-experimental design is almost a true experiment. However, researchers don't randomly select elements or participants in this type of research.
Researchers prefer to apply quasi-experimental design when there are ethical or practical concerns. Let's look at these two reasons more closely.
Ethical reasons
In some situations, the use of randomly assigned elements can be unethical. For instance, providing public healthcare to one group and withholding it to another in research is unethical. A quasi-experimental design would examine the relationship between these two groups to avoid physical danger.
Practical reasons
Randomized controlled trials may not be the best approach in research. For instance, it's impractical to trawl through large sample sizes of participants without using a particular attribute to guide your data collection .
Recruiting participants and properly designing a data-collection attribute to make the research a true experiment requires a lot of time and effort, and can be expensive if you don’t have a large funding stream.
A quasi-experimental design allows researchers to take advantage of previously collected data and use it in their study.
- Examples of quasi-experimental designs
Quasi-experimental research design is common in medical research, but any researcher can use it for research that raises practical and ethical concerns. Here are a few examples of quasi-experimental designs used by different researchers:
Example 1: Determining the effectiveness of math apps in supplementing math classes
A school wanted to supplement its math classes with a math app. To select the best app, the school decided to conduct demo tests on two apps before selecting the one they will purchase.
Scope of the research
Since every grade had two math teachers, each teacher used one of the two apps for three months. They then gave the students the same math exams and compared the results to determine which app was most effective.
Reasons why this is a quasi-experimental study
This simple study is a quasi-experiment since the school didn't randomly assign its students to the applications. They used a pre-existing class structure to conduct the study since it was impractical to randomly assign the students to each app.
Example 2: Determining the effectiveness of teaching modern leadership techniques in start-up businesses
A hypothetical quasi-experimental study was conducted in an economically developing country in a mid-sized city.
Five start-ups in the textile industry and five in the tech industry participated in the study. The leaders attended a six-week workshop on leadership style, team management, and employee motivation.
After a year, the researchers assessed the performance of each start-up company to determine growth. The results indicated that the tech start-ups were further along in their growth than the textile companies.
The basis of quasi-experimental research is a non-randomized subject-selection process. This study didn't use specific aspects to determine which start-up companies should participate. Therefore, the results may seem straightforward, but several aspects may determine the growth of a specific company, apart from the variables used by the researchers.
Example 3: A study to determine the effects of policy reforms and of luring foreign investment on small businesses in two mid-size cities
In a study to determine the economic impact of government reforms in an economically developing country, the government decided to test whether creating reforms directed at small businesses or luring foreign investments would spur the most economic development.
The government selected two cities with similar population demographics and sizes. In one of the cities, they implemented specific policies that would directly impact small businesses, and in the other, they implemented policies to attract foreign investment.
After five years, they collected end-of-year economic growth data from both cities. They looked at elements like local GDP growth, unemployment rates, and housing sales.
The study used a non-randomized selection process to determine which city would participate in the research. Researchers left out certain variables that would play a crucial role in determining the growth of each city. They used pre-existing groups of people based on research conducted in each city, rather than random groups.
- Advantages of a quasi-experimental design
Some advantages of quasi-experimental designs are:
Researchers can manipulate variables to help them meet their study objectives.
It offers high external validity, making it suitable for real-world applications, specifically in social science experiments.
Integrating this methodology into other research designs is easier, especially in true experimental research. This cuts down on the time needed to determine your outcomes.
- Disadvantages of a quasi-experimental design
Despite the pros that come with a quasi-experimental design, there are several disadvantages associated with it, including the following:
It has a lower internal validity since researchers do not have full control over the comparison and intervention groups or between time periods because of differences in characteristics in people, places, or time involved. It may be challenging to determine whether all variables have been used or whether those used in the research impacted the results.
There is the risk of inaccurate data since the research design borrows information from other studies.
There is the possibility of bias since researchers select baseline elements and eligibility.
- What are the different quasi-experimental study designs?
There are three distinct types of quasi-experimental designs:
Nonequivalent
Regression discontinuity, natural experiment.
This is a hybrid of experimental and quasi-experimental methods and is used to leverage the best qualities of the two. Like the true experiment design, nonequivalent group design uses pre-existing groups believed to be comparable. However, it doesn't use randomization, the lack of which is a crucial element for quasi-experimental design.
Researchers usually ensure that no confounding variables impact them throughout the grouping process. This makes the groupings more comparable.
Example of a nonequivalent group design
A small study was conducted to determine whether after-school programs result in better grades. Researchers randomly selected two groups of students: one to implement the new program, the other not to. They then compared the results of the two groups.
This type of quasi-experimental research design calculates the impact of a specific treatment or intervention. It uses a criterion known as "cutoff" that assigns treatment according to eligibility.
Researchers often assign participants above the cutoff to the treatment group. This puts a negligible distinction between the two groups (treatment group and control group).
Example of regression discontinuity
Students must achieve a minimum score to be enrolled in specific US high schools. Since the cutoff score used to determine eligibility for enrollment is arbitrary, researchers can assume that the disparity between students who only just fail to achieve the cutoff point and those who barely pass is a small margin and is due to the difference in the schools that these students attend.
Researchers can then examine the long-term effects of these two groups of kids to determine the effect of attending certain schools. This information can be applied to increase the chances of students being enrolled in these high schools.
This research design is common in laboratory and field experiments where researchers control target subjects by assigning them to different groups. Researchers randomly assign subjects to a treatment group using nature or an external event or situation.
However, even with random assignment, this research design cannot be called a true experiment since nature aspects are observational. Researchers can also exploit these aspects despite having no control over the independent variables.
Example of the natural experiment approach
An example of a natural experiment is the 2008 Oregon Health Study.
Oregon intended to allow more low-income people to participate in Medicaid.
Since they couldn't afford to cover every person who qualified for the program, the state used a random lottery to allocate program slots.
Researchers assessed the program's effectiveness by assigning the selected subjects to a randomly assigned treatment group, while those that didn't win the lottery were considered the control group.
- Differences between quasi-experiments and true experiments
There are several differences between a quasi-experiment and a true experiment:
Participants in true experiments are randomly assigned to the treatment or control group, while participants in a quasi-experiment are not assigned randomly.
In a quasi-experimental design, the control and treatment groups differ in unknown or unknowable ways, apart from the experimental treatments that are carried out. Therefore, the researcher should try as much as possible to control these differences.
Quasi-experimental designs have several "competing hypotheses," which compete with experimental manipulation to explain the observed results.
Quasi-experiments tend to have lower internal validity (the degree of confidence in the research outcomes) than true experiments, but they may offer higher external validity (whether findings can be extended to other contexts) as they involve real-world interventions instead of controlled interventions in artificial laboratory settings.
Despite the distinct difference between true and quasi-experimental research designs, these two research methodologies share the following aspects:
Both study methods subject participants to some form of treatment or conditions.
Researchers have the freedom to measure some of the outcomes of interest.
Researchers can test whether the differences in the outcomes are associated with the treatment.
- An example comparing a true experiment and quasi-experiment
Imagine you wanted to study the effects of junk food on obese people. Here's how you would do this as a true experiment and a quasi-experiment:
How to carry out a true experiment
In a true experiment, some participants would eat junk foods, while the rest would be in the control group, adhering to a regular diet. At the end of the study, you would record the health and discomfort of each group.
This kind of experiment would raise ethical concerns since the participants assigned to the treatment group are required to eat junk food against their will throughout the experiment. This calls for a quasi-experimental design.
How to carry out a quasi-experiment
In quasi-experimental research, you would start by finding out which participants want to try junk food and which prefer to stick to a regular diet. This allows you to assign these two groups based on subject choice.
In this case, you didn't assign participants to a particular group, so you can confidently use the results from the study.
When is a quasi-experimental design used?
Quasi-experimental designs are used when researchers don’t want to use randomization when evaluating their intervention.
What are the characteristics of quasi-experimental designs?
Some of the characteristics of a quasi-experimental design are:
Researchers don't randomly assign participants into groups, but study their existing characteristics and assign them accordingly.
Researchers study the participants in pre- and post-testing to determine the progress of the groups.
Quasi-experimental design is ethical since it doesn’t involve offering or withholding treatment at random.
Quasi-experimental design encompasses a broad range of non-randomized intervention studies. This design is employed when it is not ethical or logistically feasible to conduct randomized controlled trials. Researchers typically employ it when evaluating policy or educational interventions, or in medical or therapy scenarios.
How do you analyze data in a quasi-experimental design?
You can use two-group tests, time-series analysis, and regression analysis to analyze data in a quasi-experiment design. Each option has specific assumptions, strengths, limitations, and data requirements.
Should you be using a customer insights hub?
Do you want to discover previous research faster?
Do you share your research findings with others?
Do you analyze research data?
Start for free today, add your research, and get to key insights faster
Editor’s picks
Last updated: 18 April 2023
Last updated: 27 February 2023
Last updated: 6 February 2023
Last updated: 5 February 2023
Last updated: 16 April 2023
Last updated: 9 March 2023
Last updated: 30 April 2024
Last updated: 12 December 2023
Last updated: 11 March 2024
Last updated: 4 July 2024
Last updated: 6 March 2024
Last updated: 5 March 2024
Last updated: 13 May 2024
Latest articles
Related topics, .css-je19u9{-webkit-align-items:flex-end;-webkit-box-align:flex-end;-ms-flex-align:flex-end;align-items:flex-end;display:-webkit-box;display:-webkit-flex;display:-ms-flexbox;display:flex;-webkit-flex-direction:row;-ms-flex-direction:row;flex-direction:row;-webkit-box-flex-wrap:wrap;-webkit-flex-wrap:wrap;-ms-flex-wrap:wrap;flex-wrap:wrap;-webkit-box-pack:center;-ms-flex-pack:center;-webkit-justify-content:center;justify-content:center;row-gap:0;text-align:center;max-width:671px;}@media (max-width: 1079px){.css-je19u9{max-width:400px;}.css-je19u9>span{white-space:pre;}}@media (max-width: 799px){.css-je19u9{max-width:400px;}.css-je19u9>span{white-space:pre;}} decide what to .css-1kiodld{max-height:56px;display:-webkit-box;display:-webkit-flex;display:-ms-flexbox;display:flex;-webkit-align-items:center;-webkit-box-align:center;-ms-flex-align:center;align-items:center;}@media (max-width: 1079px){.css-1kiodld{display:none;}} build next, decide what to build next, log in or sign up.
Get started for free
Child Care and Early Education Research Connections
Experiments and quasi-experiments.
This page includes an explanation of the types, key components, validity, ethics, and advantages and disadvantages of experimental design.
An experiment is a study in which the researcher manipulates the level of some independent variable and then measures the outcome. Experiments are powerful techniques for evaluating cause-and-effect relationships. Many researchers consider experiments the "gold standard" against which all other research designs should be judged. Experiments are conducted both in the laboratory and in real life situations.
Types of Experimental Design
There are two basic types of research design:
- True experiments
- Quasi-experiments
The purpose of both is to examine the cause of certain phenomena.
True experiments, in which all the important factors that might affect the phenomena of interest are completely controlled, are the preferred design. Often, however, it is not possible or practical to control all the key factors, so it becomes necessary to implement a quasi-experimental research design.
Similarities between true and quasi-experiments:
- Study participants are subjected to some type of treatment or condition
- Some outcome of interest is measured
- The researchers test whether differences in this outcome are related to the treatment
Differences between true experiments and quasi-experiments:
- In a true experiment, participants are randomly assigned to either the treatment or the control group, whereas they are not assigned randomly in a quasi-experiment
- In a quasi-experiment, the control and treatment groups differ not only in terms of the experimental treatment they receive, but also in other, often unknown or unknowable, ways. Thus, the researcher must try to statistically control for as many of these differences as possible
- Because control is lacking in quasi-experiments, there may be several "rival hypotheses" competing with the experimental manipulation as explanations for observed results
Key Components of Experimental Research Design
The manipulation of predictor variables.
In an experiment, the researcher manipulates the factor that is hypothesized to affect the outcome of interest. The factor that is being manipulated is typically referred to as the treatment or intervention. The researcher may manipulate whether research subjects receive a treatment (e.g., antidepressant medicine: yes or no) and the level of treatment (e.g., 50 mg, 75 mg, 100 mg, and 125 mg).
Suppose, for example, a group of researchers was interested in the causes of maternal employment. They might hypothesize that the provision of government-subsidized child care would promote such employment. They could then design an experiment in which some subjects would be provided the option of government-funded child care subsidies and others would not. The researchers might also manipulate the value of the child care subsidies in order to determine if higher subsidy values might result in different levels of maternal employment.
Random Assignment
- Study participants are randomly assigned to different treatment groups
- All participants have the same chance of being in a given condition
- Participants are assigned to either the group that receives the treatment, known as the "experimental group" or "treatment group," or to the group which does not receive the treatment, referred to as the "control group"
- Random assignment neutralizes factors other than the independent and dependent variables, making it possible to directly infer cause and effect
Random Sampling
Traditionally, experimental researchers have used convenience sampling to select study participants. However, as research methods have become more rigorous, and the problems with generalizing from a convenience sample to the larger population have become more apparent, experimental researchers are increasingly turning to random sampling. In experimental policy research studies, participants are often randomly selected from program administrative databases and randomly assigned to the control or treatment groups.
Validity of Results
The two types of validity of experiments are internal and external. It is often difficult to achieve both in social science research experiments.
Internal Validity
- When an experiment is internally valid, we are certain that the independent variable (e.g., child care subsidies) caused the outcome of the study (e.g., maternal employment)
- When subjects are randomly assigned to treatment or control groups, we can assume that the independent variable caused the observed outcomes because the two groups should not have differed from one another at the start of the experiment
- For example, take the child care subsidy example above. Since research subjects were randomly assigned to the treatment (child care subsidies available) and control (no child care subsidies available) groups, the two groups should not have differed at the outset of the study. If, after the intervention, mothers in the treatment group were more likely to be working, we can assume that the availability of child care subsidies promoted maternal employment
One potential threat to internal validity in experiments occurs when participants either drop out of the study or refuse to participate in the study. If particular types of individuals drop out or refuse to participate more often than individuals with other characteristics, this is called differential attrition. For example, suppose an experiment was conducted to assess the effects of a new reading curriculum. If the new curriculum was so tough that many of the slowest readers dropped out of school, the school with the new curriculum would experience an increase in the average reading scores. The reason they experienced an increase in reading scores, however, is because the worst readers left the school, not because the new curriculum improved students' reading skills.
External Validity
- External validity is also of particular concern in social science experiments
- It can be very difficult to generalize experimental results to groups that were not included in the study
- Studies that randomly select participants from the most diverse and representative populations are more likely to have external validity
- The use of random sampling techniques makes it easier to generalize the results of studies to other groups
For example, a research study shows that a new curriculum improved reading comprehension of third-grade children in Iowa. To assess the study's external validity, you would ask whether this new curriculum would also be effective with third graders in New York or with children in other elementary grades.
Glossary terms related to validity:
- internal validity
- external validity
- differential attrition
It is particularly important in experimental research to follow ethical guidelines. Protecting the health and safety of research subjects is imperative. In order to assure subject safety, all researchers should have their project reviewed by the Institutional Review Boards (IRBS). The National Institutes of Health supplies strict guidelines for project approval. Many of these guidelines are based on the Belmont Report (pdf).
The basic ethical principles:
- Respect for persons -- requires that research subjects are not coerced into participating in a study and requires the protection of research subjects who have diminished autonomy
- Beneficence -- requires that experiments do not harm research subjects, and that researchers minimize the risks for subjects while maximizing the benefits for them
- Justice -- requires that all forms of differential treatment among research subjects be justified
Advantages and Disadvantages of Experimental Design
The environment in which the research takes place can often be carefully controlled. Consequently, it is easier to estimate the true effect of the variable of interest on the outcome of interest.
Disadvantages
It is often difficult to assure the external validity of the experiment, due to the frequently nonrandom selection processes and the artificial nature of the experimental context.
Introduction to Experimental and Quasi-Experimental Design
- First Online: 26 April 2022

Cite this chapter
- Melissa Whatley ORCID: orcid.org/0000-0002-7073-6772 2
Part of the book series: Springer Texts in Education ((SPTE))
679 Accesses
This chapter introduces readers to main concepts in experimental and quasi-experimental design. First, randomized control trials are introduced as the primary example of experimental design. Next, nonexperimental contexts, and particularly the use of propensity score matching to approximate the conditions of randomized control trials, are described. Finally, this chapter introduces two quasi-experimental design that are particularly useful in international education research: regression discontinuity and difference-in-differences.
This is a preview of subscription content, log in via an institution to check access.
Access this chapter
Subscribe and save.
- Get 10 units per month
- Download Article/Chapter or eBook
- 1 Unit = 1 Article or 1 Chapter
- Cancel anytime
- Available as PDF
- Read on any device
- Instant download
- Own it forever
- Available as EPUB and PDF
- Compact, lightweight edition
- Dispatched in 3 to 5 business days
- Free shipping worldwide - see info
Tax calculation will be finalised at checkout
Purchases are for personal use only
Institutional subscriptions
The extent to which randomization is possible in these scenarios is an important consideration that we will discuss later in this chapter.
Note that here I report only a subset of Meegan and Kashima’s (2010) results as I focus on only one of their two experimental conditions.
Advanced readers who are interested in these alternatives can read more about exact matching (Rubin, 1973 ), genetic matching (Diamond & Sekhon, 2013 ), or coarsened exact matching (Iacus, King, & Porro, 2011 ).
Sometimes, researchers are especially interested in estimating the impact of the treatment on the treated units (the average treatment effect on the treated [ATT]) rather than the average treatment effect for all units in a dataset (ATE). In this case, no treatment units are dropped from the dataset, even though some control units are.
A discussion of propensity score matching approaches is beyond the scope of this book, but several of the suggested readings at the end of this chapter delve deeper into matching techniques and their benefits and drawbacks.
Note that Iriondo (2020) estimates the impact of Erasmus participation on employment and salary using two different datasets. The results presented in this section correspond to his results for the Labor Insertion Survey.
Regression discontinuity and difference-in-differences are not the only two quasi-experimental designs available to researchers (other quasi-experimental designs include instrumental variable and time-series analyses). However, they are two of the more common designs and are also possibly the most useful to individuals conducting research in international education.
Recommended Reading
A deeper dive.
Cunningham, S. (2021). Causal inference: The mixtape . Yale University Press.
Google Scholar
DesJardins, S. L., & Flaster, A. (2013). Nonexperimental designs and causal analyses of college access, persistence, and completion. In L. W. Perna & A. Jones (Eds.), The state of college access and completion: Improving college success for students from underrepresented groups (pp. 190–207). Routledge.
Diamond, A., & Sekhon, J. S. (2013). Genetic matching for estimating causal effects: A general multivariate matching method for achieving balance in observational studies. Review of Economics and Statistics, 95 (3), 932–945.
Article Google Scholar
Furquim, F., Corral, D., & Hillman, N. (2020). A primer for interpreting and designing difference-in-difference studies in higher education research. In L. Perna (Ed.), Higher education: Handbook of theory and research (Vol. 35, pp. 2–53).
Iacus, S. M., King, G., & Porro, G. (2011). Multivariate matching methods that are monotonic imbalance bounding. Journal of the American Statistical Association, 106 (493), 345–361.
Murnane, R. J., & Willett, J. B. (2011). Methods matter: Improving causal inference in educational and social science research. Oxford University Press.
Reynolds, C. L., & DesJardins, S. L. (2009). The use of matching methods in higher education research: Answering whether attendance at a 2-year institution results in differences in educational attainment. In Higher education: Handbook of theory and research (pp. 47–97). Springer.
Rosenbaum, P. R., & Rubin, D. B. (1985). Constructing a control group using multivariate matched sampling methods that incorporate the propensity score. The American Statistician, 39 (1), 33–38.
Rubin, D. B. (1973). The use of matched sampling and regression adjustment to remove bias in observational studies. Biometrics , (Vol. 29, pp.185–203).
Additional Examples
d’Hombres, B., & Schnepf, S. V. (2021). International mobility of students in Italy and the UK: Does it pay off and for whom? Higher Education . https://doi.org/10.1007/s10734-020-00631-1
Dicks, A., & Lancee, B. (2018). Double disadvantage in school? Children of immigrants and the relative age effect: A regression discontinuity design based on the month of birth. European Sociological Review, 34 (3), 319–333.
Marini, G., & Yang, L. (2021). Globally bred Chinese talents returning home: An analysis of a reverse brain-drain flagship policy. Science and Public Policy . https://doi.org/10.1093/scipol/scab021
Monogan, J. E., & Doctor, A. C. (2017). Immigration politics and partisan realignment: California, Texas, and the 1994 election. State Politics & Policy Quarterly, 17 (1), 3–23.
Download references
Author information
Authors and affiliations.
School for International Training, Brattleboro, VT, USA
Melissa Whatley
You can also search for this author in PubMed Google Scholar
Corresponding author
Correspondence to Melissa Whatley .
Rights and permissions
Reprints and permissions
Copyright information
© 2022 The Author(s), under exclusive license to Springer Nature Switzerland AG
About this chapter
Whatley, M. (2022). Introduction to Experimental and Quasi-Experimental Design. In: Introduction to Quantitative Analysis for International Educators. Springer Texts in Education. Springer, Cham. https://doi.org/10.1007/978-3-030-93831-4_9
Download citation
DOI : https://doi.org/10.1007/978-3-030-93831-4_9
Published : 26 April 2022
Publisher Name : Springer, Cham
Print ISBN : 978-3-030-93830-7
Online ISBN : 978-3-030-93831-4
eBook Packages : Education Education (R0)
Share this chapter
Anyone you share the following link with will be able to read this content:
Sorry, a shareable link is not currently available for this article.
Provided by the Springer Nature SharedIt content-sharing initiative
- Publish with us
Policies and ethics
- Find a journal
- Track your research

An official website of the United States government
The .gov means it’s official. Federal government websites often end in .gov or .mil. Before sharing sensitive information, make sure you’re on a federal government site.
The site is secure. The https:// ensures that you are connecting to the official website and that any information you provide is encrypted and transmitted securely.
- Publications
- Account settings
Preview improvements coming to the PMC website in October 2024. Learn More or Try it out now .
- Advanced Search
- Journal List
- HHS Author Manuscripts

