- Skip to main content
- Skip to primary sidebar
- Skip to footer
- QuestionPro

- Solutions Industries Gaming Automotive Sports and events Education Government Travel & Hospitality Financial Services Healthcare Cannabis Technology Use Case AskWhy Communities Audience Contactless surveys Mobile LivePolls Member Experience GDPR Positive People Science 360 Feedback Surveys
- Resources Blog eBooks Survey Templates Case Studies Training Help center

Home Market Research Research Tools and Apps

Analytical Research: What is it, Importance + Examples
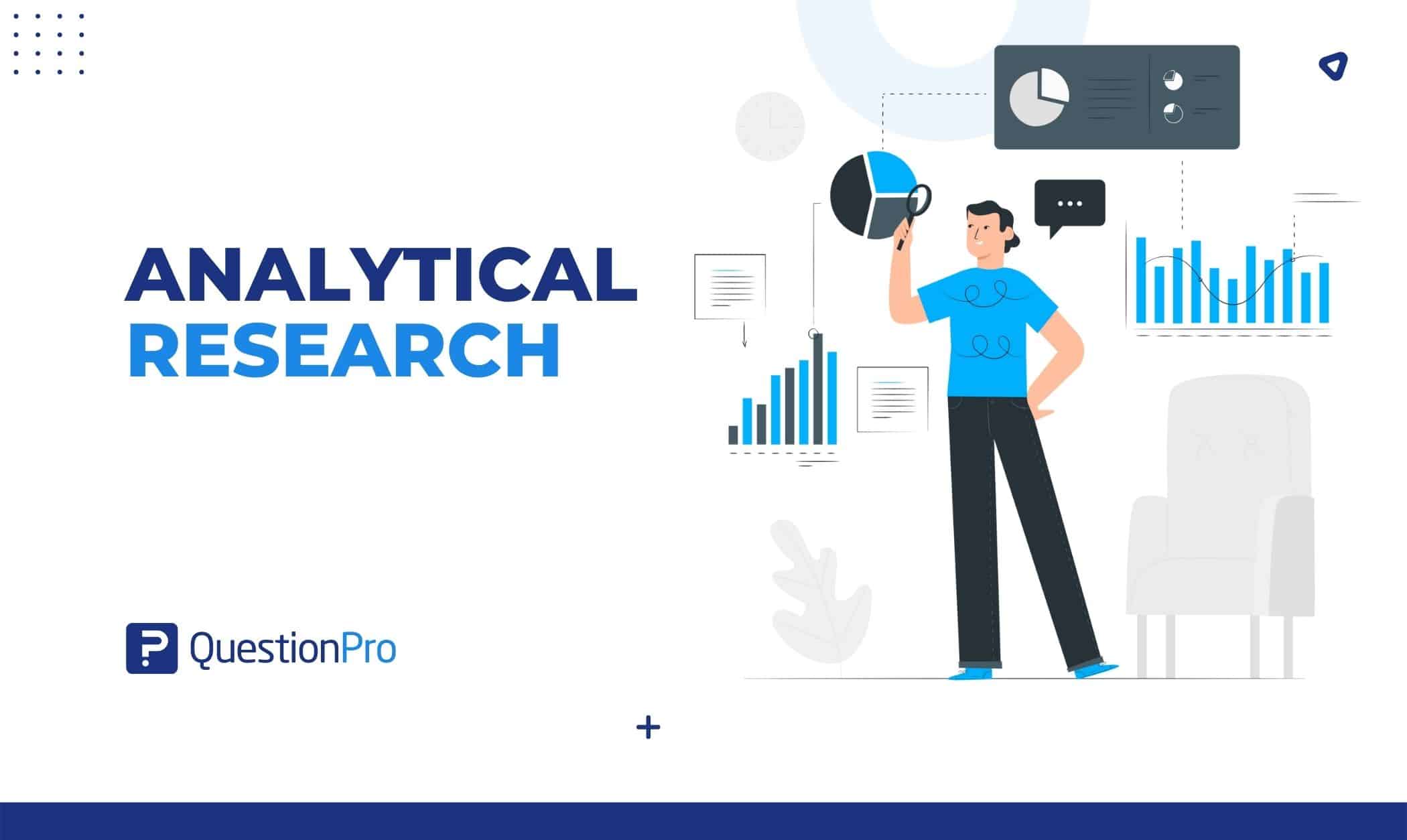
Finding knowledge is a loose translation of the word “research.” It’s a systematic and scientific way of researching a particular subject. As a result, research is a form of scientific investigation that seeks to learn more. Analytical research is one of them.
Any kind of research is a way to learn new things. In this research, data and other pertinent information about a project are assembled; after the information is gathered and assessed, the sources are used to support a notion or prove a hypothesis.
An individual can successfully draw out minor facts to make more significant conclusions about the subject matter by using critical thinking abilities (a technique of thinking that entails identifying a claim or assumption and determining whether it is accurate or untrue).
What is analytical research?
This particular kind of research calls for using critical thinking abilities and assessing data and information pertinent to the project at hand.
Determines the causal connections between two or more variables. The analytical study aims to identify the causes and mechanisms underlying the trade deficit’s movement throughout a given period.
It is used by various professionals, including psychologists, doctors, and students, to identify the most pertinent material during investigations. One learns crucial information from analytical research that helps them contribute fresh concepts to the work they are producing.
Some researchers perform it to uncover information that supports ongoing research to strengthen the validity of their findings. Other scholars engage in analytical research to generate fresh perspectives on the subject.
Various approaches to performing research include literary analysis, Gap analysis , general public surveys, clinical trials, and meta-analysis.
Importance of analytical research
The goal of analytical research is to develop new ideas that are more believable by combining numerous minute details.
The analytical investigation is what explains why a claim should be trusted. Finding out why something occurs is complex. You need to be able to evaluate information critically and think critically.
This kind of information aids in proving the validity of a theory or supporting a hypothesis. It assists in recognizing a claim