Experimental and Quasi-Experimental Designs in Implementation Research
Christopher j. miller.
a VA Boston Healthcare System, Center for Healthcare Organization and Implementation Research (CHOIR), United States Department of Veterans Affairs, Boston, MA, USA
b Department of Psychiatry, Harvard Medical School, Boston, MA, USA
Shawna N. Smith
c Department of Psychiatry, University of Michigan Medical School, Ann Arbor, MI, USA
d Survey Research Center, Institute for Social Research, University of Michigan, Ann Arbor, MI, USA
Marianne Pugatch
Implementation science is focused on maximizing the adoption, appropriate use, and sustainability of effective clinical practices in real world clinical settings. Many implementation science questions can be feasibly answered by fully experimental designs, typically in the form of randomized controlled trials (RCTs). Implementation-focused RCTs, however, usually differ from traditional efficacy- or effectiveness-oriented RCTs on key parameters. Other implementation science questions are more suited to quasi-experimental designs, which are intended to estimate the effect of an intervention in the absence of randomization. These designs include pre-post designs with a non-equivalent control group, interrupted time series (ITS), and stepped wedges, the last of which require all participants to receive the intervention, but in a staggered fashion. In this article we review the use of experimental designs in implementation science, including recent methodological advances for implementation studies. We also review the use of quasi-experimental designs in implementation science, and discuss the strengths and weaknesses of these approaches. This article is therefore meant to be a practical guide for researchers who are interested in selecting the most appropriate study design to answer relevant implementation science questions, and thereby increase the rate at which effective clinical practices are adopted, spread, and sustained.
1. Background
The first documented clinical trial was conducted in 1747 by James Lind, a royal navy physician, who tested the hypothesis that citrus fruit could cure scurvy. Since then, based on foundational work by Fisher and others (1935), the randomized controlled trial (RCT) has emerged as the gold standard for testing the efficacy of treatment versus a control condition for individual patients. Randomization of patients is seen as a crucial to reducing the impact of measured or unmeasured confounding variables, in turn allowing researchers to draw conclusions regarding causality in clinical trials.
As described elsewhere in this special issue, implementation science is ultimately focused on maximizing the adoption, appropriate use, and sustainability of effective clinical practices in real world clinical settings. As such, some implementation science questions may be addressed by experimental designs. For our purposes here, we use the term “experimental” to refer to designs that feature two essential ingredients: first, manipulation of an independent variable; and second, random assignment of subjects. This corresponds to the definition of randomized experiments originally championed by Fisher (1925) . From this perspective, experimental designs usually take the form of RCTs—but implementation- oriented RCTs typically differ in important ways from traditional efficacy- or effectiveness-oriented RCTs. Other implementation science questions require different methodologies entirely: specifically, several forms of quasi-experimental designs may be used for implementation research in situations where an RCT would be inappropriate. These designs are intended to estimate the effect of an intervention despite a lack of randomization. Quasi-experimental designs include pre-post designs with a nonequivalent control group, interrupted time series (ITS), and stepped wedge designs. Stepped wedges are studies in which all participants receive the intervention, but in a staggered fashion. It is important to note that quasi-experimental designs are not unique to implementation science. As we will discuss below, however, each of them has strengths that make them particularly useful in certain implementation science contexts.
Our goal for this manuscript is two-fold. First, we will summarize the use of experimental designs in implementation science. This will include discussion of ways that implementation-focused RCTs may differ from efficacy- or effectiveness-oriented RCTs. Second, we will summarize the use of quasi-experimental designs in implementation research. This will include discussion of the strengths and weaknesses of these types of approaches in answering implementation research questions. For both experimental and quasi-experimental designs, we will discuss a recent implementation study as an illustrative example of one approach.
1. Experimental Designs in Implementation Science
RCTs in implementation science share the same basic structure as efficacy- or effectiveness-oriented RCTs, but typically feature important distinctions. In this section we will start by reviewing key factors that separate implementation RCTs from more traditional efficacy- or effectiveness-oriented RCTs. We will then discuss optimization trials, which are a type of experimental design that is especially useful for certain implementation science questions. We will then briefly turn our attention to single subject experimental designs (SSEDs) and on-off-on (ABA) designs.
The first common difference that sets apart implementation RCTs from more traditional clinical trials is the primary research question they aim to address. For most implementation trials, the primary research question is not the extent to which a particular treatment or evidence-based practice is more effective than a comparison condition, but instead the extent to which a given implementation strategy is more effective than a comparison condition. For more detail on this pivotal issue, see Drs. Bauer and Kirchner in this special issue.
Second, as a corollary of this point, implementation RCTs typically feature different outcome measures than efficacy or effectiveness RCTs, with an emphasis on the extent to which a health intervention was successfully implemented rather than an evaluation of the health effects of that intervention ( Proctor et al., 2011 ). For example, typical implementation outcomes might include the number of patients who receive the intervention, or the number of providers who administer the intervention as intended. A variety of evaluation-oriented implementation frameworks may guide the choices of such measures (e.g. RE-AIM; Gaglio et al., 2013 ; Glasgow et al., 1999 ). Hybrid implementation-effectiveness studies attend to both effectiveness and implementation outcomes ( Curran et al., 2012 ); these designs are also covered in more detail elsewhere in this issue (Landes, this issue).
Third, given their focus, implementation RCTs are frequently cluster-randomized (i.e. with sites or clinics as the unit of randomization, and patients nested within those sites or clinics). For example, consider a hypothetical RCT that aims to evaluate the implementation of a training program for cognitive behavioral therapy (CBT) in community clinics. Randomizing at the patient level for such a trial would be inappropriate due to the risk of contamination, as providers trained in CBT might reasonably be expected to incorporate CBT principles into their treatment even to patients assigned to the control condition. Randomizing at the provider level would also risk contamination, as providers trained in CBT might discuss this treatment approach with their colleagues. Thus, many implementation trials are cluster randomized at the site or clinic level. While such clustering minimizes the risk of contamination, it can unfortunately create commensurate problems with confounding, especially for trials with very few sites to randomize. Stratification may be used to at least partially address confounding issues in cluster- randomized and more traditional trials alike, by ensuring that intervention and control groups are broadly similar on certain key variables. Furthermore, such allocation schemes typically require analytic models that account for this clustering and the resulting correlations among error structures (e.g., generalized estimating equations [GEE] or mixed-effects models; Schildcrout et al., 2018 ).
1.1. Optimization trials
Key research questions in implementation science often involve determining which implementation strategies to provide, to whom, and when, to achieve optimal implementation success. As such, trials designed to evaluate comparative effectiveness, or to optimize provision of different types or intensities of implementation strategies, may be more appealing than traditional effectiveness trials. The methods described in this section are not unique to implementation science, but their application in the context of implementation trials may be particularly useful for informing implementation strategies.
While two-arm RCTs can be used to evaluate comparative effectiveness, trials focused on optimizing implementation support may use alternative experimental designs ( Collins et al., 2005 ; Collins et al., 2007 ). For example, in certain clinical contexts, multi-component “bundles” of implementation strategies may be warranted (e.g. a bundle consisting of clinician training, technical assistance, and audit/feedback to encourage clinicians to use a new evidence-based practice). In these situations, implementation researchers might consider using factorial or fractional-factorial designs. In the context of implementation science, these designs randomize participants (e.g. sites or providers) to different combinations of implementation strategies, and can be used to evaluate the effectiveness of each strategy individually to inform an optimal combination (e.g. Coulton et al., 2009 ; Pellegrini et al., 2014 ; Wyrick, et al., 2014 ). Such designs can be particularly useful in informing multi-component implementation strategies that are not redundant or overly burdensome ( Collins et al., 2014a ; Collins et al., 2009 ; Collins et al., 2007 ).
Researchers interested in optimizing sequences of implementation strategies that adapt to ongoing needs over time may be interested in a variant of factorial designs known as the sequential, multiple-assignment randomized trial (SMART; Almirall et al., 2012 ; Collins et al., 2014b ; Kilbourne et al., 2014b ; Lei et al., 2012 ; Nahum-Shani et al., 2012 ; NeCamp et al., 2017 ). SMARTs are multistage randomized trials in which some or all participants are randomized more than once, often based on ongoing information (e.g., treatment response). In implementation research, SMARTs can inform optimal sequences of implementation strategies to maximize downstream clinical outcomes. Thus, such designs are well-suited to answering questions about what implementation strategies should be used, in what order, to achieve the best outcomes in a given context.
One example of an implementation SMART is the Adaptive Implementation of Effective Program Trial (ADEPT; Kilbourne et al., 2014a ). ADEPT was a clustered SMART ( NeCamp et al., 2017 ) designed to inform an adaptive sequence of implementation strategies for implementing an evidence-based collaborative chronic care model, Life Goals ( Kilbourne et al., 2014c ; Kilbourne et al., 2012a ), into community-based practices. Life Goals, the clinical intervention being implemented, has proven effective at improving physical and mental health outcomes for patients with unipolar and bipolar depression by encouraging providers to instruct patients in self-management, and improving clinical information systems and care management across physical and mental health providers ( Bauer et al., 2006 ; Kilbourne et al., 2012a ; Kilbourne et al., 2008 ; Simon et al., 2006 ). However, in spite of its established clinical effectiveness, community-based clinics experienced a number of barriers in trying to implement the Life Goals model, and there were questions about how best to efficiently and effectively augment implementation strategies for clinics that struggled with implementation.
The ADEPT study was thus designed to determine the best sequence of implementation strategies to offer sites interested in implementing Life Goals. The ADEPT study involved use of three different implementation strategies. First, all sites received implementation support based on Replicating Effective Programs (REP), which offered an implementation manual, brief training, and low- level technical support ( Kilbourne et al., 2007 ; Kilbourne et al., 2012b ; Neumann and Sogolow, 2000 ). REP implementation support had been previously found to be low-cost and readily scalable, but also insufficient for uptake for many community-based settings ( Kilbourne et al., 2015 ). For sites that failed to implement Life Goals under REP, two additional implementation strategies were considered as augmentations to REP: External Facilitation (EF; Kilbourne et al., 2014b ; Stetler et al., 2006 ), consisting of phone-based mentoring in strategic skills from a study team member; and Internal Facilitation (IF; Kirchner et al., 2014 ), which supported protected time for a site employee to address barriers to program adoption.
The ADEPT study was designed to evaluate the best way to augment support for these sites that were not able to implement Life Goals under REP, specifically querying whether it was better to augment REP with EF only or the more intensive EF/IF, and whether augmentations should be provided all at once, or staged. Intervention assignments are mapped in Figure 1 . Seventy-nine community-based clinics across Michigan and Colorado were provided with initial implementation support under REP. After six months, implementation of the clinical intervention, Life Goals, was evaluated at all sites. Sites that had failed to reach an adequate level of delivery (defined as those sites enrolling fewer than ten patients in Life Goals, or those at which fewer than 50% of enrolled patients had received at least three Life Goals sessions) were considered non-responsive to REP and randomized to receive additional support through either EF or combined EF/IF. After six further months, Life Goals implementation at these sites was again evaluated. Sites surpassing the implementation response benchmark had their EF or EF/IF support discontinued. EF/IF sites that remained non-responsive continued to receive EF/IF for an additional six months. EF sites that remained non-responsive were randomized a second time to either continue with EF or further augment with IF. This design thus allowed for comparison of three different adaptive implementation interventions for sites that were initially non-responsive to REP to determine the best adaptive sequence of implementation support for sites that were initially non-responsive under REP:

SMART design from ADEPT trial.
- Provide EF for 6 months; continue EF for a further six months for sites that remain nonresponsive; discontinue EF for sites that are responsive;
- Provide EF/IF for 6 months; continue EF/IF for a further six months for sites that remain non-responsive; discontinue EF/IF for sites that are responsive; and
- Provide EF for 6 months; step up to EF/IF for a further six months for sites that remain non-responsive; discontinue EF for sites that are responsive.
While analyses of this study are still ongoing, including the comparison of these three adaptive sequences of implementation strategies, results have shown that patients at sites that were randomized to receive EF as the initial augmentation to REP saw more improvement in clinical outcomes (SF-12 mental health quality of life and PHQ-9 depression scores) after 12 months than patients at sites that were randomized to receive the more intensive EF/IF augmentation.
1.2. Single Subject Experimental Designs and On-Off-On (ABA) Designs
We also note that there are a variety of Single Subject Experimental Designs (SSEDs; Byiers et al., 2012 ), including withdrawal designs and alternating treatment designs, that can be used in testing evidence-based practices. Similarly, an implementation strategy may be used to encourage the use of a specific treatment at a particular site, followed by that strategy’s withdrawal and subsequent reinstatement, with data collection throughout the process (on-off-on or ABA design). A weakness of these approaches in the context of implementation science, however, is that they usually require reversibility of the intervention (i.e. that the withdrawal of implementation support truly allows the healthcare system to revert to its pre-implementation state). When this is not the case—for example, if a hypothetical study is focused on training to encourage use of an evidence-based psychotherapy—then these designs may be less useful.
2. Quasi-Experimental Designs in Implementation Science
In some implementation science contexts, policy-makers or administrators may not be willing to have a subset of participating patients or sites randomized to a control condition, especially for high-profile or high-urgency clinical issues. Quasi-experimental designs allow implementation scientists to conduct rigorous studies in these contexts, albeit with certain limitations. We briefly review the characteristics of these designs here; other recent review articles are available for the interested reader (e.g. Handley et al., 2018 ).
2.1. Pre-Post with Non-Equivalent Control Group
The pre-post with non-equivalent control group uses a control group in the absence of randomization. Ideally, the control group is chosen to be as similar to the intervention group as possible (e.g. by matching on factors such as clinic type, patient population, geographic region, etc.). Theoretically, both groups are exposed to the same trends in the environment, making it plausible to decipher if the intervention had an effect. Measurement of both treatment and control conditions classically occurs pre- and post-intervention, with differential improvement between the groups attributed to the intervention. This design is popular due to its practicality, especially if data collection points can be kept to a minimum. It may be especially useful for capitalizing on naturally occurring experiments such as may occur in the context of certain policy initiatives or rollouts—specifically, rollouts in which it is plausible that a control group can be identified. For example, Kirchner and colleagues (2014) used this type of design to evaluate the integration of mental health services into primary care clinics at seven US Department of Veterans Affairs (VA) medical centers and seven matched controls.
One overarching drawback of this design is that it is especially vulnerable to threats to internal validity ( Shadish, 2002 ), because pre-existing differences between the treatment and control group could erroneously be attributed to the intervention. While unmeasured differences between treatment and control groups are always a possibility in healthcare research, such differences are especially likely to occur in the context of these designs due to the lack of randomization. Similarly, this design is particularly sensitive to secular trends that may differentially affect the treatment and control groups ( Cousins et al., 2014 ; Pape et al., 2013 ), as well as regression to the mean confounding study results ( Morton and Torgerson, 2003 ). For example, if a study site is selected for the experimental condition precisely because it is underperforming in some way, then regression to the mean would suggest that the site will show improvement regardless of any intervention; in the context of a pre-post with non-equivalent control group study, however, this improvement would erroneously be attributed to the intervention itself (Type I error).
There are, however, various ways that implementation scientists can mitigate these weaknesses. First, as mentioned briefly above, it is important to select a control group that is as similar as possible to the intervention site(s), which can include matching at both the health care network and clinic level (e.g. Kirchner et al., 2014 ). Second, propensity score weighting (e.g. Morgan, 2018 ) can statistically mitigate internal validity concerns, although this approach may be of limited utility when comparing secular trends between different study cohorts ( Dimick and Ryan, 2014 ). More broadly, qualitative methods (e.g. periodic interviews with staff at intervention and control sites) can help uncover key contextual factors that may be affecting study results above and beyond the intervention itself.
2.2. Interrupted Time Series
Interrupted time series (ITS; Shadish, 2002 ; Taljaard et al., 2014 ; Wagner et al., 2002 ) designs represent one of the most robust categories of quasi-experimental designs. Rather than relying on a non-equivalent control group, ITS designs rely on repeated data collections from intervention sites to determine whether a particular intervention is associated with improvement on a given metric relative to the pre-intervention secular trend. They are particularly useful in cases where a comparable control group cannot be identified—for example, following widespread implementation of policy mandates, quality improvement initiatives, or dissemination campaigns ( Eccles et al., 2003 ). In ITS designs, data are collected at multiple time points both before and after an intervention (e.g., policy change, implementation effort), and analyses explore whether the intervention was associated with the outcome beyond any pre-existing secular trend. More formally, ITS evaluations focus on identifying whether there is discontinuity in the trend (change in slope or level) after the intervention relative to before the intervention, using segmented regression to model pre- and post-intervention trends ( Gebski et al., 2012 ; Penfold and Zhang, 2013 ; Taljaard et al., 2014 ; Wagner et al., 2002 ). A number of recent implementation studies have used ITS designs, including an evaluation of implementation of a comprehensive smoke-free policy in a large UK mental health organization to reduce physical assaults ( Robson et al., 2017 ); the impact of a national policy limiting alcohol availability on suicide mortality in Slovenia ( Pridemore and Snowden, 2009 ); and the effect of delivery of a tailored intervention for primary care providers to increase psychological referrals for women with mild to moderate postnatal depression ( Hanbury et al., 2013 ).
ITS designs are appealing in implementation work for several reasons. Relative to uncontrolled pre-post analyses, ITS analyses reduce the chances that intervention effects are confounded by secular trends ( Bernal et al., 2017 ; Eccles et al., 2003 ). Time-varying confounders, such as seasonality, can also be adjusted for, provided adequate data ( Bernal et al., 2017 ). Indeed, recent work has confirmed that ITS designs can yield effect estimates similar to those derived from cluster-randomized RCTs ( Fretheim et al., 2013 ; Fretheim et al., 2015 ). Relative to an RCT, ITS designs can also allow for a more comprehensive assessment of the longitudinal effects of an intervention (positive or negative), as effects can be traced over all included time points ( Bernal et al., 2017 ; Penfold and Zhang, 2013 ).
ITS designs also present a number of challenges. First, the segmented regression approach requires clear delineation between pre- and post-intervention periods; interventions with indeterminate implementation periods are likely not good candidates for ITS. While ITS designs that include multiple ‘interruptions’ (e.g. introductions of new treatment components) are possible, they will require collection of enough time points between interruptions to ensure that each intervention’s effects can be ascertained individually ( Bernal et al., 2017 ). Second, collecting data from sufficient time points across all sites of interest, especially for the pre-intervention period, can be challenging ( Eccles et al., 2003 ): a common recommendation is at least eight time points both pre- and post-intervention ( Penfold and Zhang, 2013 ). This may be onerous, particularly if the data are not routinely collected by the health system(s) under study. Third, ITS cannot protect against confounding effects from other interventions that begin contemporaneously and may impact similar outcomes ( Eccles et al., 2003 ).
2.3. Stepped Wedge Designs
Stepped wedge trials are another type of quasi-experimental design. In a stepped wedge, all participants receive the intervention, but are assigned to the timing of the intervention in a staggered fashion ( Betran et al., 2018 ; Brown and Lilford, 2006 ; Hussey and Hughes, 2007 ), typically at the site or cluster level. Stepped wedge designs have their analytic roots in balanced incomplete block designs, in which all pairs of treatments occur an equal number of times within each block ( Hanani, 1961 ). Traditionally, all sites in stepped wedge trials have outcome measures assessed at all time points, thus allowing sites that receive the intervention later in the trial to essentially serve as controls for early intervention sites. A recent special issue of the journal Trials includes more detail on these designs ( Davey et al., 2015 ), which may be ideal for situations in which it is important for all participating patients or sites to receive the intervention during the trial. Stepped wedge trials may also be useful when resources are scarce enough that intervening at all sites at once (or even half of the sites as in a standard treatment-versus-control RCT) would not be feasible. If desired, the administration of the intervention to sites in waves allows for lessons learned in early sites to be applied to later sites (via formative evaluation; see Elwy et al., this issue).
The Behavioral Health Interdisciplinary Program (BHIP) Enhancement Project is a recent example of a stepped-wedge implementation trial ( Bauer et al., 2016 ; Bauer et al., 2019 ). This study involved using blended facilitation (including internal and external facilitators; Kirchner et al., 2014 ) to implement care practices consistent with the collaborative chronic care model (CCM; Bodenheimer et al., 2002a , b ; Wagner et al., 1996 ) in nine outpatient mental health teams in VA medical centers. Figure 2 illustrates the implementation and stepdown periods for that trial, with black dots representing primary data collection points.

BHIP Enhancement Project stepped wedge (adapted form Bauer et al., 2019).
The BHIP Enhancement Project was conducted as a stepped wedge for several reasons. First, the stepped wedge design allowed the trial to reach nine sites despite limited implementation resources (i.e. intervening at all nine sites simultaneously would not have been feasible given study funding). Second, the stepped wedge design aided in recruitment and retention, as all participating sites were certain to receive implementation support during the trial: at worst, sites that were randomized to later- phase implementation had to endure waiting periods totaling about eight months before implementation began. This was seen as a major strength of the design by its operational partner, the VA Office of Mental Health and Suicide Prevention. To keep sites engaged during the waiting period, the BHIP Enhancement Project offered a guiding workbook and monthly technical support conference calls.
Three additional features of the BHIP Enhancement Project deserve special attention. First, data collection for late-implementing sites did not begin until immediately before the onset of implementation support (see Figure 2 ). While this reduced statistical power, it also significantly reduced data collection burden on the study team. Second, onset of implementation support was staggered such that wave 2 began at the end of month 4 rather than month 6. This had two benefits: first, this compressed the overall amount of time required for implementation during the trial. Second, it meant that the study team only had to collect data from one site at a time, with data collection periods coming every 2–4 months. More traditional stepped wedge approaches typically have data collection across sites temporally aligned (e.g. Betran et al., 2018 ). Third, the BHIP Enhancement Project used a balancing algorithm ( Lew et al., 2019 ) to assign sites to waves, retaining some of the benefits of randomization while ensuring balance on key site characteristics (e.g. size, geographic region).
Despite their utility, stepped wedges have some important limitations. First, because they feature delayed implementation at some sites, stepped wedges typically take longer than similarly-sized parallel group RCTs. This increases the chances that secular trends, policy changes, or other external forces impact study results. Second, as with RCTs, imbalanced site assignment can confound results. This may occur deliberately in some cases—for example, if sites that develop their implementation plans first are assigned to earlier waves. Even if sites are randomized, however, early and late wave sites may still differ on important characteristics such as size, rurality, and case mix. The resulting confounding between site assignment and time can threaten the internal validity of the study—although, as above, balancing algorithms can reduce this risk. Third, the use of formative evaluation (Elwy, this issue), while useful for maximizing the utility of implementation efforts in a stepped wedge, can mean that late-wave sites receive different implementation strategies than early-wave sites. Similarly, formative evaluation may inform midstream adaptations to the clinical innovation being implemented. In either case, these changes may again threaten internal validity. Overall, then, stepped wedges represent useful tools for evaluating the impact of health interventions that (as with all designs) are subject to certain weaknesses and limitations.
3. Conclusions and Future Directions
Implementation science is focused on maximizing the extent to which effective healthcare practices are adopted, used, and sustained by clinicians, hospitals, and systems. Answering questions in these domains frequently requires different research methods than those employed in traditional efficacy- or effectiveness-oriented randomized clinical trials (RCTs). Implementation-oriented RCTs typically feature cluster or site-level randomization, and emphasize implementation outcomes (e.g. the number of patients receiving the new treatment as intended) rather than traditional clinical outcomes. Hybrid implementation-effectiveness designs incorporate both types of outcomes; more details on these approaches can be found elsewhere in this special issue (Landes, this issue). Other methodological innovations, such as factorial designs or sequential, multiple-assignment randomized trials (SMARTs), can address questions about multi-component or adaptive interventions, still under the umbrella of experimental designs. These types of trials may be especially important for demystifying the “black box” of implementation—that is, determining what components of an implementation strategy are most strongly associated with implementation success. In contrast, pre-post designs with non-equivalent control groups, interrupted time series (ITS), and stepped wedge designs are all examples of quasiexperimental designs that may serve implementation researchers when experimental designs would be inappropriate. A major theme cutting across each of these designs is that there are relative strengths and weaknesses associated with any study design decision. Determining what design to use ultimately will need to be informed by the primary research question to be answered, while simultaneously balancing the need for internal validity, external validity, feasibility, and ethics.
New innovations in study design are constantly being developed and refined. Several such innovations are covered in other articles within this special issue (e.g. Kim et al., this issue). One future direction relevant to the study designs presented in this article is the potential for adaptive trial designs, which allow information gleaned during the trial to inform the adaptation of components like treatment allocation, sample size, or study recruitment in the later phases of the same trial ( Pallmann et al., 2018 ). These designs are becoming increasingly popular in clinical treatment ( Bhatt and Mehta, 2016 ) but could also hold promise for implementation scientists, especially as interest grows in rapid-cycle testing of implementation strategies or efforts. Adaptive designs could potentially be incorporated into both SMART designs and stepped wedge studies, as well as traditional RCTs to further advance implementation science ( Cheung et al., 2015 ). Ideally, these and other innovations will provide researchers with increasingly robust and useful methodologies for answering timely implementation science questions.
- Many implementation science questions can be addressed by fully experimental designs (e.g. randomized controlled trials [RCTs]).
- Implementation trials differ in important ways, however, from more traditional efficacy- or effectiveness-oriented RCTs.
- Adaptive designs represent a recent innovation to determine optimal implementation strategies within a fully experimental framework.
- Quasi-experimental designs can be used to answer implementation science questions in the absence of randomization.
- The choice of study designs in implementation science requires careful consideration of scientific, pragmatic, and ethical issues.
Acknowledgments
This work was supported by Department of Veterans Affairs grants QUE 15–289 (PI: Bauer) and CIN 13403 and National Institutes of Health grant RO1 MH 099898 (PI: Kilbourne).
Publisher's Disclaimer: This is a PDF file of an unedited manuscript that has been accepted for publication. As a service to our customers we are providing this early version of the manuscript. The manuscript will undergo copyediting, typesetting, and review of the resulting proof before it is published in its final citable form. Please note that during the production process errors may be discovered which could affect the content, and all legal disclaimers that apply to the journal pertain.
- Almirall D, Compton SN, Gunlicks-Stoessel M, Duan N, Murphy SA, 2012. Designing a pilot sequential multiple assignment randomized trial for developing an adaptive treatment strategy . Stat Med 31 ( 17 ), 1887–1902. [ PMC free article ] [ PubMed ] [ Google Scholar ]
- Bauer MS, McBride L, Williford WO, Glick H, Kinosian B, Altshuler L, Beresford T, Kilbourne AM, Sajatovic M, Cooperative Studies Program 430 Study, T., 2006. Collaborative care for bipolar disorder: Part II. Impact on clinical outcome, function, and costs . Psychiatr Serv 57 ( 7 ), 937–945. [ PubMed ] [ Google Scholar ]
- Bauer MS, Miller C, Kim B, Lew R, Weaver K, Coldwell C, Henderson K, Holmes S, Seibert MN, Stolzmann K, Elwy AR, Kirchner J, 2016. Partnering with health system operations leadership to develop a controlled implementation trial . Implement Sci 11 , 22. [ PMC free article ] [ PubMed ] [ Google Scholar ]
- Bauer MS, Miller CJ, Kim B, Lew R, Stolzmann K, Sullivan J, Riendeau R, Pitcock J, Williamson A, Connolly S, Elwy AR, Weaver K, 2019. Effectiveness of Implementing a Collaborative Chronic Care Model for Clinician Teams on Patient Outcomes and Health Status in Mental Health: A Randomized Clinical Trial . JAMA Netw Open 2 ( 3 ), e190230. [ PMC free article ] [ PubMed ] [ Google Scholar ]
- Bernal JL, Cummins S, Gasparrini A, 2017. Interrupted time series regression for the evaluation of public health interventions: a tutorial . Int J Epidemiol 46 ( 1 ), 348–355. [ PMC free article ] [ PubMed ] [ Google Scholar ]
- Betran AP, Bergel E, Griffin S, Melo A, Nguyen MH, Carbonell A, Mondlane S, Merialdi M, Temmerman M, Gulmezoglu AM, 2018. Provision of medical supply kits to improve quality of antenatal care in Mozambique: a stepped-wedge cluster randomised trial . Lancet Glob Health 6 ( 1 ), e57–e65. [ PMC free article ] [ PubMed ] [ Google Scholar ]
- Bhatt DL, Mehta C, 2016. Adaptive Designs for Clinical Trials . N Engl J Med 375 ( 1 ), 65–74. [ PubMed ] [ Google Scholar ]
- Bodenheimer T, Wagner EH, Grumbach K, 2002a. Improving primary care for patients with chronic illness . JAMA 288 ( 14 ), 1775–1779. [ PubMed ] [ Google Scholar ]
- Bodenheimer T, Wagner EH, Grumbach K, 2002b. Improving primary care for patients with chronic illness: the chronic care model, Part 2 . JAMA 288 ( 15 ), 1909–1914. [ PubMed ] [ Google Scholar ]
- Brown CA, Lilford RJ, 2006. The stepped wedge trial design: a systematic review . BMC medical research methodology 6 ( 1 ), 54. [ PMC free article ] [ PubMed ] [ Google Scholar ]
- Byiers BJ, Reichle J, Symons FJ, 2012. Single-subject experimental design for evidence-based practice . Am J Speech Lang Pathol 21 ( 4 ), 397–414. [ PMC free article ] [ PubMed ] [ Google Scholar ]
- Cheung YK, Chakraborty B, Davidson KW, 2015. Sequential multiple assignment randomized trial (SMART) with adaptive randomization for quality improvement in depression treatment program . Biometrics 71 ( 2 ), 450–459. [ PMC free article ] [ PubMed ] [ Google Scholar ]
- Collins LM, Dziak JJ, Kugler KC, Trail JB, 2014a. Factorial experiments: efficient tools for evaluation of intervention components . Am J Prev Med 47 ( 4 ), 498–504. [ PMC free article ] [ PubMed ] [ Google Scholar ]
- Collins LM, Dziak JJ, Li R, 2009. Design of experiments with multiple independent variables: a resource management perspective on complete and reduced factorial designs . Psychol Methods 14 ( 3 ), 202–224. [ PMC free article ] [ PubMed ] [ Google Scholar ]
- Collins LM, Murphy SA, Bierman KL, 2004. A conceptual framework for adaptive preventive interventions . Prev Sci 5 ( 3 ), 185–196. [ PMC free article ] [ PubMed ] [ Google Scholar ]
- Collins LM, Murphy SA, Nair VN, Strecher VJ, 2005. A strategy for optimizing and evaluating behavioral interventions . Ann Behav Med 30 ( 1 ), 65–73. [ PubMed ] [ Google Scholar ]
- Collins LM, Murphy SA, Strecher V, 2007. The multiphase optimization strategy (MOST) and the sequential multiple assignment randomized trial (SMART): new methods for more potent eHealth interventions . Am J Prev Med 32 ( 5 Suppl ), S112–118. [ PMC free article ] [ PubMed ] [ Google Scholar ]
- Collins LM, Nahum-Shani I, Almirall D, 2014b. Optimization of behavioral dynamic treatment regimens based on the sequential, multiple assignment, randomized trial (SMART) . Clin Trials 11 ( 4 ), 426–434. [ PMC free article ] [ PubMed ] [ Google Scholar ]
- Coulton S, Perryman K, Bland M, Cassidy P, Crawford M, Deluca P, Drummond C, Gilvarry E, Godfrey C, Heather N, Kaner E, Myles J, Newbury-Birch D, Oyefeso A, Parrott S, Phillips T, Shenker D, Shepherd J, 2009. Screening and brief interventions for hazardous alcohol use in accident and emergency departments: a randomised controlled trial protocol . BMC Health Serv Res 9 , 114. [ PMC free article ] [ PubMed ] [ Google Scholar ]
- Cousins K, Connor JL, Kypri K, 2014. Effects of the Campus Watch intervention on alcohol consumption and related harm in a university population . Drug Alcohol Depend 143 , 120–126. [ PubMed ] [ Google Scholar ]
- Curran GM, Bauer M, Mittman B, Pyne JM, Stetler C, 2012. Effectiveness-implementation hybrid designs: combining elements of clinical effectiveness and implementation research to enhance public health impact . Med Care 50 ( 3 ), 217–226. [ PMC free article ] [ PubMed ] [ Google Scholar ]
- Davey C, Hargreaves J, Thompson JA, Copas AJ, Beard E, Lewis JJ, Fielding KL, 2015. Analysis and reporting of stepped wedge randomised controlled trials: synthesis and critical appraisal of published studies, 2010 to 2014 . Trials 16 ( 1 ), 358. [ PMC free article ] [ PubMed ] [ Google Scholar ]
- Dimick JB, Ryan AM, 2014. Methods for evaluating changes in health care policy: the difference-in- differences approach . JAMA 312 ( 22 ), 2401–2402. [ PubMed ] [ Google Scholar ]
- Eccles M, Grimshaw J, Campbell M, Ramsay C, 2003. Research designs for studies evaluating the effectiveness of change and improvement strategies . Qual Saf Health Care 12 ( 1 ), 47–52. [ PMC free article ] [ PubMed ] [ Google Scholar ]
- Fisher RA, 1925, July Theory of statistical estimation In Mathematical Proceedings of the Cambridge Philosophical Society (Vol. 22, No. 5, pp. 700–725). Cambridge University Press. [ Google Scholar ]
- Fisher RA, 1935. The design of experiments . Oliver and Boyd, Edinburgh. [ Google Scholar ]
- Fretheim A, Soumerai SB, Zhang F, Oxman AD, Ross-Degnan D, 2013. Interrupted time-series analysis yielded an effect estimate concordant with the cluster-randomized controlled trial result . Journal of Clinical Epidemiology 66 ( 8 ), 883–887. [ PubMed ] [ Google Scholar ]
- Fretheim A, Zhang F, Ross-Degnan D, Oxman AD, Cheyne H, Foy R, Goodacre S, Herrin J, Kerse N, McKinlay RJ, Wright A, Soumerai SB, 2015. A reanalysis of cluster randomized trials showed interrupted time-series studies were valuable in health system evaluation . J Clin Epidemiol 68 ( 3 ), 324–333. [ PubMed ] [ Google Scholar ]
- Gaglio B, Shoup JA, Glasgow RE, 2013. The RE-AIM framework: a systematic review of use over time . Am J Public Health 103 ( 6 ), e38–46. [ PMC free article ] [ PubMed ] [ Google Scholar ]
- Gebski V, Ellingson K, Edwards J, Jernigan J, Kleinbaum D, 2012. Modelling interrupted time series to evaluate prevention and control of infection in healthcare . Epidemiol Infect 140 ( 12 ), 2131–2141. [ PMC free article ] [ PubMed ] [ Google Scholar ]
- Glasgow RE, Vogt TM, Boles SM, 1999. Evaluating the public health impact of health promotion interventions: the RE-AIM framework . Am J Public Health 89 ( 9 ), 1322–1327. [ PMC free article ] [ PubMed ] [ Google Scholar ]
- Hanani H, 1961. The existence and construction of balanced incomplete block designs . The Annals of Mathematical Statistics 32 ( 2 ), 361–386. [ Google Scholar ]
- Hanbury A, Farley K, Thompson C, Wilson PM, Chambers D, Holmes H, 2013. Immediate versus sustained effects: interrupted time series analysis of a tailored intervention . Implement Sci 8 , 130. [ PMC free article ] [ PubMed ] [ Google Scholar ]
- Handley MA, Lyles CR, McCulloch C, Cattamanchi A, 2018. Selecting and Improving Quasi-Experimental Designs in Effectiveness and Implementation Research . Annu Rev Public Health 39 , 5–25. [ PMC free article ] [ PubMed ] [ Google Scholar ]
- Hussey MA, Hughes JP, 2007. Design and analysis of stepped wedge cluster randomized trials . Contemp Clin Trials 28 ( 2 ), 182–191. [ PubMed ] [ Google Scholar ]
- Kilbourne AM, Almirall D, Eisenberg D, Waxmonsky J, Goodrich DE, Fortney JC, Kirchner JE, Solberg LI, Main D, Bauer MS, Kyle J, Murphy SA, Nord KM, Thomas MR, 2014a. Protocol: Adaptive Implementation of Effective Programs Trial (ADEPT): cluster randomized SMART trial comparing a standard versus enhanced implementation strategy to improve outcomes of a mood disorders program . Implement Sci 9 , 132. [ PMC free article ] [ PubMed ] [ Google Scholar ]
- Kilbourne AM, Almirall D, Goodrich DE, Lai Z, Abraham KM, Nord KM, Bowersox NW, 2014b. Enhancing outreach for persons with serious mental illness: 12-month results from a cluster randomized trial of an adaptive implementation strategy . Implement Sci 9 , 163. [ PMC free article ] [ PubMed ] [ Google Scholar ]
- Kilbourne AM, Bramlet M, Barbaresso MM, Nord KM, Goodrich DE, Lai Z, Post EP, Almirall D, Verchinina L, Duffy SA, Bauer MS, 2014c. SMI life goals: description of a randomized trial of a collaborative care model to improve outcomes for persons with serious mental illness . Contemp Clin Trials 39 ( 1 ), 74–85. [ PMC free article ] [ PubMed ] [ Google Scholar ]
- Kilbourne AM, Goodrich DE, Lai Z, Clogston J, Waxmonsky J, Bauer MS, 2012a. Life Goals Collaborative Care for patients with bipolar disorder and cardiovascular disease risk . Psychiatr Serv 63 ( 12 ), 1234–1238. [ PMC free article ] [ PubMed ] [ Google Scholar ]
- Kilbourne AM, Goodrich DE, Nord KM, Van Poppelen C, Kyle J, Bauer MS, Waxmonsky JA, Lai Z, Kim HM, Eisenberg D, Thomas MR, 2015. Long-Term Clinical Outcomes from a Randomized Controlled Trial of Two Implementation Strategies to Promote Collaborative Care Attendance in Community Practices . Adm Policy Ment Health 42 ( 5 ), 642–653. [ PMC free article ] [ PubMed ] [ Google Scholar ]
- Kilbourne AM, Neumann MS, Pincus HA, Bauer MS, Stall R, 2007. Implementing evidence-based interventions in health care: application of the replicating effective programs framework . Implement Sci 2 , 42. [ PMC free article ] [ PubMed ] [ Google Scholar ]
- Kilbourne AM, Neumann MS, Waxmonsky J, Bauer MS, Kim HM, Pincus HA, Thomas M, 2012b. Public-academic partnerships: evidence-based implementation: the role of sustained community-based practice and research partnerships . Psychiatr Serv 63 ( 3 ), 205–207. [ PMC free article ] [ PubMed ] [ Google Scholar ]
- Kilbourne AM, Post EP, Nossek A, Drill L, Cooley S, Bauer MS, 2008. Improving medical and psychiatric outcomes among individuals with bipolar disorder: a randomized controlled trial . Psychiatr Serv 59 ( 7 ), 760–768. [ PubMed ] [ Google Scholar ]
- Kirchner JE, Ritchie MJ, Pitcock JA, Parker LE, Curran GM, Fortney JC, 2014. Outcomes of a partnered facilitation strategy to implement primary care-mental health . J Gen Intern Med 29 Suppl 4 , 904–912. [ PMC free article ] [ PubMed ] [ Google Scholar ]
- Lei H, Nahum-Shani I, Lynch K, Oslin D, Murphy SA, 2012. A “SMART” design for building individualized treatment sequences . Annu Rev Clin Psychol 8 , 21–48. [ PMC free article ] [ PubMed ] [ Google Scholar ]
- Lew RA, Miller CJ, Kim B, Wu H, Stolzmann K, Bauer MS, 2019. A robust method to reduce imbalance for site-level randomized controlled implementation trial designs . Implementation Sci , 14 , 46. [ PMC free article ] [ PubMed ] [ Google Scholar ]
- Morgan CJ, 2018. Reducing bias using propensity score matching . J Nucl Cardiol 25 ( 2 ), 404–406. [ PubMed ] [ Google Scholar ]
- Morton V, Torgerson DJ, 2003. Effect of regression to the mean on decision making in health care . BMJ 326 ( 7398 ), 1083–1084. [ PMC free article ] [ PubMed ] [ Google Scholar ]
- Nahum-Shani I, Qian M, Almirall D, Pelham WE, Gnagy B, Fabiano GA, Waxmonsky JG, Yu J, Murphy SA, 2012. Experimental design and primary data analysis methods for comparing adaptive interventions . Psychol Methods 17 ( 4 ), 457–477. [ PMC free article ] [ PubMed ] [ Google Scholar ]
- NeCamp T, Kilbourne A, Almirall D, 2017. Comparing cluster-level dynamic treatment regimens using sequential, multiple assignment, randomized trials: Regression estimation and sample size considerations . Stat Methods Med Res 26 ( 4 ), 1572–1589. [ PMC free article ] [ PubMed ] [ Google Scholar ]
- Neumann MS, Sogolow ED, 2000. Replicating effective programs: HIV/AIDS prevention technology transfer . AIDS Educ Prev 12 ( 5 Suppl ), 35–48. [ PubMed ] [ Google Scholar ]
- Pallmann P, Bedding AW, Choodari-Oskooei B, Dimairo M, Flight L, Hampson LV, Holmes J, Mander AP, Odondi L.o., Sydes MR, Villar SS, Wason JMS, Weir CJ, Wheeler GM, Yap C, Jaki T, 2018. Adaptive designs in clinical trials: why use them, and how to run and report them . BMC medicine 16 ( 1 ), 29–29. [ PMC free article ] [ PubMed ] [ Google Scholar ]
- Pape UJ, Millett C, Lee JT, Car J, Majeed A, 2013. Disentangling secular trends and policy impacts in health studies: use of interrupted time series analysis . J R Soc Med 106 ( 4 ), 124–129. [ PMC free article ] [ PubMed ] [ Google Scholar ]
- Pellegrini CA, Hoffman SA, Collins LM, Spring B, 2014. Optimization of remotely delivered intensive lifestyle treatment for obesity using the Multiphase Optimization Strategy: Opt-IN study protocol . Contemp Clin Trials 38 ( 2 ), 251–259. [ PMC free article ] [ PubMed ] [ Google Scholar ]
- Penfold RB, Zhang F, 2013. Use of Interrupted Time Series Analysis in Evaluating Health Care Quality Improvements . Academic Pediatrics 13 ( 6, Supplement ), S38–S44. [ PubMed ] [ Google Scholar ]
- Pridemore WA, Snowden AJ, 2009. Reduction in suicide mortality following a new national alcohol policy in Slovenia: an interrupted time-series analysis . Am J Public Health 99 ( 5 ), 915–920. [ PMC free article ] [ PubMed ] [ Google Scholar ]
- Proctor E, Silmere H, Raghavan R, Hovmand P, Aarons G, Bunger A, Griffey R, Hensley M, 2011. Outcomes for implementation research: conceptual distinctions, measurement challenges, and research agenda . Adm Policy Ment Health 38 ( 2 ), 65–76. [ PMC free article ] [ PubMed ] [ Google Scholar ]
- Robson D, Spaducci G, McNeill A, Stewart D, Craig TJK, Yates M, Szatkowski L, 2017. Effect of implementation of a smoke-free policy on physical violence in a psychiatric inpatient setting: an interrupted time series analysis . Lancet Psychiatry 4 ( 7 ), 540–546. [ PubMed ] [ Google Scholar ]
- Schildcrout JS, Schisterman EF, Mercaldo ND, Rathouz PJ, Heagerty PJ, 2018. Extending the Case-Control Design to Longitudinal Data: Stratified Sampling Based on Repeated Binary Outcomes . Epidemiology 29 ( 1 ), 67–75. [ PMC free article ] [ PubMed ] [ Google Scholar ]
- Shadish WR, Cook Thomas D., Campbell Donald T, 2002. Experimental and quasi-experimental designs for generalized causal inference . Houghton Miffflin Company, Boston, MA. [ Google Scholar ]
- Simon GE, Ludman EJ, Bauer MS, Unutzer J, Operskalski B, 2006. Long-term effectiveness and cost of a systematic care program for bipolar disorder . Arch Gen Psychiatry 63 ( 5 ), 500–508. [ PubMed ] [ Google Scholar ]
- Stetler CB, Legro MW, Rycroft-Malone J, Bowman C, Curran G, Guihan M, Hagedorn H, Pineros S, Wallace CM, 2006. Role of “external facilitation” in implementation of research findings: a qualitative evaluation of facilitation experiences in the Veterans Health Administration . Implement Sci 1 , 23. [ PMC free article ] [ PubMed ] [ Google Scholar ]
- Taljaard M, McKenzie JE, Ramsay CR, Grimshaw JM, 2014. The use of segmented regression in analysing interrupted time series studies: an example in pre-hospital ambulance care . Implement Sci 9 , 77. [ PMC free article ] [ PubMed ] [ Google Scholar ]
- Wagner AK, Soumerai SB, Zhang F, Ross-Degnan D, 2002. Segmented regression analysis of interrupted time series studies in medication use research . J Clin Pharm Ther 27 ( 4 ), 299–309. [ PubMed ] [ Google Scholar ]
- Wagner EH, Austin BT, Von Korff M, 1996. Organizing care for patients with chronic illness . Milbank Q 74 ( 4 ), 511–544. [ PubMed ] [ Google Scholar ]
- Wyrick DL, Rulison KL, Fearnow-Kenney M, Milroy JJ, Collins LM, 2014. Moving beyond the treatment package approach to developing behavioral interventions: addressing questions that arose during an application of the Multiphase Optimization Strategy (MOST) . Transl Behav Med 4 ( 3 ), 252–259. [ PMC free article ] [ PubMed ] [ Google Scholar ]
- Voxco Online
- Voxco Panel Management
- Voxco Panel Portal
- Voxco Audience
- Voxco Mobile Offline
- Voxco Dialer Cloud
- Voxco Dialer On-premise
- Voxco TCPA Connect
- Voxco Analytics
- Voxco Text & Sentiment Analysis

- 40+ question types
- Drag-and-drop interface
- Skip logic and branching
- Multi-lingual survey
- Text piping
- Question library
- CSS customization
- White-label surveys
- Customizable ‘Thank You’ page
- Customizable survey theme
- Reminder send-outs
- Survey rewards
- Social media
- Website surveys
- Correlation analysis
- Cross-tabulation analysis
- Trend analysis
- Real-time dashboard
- Customizable report
- Email address validation
- Recaptcha validation
- SSL security
Take a peek at our powerful survey features to design surveys that scale discoveries.
Download feature sheet.
- Hospitality
- Academic Research
- Customer Experience
- Employee Experience
- Product Experience
- Market Research
- Social Research
- Data Analysis
Explore Voxco
Need to map Voxco’s features & offerings? We can help!
Watch a Demo
Download Brochures
Get a Quote
- NPS Calculator
- CES Calculator
- A/B Testing Calculator
- Margin of Error Calculator
- Sample Size Calculator
- CX Strategy & Management Hub
- Market Research Hub
- Patient Experience Hub
- Employee Experience Hub
- NPS Knowledge Hub
- Market Research Guide
- Customer Experience Guide
- Survey Research Guides
- Survey Template Library
- Webinars and Events
- Feature Sheets
- Try a sample survey
- Professional Services

Get exclusive insights into research trends and best practices from top experts! Access Voxco’s ‘State of Research Report 2024 edition’ .
We’ve been avid users of the Voxco platform now for over 20 years. It gives us the flexibility to routinely enhance our survey toolkit and provides our clients with a more robust dataset and story to tell their clients.
VP Innovation & Strategic Partnerships, The Logit Group
- Client Stories
- Voxco Reviews
- Why Voxco Research?
- Careers at Voxco
- Vulnerabilities and Ethical Hacking
Explore Regional Offices
- Survey Software The world’s leading omnichannel survey software
- Online Survey Tools Create sophisticated surveys with ease.
- Mobile Offline Conduct efficient field surveys.
- Text Analysis
- Close The Loop
- Automated Translations
- NPS Dashboard
- CATI Manage high volume phone surveys efficiently
- Cloud/On-premise Dialer TCPA compliant Cloud on-premise dialer
- IVR Survey Software Boost productivity with automated call workflows.
- Analytics Analyze survey data with visual dashboards
- Panel Manager Nurture a loyal community of respondents.
- Survey Portal Best-in-class user friendly survey portal.
- Voxco Audience Conduct targeted sample research in hours.
- Predictive Analytics
- Customer 360
- Customer Loyalty
- Fraud & Risk Management
- AI/ML Enablement Services
- Credit Underwriting

Find the best survey software for you! (Along with a checklist to compare platforms)
Get Buyer’s Guide
- 100+ question types
- SMS surveys
- Financial Services
- Banking & Financial Services
- Retail Solution
- Risk Management
- Customer Lifecycle Solutions
- Net Promoter Score
- Customer Behaviour Analytics
- Customer Segmentation
- Data Unification
Explore Voxco
Watch a Demo
Download Brochures
- CX Strategy & Management Hub
- The Voxco Guide to Customer Experience
- Professional services
- Blogs & White papers
- Case Studies
Find the best customer experience platform
Uncover customer pain points, analyze feedback and run successful CX programs with the best CX platform for your team.
Get the Guide Now

VP Innovation & Strategic Partnerships, The Logit Group
- Why Voxco Intelligence?
- Our clients
- Client stories
- Featuresheets
Explaining Quasi-Experimental Design And Its Various Methods
- September 27, 2021
SHARE THE ARTICLE ON
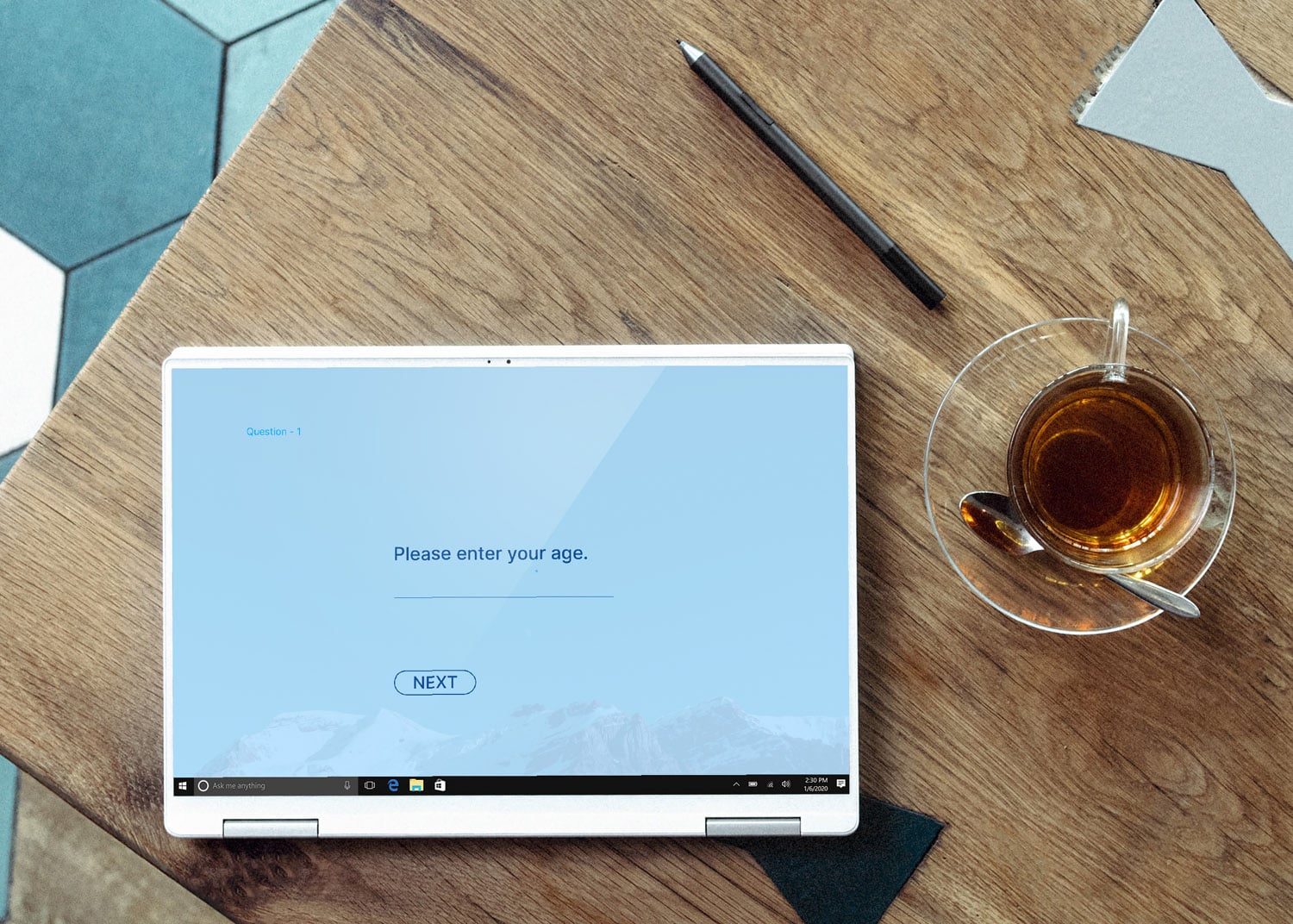
As you strive to uncover causal (cause-and-effect) relationships between variables, you may often encounter ethical or practical constraints while conducting controlled experiments.
Quasi-experimental design steps in as a powerful alternative that helps you overcome these challenges and offer valuable insights.
In this blog, we’ll look into its characteristics, examples, types, and how it differs from true-experimental research design. The purpose of this blog is to understand how this research methodology bridges the gap between a fully controlled experiment and a purely observational study.
What Is Quasi-Experimental Design?
A quasi-experimental design is pretty much different from an experimental design, except for the fact that they both manifest the cause-effect relationship between the independent and dependent variables .
So, how is quasi-experimental design different?
Well, unlike experimental design, quasi-experiments do not include random assignments of participants meaning, the participants are placed in the experimental groups based on some of the other criteria. Let us take a deeper look at how quasi-experimental design works.
Read how Voxco helped Modus Research increase research efficiency with Voxco Online, CATI, IVR, and panel systems.
Experimental design has three characteristics:, 1. manipulation.
Manipulation simply means evaluating the effect of the independent variable on the dependent variable.
Example: A chocolate and a crying child.
- Independent variable: Type of chocolate.
- Dependent variable: The child is crying for chocolate.
So manipulation means the effect of an independent variable, that is, chocolate, on the dependent variable, that is, the crying child. In short, you are using an outside source on the dependent variable. This proves that after getting the chocolate (independent variable), the child stops crying (dependent variable).
2. Randomization
Randomization means sudden selection without any plan. Example: A lottery system. The lottery numbers are announced at random so everyone who buys a lottery has an equal chance. Hence, it means you select a sample without any plan and everyone has an equal chance of getting into any one of the experimental groups.
This means using a control group in the experiment. In this group, researchers keep the independent variable constant. This control group is then compared to a treatment group, where the researchers have changed the independent variable. Well, for obvious reasons, researchers are more interested in the treatment group as it has a scope of change in the dependent variable.
Example: You want to find out whether the workers work more efficiently if there is a pay raise.
Here, you will put certain workers in the treatment group and some in the control group.
- Treatment group: You pay more to the workers
- Control group: You don’t pay any extra to the workers, and things remain the same.
By comparing these two groups, you understand that the workers who got paid more worked more efficiently than the workers who didn’t.
As for the quasi-experimental design, the manipulation characteristic of the true experiment remains the same. However randomization or control characteristics are present in contrast to each other or none at all.
Hence, these experiments are conducted where random selection is difficult or even impossible. The quasi-experiment does not include random assignment, as the independent variable is manipulated before the measurement of the dependent variable.
See how easily you can create, test, distribute, and design the surveys.
- 50+ question types
- Advanced logic
- White-label
- Auto-translations in 100+ languages
What are the types of quasi-experimental design?
Amongst all the various types of quasi-experimental design, let us first get to know two main types of quasi-experimental design:
- Non-equivalent group design (NEGD)
- Regression discontinuity design
1. Non-Equivalent Group Design (NEGD)
You can picture non-equivalent group designs as a mixture of both true experimental design as well as quasi-experimental design. The reason is, that it uses both their qualities. Like a true experiment, NEGD uses the pre-existing groups that we feel are similar, namely treatment and control groups. However it lacks the randomization characteristic of a quasi-experiment.
While grouping, researchers see to it that they are not influenced by any third variables or confounding variables. Hence, the groups are as similar as possible. For example, when talking about political study, we might select groups that are more similar to each other.
Let us understand it with an example:
Take the previous example where you studied whether the workers work more efficiently if there is a pay rise.
You give a pre-test to the workers in one company while their pay is normal. Then you put them under the treatment group where they work and their pay is being increased. After the experiment, you take their post-test about their experience and attitude towards their work.
Later, you give the same pre-test to the workers from a similar company and put them in a control group where their pay is not raised, and then conduct a post-test.
Hence, the Non-equivalent design has a name to remind us that the groups are not equivalent and are not assigned on a random practice.
2. Regression discontinuity design or RDD
Regression discontinuity design, or RDD, is a quasi-experimental design technique that computes the influence of a treatment or intervention. It does so by using a mechanism that assigns the treatment based on eligibility, known as a “cut-off”.
So the participants above the cut-off get to be in a treatment group and those below the cut-off doesn’t. Although the difference between these two groups is negligible.
Let’s take a look at an example:
A school wants to grant a $50 scholarship to students, depending on an independent test taken to measure their intellect and household.
Those who pass the test will get a scholarship. However, the students who are just below the cut-off and those just above it can be considered similar. We can say the differences in their scores occurred randomly. Hence you can keep on studying both groups to get a long-term outcome.
One-stop-shop to gather, measure, uncover, and act on insightful data.
Curious About The Price? Click Below To Get A Personalized Quote.
What are the advantages of a quasi-experimental design?
The quasi-experiment design, also known as external validity, can be perfect for determining what is best for the population. Let’s look at some advantages of this research methodology type.
- It gives the researchers power over the variables by being able to control them.
- The quasi-experiment method can be combined with other experimental methods too.
- It provides transferability to a greater extent.
- It is an intuitive process that is well-shaped by the researchers.
- Involves real-world problems and solutions and not any artificial ones.
- Offers better control over the third variable, known as the confounding variable, which influences the cause and effect.
What are the disadvantages of a quasi-experimental design?
As a research design, it is bound to have some limitations, let’s look at some of the disadvantages you should consider when selecting the design for your research.
- It serves less internal validity than true experiments.
- Due to no randomization, you cannot tell for sure that the confounding or third variable is eradicated.
- It has scope for human errors.
- It can allow the researcher’s personal bias to get involved.
- Human responses are difficult to measure; hence, there is a chance that the results will be produced artificially.
- Using old or backdated data can be incorrect and inadequate for the study.

Other Quasi-Experimental Designs
Apart from the above-mentioned types, there are other equally important quasi-experimental designs that have different applications depending on their characteristics and their respective design notations .
Let’s take a look at all of them in detail:
1. The proxy Pre-Test Design
The proxy pre-test design works the same as a typical pre-test and post-test design. Except, the pre-test here is conducted AFTER the treatment is given. Got confused? How is it pre-test if it is conducted after? Well, the keyword here is “proxy”. These proxy variables tell where the groups would have been in the pre-test.
You ask the group after their program about how they’d have answered the same questions before their treatment. Although, this technique is not very reliable as we cannot expect the participants to remember how they felt a long time ago, and we surely cannot tell if they are faking their answers.
As this design is highly not recommended, you can use this under some unavoidable circumstances like the treatment has already begun and you couldn’t take the pre-test.
In such cases, this approach will help rather than depending totally on the post-test.

You want to study the workers’ performance after the pay rise. But you were called to do the pre-test after the program had started. In that case, you will have to take the post-test and study a proxy variable, such as productivity from the time before the program and after the program
2. The Separate Pre-Post Samples Design
This technique also works on the pre-test and post-test designs. The difference is that the participants you used for the pre-test won’t be the same for the post-test.

You want to study the client satisfaction of two similar companies. You take one for the treatment and the other for the control. Let’s say you conducted a pre-test in both companies at the same time and then begin your experiment.
After a while, when the program is complete, you go to take a post-test. Now, the set of clients you take in for the test is going to be different than the pre-test ones, the reason being clients change after the course of the period.
In this case, you cannot derive one-to-one results, but you can tell the average client satisfaction in both companies.
3. The Double Pre-Test Design
The double pre-test design is a very robust quasi-experimental design designed to rule out the internal validity problem we had with the non-equivalent design. It has two pre-tests before the program. It is when the two groups are progressing at a different pace that you should change from pre-test 1 to pre-test 2.
Due to the benefit of two pre-tests, you can determine the null case scenario. It assumes the difference between the scores in the pre-test and post-test is due to random chance, as it doesn’t allow one person to take the pre-test twice.
4. The Switching Replications Design
In the switching replications design, as the name suggests, the role of the group is switched. It follows the same treatment-control group pattern, except it has two phases.
Phase 1: Both the groups are pre-tested, then they undergo their respective program. Later they are post-tested.
Phase 2: In this phase, an original treatment group is now a control group and an original control group is now a treatment group.

The main benefit of inculcating this design is that it proves strong against internal validation as well as external validation. The reason is that two parallel implementations of the program allow all the participants to experience the program, making it ethically strong as well.
5.The Non-equivalent Dependent Variables (NEDV) Design
NEDV design, in its simplest form, is not the most reliable one and does not work wonders against internal validity either. But then, what is the use of NEDV?
Well, sometimes the treatment group may be affected by some external factors. Hence, there are two pre and post-tests applied to the participants, one regarding the treatment itself and the other regarding that external variable.

Wait, how about we take an example to understand this?
Let us say you started a program to test history teaching techniques. You design standards tests for history (treatment group) and show historical movies (external variable). Later in the post-tests, you find out that along with the history scores, students’ interest in historical movies has also increased, suggesting that showing historical movies has influenced students to study the subject.
6. The regression Point Displacement (RPD) Design
RPD design is used when measures for already existing groups are available and can be compared with those for treatment groups. The treatment group is the only group present, and both pre-test and post-tests are conducted.
This method is widely beneficial for larger groups, communities, and companies. RPD works by comparing a single program unit with a larger comparison unit.

Consider a community-based COVID awareness program. It has been decided to start the initiative in a particular town or a vast district. The representatives forecast the active cases in that town and use the remaining towns as a comparison. Now rather than giving the average for the rest of the towns’ COVID cases, they show their count.
Looking for World’s best Survey Platform?
Voxco is the leading survey software trusted by 450+ brands across 40+ countries., when to use a quasi-experimental design.
All that studying but shouldn’t you know when to perfectly use quasi-experiments? Well, now as we are to the end of the matter, let us discuss when to use quasi-experiments and for what reasons.
1. For ethical reasons
Remember when we discussed the “willingness” of obese people to participate in the experiment? That is when ethics start to matter. You cannot go on putting random participants under treatments as you do with true experiments.
Especially when it directly affects the participants’ lives. One of the best examples is Oregon Health Study where health insurance is given to certain people while others were restricted from it.
2. For practical reasons
True experiments, despite having higher internal validity, can be expensive. Also, it requires enough participants so that the true experiment can be justified. Unlike that, in a quasi-experiment, you can use the already gathered data.
The data is collected and paid by some strong entity, say the government, and you use that to study your questions.
Well, that concludes our guide. If you’re looking for extensive research tools, Voxco offers a complete market research tool kit that includes market research trends, a guide to online surveys, an agile market research guide, and five market research templates.
Also read: Experimental Research .

Differences between quasi-experiments and true experiments
The above description is overwhelming? Don’t worry. Here is the straight difference between the quasi-experiments and true experiments so that you can understand how both vary from each other.
TRUE EXPERIMENT | QUASI EXPERIMENT |
Participants are assigned randomly to the experimental groups. | Participants are not randomly assigned to the experimental groups. |
Participants have an equal chance of getting into any of the experimental groups. | Participants are categorized and then put into a respective experimental group. |
Researchers design the treatment participants will go through. | Researchers do not design a treatment. |
There are no various groups of treatments. | Researchers study the existing groups of treatments received. |
Includes control groups and treatment groups. | Does not necessarily require control groups, apart from the fact they are generally used. |
It does not include a pre-test. | It includes a pre-test. |
Example of true-experimental design:
While starting the true experiment, you assign some participants in the treatment group where they are fed only junk food. While the other half of the participants go to the control group , where they have their regular ongoing diet (standard course).
You decide to take obese people’s reports every day after their meals to note down their health and discomfort, if any.
However, participants who are assigned to the treatment group would not like to change their diet to complete junk food for personal reasons. In this case, you cannot conduct a true experiment against their will. This is when quasi-experiment comes in.
Example of quasi-experimental design:
While talking to the participants, you find out that some of the participants want to try the junk food effect while the others don’t want to experiment with their diet and choose to stick with a regular diet.
You can now assign already existing groups to the participants according to their choices. Study how the regular consumption of junk food affects the obese from that group.
Here, you did not assign groups to the random participants and can be confident about the difference occurring due to the conducted experiment.
High Performer in G2’s Winter Reports
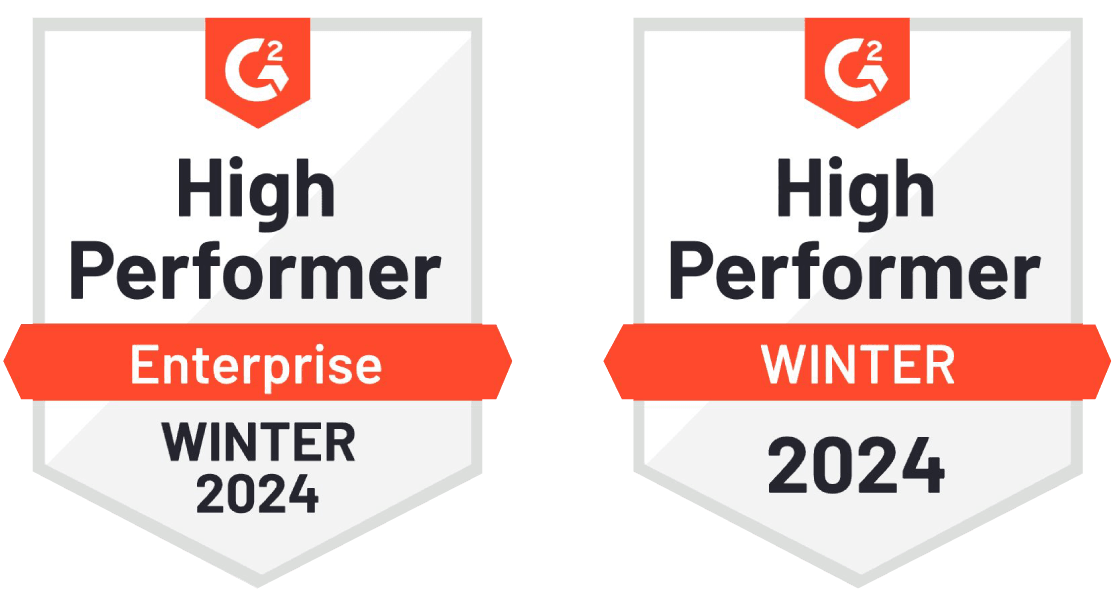
Quasi-experimental design has a unique approach that allows you to uncover causal relationship between variables when controlled experiments are not feasible or ethical. While it may not posses the level of control and randomization that you have when performing true-experiment; quasi-experimental research design enables you to make meaningful contribution by providing valuable insights to various fields.
Explore Voxco Survey Software
+ Omnichannel Survey Software
+ Online Survey Software
+ CATI Survey Software
+ IVR Survey Software
+ Market Research Tool
+ Customer Experience Tool
+ Product Experience Software
+ Enterprise Survey Software

Cloud Hosting: 5 Things You Need to Know for Phone Survey Software
Things You Need To Know About Cloud Phone Survey System SHARE THE ARTICLE ON Table of Contents When organizations shop around for a phone survey
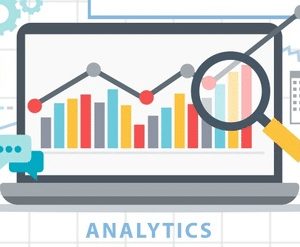
Business Research
Business Research Try a free Voxco Online sample survey! Unlock your Sample Survey SHARE THE ARTICLE ON The goal of any business is sales and

Building a research panel
How to build a market research panel? Transform your insight generation process Use our in-depth online survey guide to create an actionable feedback collection survey
The Role of Sentiment Analysis in Competitive Intelligence & Market Research
The Role of Sentiment Analysis in Competitive Intelligence & Market Research SHARE THE ARTICLE ON Table of Contents When it comes to customer experience, great

How is sentiment analysis done and challenges faced
Sentiment Analysis SHARE THE ARTICLE ON Table of Contents Sentiment analysis is a subset of text research, sometimes known as mining. It employs a combination

The 5 Key Steps in an Effective Risk Management Process
The 5 Key Steps in an Effective Risk Management Process SHARE THE ARTICLE ON Table of Contents Introduction Any business that wants to succeed and
We use cookies in our website to give you the best browsing experience and to tailor advertising. By continuing to use our website, you give us consent to the use of cookies. Read More
Name | Domain | Purpose | Expiry | Type |
---|---|---|---|---|
hubspotutk | www.voxco.com | HubSpot functional cookie. | 1 year | HTTP |
lhc_dir_locale | amplifyreach.com | --- | 52 years | --- |
lhc_dirclass | amplifyreach.com | --- | 52 years | --- |
Name | Domain | Purpose | Expiry | Type |
---|---|---|---|---|
_fbp | www.voxco.com | Facebook Pixel advertising first-party cookie | 3 months | HTTP |
__hstc | www.voxco.com | Hubspot marketing platform cookie. | 1 year | HTTP |
__hssrc | www.voxco.com | Hubspot marketing platform cookie. | 52 years | HTTP |
__hssc | www.voxco.com | Hubspot marketing platform cookie. | Session | HTTP |
Name | Domain | Purpose | Expiry | Type |
---|---|---|---|---|
_gid | www.voxco.com | Google Universal Analytics short-time unique user tracking identifier. | 1 days | HTTP |
MUID | bing.com | Microsoft User Identifier tracking cookie used by Bing Ads. | 1 year | HTTP |
MR | bat.bing.com | Microsoft User Identifier tracking cookie used by Bing Ads. | 7 days | HTTP |
IDE | doubleclick.net | Google advertising cookie used for user tracking and ad targeting purposes. | 2 years | HTTP |
_vwo_uuid_v2 | www.voxco.com | Generic Visual Website Optimizer (VWO) user tracking cookie. | 1 year | HTTP |
_vis_opt_s | www.voxco.com | Generic Visual Website Optimizer (VWO) user tracking cookie that detects if the user is new or returning to a particular campaign. | 3 months | HTTP |
_vis_opt_test_cookie | www.voxco.com | A session (temporary) cookie used by Generic Visual Website Optimizer (VWO) to detect if the cookies are enabled on the browser of the user or not. | 52 years | HTTP |
_ga | www.voxco.com | Google Universal Analytics long-time unique user tracking identifier. | 2 years | HTTP |
_uetsid | www.voxco.com | Microsoft Bing Ads Universal Event Tracking (UET) tracking cookie. | 1 days | HTTP |
vuid | vimeo.com | Vimeo tracking cookie | 2 years | HTTP |
Name | Domain | Purpose | Expiry | Type |
---|---|---|---|---|
__cf_bm | hubspot.com | Generic CloudFlare functional cookie. | Session | HTTP |
Name | Domain | Purpose | Expiry | Type |
---|---|---|---|---|
_gcl_au | www.voxco.com | --- | 3 months | --- |
_gat_gtag_UA_3262734_1 | www.voxco.com | --- | Session | --- |
_clck | www.voxco.com | --- | 1 year | --- |
_ga_HNFQQ528PZ | www.voxco.com | --- | 2 years | --- |
_clsk | www.voxco.com | --- | 1 days | --- |
visitor_id18452 | pardot.com | --- | 10 years | --- |
visitor_id18452-hash | pardot.com | --- | 10 years | --- |
lpv18452 | pi.pardot.com | --- | Session | --- |
lhc_per | www.voxco.com | --- | 6 months | --- |
_uetvid | www.voxco.com | --- | 1 year | --- |

Want to create or adapt books like this? Learn more about how Pressbooks supports open publishing practices.
7.3 Quasi-Experimental Research
Learning objectives.
- Explain what quasi-experimental research is and distinguish it clearly from both experimental and correlational research.
- Describe three different types of quasi-experimental research designs (nonequivalent groups, pretest-posttest, and interrupted time series) and identify examples of each one.
The prefix quasi means “resembling.” Thus quasi-experimental research is research that resembles experimental research but is not true experimental research. Although the independent variable is manipulated, participants are not randomly assigned to conditions or orders of conditions (Cook & Campbell, 1979). Because the independent variable is manipulated before the dependent variable is measured, quasi-experimental research eliminates the directionality problem. But because participants are not randomly assigned—making it likely that there are other differences between conditions—quasi-experimental research does not eliminate the problem of confounding variables. In terms of internal validity, therefore, quasi-experiments are generally somewhere between correlational studies and true experiments.
Quasi-experiments are most likely to be conducted in field settings in which random assignment is difficult or impossible. They are often conducted to evaluate the effectiveness of a treatment—perhaps a type of psychotherapy or an educational intervention. There are many different kinds of quasi-experiments, but we will discuss just a few of the most common ones here.
Nonequivalent Groups Design
Recall that when participants in a between-subjects experiment are randomly assigned to conditions, the resulting groups are likely to be quite similar. In fact, researchers consider them to be equivalent. When participants are not randomly assigned to conditions, however, the resulting groups are likely to be dissimilar in some ways. For this reason, researchers consider them to be nonequivalent. A nonequivalent groups design , then, is a between-subjects design in which participants have not been randomly assigned to conditions.
Imagine, for example, a researcher who wants to evaluate a new method of teaching fractions to third graders. One way would be to conduct a study with a treatment group consisting of one class of third-grade students and a control group consisting of another class of third-grade students. This would be a nonequivalent groups design because the students are not randomly assigned to classes by the researcher, which means there could be important differences between them. For example, the parents of higher achieving or more motivated students might have been more likely to request that their children be assigned to Ms. Williams’s class. Or the principal might have assigned the “troublemakers” to Mr. Jones’s class because he is a stronger disciplinarian. Of course, the teachers’ styles, and even the classroom environments, might be very different and might cause different levels of achievement or motivation among the students. If at the end of the study there was a difference in the two classes’ knowledge of fractions, it might have been caused by the difference between the teaching methods—but it might have been caused by any of these confounding variables.
Of course, researchers using a nonequivalent groups design can take steps to ensure that their groups are as similar as possible. In the present example, the researcher could try to select two classes at the same school, where the students in the two classes have similar scores on a standardized math test and the teachers are the same sex, are close in age, and have similar teaching styles. Taking such steps would increase the internal validity of the study because it would eliminate some of the most important confounding variables. But without true random assignment of the students to conditions, there remains the possibility of other important confounding variables that the researcher was not able to control.
Pretest-Posttest Design
In a pretest-posttest design , the dependent variable is measured once before the treatment is implemented and once after it is implemented. Imagine, for example, a researcher who is interested in the effectiveness of an antidrug education program on elementary school students’ attitudes toward illegal drugs. The researcher could measure the attitudes of students at a particular elementary school during one week, implement the antidrug program during the next week, and finally, measure their attitudes again the following week. The pretest-posttest design is much like a within-subjects experiment in which each participant is tested first under the control condition and then under the treatment condition. It is unlike a within-subjects experiment, however, in that the order of conditions is not counterbalanced because it typically is not possible for a participant to be tested in the treatment condition first and then in an “untreated” control condition.
If the average posttest score is better than the average pretest score, then it makes sense to conclude that the treatment might be responsible for the improvement. Unfortunately, one often cannot conclude this with a high degree of certainty because there may be other explanations for why the posttest scores are better. One category of alternative explanations goes under the name of history . Other things might have happened between the pretest and the posttest. Perhaps an antidrug program aired on television and many of the students watched it, or perhaps a celebrity died of a drug overdose and many of the students heard about it. Another category of alternative explanations goes under the name of maturation . Participants might have changed between the pretest and the posttest in ways that they were going to anyway because they are growing and learning. If it were a yearlong program, participants might become less impulsive or better reasoners and this might be responsible for the change.
Another alternative explanation for a change in the dependent variable in a pretest-posttest design is regression to the mean . This refers to the statistical fact that an individual who scores extremely on a variable on one occasion will tend to score less extremely on the next occasion. For example, a bowler with a long-term average of 150 who suddenly bowls a 220 will almost certainly score lower in the next game. Her score will “regress” toward her mean score of 150. Regression to the mean can be a problem when participants are selected for further study because of their extreme scores. Imagine, for example, that only students who scored especially low on a test of fractions are given a special training program and then retested. Regression to the mean all but guarantees that their scores will be higher even if the training program has no effect. A closely related concept—and an extremely important one in psychological research—is spontaneous remission . This is the tendency for many medical and psychological problems to improve over time without any form of treatment. The common cold is a good example. If one were to measure symptom severity in 100 common cold sufferers today, give them a bowl of chicken soup every day, and then measure their symptom severity again in a week, they would probably be much improved. This does not mean that the chicken soup was responsible for the improvement, however, because they would have been much improved without any treatment at all. The same is true of many psychological problems. A group of severely depressed people today is likely to be less depressed on average in 6 months. In reviewing the results of several studies of treatments for depression, researchers Michael Posternak and Ivan Miller found that participants in waitlist control conditions improved an average of 10 to 15% before they received any treatment at all (Posternak & Miller, 2001). Thus one must generally be very cautious about inferring causality from pretest-posttest designs.
Does Psychotherapy Work?
Early studies on the effectiveness of psychotherapy tended to use pretest-posttest designs. In a classic 1952 article, researcher Hans Eysenck summarized the results of 24 such studies showing that about two thirds of patients improved between the pretest and the posttest (Eysenck, 1952). But Eysenck also compared these results with archival data from state hospital and insurance company records showing that similar patients recovered at about the same rate without receiving psychotherapy. This suggested to Eysenck that the improvement that patients showed in the pretest-posttest studies might be no more than spontaneous remission. Note that Eysenck did not conclude that psychotherapy was ineffective. He merely concluded that there was no evidence that it was, and he wrote of “the necessity of properly planned and executed experimental studies into this important field” (p. 323). You can read the entire article here:
http://psychclassics.yorku.ca/Eysenck/psychotherapy.htm
Fortunately, many other researchers took up Eysenck’s challenge, and by 1980 hundreds of experiments had been conducted in which participants were randomly assigned to treatment and control conditions, and the results were summarized in a classic book by Mary Lee Smith, Gene Glass, and Thomas Miller (Smith, Glass, & Miller, 1980). They found that overall psychotherapy was quite effective, with about 80% of treatment participants improving more than the average control participant. Subsequent research has focused more on the conditions under which different types of psychotherapy are more or less effective.

In a classic 1952 article, researcher Hans Eysenck pointed out the shortcomings of the simple pretest-posttest design for evaluating the effectiveness of psychotherapy.
Wikimedia Commons – CC BY-SA 3.0.
Interrupted Time Series Design
A variant of the pretest-posttest design is the interrupted time-series design . A time series is a set of measurements taken at intervals over a period of time. For example, a manufacturing company might measure its workers’ productivity each week for a year. In an interrupted time series-design, a time series like this is “interrupted” by a treatment. In one classic example, the treatment was the reduction of the work shifts in a factory from 10 hours to 8 hours (Cook & Campbell, 1979). Because productivity increased rather quickly after the shortening of the work shifts, and because it remained elevated for many months afterward, the researcher concluded that the shortening of the shifts caused the increase in productivity. Notice that the interrupted time-series design is like a pretest-posttest design in that it includes measurements of the dependent variable both before and after the treatment. It is unlike the pretest-posttest design, however, in that it includes multiple pretest and posttest measurements.
Figure 7.5 “A Hypothetical Interrupted Time-Series Design” shows data from a hypothetical interrupted time-series study. The dependent variable is the number of student absences per week in a research methods course. The treatment is that the instructor begins publicly taking attendance each day so that students know that the instructor is aware of who is present and who is absent. The top panel of Figure 7.5 “A Hypothetical Interrupted Time-Series Design” shows how the data might look if this treatment worked. There is a consistently high number of absences before the treatment, and there is an immediate and sustained drop in absences after the treatment. The bottom panel of Figure 7.5 “A Hypothetical Interrupted Time-Series Design” shows how the data might look if this treatment did not work. On average, the number of absences after the treatment is about the same as the number before. This figure also illustrates an advantage of the interrupted time-series design over a simpler pretest-posttest design. If there had been only one measurement of absences before the treatment at Week 7 and one afterward at Week 8, then it would have looked as though the treatment were responsible for the reduction. The multiple measurements both before and after the treatment suggest that the reduction between Weeks 7 and 8 is nothing more than normal week-to-week variation.
Figure 7.5 A Hypothetical Interrupted Time-Series Design

The top panel shows data that suggest that the treatment caused a reduction in absences. The bottom panel shows data that suggest that it did not.
Combination Designs
A type of quasi-experimental design that is generally better than either the nonequivalent groups design or the pretest-posttest design is one that combines elements of both. There is a treatment group that is given a pretest, receives a treatment, and then is given a posttest. But at the same time there is a control group that is given a pretest, does not receive the treatment, and then is given a posttest. The question, then, is not simply whether participants who receive the treatment improve but whether they improve more than participants who do not receive the treatment.
Imagine, for example, that students in one school are given a pretest on their attitudes toward drugs, then are exposed to an antidrug program, and finally are given a posttest. Students in a similar school are given the pretest, not exposed to an antidrug program, and finally are given a posttest. Again, if students in the treatment condition become more negative toward drugs, this could be an effect of the treatment, but it could also be a matter of history or maturation. If it really is an effect of the treatment, then students in the treatment condition should become more negative than students in the control condition. But if it is a matter of history (e.g., news of a celebrity drug overdose) or maturation (e.g., improved reasoning), then students in the two conditions would be likely to show similar amounts of change. This type of design does not completely eliminate the possibility of confounding variables, however. Something could occur at one of the schools but not the other (e.g., a student drug overdose), so students at the first school would be affected by it while students at the other school would not.
Finally, if participants in this kind of design are randomly assigned to conditions, it becomes a true experiment rather than a quasi experiment. In fact, it is the kind of experiment that Eysenck called for—and that has now been conducted many times—to demonstrate the effectiveness of psychotherapy.
Key Takeaways
- Quasi-experimental research involves the manipulation of an independent variable without the random assignment of participants to conditions or orders of conditions. Among the important types are nonequivalent groups designs, pretest-posttest, and interrupted time-series designs.
- Quasi-experimental research eliminates the directionality problem because it involves the manipulation of the independent variable. It does not eliminate the problem of confounding variables, however, because it does not involve random assignment to conditions. For these reasons, quasi-experimental research is generally higher in internal validity than correlational studies but lower than true experiments.
- Practice: Imagine that two college professors decide to test the effect of giving daily quizzes on student performance in a statistics course. They decide that Professor A will give quizzes but Professor B will not. They will then compare the performance of students in their two sections on a common final exam. List five other variables that might differ between the two sections that could affect the results.
Discussion: Imagine that a group of obese children is recruited for a study in which their weight is measured, then they participate for 3 months in a program that encourages them to be more active, and finally their weight is measured again. Explain how each of the following might affect the results:
- regression to the mean
- spontaneous remission
Cook, T. D., & Campbell, D. T. (1979). Quasi-experimentation: Design & analysis issues in field settings . Boston, MA: Houghton Mifflin.
Eysenck, H. J. (1952). The effects of psychotherapy: An evaluation. Journal of Consulting Psychology, 16 , 319–324.
Posternak, M. A., & Miller, I. (2001). Untreated short-term course of major depression: A meta-analysis of studies using outcomes from studies using wait-list control groups. Journal of Affective Disorders, 66 , 139–146.
Smith, M. L., Glass, G. V., & Miller, T. I. (1980). The benefits of psychotherapy . Baltimore, MD: Johns Hopkins University Press.
Research Methods in Psychology Copyright © 2016 by University of Minnesota is licensed under a Creative Commons Attribution-NonCommercial-ShareAlike 4.0 International License , except where otherwise noted.

- Liberty University
- Jerry Falwell Library
- Special Collections
- < Previous
Home > ETD > Doctoral > 5868
Doctoral Dissertations and Projects
A quasi-experimental study on the effects of small group learning on mathematical resilience in upper elementary students.
Joshua Adam Costelnock , Liberty University Follow
School of Education
Doctor of Philosophy in Education (PhD)
Janice Kooken
whole group instruction, small group learning, mathematical resilience, progressive classroom
Disciplines
Recommended citation.
Costelnock, Joshua Adam, "A Quasi-Experimental Study on the Effects of Small Group Learning on Mathematical Resilience in Upper Elementary Students" (2024). Doctoral Dissertations and Projects . 5868. https://digitalcommons.liberty.edu/doctoral/5868
The purpose of this quantitative, quasi-experimental study was to determine the effect of small group learning during the core mathematics block on 5th-grade students’ mathematical resilience, compared to a control group. Student collaboration and mathematical discourse decreased during the COVID-19 pandemic, leading to a drop in math proficiency levels in the United States. Approximately 80 5th-grade students from the southwest United States were divided into two sample groups of about 40 each. These groups were assessed using the Upper Elementary Mathematics Resilience Scale. One group primarily experienced teacher-centered whole group instruction, while the other group spent half of their daily core learning block in student-centered small group instruction. Differences between the two groups were analyzed using ANCOVA on the two measures of the Mathematical Resilience Scale: value and growth mindset. The ANCOVA tested for differences in the post-test, using the pre-test as the covariate. Data for the value subscale showed a statistically significant change between the groups, though the direction of the change was unexpected. Data for the growth subscale did not reach appropriate levels of significance. For future research, it is recommended that the scale be administered at the beginning of the school year instead of the end, and that the sample size be increased in both groups.
Since August 09, 2024
Included in
Education Commons
- Collections
- Faculty Expert Gallery
- Theses and Dissertations
- Conferences and Events
- Open Educational Resources (OER)
- Explore Disciplines
Advanced Search
- Notify me via email or RSS .
Faculty Authors
- Submit Research
- Expert Gallery Login
Student Authors
- Undergraduate Submissions
- Graduate Submissions
- Honors Submissions
Home | About | FAQ | My Account | Accessibility Statement
Privacy Copyright
- Systematic Review
- Open access
- Published: 16 August 2024
Emergency infection prevention and control training in fragile, conflict-affected or vulnerable settings: a scoping review
- Julii Brainard 1 ,
- Isabel Catalina Swindells 2 ,
- Joanna Wild 3 ,
- Charlotte Christiane Hammer 4 ,
- Emilio Hornsey 5 ,
- Hibak Osman Mahamed 6 &
- Victoria Willet 6
BMC Health Services Research volume 24 , Article number: 937 ( 2024 ) Cite this article
31 Accesses
Metrics details
It is uncertain what could be the best training methods for infection prevention and control when an infectious disease threat is active or imminent in especially vulnerable or resource-scarce settings.
A scoping review was undertaken to find and summarise relevant information about training modalities, replicability and effectiveness of IPC training programmes for clinical staff as reported in multiple study designs. Eligible settings were conflict-affected or in countries classified as low-income or lower-middle income (World Bank 2022 classifications). Search terms for LILACS and Scopus were developed with input of an expert working group. Initially found articles were dual-screened independently, data were extracted especially about infection threat, training outcomes, needs assessment and teaching modalities. Backwards and forwards citation searches were done to find additional studies. Narrative summary describes outcomes and aspects of the training programmes. A customised quality assessment tool was developed to describe whether each study could be informative for developing specific future training programmes in relevant vulnerable settings, based on six questions about replicability and eight questions about other biases.
Included studies numbered 29, almost all ( n = 27) were pre-post design, two were trials. Information within the included studies to enable replicability was low (average score 3.7/6). Nearly all studies reported significant improvement in outcomes suggesting that the predominant study design (pre-post) is inadequate to assess improvement with low bias, that any and all such training is beneficial, or that publication bias prevented reporting of less successful interventions and thus a informative overview.
It seems likely that many possible training formats and methods can lead to improved worker knowledge, skills and / or practice in infection prevention and control. Definitive evidence in favour of any specific training format or method is hard to demonstrate due to incomplete descriptions, lack of documentation about unsuccessful training, and few least-biased study designs (experimental trials). Our results suggest that there is a significant opportunity to design experiments that could give insights in favour of or against specific training methods. “Sleeping” protocols for randomised controlled trials could be developed and then applied quickly when relevant future events arise, with evaluation for outcomes such as knowledge, practices, skills, confidence, and awareness.
Peer Review reports
A survey of health and care workers in low or lower middle countries in 2017–18 suggested that infection prevention and control (IPC) training while in post was unusual in many countries (reported in 54% of respondent countries [ 1 ]). Moreover, such training may only happen when there is already a defined infectious threat present or likely to arrive imminently. A highly responsive strategy in developing and delivering IPC training means opportunity to customise training formats and methods for local workforce contexts and curricula with regard to very specific pathogens and transmission pathways. However, the context of needing to deliver training urgently with little advance notice of specific pathogen or local context means that such training may be designed and delivered hurriedly, and with minimal setting-specific needs assessment and little evaluation for effectiveness.
As part of past pandemic recovery and future pandemic preparedness, it is useful to collate evidence about which IPC training methods have been applied in specific settings or contexts. Evidence would be especially useful that could be used to inform ongoing development of best training delivery guidelines in settings that may be described as fragile, conflict-affected or otherwise vulnerable (FCV). Best quality evidence may be defined with regard to completeness of reporting (if the training methods are replicable) as well as evidence of effectiveness (desired outcomes). We searched on Google Scholar and Prospero in August 2023 for completed or registered systematic or scoping reviews addressing the topic of emergency IPC training in vulnerable settings. The most similar and comprehensive existing systematic review (Nayahangan et al. 2021; [ 2 ]) described medical and/or nursing training (delivered for any clinical training purpose, not just IPC) delivered during viral epidemics (only). The search date for the Nayahangan et al. review was April 2020, more than 3 years before our own study commenced. Systematic literature reviews may be considered ‘out of date’ by two years after their most recent search date [ 3 ]. Nayahangan et al. included clinical settings in any country and was not confined to training delivered in emergency or urgent contexts (readiness or response phases [ 4 , 5 ]). Nayahangan et. al . performed quality assessment using the Educational Interventions Checklist [ 6 ] which focuses on replicability and mapping of reported teaching methods in the primary research, but only indirectly addresses effectiveness. Nayahangan et. al . concluded that previous studies had used a variety of training methods and settings but few training methods had been related to specific patient or other epidemic outcomes. Another somewhat similar previous systematic review was Barrera-Cancedda et al. [ 7 ] which described and assessed IPC training strategies in sub-Saharan Africa for nurses. Most of the strategies they found and described were during “business as usual” conditions, rather than readiness or response phases of an outbreak or epidemic presenting imminent threat. Their quality assessment tools were for assessing bias in effectiveness rather than replicability. Their focus was narrowly on nurses in a specific geographic region. Their conclusions arose from considering evidence that went far beyond staff training methods. Barrera-Cancedda et al. concluded that creating good future guidelines for evidence-based practice required that additional primary research to be undertaken from an implementation science-specific perspective.
A challenge in emergency IPC training manifest during the Covid-19 pandemic is inherent to other emerging diseases: early in an outbreak situation there is often uncertainty about the best IPC practices. The actual best practices may vary according to predominant disease transmission pathway(s) that are not yet well-understood. There is merit in considering evidence according to what disease(s) are being prepared for.
This study aimed to provide an updated evidence summary about IPC training formats and apparent effectiveness in a scoping review design. We collected and summarised evidence about IPC training formats and methods as delivered in FCV settings when there was an active infectious disease present (response phase) or the infection arrival was fairly imminent (expected within 6 months, readiness phase) [ 4 , 5 ]. We undertook a scoping review of IPC training programmes reported in peer reviewed scientific literature to summarise which training formats or methods had been described in FCV settings, and to describe how often such training was associated with success in these settings. Key effectiveness outcomes were: knowledge, skills, compliance, case counts or case mortality while training delivery was summarised according to key features such as format, duration and delivery mode.
PROSPERO registration number is CRD42023472400. We originally planned to undertake a systematic review but later realised that answering our research question was better suited to a scoping review format, where evidence is summarised narratively with respect to creating a comprehensive overview of evidence rather than obtaining evidence to be evaluated for effectiveness. There were two other notable deviations from protocol: we did not use the Covidence platform and we decided to develop and apply a customised quality assessment (QA) checklist instead of originally listed QA instruments. This article is one of several outputs arising from the same protocol.
Training programmes had to take place in FCV settings or for staff about to be deployed to FCV settings. Fragile or vulnerable settings were defined as being in countries that were designated as low income or lower-middle income by the (World Bank 2022 classification; [ 8 ]). Conflicted-affected settings were determined using reader judgement for individual studies, and had to feature concurrent with the training and care delivery, high threat of armed violence or civil unrest. Participants had to be health care professionals (HCPs), social care staff, student or trainee HCPs or trainee social care staff working in an FCV setting. If in doubt about whether the participants qualified, we deferred to World Health Organisation occupational definitions [ 9 ]. Voluntary carers such as family members or community hygiene champions as targets were excluded. Eligible interventions could be described as training or education related to any aspect of IPC outcomes.
Intervention
The training programme could be any training or education that was delivered in a response phase (when there was a concurrently present infectious disease threat) or in the readiness phase [ 5 ], when there was high risk that the infectious threat would become present in the clinical environment within six months, such as soon after Covid-19 was declared to be a public health threat of international concern in January 2020.
Comparators were either the same cohort measured at baseline or a contemporaneous cohort in same setting who did not receive IPC training.
Effectiveness outcomes
Changes in individual knowledge, skills, adherence (compliance or practice), case counts or mortality related to infection were primary effectiveness outcomes. These were chosen because preliminary searches suggested they were commonly reported outcomes in the likely literature. Most of these were immediate benefits that could result as soon as training was completed. We also included case incidence and infection-related mortality as primary outcomes because we knew from preliminary literature searches that these were often the only specific outcomes reported after IPC training. Secondary outcomes (data only collected from articles with at least one primary outcome) were attitudes, acceptability of the training, self-efficacy, confidence, trust in IPC, awareness, index-of-suspicion, ratings for value or relevance of the training, objectives of the training, lessons learned about training needs or recommendations about training needs to be addressed in similar subsequent training programmes.
Outcomes could be objectively- or self-assessed. We wanted to extract outcomes that could be most comparable between studies (not adjusted for heterogenous covariates) and that were objectively assessed rather than self-reported, if possible. Hence, objectively assessed outcomes were extracted and are reported if both objectively- and self-assessed outcomes were available, else self-reported outcomes were extracted and are reported. We extracted and report unadjusted outcomes where available, but adjusted results after post-processing (such as using regression models) were extracted if no unadjusted results were reported.
Inventory and description of training methods
Specific aspects of how training was delivered were key to understanding the potential that each training programme might have to achieve replicable results elsewhere. We used an iterative process with an expert working group giving advice to develop a list of training features such as setting, duration, target participants and programme design (see list below). These categorisations are not presented as definitive but rather they were pragmatically determined attributes for what information could be gathered in the eligible studies and that directly inform how replicable each education programme was, and how generalisable its results might be in other settings/with other target participants. We extracted information from the studies to categorise the training that they described according to the below features. Multiple answers were possible for many of these features. “Unclear” or “Mixture” were possible answers, too.
Where (location) : Off-site without real patients; in house but not while caring for patients; on the job training (during patient care).
Length of the training session(s): such as 1 h on one day, or 6 sessions over 8 weeks, etc.
When (timing with respect to possible threat) : Pre-deployment to clinical environment; in post or as continuing professional development.
Mode (of delivery) : 3 options which were: face to face; blended (a mix of face to face and online) or hybrid (face to face with opportunity for some participants to join in remotely); only digital: e.g. digital resources uploaded to an USB stick or online via an online platform, either synchronous or asynchronous.
Broad occupational category receiving the training : Clinical frontline staff; trainers who were expected to directly train others; programme overseers or senior managers.
Specific occupations receiving the training : Nurses, doctors/physicians, others.
Learning group size : Individual or group.
Format : Workshops; courses; seminars/webinars; mentoring/shadowing; e-learning; e-resources, other.
Methods : Didactic instruction/lectures/audio-visual presentations; demonstrations/modelling; discussion/debate; case studies or scenarios; role play or clinical practice simulations; assessment or exams with formative assessment; hands-on practice / experience; games; field trips or site visits; virtual reality or immersive learning; repeated training; shadowing; other.
Additional inclusion and exclusion criteria
We included scientific studies with concurrent comparison groups (CCT or RCT) where post-training outcomes were reported for both arms and pre-post studies where both baseline and post-training measurements of a primary effectiveness outcome were reported. Clinical cases, case reports, cross-sectional studies, letters to the editor, editorials, commentaries, perspectives, technical notes, and review summaries were excluded unless they reported baseline and post-training eligible effectiveness outcomes. Studies must have been published in 2000 or later. Infectious biological entities could be bacteria, viruses, protozoa or funghi, but not complex multicellular organisms (like mites or lice).
Studies could be published in any language that members of the team could read or translate to coherent English using Google Translate. Training in infection prevention and control had to be applicable to a clinical or social care environment for humans. Non-residential care settings (such as daily childcare facilities) were excluded. Studies about controlling infection risks from or to animals or risk reduction in non-clinical environments (such as removing mosquito breeding sites) were excluded.
We wanted to focus on IPC training that related to individual action and could result in immediate benefits and in clinical not community environments. For this reason, we excluded interventions or outcomes that related to: forms of patient care (e.g., anti-viral treatment) that might hasten end of infectious period; vaccination programmes; surveillance; availability of personal protective equipment (PPE) or other resources that reflect institutional will and opportunity as much as any individual action; testing strategies or protocols or actions to speed up test results or screening patients for infection. Also excluded were training programmes in environmental management outside of the clinical/care environment with exception for waste management generated within clinic and managed on site which might include some outdoor/away from clinic/care location handling and disposal decisions.
Eligible studies had to report at least one of our primary outcomes so that we could summarise the evidence base about which training methods linked to evidence of effectiveness. To focus on the response and readiness phase of emergencies, we excluded studies where the primary outcome was only measured > 12 months after training started (i.e., quality improvement reports).
MEDLINE, Scopus, LILACS were searched on 9 October 2023 with the search phrase (Scopus syntax):
(“infection-control”[Title/Abstract] or “transmission”[Title/Abstract] or.
“prevent-infectio*”[Title/Abstract]).
(“emergency”[Title/Abstract] or “epidemic”[Title/Abstract] or “outbreak”[Title/Abstract]).
(“training”[Title/Abstract] or “educat*”[Title/Abstract] or “teach*”[Title/Abstract]).
Included studies in a recent and highly relevant systematic review [ 2 ] were also screened. Initially included studies from those search strategy steps were then subjected to forward and backward citation searches to look for additional primary studies.
After deduplication, two authors independently screened all studies found by the search strategy, recording decisions on MS Excel spreadsheets. All studies selected by at least one author had full text review for final decision about inclusion.
Quality assessment
We assess quality indicatively and with regard to usefulness of the studies to inform development of future IPC training programmes in relevant settings. The focus was on two broad domains that informed A) how replicable the training programme was, as described; B) how biased its results were likely to be. Our protocol planned to apply the Cochrane Risk of Bias 1.0 for trials (ROB.1) and Newcastle Ottawa Scale (NOS) tools to undertake quality assessment for pre-post study designs. However, we realised that neither of these tools captured whether the original research had reported sufficient details to make the original training programme replicable. Another problem is that the judgements arising from the RoB.1 and NOS would not be strictly comparable, given the different assessment criteria. Other existing quality checklists that we are aware of that were suitable for each of trials, cohorts or pre-post study designs had the shortcomings of only capturing replicability or bias in apparent effectiveness (not both), and tending to be suitable for only one study design. Some checklists (eg The Cochrane Risk of Bias 2.0 tool [ 10 ] or Mixed Methods Appraisal Tool [ 11 ]) require more resources to operationalise than we had or that was required for a scoping review. Instead, we devised and applied an indicative quality checklist that comprised 14 simple questions with possible answers that were “yes, no or uncertain” using specific predefined criteria for deciding each answer. Our checklist is available as File S 1 . These questions were modified from suggested questions in the USA National Institutes of Health assessment checklist for pre-post designs [ 12 ]. Applying a single quality assessment tool across multiple study designs had the further advantage of facilitating comparability with regard to identifying relative informativeness for future effectiveness evaluation and training programme design. The answers were scored as 1 point per yes answer, so maximum score (for least biased and most replicable studies) would be 14. We interpret the overall quality assessment results as follows: ≥ 11/14 = most informative, 8–10 = somewhat informative, ≤ 7/14 least informative. The quality assessment results are reported quantitatively and narratively. Subdomains for replicability and other bias (generalisability) scores are reported separately.
Data extraction and interpretation (selection and coding)
These data were extracted: author of the study, year of publication, study country, study design, sample size in comparator arms, relevant infectious diseases (that author identified), primary outcomes, secondary outcomes. With regard to training delivered, we also extracted information about any needs assessment that was undertaken, training objectives and any statements about lessons learned or what should be addressed in future design of such programmes or in research. One author extracted data which was confirmed by a second author. Results are reported quantitatively (counts of studies with any particular training aspect) and narratively for needs assessment, objectives and lessons learned.
To interpret likely usefulness, we prioritise higher scores (for informativeness), but also consider study design, with trials presumed to have less biased results with regard to effectiveness outcomes. We address potential differences that were monitored or observed between knowledge, skills or practices with respect to the training attributes. For instance, were outcomes assessed immediately after training (within 1 day) as opposed to (ideally) observed and assessed independently at least three weeks later, which would suggest knowledge, skills and/or practice retention. We also highlight when training applicable to conflict-affected settings was delivered in that same conflicted-affected setting or prior to entry to the setting (such as for military personnel deployed overseas).
Figure 1 shows the study selection process. 29 studies were included. Extracted data for each study are in File S 2 . Almost all ( n = 27) were pre-post design; 2 were experimental studies [ 13 , 14 ]. Table 1 lists summary information about the included studies. Seven reports described training delivered in single low-income countries, 19 studies described training in single lower middle income countries. Two articles described IPC training for staff in context of conflict-affected settings, either in the USA prior to military deployment [ 15 ] or in the affected setting during a period of civil unrest (in Haiti in 2010; [ 16 ]). Two studies [ 17 , 18 ] described training using a common core curriculum in multiple African countries (mix of low and lower middle income). The most represented countries were India (4 studies) and Nigeria (6 studies). Nine studies were about Ebola disease, 14 related to controlling Covid-19. Other studies addressed cholera ( n = 2), antimicrobial resistant organisms ( n = 3) and tuberculosis ( n = 1). Clinical environments were most commonly described as hospitals ( n = 9) while twelve studies described programmes for staff working in multiple types of health care facilities. 21 studies were undertaken in response phase, two in readiness phase and six in mixed readiness/response phases. Nurses were the most commonly specified type of health care worker (mentioned in 24 studies). In Table 1 , higher scores for knowledge, attitudes, practices or skills were the better clinical outcomes unless otherwise stated. Some additional outcome information for LN Patel, S Kozikott, R Ilboudo, M Kamateeka, M Lamorde, M Subah, F Tsiouris, A Vorndran, CT Lee and C of Practice [ 18 ] and N Zafar, Z Jamal and M Mujeeb Khan [ 19 ] are in the original studies but could not be concisely repeated in Table 1 . Most articles reported statistically significant (at p < 0.05) improvements in outcomes after training. A notable exception is OO Odusanya, A Adeniran, OQ Bakare, BA Odugbemi, OA Enikuomehin, OO Jeje and AC Emechebe [ 20 ] who attributed a lack of improvement after training to very good baseline knowledge, attitudes and practices.
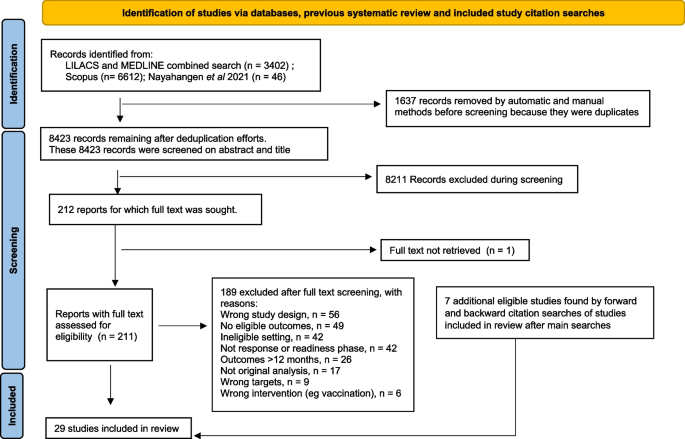
Selection procedure for eligible studies
Outcomes were assessed immediately after training ended in 14 studies; assessment point was unclear in two studies. Other outcome assessments ( n = 13 studies) took place between 1 week and 6 months after training finished (especially with respect to case counts or mortality). Because almost all studies reported outcome benefits, studies with delayed assessment cannot be said to have achieved greater benefits.
Needs assessment was described in most studies ( n = 27). For instance, C Carlos, R Capistrano, CF Tobora, MR delos Reyes, S Lupisan, A Corpuz, C Aumentado, LL Suy, J Hall and J Donald [ 32 ] stated that “Although briefings for health care workers (HCWs) in Ebola treatment centres have been published, we were unable to locate a course designed to prepare clinicians for imported Ebola virus disease in developing country settings.” HM Soeters, L Koivogui, L de Beer, CY Johnson, D Diaby, A Ouedraogo, F Touré, FO Bangoura, MA Chang and N Chea [ 38 ] cited widespread evidence that there was a high transmission rate to health care workers within Ebola Treatment centres to justify the need for IPC training in these settings. S Ahmed, PK Bardhan, A Iqbal, RN Mazumder, AI Khan, MS Islam, AK Siddique and A Cravioto [ 41 ], A Das, R Garg, ES Kumar, D Singh, B Ojha, HL Kharchandy, BK Pathak, P Srikrishnan, R Singh and I Joshua [ 21 ] and MO Oji, M Haile, A Baller, N Trembley, N Mahmoud, A Gasasira, V Ladele, C Cooper, FN Kateh and T Nyenswah [ 35 ] describe that expert observers identified deficiencies in existing IPC practices and developed training based on those observations. Independent observations of training needs were formalised as a cross-sectional survey of dental student IPC knowledge in A Etebarian, S Khoramian Tusi, Z Momeni and K Hejazi [ 22 ], and by applying a validated IPC checklist in L Kabego, M Kourouma, K Ousman, A Baller, J-P Milambo, J Kombe, B Houndjo, FE Boni, C Musafiri and S Molembo [ 34 ].
All studies stated specific training objectives and gave at least some information about the specific topics and curriculum. Objectives statements mentioned improvement ( n = 10 studies), knowledge ( n = 7), safety ( n = 6), attitudes ( n = 3), increasing capacity or skills ( n = 6), and development ( n = 1). Examples of other objectives statements were to “teach the basics” [ 41 ] or “to cover the practical essentials” [ 16 ]. Training content and delivery were often highly adapted for local delivery [ 23 , 24 , 25 , 28 , 29 , 32 , 33 , 36 , 38 , 41 ]. Training materials were entirely or mostly derived from published guidance in some studies [ 16 , 19 , 34 , 35 , 37 ]. F Tsiouris, K Hartsough, M Poimboeuf, C Raether, M Farahani, T Ferreira, C Kamanzi, J Maria, M Nshimirimana and J Mwanza [ 17 ] and LN Patel, S Kozikott, R Ilboudo, M Kamateeka, M Lamorde, M Subah, F Tsiouris, A Vorndran, CT Lee and C of Practice [ 18 ] both report that training delivery methods were highly adapted and variable, but developed using the same core course content about Covid-19 in 11 or 22 African countries. Other studies were unclear about how much of their programme was original and how much relied on previously published guidance and recommendations [ 13 , 15 , 20 , 21 , 24 , 26 , 27 , 30 , 31 , 39 , 40 ].
Counts of training locations were: ten off-site; seven on-site but not during patient care; nine were a mix of learning locations; three had unclear locations relative to clinical facility location. Among the 21 studies that described the specific cumulative duration of training sessions, median training duration was 24 h (typically delivered over 3 consecutive days), ranging from about 15 min to 8 full days. Most studies ( n = 21) described training where it was clear that many or most participants were in post, 3 studies clearly described training being provided prior to deployment, another 5 training programmes had mixed or unclear timing with regard to deployment. Twelve studies described training that was delivered only in person, 9 studies described purely digital delivery, 7 were blended delivery and 1 programme was unclear whether the training was delivered digitally or in person. In terms of IPC roles, all studies included at least some frontline workers. In addition, six studies were explicitly designed to train people who would educate others about IPC, seven studies reported including facility managers or supervisors among the trainees. 23 studies mentioned nurses specifically among the trainees, 17 studies specifically mentioned doctors or physicians. Other professionals mentioned were cleaners, porters, paramedics, midwives, anaesthesiologists, hygienists, housekeeping staff, lab technicians, medical technologists and pharmacists. Almost half ( n = 14) of studies were group education; purely individual learning was specified in just one study and others ( n = 14) were unclear or could be either individual or group learning.
Often training formats or teaching methods were described unclearly. With regard to formats that were described clearly, counts were workshop ( n = 10), course (22), seminar or webinar (1), mentoring or shadowing (4), e-learning (13) and inclusion of e-resources (14). Counts of studies using specific teaching methods that were described clearly were didactic (23), demonstrations (17), discussion or debate (8), case-studies or scenarios (6), role play or simulations (9), formative assessment (3), hands-on practice (12), site visits (2), repeat or refresher training (5), shadowing (3). Additional teaching methods described specifically were poster reminders, monitoring (active and passive as well as observation), re-enforcement (updating procedure documents, re-assessing, more training), brainstorming, small group work and other visual aids. Many articles described multiple formats or teaching methods that were used as part of the same training programme, hence these categorisations sum up to more than the total count of included studies.
Most studies ( n = 25) provided some commentary that could be interpreted as “lessons learned” about training methods and delivery. That success of such programmes depends as much on improving mindset or attitude about IPC as teaching other skills or habits was mentioned by at least 6 studies [ 13 , 14 , 20 , 22 , 32 , 39 ]. The merits of capacity building were explicitly reiterated in concluding commentary in seven studies [ 21 , 26 , 29 , 30 , 31 , 35 ]. Other aspects repeatedly endorsed (at least three times) in concluding comments in the included studies were the value of IPC champions or leaders [ 21 , 34 , 35 ] the value of training relevant to specific job role [ 14 , 18 , 22 , 31 ]; advantages of digital not in-person learning [ 13 , 14 , 19 , 20 , 23 ]; value of refresher sessions [ 13 , 14 , 17 , 21 , 30 , 35 ] and merits of evaluation beyond the immediate end of the training programme to make sure that benefits were sustained [ 21 , 29 , 38 , 39 ]. Regarding lessons learned, Thomas et al. 2022 [ 29 ] and Otu et al. 2021 [ 24 ] (both Nigerian studies) gave specific details about challenges and benefits of mobile phone digital training delivery, for instance reliance on assumed e-literacy, uncertainty about consistent access to Internet or access to devices with suitable versions of the Android operating system. Four studies [ 14 , 25 , 29 , 38 ] listed benefits when training was delivered in participant’s native language(s).
Quality assessment scores are shown in Table 2 . Recall that the customised quality assessment evaluation addressed two broad domains: replicability and other biases (other potential for generalisability), with results interpreted as usefulness of the study to inform future design of similar IPC training programmes. The quality assessment found that replicability potential was not high overall, with an average score of 3.7/6. There was insufficient easily available information (score was < 4 of 6 replicability domains in QA checklist) to undertake the same intervention again for 11 studies, while replicability was relatively high (≥ 5/6) for 9 studies. The generalisability domain in the quality assessment checklist addressed other factors that may have biased the apparent effectiveness outcomes of each training programme . 22 studies scored < 5/8 for generalisability (suggesting they were likely to be at high risk of bias with regard to outcomes reported). Only one study was assessed to be of overall relatively higher quality (quality checklist score ≥ 11/14) and can be considered especially (“most”) useful for informing design of such IPC training in future. Shreshtha et al. [ 28 ] had a pre-post design and is especially thorough in describing training in intubation and triage protocols in Nepal to prevent Covid-19 transmission. The two controlled trials included in our review [ 13 , 14 ] both scored below 11 (10/14) in the quality assessment because they had unclear information about how many participants were assessed and did not provide specific training or assessment materials. There was minimal or no difference in most outcome improvements between arms in one of the trials (Jafree et al. 2022; [ 14 ]), but statistically significant greater improvement in outcomes, especially knowledge, in the active intervention arm, in the other trial. (Sharma et al. 2021; [ 13 ]). This number of experimental trials was small ( n = 2) and they described fairly different format training programmes for different diseases.
The evidence available is difficult to interpret because of incomplete reporting and lack of specific descriptions. Training delivery was often vaguely described, or even explicitly described as highly diverse while relatively few pathogens were addressed. Only two moderate size ( n = about 200 in each) experimental trials were found which is insufficient for making broad conclusions about effectiveness. It seems likely that many possible training methods can successfully improve HCW knowledge, skills, attitude, practices, etc. We note that there is unlikely to be definitive evidence in favour of or against specific training methods due to lack of thorough description of training methods in addition to lack of robust study designs (very few clinical trials). Lack of specificity about which aspects of training were least or most beneficial may hinder successful development of future training programmes. Lack of controlled trials and generally poor description of any training programmes that existed prior to implementation of the programmes described in pre-post studies means that we can’t discern if training was effective because of how it was delivered or because relevant training had never been given previously. It seems clear that there is huge opportunity for design of well-run controlled trials in IPC training delivery. A controlled trial could be designed and tested with a pre-specified curriculum for a common and recurring type of pathogen (e.g., influenza-like illness or for a specific common anti-microbial resistant organism), but with 2 or more delivery formats pre-approved with institutional review bodies, and thus ready to be implemented when a relevant crisis arose. Suitable outcomes to include in the trial design would measure aspects of knowledge, practices, skills, confidence and awareness. Complexity-informed evaluation strategies [ 42 ] are likely to be desirable in fragile, conflict-affected or vulnerable settings, too. (Nayahangan et al. 2021; [ 2 ]) recommended that medical training be more standardised during viral epidemics. We did not find evidence to show that universally formatted IPC training programmes are optimal in FCV settings. We have, however, provided information that can be used to begin to assess effectiveness of training programmes that are either universally formatted or more highly locally adapted.
Only two of our studies described training that was applied in conflict-affected settings; one of these [ 15 ] described training that was also delivered prior to worker arrival in the conflict-affected setting. We judge that these two studies are too few and too heterogenous to pool, so we cannot draw broad conclusions about training delivery and benefits in a conflict-affected area context or in a high resource setting prior to deployment.
Other researchers have systematically described many key issues that affect effectiveness of IPC training in low resource or conflict-affected settings. For instance, Qureshi et al. 2022 [ 43 ] undertook a scoping review of national guidelines for occupational IPC training. They audited how up to date such guidelines were. They identified key deficiencies, especially in LMIC countries with regard to the most recent best recommended practices in evaluation and adult learning principles. A global situational analysis undertaken in 2017–2018 [ 1 ] concluded that although nearly all countries audited had relevant national training guidelines in IPC, there was far less training of HCWs taking place, less surveillance and lower staffing levels in lower-middle and lower-income countries (World Bank classifications) than in upper-middle and high income countries.
Data and analyses have been undertaken to specifically describe challenges and potential strategies to meet those challenges, when undertaking IPC in conflict affected settings [ 44 ] or low and middle income countries dealing with a specific disease [e.g., tuberculosis; 45 ]. These studies are fundamentally qualitative in design and narrative, so while they provide insight, they do not lead to confident conclusions about which if any training methods are most likely to be successful. There is a dearth of experimental evidence in lower-middle and lower income countries. The Covid-19 pandemic especially focused interest on IPC guidelines for respiratory infection prevention. A review by Silva et al. 2021 [ 46 ] of randomised controlled trials that tried to improve adherence to IPC guidelines on preventing respiratory infections in healthcare workplaces included 14 interventions, only one of which was not in a high income setting [in Iran; 47 ], and all were in arguably undertaken in preparation phase (not response or readiness).
Limitations
Although we included incidence and mortality as primary outcomes, these outcomes are often not immediate benefits from good IPC training and thus are problematic indicators of IPC success. Case incidence is highly dependent on local community prevalence of relevant pathogen(s), while mortality rates often reflect quality of medical care available in addition to population awareness and subsequent timing of presentation. Our search strategy was not tested using eligible exemplar studies, nor did it include controlled vocabulary which might have found additional eligible studies. We did not rigorously determine risk of bias in each of the few trials available. We did not explicitly look for evidence of publication bias [ 48 ] in this evidence group, but we suspect that the near total absence of any information about failed interventions biases what we can say with confidence about truly successful training formats and methods.
A key limitation when we graded the studies for likely usefulness is that we did not attempt to contact primary study authors to obtain more information or specific training materials. Additional materials are likely to be available from most of the primary study authors and would boost their study replicability and apparent biases. However, such contact could also be a very demanding and not necessarily productive exercise. A broader review than ours could have collected all evidence about any training modalities when delivered in eligible contexts (readiness or response phase in FCV settings), regardless of whether effectiveness outcomes were reported. A review with similar such objectives was published in 2019 [ 7 ], which inventoried implementation strategies for IPC promotion in nurses in Sub-Saharan Africa.
We decline to adopt a broad inventorying approach because the information obtained would still lack evidence of effectiveness. We found some studies [e.g., 49 ] which provided a thorough description of training delivery, but without evaluation of our outcomes and therefore ineligible for inclusion in our review. A broader review than ours would have included grey literature and qualitative studies. Qualitative studies especially provide information about effective communication and leadership, acceptability of training delivery methods, incentives, accountability strategies, satisfaction ratings and barriers to learning [ 50 ]. While those are highly relevant outcomes to effective training in IPC, they were removed from the core outcome that is likely to matter most in achieving good IPC, which is consistency of desired practices.
Our conclusions are limited because of the mediocre quality of evidence available. Although existing evidence in favour of or against any specific training approach is far from definitive, there is much opportunity to design future studies which explicitly and robustly test specific training formats and strategies.
Availability of data and materials
The datasets used and/or analysed during the current study are available from the corresponding author upon reasonable request.
Tartari E, Tomczyk S, Pires D, Zayed B, Rehse AC, Kariyo P, Stempliuk V, Zingg W, Pittet D, Allegranzi B. Implementation of the infection prevention and control core components at the national level: a global situational analysis. J Hosp Infect. 2021;108:94–103.
Article CAS PubMed PubMed Central Google Scholar
Nayahangan LJ, Konge L, Russell L, Andersen S. Training and education of healthcare workers during viral epidemics: a systematic review. BMJ Open. 2021;11(5): e044111.
Article PubMed PubMed Central Google Scholar
Shojania KG, Sampson M, Ansari MT, Ji J, Doucette S, Moher D. How quickly do systematic reviews go out of date? A survival analysis. Ann Intern Med. 2007;147(4):224–33.
Article PubMed Google Scholar
World Health Organization. Framework and toolkit for infection prevention and control in outbreak preparedness, readiness and response at the national level. 2021. p. 84. https://www.who.int/publications/i/item/9789240032729 .
Emergency cycle. 2024. https://www.who.int/europe/emergencies/emergency-cycle . Accessed 9 Jan 2024.
Meinema JG, Buwalda N, van Etten-Jamaludin FS, Visser MR, van Dijk N. Intervention descriptions in medical education: what can be improved? A systematic review and checklist. Acad Med. 2019;94(2):281–90.
Barrera-Cancedda AE, Riman KA, Shinnick JE, Buttenheim AM. Implementation strategies for infection prevention and control promotion for nurses in Sub-Saharan Africa: a systematic review. Implement Sci. 2019;14(1):1–41.
Article Google Scholar
New World Bank country classifications by income level: 2022–2023. 2023. https://blogs.worldbank.org/opendata/new-world-bank-country-classifications-income-level-2022-2023 . Accessed 10 Jan 2024.
Health Workforce-related terminology: terminology work carried out by the WHO Language department at the request of the Health Workforce department. 2021. https://cdn.who.int/media/docs/default-source/health-workforce/hwp/202100608-health-workforce-terminology.pdf . Accessed 9 Jan 2024.
Martimbianco ALC, Sá KMM, Santos GM, Santos EM, Pacheco RL, Riera R. Most Cochrane systematic reviews and protocols did not adhere to the Cochrane’s risk of bias 2.0 tool. Rev Assoc Med Brasi. 2023;69(3):469–72.
Pluye P, Hong QN. Combining the power of stories and the power of numbers: mixed methods research and mixed studies reviews. Annu Rev Public Health. 2014;35(1):29–45.
Study quality assessment tools. 2013. https://www.nhlbi.nih.gov/health-topics/study-quality-assessment-tools . Accessed 9 Jan 2024.
Sharma SK, Mandal A, Mishra M. Effectiveness of m-learning on knowledge and attitude of nurses about the prevention and control of MDR TB: a quasi-randomized study. Indian J Tuberc. 2021;68(1):3–8.
Jafree SR, Zakar R, Rafiq N, Javed A, Durrani RR, Burhan SK, Hasnain Nadir SM, Ali F, Shahid A, Wrona KJ. WhatsApp-delivered intervention for continued learning for nurses in Pakistan during the COVID-19 pandemic: results of a randomized-controlled trial. Front Public Health. 2022;10:739761.
Crouch HK, Murray CK, Hospenthal DR. Development of a deployment infection control course. Mil Med. 2010;175(12):983–9.
Tauxe RV, Lynch M, Lambert Y, Sobel J, Domerçant JW, Khan A. Rapid development and use of a nationwide training program for cholera management, Haiti, 2010. Emerg Infect Dis. 2011;17(11):2094.
Tsiouris F, Hartsough K, Poimboeuf M, Raether C, Farahani M, Ferreira T, Kamanzi C, Maria J, Nshimirimana M, Mwanza J. Rapid scale-up of COVID-19 training for frontline health workers in 11 African countries. Hum Resour Health. 2022;20(1):43.
Patel LN, Kozikott S, Ilboudo R, Kamateeka M, Lamorde M, Subah M, Tsiouris F, Vorndran A, Lee CT, of Practice C. Safer primary healthcare facilities are needed to protect healthcare workers and maintain essential services: lessons learned from a multicountry COVID-19 emergency response initiative. BMJ Global Health. 2021;6(6):e005833.
Zafar N, Jamal Z, Mujeeb KM. Preparedness of the healthcare personnel against the coronavirus disease 2019 (COVID-19) outbreak: an audit cycle. Front Public Health. 2020;8:502.
Odusanya OO, Adeniran A, Bakare OQ, Odugbemi BA, Enikuomehin OA, Jeje OO, Emechebe AC. Building capacity of primary health care workers and clients on COVID-19: results from a web-based training. PLoS One. 2022;17(10):e0274750.
Das A, Garg R, Kumar ES, Singh D, Ojha B, Kharchandy HL, Pathak BK, Srikrishnan P, Singh R, Joshua I. Implementation of infection prevention and control practices in an upcoming COVID-19 hospital in India: an opportunity not missed. PLoS One. 2022;17(5): e0268071.
Etebarian A, Khoramian Tusi S, Momeni Z, Hejazi K. Impact of educational intervention regarding COVID-19 on knowledge, attitude, and practice of students before dental school re-opening. BMC Oral Health. 2023;23(1):1–6.
Otu A, Okuzu O, Effa E, Ebenso B, Ameh S, Nihalani N, Onwusaka O, Tawose T, Olayinka A, Walley J. Training health workers at scale in Nigeria to fight COVID-19 using the InStrat COVID-19 tutorial app: an e-health interventional study. Ther Adv Infect Dis. 2021;8:20499361211040704.
CAS PubMed PubMed Central Google Scholar
Otu A, Okuzu O, Ebenso B, Effa E, Nihalani N, Olayinka A, Yaya S. Introduction of mobile health tools to support COVID-19 training and surveillance in Ogun State Nigeria. Front Sustain Cities. 2021;3: 638278.
Perera N, Haldane V, Ratnapalan S, Samaraweera S, Karunathilake M, Gunarathna C, Bandara P, Kawirathne P, Wei X. Implementation of a coronavirus disease 2019 infection prevention and control training program in a low-middle income country. Int J Evid Based Healthc. 2022;20(3):228–35.
PubMed Central Google Scholar
Rao S, Rohilla KK, Kathrotia R, Naithani M, Varghese A, Bahadur A, Dhar P, Aggarwal P, Gupta M, Kant R. Rapid workforce development to combat the COVID-19 pandemic: experience from a tertiary health care centre in North India. Cureus. 2021;13(6):e15585.
PubMed PubMed Central Google Scholar
Shehu N, Okwor T, Dooga J, Wele A, Cihambanya L, Okonkon I, Gadanya M, Sebastine J, Okoro B, Okafor O. Train-the-trainers intervention for national capacity building in infection prevention and control for COVID-19 in Nigeria. Heliyon. 2023;9(11).
Shrestha A, Shrestha A, Sonnenberg T, Shrestha R. COVID-19 emergency department protocols: experience of protocol implementation through in-situ simulation. Open Access Emerg Med. 2020;12:293–303.
Thomas MP, Kozikott S, Kamateeka M, Abdu-Aguye R, Agogo E, Bello BG, Brudney K, Manzi O, Patel LN, Barrera-Cancedda AE. Development of a simple and effective online training for health workers: results from a pilot in Nigeria. BMC Public Health. 2022;22(1):1–10.
Bemah P, Baller A, Cooper C, Massaquoi M, Skrip L, Rude JM, Twyman A, Moses P, Seifeldin R, Udhayashankar K. Strengthening healthcare workforce capacity during and post Ebola outbreaks in Liberia: an innovative and effective approach to epidemic preparedness and response. Pan Afr Med J. 2019;33(Suppl 2):9.
Bazeyo W, Bagonza J, Halage A, Okure G, Mugagga M, Musoke R, Tumwebaze M, Tusiime S, Ssendagire S, Nabukenya I. Ebola a reality of modern public health; need for surveillance, preparedness and response training for health workers and other multidisciplinary teams: a case for Uganda. Pan Afr Med J. 2015;20:20.
Carlos C, Capistrano R, Tobora CF, delos Reyes MR, Lupisan S, Corpuz A, Aumentado C, Suy LL, Hall J, Donald J. Hospital preparedness for Ebola virus disease: a training course in the Philippines. Western Pac Surveill Response J. 2015;6(1):33.
Jones-Konneh TEC, Murakami A, Sasaki H, Egawa S. Intensive education of health care workers improves the outcome of Ebola virus disease: lessons learned from the 2014 outbreak in Sierra Leone. Tohoku J Exp Med. 2017;243(2):101–5.
Kabego L, Kourouma M, Ousman K, Baller A, Milambo JP, Kombe J, Houndjo B, Boni FE, Musafiri C, Molembo S. Impact of multimodal strategies including a pay for performance strategy in the improvement of infection prevention and control practices in healthcare facilities during an Ebola virus disease outbreak. BMC Infect Dis. 2023;23(1):1–7.
Oji MO, Haile M, Baller A, Trembley N, Mahmoud N, Gasasira A, Ladele V, Cooper C, Kateh FN, Nyenswah T. Implementing infection prevention and control capacity building strategies within the context of Ebola outbreak in a” Hard-to-Reach” area of Liberia. Pan Afr Med J. 2018;31(1).
Otu A, Ebenso B, Okuzu O, Osifo-Dawodu E. Using a mHealth tutorial application to change knowledge and attitude of frontline health workers to Ebola virus disease in Nigeria: a before-and-after study. Hum Resour Health. 2016;14(1):1–9.
Ousman K, Kabego L, Talisuna A, Diaz J, Mbuyi J, Houndjo B, Ngandu JP, Omba G, Aruna A, Mossoko M. The impact of Infection Prevention and control (IPC) bundle implementationon IPC compliance during the Ebola virus outbreak in Mbandaka/Democratic Republic of the Congo: a before and after design. BMJ Open. 2019;9(9):e029717.
Soeters HM, Koivogui L, de Beer L, Johnson CY, Diaby D, Ouedraogo A, Touré F, Bangoura FO, Chang MA, Chea N. Infection prevention and control training and capacity building during the Ebola epidemic in Guinea. PLoS One. 2018;13(2): e0193291.
El-Sokkary RH, Negm EM, Othman HA, Tawfeek MM, Metwally WS. Stewardship actions for device associated infections: an intervention study in the emergency intensive care unit. J Infect Public Health. 2020;13(12):1927–31.
Wassef M, Mukhtar A, Nabil A, Ezzelarab M, Ghaith D. Care bundle approach to reduce surgical site infections in acute surgical intensive care unit, Cairo, Egypt. Infect Drug Resist. 2020;13:229–36.
Ahmed S, Bardhan PK, Iqbal A, Mazumder RN, Khan AI, Islam MS, Siddique AK, Cravioto A. The 2008 cholera epidemic in Zimbabwe: experience of the icddr,b team in the field. J Health Popul Nutr. 2011;29(5):541–5.
Carroll Á, Collins C, McKenzie J, Stokes D, Darley A. Application of complexity theory in health and social care research: a scoping review. BMJ Open. 2023;13(3): e069180.
Qureshi MO, Chughtai AA, Seale H. Recommendations related to occupational infection prevention and control training to protect healthcare workers from infectious diseases: a scoping review of infection prevention and control guidelines. BMC Health Serv Res. 2022;22(1):272.
Lowe H, Woodd S, Lange IL, Janjanin S, Barnett J, Graham W. Challenges and opportunities for infection prevention and control in hospitals in conflict-affected settings: a qualitative study. Confl Heal. 2021;15:1–10.
Google Scholar
Tan C, Kallon II, Colvin CJ, Grant AD. Barriers and facilitators of tuberculosis infection prevention and control in low-and middle-income countries from the perspective of healthcare workers: a systematic review. PLoS One. 2020;15(10): e0241039.
Silva MT, Galvao TF, Chapman E, da Silva EN, Barreto JOM. Dissemination interventions to improve healthcare workers’ adherence with infection prevention and control guidelines: a systematic review and meta-analysis. Implement Sci. 2021;16(1):1–15.
Jeihooni AK, Kashfi SH, Bahmandost M, Harsini PA. Promoting preventive behaviors of nosocomial infections in nurses: the effect of an educational program based on health belief model. Invest Educ Enferm. 2018;36(1):e09.
Song F, Hooper L, Loke YK. Publication bias: what is it? How do we measure it? How do we avoid it? Open Access J Clin Trials. 2013;5:71.
Kessy SJ, Gon G, Alimi Y, Bakare WA, Gallagher K, Hornsey E, Sithole L, Onwekwe EVC, Okwor T, Sekoni A. Training a continent: a process evaluation of virtual training on infection prevention and control in Africa during COVID-19. Glob Health Sci Pract. 2023;11(2):e2200051.
Tomczyk S, Storr J, Kilpatrick C, Allegranzi B. Infection prevention and control (IPC) implementation in low-resource settings: a qualitative analysis. Antimicrob Resist Infect Control. 2021;10(1):1–11.
Download references
Acknowledgements
We thank members of the WHO Expert Working Group for comments and guidance.
This work was primarily funded by a grant from the World Health Organization (WHO) based on a grant from the United States Centers for Disease Control and Prevention (US CDC). JB and ICS were also supported by the UK NHIR Health Protection Research Unit (NIHR HPRU) in Emergency Preparedness and Response at King’s College London in partnership with the UK Health Security Agency (UKHSA) in collaboration with the University of East Anglia. EH is affiliated with the UK Public Health Rapid Support Team, funded by UK Aid from the Department of Health and Social Care and is jointly run by UK Health Security Agency and the London School of Hygiene & Tropical Medicine. The views expressed are those of the author(s) and not necessarily those of the WHO, NHS, NIHR, UEA, UK Department of Health, UKHSA or US CDC.
Author information
Authors and affiliations.
Norwich Medical School University of East, Anglia Norwich, UK
Julii Brainard
UCL Medical School, University College London, London, UK
Isabel Catalina Swindells
OL4All, Oxford, UK
Joanna Wild
Department of Veterinary Medicine, University of Cambridge, Cambridge, UK
Charlotte Christiane Hammer
London School of Hygiene & Tropical Medicine, UK Public Health Rapid Support Team, UK Health Security Agency, and , London, UK
Emilio Hornsey
Country Readiness Strengthening, World Health Organization, Geneva, Switzerland
Hibak Osman Mahamed & Victoria Willet
You can also search for this author in PubMed Google Scholar
Contributions
Analysis plan: JB, CCH, JW, VW, HOM. Comments on draft manuscript: All. Conception: EH, JB, VW, HOM. Data acquisition and extraction: JB, ICS, JW. Data curation: JB. Data summary: JB. Funding: JB, CCH, VW, HOM. Interpretation: JB, CCH, VW, HOM. Research governance: JB. Screening: JB, ICS, CCH. Searches: JB, ICS. Writing first draft, assembling revisions: JB.
Corresponding author
Correspondence to Julii Brainard .
Ethics declarations
Ethics approval and consent to participate.
This research was exempt from needing ethics approval because the (anonymised and aggregated) information we analyse and describe was already in the public domain.
Consent for publication
Not applicable.
Competing interests
JW runs an educational consultancy that advises the WHO and other healthcare training delivery organisations. VW and HM work for the World Health Organization who commissioned this research. All other authors declare no competing interests.
Additional information
Publisher's note.
Springer Nature remains neutral with regard to jurisdictional claims in published maps and institutional affiliations.
Supplementary Information
Supplementary material 1., supplementary material 2., rights and permissions.
Open Access This article is licensed under a Creative Commons Attribution 4.0 International License, which permits use, sharing, adaptation, distribution and reproduction in any medium or format, as long as you give appropriate credit to the original author(s) and the source, provide a link to the Creative Commons licence, and indicate if changes were made. The images or other third party material in this article are included in the article's Creative Commons licence, unless indicated otherwise in a credit line to the material. If material is not included in the article's Creative Commons licence and your intended use is not permitted by statutory regulation or exceeds the permitted use, you will need to obtain permission directly from the copyright holder. To view a copy of this licence, visit http://creativecommons.org/licenses/by/4.0/ . The Creative Commons Public Domain Dedication waiver ( http://creativecommons.org/publicdomain/zero/1.0/ ) applies to the data made available in this article, unless otherwise stated in a credit line to the data.
Reprints and permissions
About this article
Cite this article.
Brainard, J., Swindells, I.C., Wild, J. et al. Emergency infection prevention and control training in fragile, conflict-affected or vulnerable settings: a scoping review. BMC Health Serv Res 24 , 937 (2024). https://doi.org/10.1186/s12913-024-11408-y
Download citation
Received : 27 May 2024
Accepted : 06 August 2024
Published : 16 August 2024
DOI : https://doi.org/10.1186/s12913-024-11408-y
Share this article
Anyone you share the following link with will be able to read this content:
Sorry, a shareable link is not currently available for this article.
Provided by the Springer Nature SharedIt content-sharing initiative
- Infection-control
BMC Health Services Research
ISSN: 1472-6963
- General enquiries: [email protected]
Information
- Author Services
Initiatives
You are accessing a machine-readable page. In order to be human-readable, please install an RSS reader.
All articles published by MDPI are made immediately available worldwide under an open access license. No special permission is required to reuse all or part of the article published by MDPI, including figures and tables. For articles published under an open access Creative Common CC BY license, any part of the article may be reused without permission provided that the original article is clearly cited. For more information, please refer to https://www.mdpi.com/openaccess .
Feature papers represent the most advanced research with significant potential for high impact in the field. A Feature Paper should be a substantial original Article that involves several techniques or approaches, provides an outlook for future research directions and describes possible research applications.
Feature papers are submitted upon individual invitation or recommendation by the scientific editors and must receive positive feedback from the reviewers.
Editor’s Choice articles are based on recommendations by the scientific editors of MDPI journals from around the world. Editors select a small number of articles recently published in the journal that they believe will be particularly interesting to readers, or important in the respective research area. The aim is to provide a snapshot of some of the most exciting work published in the various research areas of the journal.
Original Submission Date Received: .
- Active Journals
- Find a Journal
- Proceedings Series
- For Authors
- For Reviewers
- For Editors
- For Librarians
- For Publishers
- For Societies
- For Conference Organizers
- Open Access Policy
- Institutional Open Access Program
- Special Issues Guidelines
- Editorial Process
- Research and Publication Ethics
- Article Processing Charges
- Testimonials
- Preprints.org
- SciProfiles
- Encyclopedia
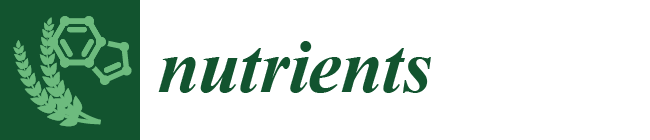
Article Menu

- Subscribe SciFeed
- Recommended Articles
- Google Scholar
- on Google Scholar
- Table of Contents
Find support for a specific problem in the support section of our website.
Please let us know what you think of our products and services.
Visit our dedicated information section to learn more about MDPI.
JSmol Viewer
Dietary supplementation on physical performance and recovery in active-duty military personnel: a systematic review of randomized and quasi-experimental controlled trials.
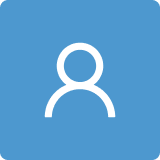
1. Introduction
2. materials and methods, 3.1. protein and carbohydrate, 3.2. beta-alanine and creatine, 3.3. mixed nutritional supplements, 3.4. probiotics, 3.5. phytonutrients, 4. discussion, 4.1. supplement use for muscle-related physical performance and recovery, 4.2. improvements in physical performance, 4.3. improvements in recovery or reduced injury, 4.4. limitations, 5. conclusions, author contributions, conflicts of interest.
- Xu, J.; Haigney, M.C.; Levine, B.D.; Dineen, E.H. The Tactical Athlete: Definitions, Cardiovascular Assessment, and Management, and “Fit for Duty” Standards. Cardiol. Clin. 2023 , 41 , 93–105. [ Google Scholar ] [ CrossRef ]
- Austin, K.G.; Price, L.L.; McGraw, S.M.; Leahy, G.; Lieberman, H.R. Demographic, Lifestyle Factors, and Reasons for Use of Dietary Supplements by Air Force Personnel. Aerosp. Med. Hum. Perform. 2016 , 87 , 628–637. [ Google Scholar ] [ CrossRef ] [ PubMed ]
- Austin, K.G.; Price, L.L.; McGraw, S.M.; McLellan, T.M.; Lieberman, H.R. Longitudinal Trends in Use of Dietary Supplements by U.S. Army Personnel Differ from Those of Civilians. Appl. Physiol. Nutr. Metab. Physiol. Appl. Nutr. Metab. 2016 , 41 , 1217–1224. [ Google Scholar ] [ CrossRef ]
- Knapik, J.J.; Austin, K.G.; Farina, E.K.; Lieberman, H.R. Dietary Supplement Use in a Large, Representative Sample of the US Armed Forces. J. Acad. Nutr. Diet. 2018 , 118 , 1370–1388. [ Google Scholar ] [ CrossRef ]
- Knapik, J.J.; Trone, D.W.; Steelman, R.A.; Farina, E.K.; Lieberman, H.R. Prevalence of and Factors Associated with Dietary Supplement Use in a Stratified, Random Sample of US Military Personnel: The US Military Dietary Supplement Use Study. J. Nutr. 2021 , 151 , 3495–3506. [ Google Scholar ] [ CrossRef ] [ PubMed ]
- Knapik, J.J.; Trone, D.W.; Austin, K.G.; Steelman, R.A.; Farina, E.K.; Lieberman, H.R. Prevalence, Adverse Events, and Factors Associated with Dietary Supplement and Nutritional Supplement Use by US Navy and Marine Corps Personnel. J. Acad. Nutr. Diet. 2016 , 116 , 1423–1442. [ Google Scholar ] [ CrossRef ]
- Maughan, R.J.; Burke, L.M.; Dvorak, J.; Larson-Meyer, D.E.; Peeling, P.; Phillips, S.M.; Rawson, E.S.; Walsh, N.P.; Garthe, I.; Geyer, H.; et al. IOC Consensus Statement: Dietary Supplements and the High-Performance Athlete. Br. J. Sports Med. 2018 , 52 , 439–455. [ Google Scholar ] [ CrossRef ] [ PubMed ]
- Crawford, C.; Avula, B.; Lindsey, A.T.; Katragunta, K.; Khan, I.A.; Deuster, P.A. Label Accuracy of Weight Loss Dietary Supplements Marketed Online with Military Discounts. JAMA Netw. Open 2024 , 7 , e249131. [ Google Scholar ] [ CrossRef ]
- Ouzzani, M.; Hammady, H.; Fedorowicz, Z.; Elmagarmid, A. Rayyan—A Web and Mobile App for Systematic Reviews. Syst. Rev. 2016 , 5 , 210. [ Google Scholar ] [ CrossRef ]
- Scanlan, C. Critical Review Form—Quantitative Studies. Available online: https://www.mcgill.ca/cpengine/files/cpengine/quanreview_form1.doc (accessed on 16 August 2024).
- Armentano, M.J.; Brenner, A.K.; Hedman, T.L.; Solomon, Z.T.; Chavez, J.; Kemper, G.B.; Salzberg, D.; Battafarano, D.F.; Christie, D.S. The Effect and Safety of Short-Term Creatine Supplementation on Performance of Push-Ups. Mil. Med. 2007 , 172 , 312–317. [ Google Scholar ] [ CrossRef ]
- Berryman, C.E.; Sepowitz, J.J.; McClung, H.L.; Lieberman, H.R.; Farina, E.K.; McClung, J.P.; Ferrando, A.A.; Pasiakos, S.M. Supplementing an Energy Adequate, Higher Protein Diet with Protein Does Not Enhance Fat-Free Mass Restoration after Short-Term Severe Negative Energy Balance. J. Appl. Physiol. 2017 , 122 , 1485–1493. [ Google Scholar ] [ CrossRef ] [ PubMed ]
- Fortes, M.B.; Diment, B.C.; Greeves, J.P.; Casey, A.; Izard, R.; Walsh, N.P. Effects of a Daily Mixed Nutritional Supplement on Physical Performance, Body Composition, and Circulating Anabolic Hormones during 8 Weeks of Arduous Military Training. Appl. Physiol. Nutr. Metab. 2011 , 36 , 967–975. [ Google Scholar ] [ CrossRef ]
- Gepner, Y.; Hoffman, J.R.; Shemesh, E.; Stout, J.R.; Church, D.D.; Varanoske, A.N.; Zelicha, H.; Shelef, I.; Chen, Y.; Frankel, H.; et al. Combined Effect of Bacillus coagulans GBI-30, 6086 and HMB Supplementation on Muscle Integrity and Cytokine Response during Intense Military Training. J. Appl. Physiol. 2017 , 123 , 11–18. [ Google Scholar ] [ CrossRef ]
- Hoffman, J.R.; Landau, G.; Stout, J.R.; Hoffman, M.W.; Shavit, N.; Rosen, P.; Moran, D.S.; Fukuda, D.H.; Shelef, I.; Carmom, E.; et al. β-Alanine Ingestion Increases Muscle Carnosine Content and Combat Specific Performance in Soldiers. Amino Acids 2015 , 47 , 627–636. [ Google Scholar ] [ CrossRef ]
- Hoffman, J.R.; Gepner, Y.; Hoffman, M.W.; Zelicha, H.; Shapira, S.; Ostfeld, I. Effect of High-Dose, Short-Duration β-Alanine Supplementation on Circulating IL-10 Concentrations During Intense Military Training. J. Strength Cond. Res. 2018 , 32 , 2978–2981. [ Google Scholar ] [ CrossRef ] [ PubMed ]
- Hoffman, J.R.; Hoffman, M.W.; Zelicha, H.; Gepner, Y.; Willoughby, D.S.; Feinstein, U.; Ostfeld, I. The Effect of 2 Weeks of Inactivated Probiotic Bacillus Coagulans on Endocrine, Inflammatory, and Performance Responses During Self-Defense Training in Soldiers. J. Strength Cond. Res. 2019 , 33 , 2330–2337. [ Google Scholar ] [ CrossRef ] [ PubMed ]
- Macedo, R.C.S.; Vieira, A.; Marin, D.P.; Otton, R. Effects of Chronic Resveratrol Supplementation in Military Firefighters Undergo a Physical Fitness Test—A Placebo-Controlled, Double Blind Study. Chem. Biol. Interact. 2015 , 227 , 89–95. [ Google Scholar ] [ CrossRef ] [ PubMed ]
- Marshall, A.R.; Rimmer, J.E.; Shah, N.; Bye, K.; Kipps, C.; Woods, D.R.; O’Hara, J.; Boos, C.J.; Barlow, M. Marching to the Beet: The Effect of Dietary Nitrate Supplementation on High Altitude Exercise Performance and Adaptation during a Military Trekking Expedition. Nitric Oxide 2021 , 113–114 , 70–77. [ Google Scholar ] [ CrossRef ]
- McAdam, J.; McGinnis, K.; Beck, D.; Haun, C.; Romero, M.; Mumford, P.; Roberson, P.; Young, K.; Lohse, K.; Lockwood, C.; et al. Effect of Whey Protein Supplementation on Physical Performance and Body Composition in Army Initial Entry Training Soldiers. Nutrients 2018 , 10 , 1248. [ Google Scholar ] [ CrossRef ]
- McGinnis, K.; McAdam, J.; Lockwood, C.; Young, K.; Roberts, M.; Sefton, J. Impact of Protein and Carbohydrate Supplementation on Musculoskeletal Injuries in Army Initial Entry Training Soldiers. Nutrients 2018 , 10 , 1938. [ Google Scholar ] [ CrossRef ]
- Samadi, M.; Askarian, A.; Shirvani, H.; Shamsoddini, A.; Shakibaee, A.; Forbes, S.C.; Kaviani, M. Effects of Four Weeks of Beta-Alanine Supplementation Combined with One Week of Creatine Loading on Physical and Cognitive Performance in Military Personnel. Int. J. Environ. Res. Public. Health 2022 , 19 , 7992. [ Google Scholar ] [ CrossRef ] [ PubMed ]
- Sharp, M.A.; Hendrickson, N.R.; Staab, J.S.; McClung, H.L.; Nindl, B.C.; Michniak-Kohn, B.B. Effects of Short-Term Quercetin Supplementation on Soldier Performance. J. Strength Cond. Res. 2012 , 26 , S53–S60. [ Google Scholar ] [ CrossRef ] [ PubMed ]
- Shirvani, H.; Bazgir, B.; Shamsoddini, A.; Saeidi, A.; Tayebi, S.M.; Escobar, K.A.; Laher, I.; VanDusseldorp, T.A.; Weiss, K.; Knechtle, B.; et al. Oregano ( Origanum vulgare ) Consumption Reduces Oxidative Stress and Markers of Muscle Damage after Combat Readiness Tests in Soldiers. Nutrients 2022 , 15 , 137. [ Google Scholar ] [ CrossRef ] [ PubMed ]
- Walker, T.B.; Smith, J.; Herrera, M.; Lebegue, B.; Pinchak, A.; Fischer, J. The Influence of 8 Weeks of Whey-Protein and Leucine Supplementation on Physical and Cognitive Performance. Int. J. Sport Nutr. Exerc. Metab. 2010 , 20 , 409–417. [ Google Scholar ] [ CrossRef ] [ PubMed ]
- Zwilling, C.E.; Strang, A.; Anderson, E.; Jurcsisn, J.; Johnson, E.; Das, T.; Kuchan, M.J.; Barbey, A.K. Enhanced Physical and Cognitive Performance in Active Duty Airmen: Evidence from a Randomized Multimodal Physical Fitness and Nutritional Intervention. Sci. Rep. 2020 , 10 , 17826. [ Google Scholar ] [ CrossRef ]
- Buford, T.W.; Kreider, R.B.; Stout, J.R.; Greenwood, M.; Campbell, B.; Spano, M.; Ziegenfuss, T.; Lopez, H.; Landis, J.; Antonio, J. International Society of Sports Nutrition Position Stand: Creatine Supplementation and Exercise. J. Int. Soc. Sports Nutr. 2007 , 4 , 6. [ Google Scholar ] [ CrossRef ] [ PubMed ]
- Kumar, V.; Atherton, P.; Smith, K.; Rennie, M.J. Human Muscle Protein Synthesis and Breakdown during and after Exercise. J. Appl. Physiol. 2009 , 106 , 2026–2039. [ Google Scholar ] [ CrossRef ] [ PubMed ]
- Morton, R.W.; Murphy, K.T.; McKellar, S.R.; Schoenfeld, B.J.; Henselmans, M.; Helms, E.; Aragon, A.A.; Devries, M.C.; Banfield, L.; Krieger, J.W.; et al. A Systematic Review, Meta-Analysis and Meta-Regression of the Effect of Protein Supplementation on Resistance Training-Induced Gains in Muscle Mass and Strength in Healthy Adults. Br. J. Sports Med. 2018 , 52 , 376–384. [ Google Scholar ] [ CrossRef ] [ PubMed ]
- Saunders, B.; Elliott-Sale, K.; Artioli, G.G.; Swinton, P.A.; Dolan, E.; Roschel, H.; Sale, C.; Gualano, B. β-Alanine Supplementation to Improve Exercise Capacity and Performance: A Systematic Review and Meta-Analysis. Br. J. Sports Med. 2017 , 51 , 658–669. [ Google Scholar ] [ CrossRef ]
- Bojarczuk, A.; Dzitkowska-Zabielska, M. Polyphenol Supplementation and Antioxidant Status in Athletes: A Narrative Review. Nutrients 2022 , 15 , 158. [ Google Scholar ] [ CrossRef ]
- Quaresma, M.V.L.D.S.; Mancin, L.; Paoli, A.; Mota, J.F. The Interplay between Gut Microbiome and Physical Exercise in Athletes. Curr. Opin. Clin. Nutr. Metab. Care 2024 , 27 , 428–433. [ Google Scholar ] [ CrossRef ] [ PubMed ]
- Abdulla, H.; Smith, K.; Atherton, P.J.; Idris, I. Role of Insulin in the Regulation of Human Skeletal Muscle Protein Synthesis and Breakdown: A Systematic Review and Meta-Analysis. Diabetologia 2016 , 59 , 44–55. [ Google Scholar ] [ CrossRef ]
- Elstad, K.; Malone, C.; Luedke, J.; Jaime, S.J.; Dobbs, W.C.; Almonroeder, T.; Kerksick, C.M.; Markert, A.; Jagim, A.R. The Effects of Protein and Carbohydrate Supplementation, with and without Creatine, on Occupational Performance in Firefighters. Nutrients 2023 , 15 , 5134. [ Google Scholar ] [ CrossRef ] [ PubMed ]
- Naderi, A.; de Oliveira, E.P.; Ziegenfuss, T.N.; Willems, M.E.T. Timing, Optimal Dose and Intake Duration of Dietary Supplements with Evidence-Based Use in Sports Nutrition. J. Exerc. Nutr. Biochem. 2016 , 20 , 1–12. [ Google Scholar ] [ CrossRef ]
- Hobson, R.M.; Harris, R.C.; Martin, D.; Smith, P.; Macklin, B.; Gualano, B.; Sale, C. Effect of Beta-Alanine, with and without Sodium Bicarbonate, on 2000-m Rowing Performance. Int. J. Sport Nutr. Exerc. Metab. 2013 , 23 , 480–487. [ Google Scholar ] [ CrossRef ]
- Kurtz, J.A.; Vandusseldorp, T.A.; Uken, B.; Otis, J. Quercetin in Sports and Exercise: A Review. Int. J. Exerc. Sci. 2023 , 16 , 1334–1384. [ Google Scholar ]
- Bornstein, D.B.; Sacko, R.S.; Nelson, S.P.; Grieve, G.; Beets, M.; Forrest, L.; Hauret, K.; Whitsel, L.; Jones, B. A State-by-State and Regional Analysis of the Direct Medical Costs of Treating Musculoskeletal Injuries among US Army Trainees. Prog. Cardiovasc. Dis. 2022 , 74 , 53–59. [ Google Scholar ] [ CrossRef ]
- Travis, T.W.; Brown, D.L. Human Performance Optimization: A Framework for the Military Health System. Mil. Med. 2023 , 188 , 44–48. [ Google Scholar ] [ CrossRef ]
- Mountjoy, M.; Sundgot-Borgen, J.; Burke, L.; Ackerman, K.E.; Blauwet, C.; Constantini, N.; Lebrun, C.; Lundy, B.; Melin, A.; Meyer, N.; et al. International Olympic Committee (IOC) Consensus Statement on Relative Energy Deficiency in Sport (RED-S): 2018 Update. Int. J. Sport Nutr. Exerc. Metab. 2018 , 28 , 316–331. [ Google Scholar ] [ CrossRef ] [ PubMed ]
- Jäger, R.; Mohr, A.E.; Carpenter, K.C.; Kerksick, C.M.; Purpura, M.; Moussa, A.; Townsend, J.R.; Lamprecht, M.; West, N.P.; Black, K.; et al. International Society of Sports Nutrition Position Stand: Probiotics. J. Int. Soc. Sports Nutr. 2019 , 16 , 62. [ Google Scholar ] [ CrossRef ]
- Beckner, M.E.; Main, L.; Tait, J.L.; Martin, B.J.; Conkright, W.R.; Nindl, B.C. Circulating Biomarkers Associated with Performance and Resilience during Military Operational Stress. Eur. J. Sport Sci. 2022 , 22 , 72–86. [ Google Scholar ] [ CrossRef ] [ PubMed ]
- Smith, E.S.; McKay, A.K.A.; Kuikman, M.; Ackerman, K.E.; Harris, R.; Elliott-Sale, K.J.; Stellingwerff, T.; Burke, L.M. Auditing the Representation of Female Versus Male Athletes in Sports Science and Sports Medicine Research: Evidence-Based Performance Supplements. Nutrients 2022 , 14 , 953. [ Google Scholar ] [ CrossRef ] [ PubMed ]
- Eliasson, A.; Kashani, M.; Dela Cruz, G.; Vernalis, M. Readiness and Associated Health Behaviors and Symptoms in Recently Deployed Army National Guard Solders. Mil. Med. 2012 , 177 , 1254–1260. [ Google Scholar ] [ CrossRef ] [ PubMed ]
Click here to enlarge figure
Database | Search Terms Used |
---|---|
CINAHL | (Military personnel or soldiers or armed forces or service men) AND (supplements dietary) OR strength OR performance OR recovery OR muscle OR strength performance AND (“randomized controlled trials”) AND (“longitudinal”)—peer reviewed, randomized control trials, past 10 years, 19–44 years old |
Medline | (((supplement OR protein OR “vitamin D” OR “fish oils” OR “omega 3” OR “collagen” OR “creatine” OR “beta alanine”)) AND (“tactical athlete” OR soldier OR warfighter OR sailor OR army OR marine OR navy OR coast guard OR air force)) AND (“brain health” OR “mental health” OR “performance” OR “recovery” OR “strength”) |
Participants | Active-duty military members, aged 19 years or older |
Intervention | Dietary supplementation defined as the provision of nutrients or food separate from the diet OR as a part of the diet AND approved by Department of Defense |
Comparisons | Control group receiving a placebo or no nutrient/dietary supplementation |
Outcomes | Subjective and objective measures of muscle performance, recovery, or body composition |
Study Design | Randomized Controlled Trial |
Reference | Participants | Study Design | Nutrition Intervention | Primary Physical Outcomes | Results |
---|---|---|---|---|---|
Armentano et al., 2017 [ ] | United States Army soldiers, n = 20 men and n = 15 women | Double-blind, placebo controlled | Group 1: 5 g creatine, 4× per day; Group 2: 5 g taurine, 16 g sucrose, 4× per day for 7 days | Performance | 2 min pushup: no significant difference in creatine and taurine groups (p = 0.0437) Creatine: serum creatine levels (p < 0.001), no changes in body composition, weight, blood pressure or serum phosphokinase levels |
Berryman et al., 2017 [ ] | United States Marines, n = 63 male | Randomized, double-blind, placebo controlled | Group 1: Higher protein (133 g/day); Group 2: Moderate protein (84 g/day); Group 3: Carbohydrate based low protein (7 g/day); Intervention was 27-days after 7 days of caloric restriction (CR) during training | Recovery | Total body mass (TBM; 5.8 1.0 kg, 7.0%), FFM (3.1 1.6 kg, 4.7%), and net protein balance (1.7 1.1 g protein·kg 1 ·day 1) were lower after CR. After 27-days, TBM (5.9 1.7 kg, 7.8%) and FFM (3.6 1.8 kg, 5.7%) improved in all groups. |
Fortes et al., 2011 [ ] | British Soldiers, n = 30 male | Quasi-Experimental Controlled | Group 1: habitual diet alone (CON); Group 2: habitual diet and daily mixed supplement with increased calories (SUP); Intervention was 8 weeks | Performance | Body mass loss (mean ± SD) (CON 5.0 ± 2.3, SUP 1.6 ± 1.5 kg), lean mass loss (CON 2.0 ± 1.5, SUP 0.7 ± 1.5 kg), and fat mass loss (CON 3.0 ± 1.6, SUP 0.9 ± 1.8 kg) were significantly blunted by SUP. CON experienced significant decrements in maximum dynamic lift strength (14%), vertical jump (10%), and explosive leg power (11%) that were prevented by SUP. |
Gepner et al., 2017 [ ] | Israel Defense Force soldiers; n = 26 male | Double-blind, parallel design | Group 1: Beta hydroxy beta methylbutyrate calcium (CaHMB; 3 g) with Bacillus coagulans (BC30); Group 2: CaHMB 3 g with placebo; Group 3: control group; Intervention was 20 days. | Recovery | Significant attenuation in IL-1, IL-2, IL-6, CX3CL1, and TNF for both CaHMBBC30 and CaHMBPL compared with the control. Significant differences post test in plasma IL-6 (F = 6.27, p = 0.012) and IL-10 (F = 3.72, p = 0.041) concentrations. Positive effects on muscle integrity in rectus femoris (p = 0.014) and vastus lateralis (p = 0.23). No changes in body mass between groups (p = 0.22). |
Hoffman et. al., 2015 [ ] | Israel Defense Force Soldiers; n = 18 male | Randomized, double-blind study, placebo controlled | Group 1: 6 g/day beta-alanine; Group 2: 6 g/day placebo (rice flour); Intervention was 30 days | Recovery | Elevation in muscle carnosine content (p = 0.048) associated with improvement in fatigue (p = 0.06). Improvement in 50 m casualty carry (p = 0.044), and serial subtraction test (p = 0.022) with no differences in 1 min sprint, repeat sprint, marksmanship performance, or 2.5 km run. |
Hoffman et. al., 2018 [ ] | Israel Defense Force Soldiers; n = 20 male | Double-blind, parallel field study | Group 1: 6 g/day of sustained release beta-alanine (BA); Group 2: 6 g/day of rice powder (PL); Intervention was 30 days | Recovery | Changes in circulating IL-10 concentrations (mean difference 0.86 pg/mL) was possibly greater (57%) for BA than PL |
Hoffman et. al., 2018 [ ] | Israel Defense Force Soldiers; n = 16 male | Double-blind, parallel design | Group 1: Inactivated Bacillus coagulans (iBC) supplement, 1.0 × 10 colony-forming units; Group 2: Placebo; Intervention was 14 days. | Performance | In all analyses of performance (jump test, maximum pull ups, simulated casualty drag, shuttle run) and blood (cytokines and chemokines), there were no significant differences between groups. Magnitude based inferential analysis demonstrated changes in jump test and the casualty drag could have been advantageous (90.7% and 80.4% likelihood effect) |
Macedo et. al., 2014 [ ] | Brazilian military firefighters, n = 60 male | Triple-blind, placebo controlled | Group 1: 100 mg of resveratrol; Group 2: 100 mg of placebo; Intervention was 90 days | Recovery | Glucose (p = 96.06 ± 2.67) and triglycerides (p = 5.4 ± 1.43) were elevated post test in resveratrol group, but IL-6 and TNFa were reduced by 29% and 39%. Total antioxidant capacity was increased in both resveratrol and placebo groups. |
Marshall et. al., 2021 [ ] | British Military Soldiers; n = 12 male, n = 10 female | Single-blind, randomized control | Group 1: 14-mL concentrated beet root juice (BRJ; 12.5 mmol nitrate); Group 2: 14 mL calorie, color, volume—matched dose with 15.4 g maltodextrin, 2.8 g protein, 14 mL’s blackcurrant cordial with negligible phytochemical content and 70 mL’s Buxton; Intervention was 17 days. | Performance | BRJ enhanced the salivary levels of nitrite (p = 0.007). Harvard Step Test: Scores declined as altitude increased in control group (p = 0.003), no decline as altitude increased (p = 0.26) in BRJ group. Heart rate recovery was prolonged in control group (p < 0.01), unchanged with BRJ (p = 0.61) |
McAdam et al., 2017 [ ] | United States Army Soldiers, n = 69 male | Repeated measure, double-blind, parallel group | Group 1: Whey protein (77 g/day), 2× per day; Group 2: Energy matched carbohydrate (127 g/day, 2× per day; Intervention was 8 weeks | Performance | Post-testing pushup averaged 7 repetitions more in whey protein group (p < 0.001) with post-training fat mass loss (p = 0.01). No change in run time (p = 0.065) or fat free mass (F = 0.70, p = 0.41) |
McGinnis et. al., 2018 [ ] | United States Army Soldiers, n = 2175 male | Quasi experimental, double-blind, controlled | Group 1: One protein (38.6 g, 293 kcal), after physical training and before bed, or before bed only; Group 2: One carbohydrate (63.4 g, 291 kcal), after physical training and before bed, or before bed only; Group 3: Two protein (77.2 g, 586 kcal), after physical training and before bed, or before bed only; Group 4: Two carbohydrate servings/day (126.8 g, 582 kcal) after physical training and before bed, or before bed only; Control: historical data | Injury | Non supplemented soldiers were 5× more likely to sustain musculoskeletal injury (MSI) (p < 0.001) and 4× more likely to miss training p = 0.003) compared totwoservings of protein; Non supplemented soldiers missed 5 additional training days (p = 0.02) compared totwoservings of protein. One serving of protein per day was 3× more likely to have MSI thantwoservings (p = 0.002) |
Samadi et. al., 2022 [ ] | Iranian Soldiers, n = 20 male | Double-blind, randomized | Group 1: 6.4 g/day beta-alanine plus 0.3 g/kg creatine (BA + Cr); Group 2: 6.4 g/day beta-alanine plus placebo (BA + Pl); Intervention was 28 days. | Performance | BA + Cr increased bench press (p = 0.026), leg press (p = 0.017), vertical jump (p = 0.009), and sprint power (0.023) and simulated casualty test (p = 0.003), while BA + Pl did not. Vertical jump (p = 0.005) and testosterone (p = 0.006) were increased in BA + Cr compared to BA + Pl. No changes in cortisol, IGF-1, or lactate in either group. |
Sharp et. al., 2012 [ ] | United States Army Soldiers, n = 16 male | Double-blind, placebo controlled, crossover study | Group 1: 1000 mg of quercetin (food bar); Group 2: 1000 mg of placebo (food bar); Intervention was 7 days. | Performance | Quercetin supplementation did not show any aerobic changes in performance (VO2peak, time trial, respiratory exchange ratio, ratings of perceived exertion). |
Shirvani et al., 2022 [ ] | Iranian Soldiers, n = 24 male | Randomized block design | Group 1: 500 mg oregano immediately after exercise; Group 2: 500 mg of placebo (starch); Intervention only measured after one day of supplementation and testing. | Recovery | Oregano supplementation demonstrated improvements in creatine kinase (p < 0.0001), lactate dehydrogenase (p < 0.0001), malondialdehyde (p < 0.0001), super oxide dismutase (p < 0.0001), antioxidant capacity (p < 0.0001) and glutathione peroxidase (p < 0.0001). In all variables, the difference between placebo and oregano groups were significant at 60 (p < 0.0001) and 120 (p < 0.0001) minutes after army combat readiness test. |
Walker et al., 2010 [ ] | United States Air Force Airmen, n = 24 male, n = 6 male non-military | Randomized, double-blind | Group 1: Whey protein (19.7 g) and leucine (6.2 g); Group 2: Energy matched placebo; Intervention was 8 weeks | Performance | Overall, 55.6% of participants consuming whey protein demonstrated 5% or greater improvement compared to only 16.7% of individuals in the placebo group (p = 0.033). Whey Protein saw improvement of 12.8% pushup and 7.2% crunch increase while, placebo showed 7.6% and 3.4% increases, respectively with no between group differences. No difference was noted for run time, pull ups, or cognitive tests. |
Zwilling et al., 2020 [ ] | United States Airforce Airmen, n = 107 male and n = 41 female | Randomized Controlled Trial | Group 1: Two 8-oz mixed nutritional supplement (Protein, Carbohydrate, Fat, Ca-HMB, Choline, DHA, Folic Acid, Lutein, Magnesium, Phospholipid, Selenium, Vitamins: B1, B2, B3, B5, B6, B12, C, D, E, and Zinc) plus exercise; Group 2: Two 8-oz placebo plus exercise; Intervention: 12 weeks. | Performance | Relative to exercise alone, the mixed ingredient supplement resulted in increased lean muscle mass (p = 0.0003), decreased heart rate (p = 0.007), improved working memory (p = 0.01) and processing efficiency (p = 0.08). There was no significant difference between placebo and supplementation for strength and endurance, mobility and stability, or power. |
The statements, opinions and data contained in all publications are solely those of the individual author(s) and contributor(s) and not of MDPI and/or the editor(s). MDPI and/or the editor(s) disclaim responsibility for any injury to people or property resulting from any ideas, methods, instructions or products referred to in the content. |
Share and Cite
Harlow, J.; Blodgett, K.; Stedman, J.; Pojednic, R. Dietary Supplementation on Physical Performance and Recovery in Active-Duty Military Personnel: A Systematic Review of Randomized and Quasi-Experimental Controlled Trials. Nutrients 2024 , 16 , 2746. https://doi.org/10.3390/nu16162746
Harlow J, Blodgett K, Stedman J, Pojednic R. Dietary Supplementation on Physical Performance and Recovery in Active-Duty Military Personnel: A Systematic Review of Randomized and Quasi-Experimental Controlled Trials. Nutrients . 2024; 16(16):2746. https://doi.org/10.3390/nu16162746
Harlow, Jacie, Kylie Blodgett, Jenna Stedman, and Rachele Pojednic. 2024. "Dietary Supplementation on Physical Performance and Recovery in Active-Duty Military Personnel: A Systematic Review of Randomized and Quasi-Experimental Controlled Trials" Nutrients 16, no. 16: 2746. https://doi.org/10.3390/nu16162746
Article Metrics
Article access statistics, further information, mdpi initiatives, follow mdpi.
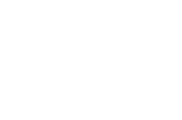
Subscribe to receive issue release notifications and newsletters from MDPI journals
Grab your spot at the free arXiv Accessibility Forum
Help | Advanced Search
Mathematics > Logic
Title: quasi-invariant measures concentrating on countable structures.
Abstract: Countable $\mathcal{L}$-structures $\mathcal{N}$ whose isomorphism class supports a permutation invariant probability measure in the logic action have been characterized by Ackerman-Freer-Patel to be precisely those $\mathcal{N}$ which have no algebraicity. Here we characterize those countable $\mathcal{L}$-structure $\mathcal{N}$ whose isomorphism class supports a quasi-invariant probability measure. These turn out to be precisely those $\mathcal{N}$ which are not "highly algebraic" -- we say that $\mathcal{N}$ is highly algebraic if outside of every finite $F$ there is some $b$ and a tuple $\bar{a}$ disjoint from $b$ so that $b$ has a finite orbit under the pointwise stabilizer of $\bar{a}$ in $\mathrm{Aut}(\mathcal{N})$. As a bi-product of our proof we show that whenever the isomorphism class of $\mathcal{N}$ admits a quasi-invariant measure, then it admits one with continuous Radon--Nikodym cocycles.
Subjects: | Logic (math.LO); Dynamical Systems (math.DS) |
Cite as: | [math.LO] |
(or [math.LO] for this version) | |
Focus to learn more arXiv-issued DOI via DataCite |
Submission history
Access paper:.
- HTML (experimental)
- Other Formats

References & Citations
- Google Scholar
- Semantic Scholar
BibTeX formatted citation

Bibliographic and Citation Tools
Code, data and media associated with this article, recommenders and search tools.
- Institution
arXivLabs: experimental projects with community collaborators
arXivLabs is a framework that allows collaborators to develop and share new arXiv features directly on our website.
Both individuals and organizations that work with arXivLabs have embraced and accepted our values of openness, community, excellence, and user data privacy. arXiv is committed to these values and only works with partners that adhere to them.
Have an idea for a project that will add value for arXiv's community? Learn more about arXivLabs .

IMAGES
COMMENTS
True experimental design Quasi-experimental design; Assignment to treatment: The researcher randomly assigns subjects to control and treatment groups.: Some other, non-random method is used to assign subjects to groups. Control over treatment: The researcher usually designs the treatment.: The researcher often does not have control over the treatment, but instead studies pre-existing groups ...
A quasi-experiment is an empirical interventional study used to estimate the causal impact of an intervention on target population without random assignment.Quasi-experimental research shares similarities with the traditional experimental design or randomized controlled trial, but it specifically lacks the element of random assignment to treatment or control.
Quasi-experimental Designs That Use Control Groups and Pretests. The reader should note that with all the studies in this category, the intervention is not randomized. The control groups chosen are comparison groups. Obtaining pretest measurements on both the intervention and control groups allows one to assess the initial comparability of the ...
In contrast, true experiments use random assignment to the treatment and control groups to control confounding variables, making them the gold standard for identifying cause-and-effect relationships.. Quasi-experimental research is a design that closely resembles experimental research but is different. The term "quasi" means "resembling," so you can think of it as a cousin to actual ...
quasi-experimental designs for prospective evalution of interventions Table 1 summarizes the main QEDs that have been used for prospective evaluation of health intervention in real-world settings; pre-post designs with a non-equivalent control group, interrupted time series and stepped wedge designs.
The strongest quasi-experimental designs for causal inference are regression discontinuity designs, instrumental variable designs, matching and propensity score designs, and comparative interrupted time series designs. ... For a dichotomous treatment variable (i.e., a treatment and control condition), each subject i has a potential treatment ...
Chapter 11: Quasi-Experimental Designs Psychology 2301 Introduction to Research Methods ... !Control for extraneous variables!Measure dependent variable!Differences in dependent measure are due to independent variable (internal validity) Quasi-Experimental Design!If no manipulation is performed on the IV, the design is correlational.
An experimental design is a randomized study design used to evaluate the effect of an intervention. In its simplest form, the participants will be randomly divided into 2 groups: A treatment group: where participants receive the new intervention which effect we want to study. A control or comparison group: where participants do not receive any ...
Quasi-Experimental Design. Quasi-Experimental Design is a unique research methodology because it is characterized by what is lacks. ... encompassing pre-experiments, quasi-experiments making use of a control or comparison group, and time-series designs. An introductory chapter describes the valuable role these types of studies have played in ...
Quasi-experimental design is a research method that seeks to evaluate the causal relationships between variables, but without the full control over the independent variable (s) that is available in a true experimental design. In a quasi-experimental design, the researcher uses an existing group of participants that is not randomly assigned to ...
Quasi-experimental designs do not randomly assign participants to treatment and control groups. Quasi-experimental designs identify a comparison group that is as similar as possible to the treatment group in terms of pre-intervention (baseline) characteristics. There are different types of quasi -experimental designs and they use different ...
The prefix quasi means "resembling." Thus quasi-experimental research is research that resembles experimental research but is not true experimental research. Although the independent variable is manipulated, participants are not randomly assigned to conditions or orders of conditions (Cook & Campbell, 1979). [1] Because the independent variable is manipulated before the dependent variable ...
In traditional experimental designs, randomization is a powerful tool for ensuring that groups are equivalent at the outset of a study. However, quasi-experimental design often involves non-randomization due to the nature of the research. This means that participants are not randomly assigned to treatment and control groups.
AIMS OF THIS CHAPTER. This chapter deals with experiments where, for a variety of reasons, you do not have full control over the allocation of participants to experimental conditions as is required in true experiments. Three common quasi-experimental designs are described; the non-equivalent control group design, the time series design and the ...
A quasi-experimental study (also known as a non-randomized pre-post intervention) is a research design in which the independent variable is manipulated, but participants are not randomly assigned to conditions. Commonly used in medical informatics (a field that uses digital information to ensure better patient care), researchers generally use ...
The researchers test whether differences in this outcome are related to the treatment. Differences between true experiments and quasi-experiments: In a true experiment, participants are randomly assigned to either the treatment or the control group, whereas they are not assigned randomly in a quasi-experiment. In a quasi-experiment, the control ...
using the full array of quasi-experimental designs possible and, in the third example, statistical control of variance. I give three examples here. Explore the designs in "types of true experiments" to see how you can adapt those designs to strengthen quasi-experiments. Also
A quasi-experimental design can be a great option when ethical or practical concerns make true experiments impossible, but the research methodology does have its drawbacks. Learn all the ins and outs of a quasi-experimental design. END-OF-SUMMER SALE. Get 40% off . END-OF-SUMMER SALE. Get 40% off .
Abstract. This chapter introduces readers to main concepts in experimental and quasi-experimental design. First, randomized control trials are introduced as the primary example of experimental design. Next, nonexperimental contexts, and particularly the use of propensity score matching to approximate the conditions of randomized control trials ...
Quasi-experimental designs include pre-post designs with a nonequivalent control group, interrupted time series (ITS), and stepped wedge designs. Stepped wedges are studies in which all participants receive the intervention, but in a staggered fashion. It is important to note that quasi-experimental designs are not unique to implementation science.
A quasi-experimental design is pretty much different from an experimental design, except for the fact that they both manifest the cause-effect relationship between the independent and dependent variables. ... This means using a control group in the experiment. In this group, researchers keep the independent variable constant. This control group ...
Lack of control: Case studies lack experimental control, which makes it difficult to establish cause-and-effect relationships. It is also difficult to replicate the same conditions across different cases, making it difficult to determine if the findings are consistent. ... Quasi-experimental designs are common in psychology research and feature ...
Describe three different types of quasi-experimental research designs (nonequivalent groups, pretest-posttest, and interrupted time series) and identify examples of each one. The prefix quasi means "resembling.". Thus quasi-experimental research is research that resembles experimental research but is not true experimental research.
The purpose of this quantitative, quasi-experimental study was to determine the effect of small group learning during the core mathematics block on 5th-grade students' mathematical resilience, compared to a control group. Student collaboration and mathematical discourse decreased during the COVID-19 pandemic, leading to a drop in math proficiency levels in the United States.
This article takes STC long and short columns as the research objects, and first conducts quasi-static tests to preliminarily explore the failure characteristics of STC long and short columns under...
Figure 1 shows the study selection process. 29 studies were included. Extracted data for each study are in File S2.Almost all (n = 27) were pre-post design; 2 were experimental studies [13, 14].Table 1 lists summary information about the included studies. Seven reports described training delivered in single low-income countries, 19 studies described training in single lower middle income ...
Methods: Randomized controlled trials and quasi-experimental controlled trials of oral dietary supplementation in active-duty military members were examined. A protocol was registered (PROSPERO CRD42023401472), and a systematic search of MEDLINE and CINAHL was undertaken. ... Studies were excluded if the design was case-control, cohort, cross ...
View a PDF of the paper titled Quasi-Monte Carlo Beyond Hardy-Krause, by Nikhil Bansal and 1 other authors. View PDF HTML ... arXivLabs: experimental projects with community collaborators. arXivLabs is a framework that allows collaborators to develop and share new arXiv features directly on our website.
Abstract. In recent years, the demand for efficient waterborne transportation has led to the development of high-speed catamarans. However, increased operating speeds result in elevated ship motions, making ride control systems desirable for passenger comfort, crew workability, and operational efficiency. This paper presents comprehensive towing tank experiments on a 2.5 m hydroelastic ...
View a PDF of the paper titled Quasi-invariant measures concentrating on countable structures, by Clinton Conley and 2 other authors. View PDF HTML (experimental) Abstract: Countable $\mathcal{L}$-structures $\mathcal{N}$ whose isomorphism class supports a permutation invariant probability measure in the logic action have been characterized by ...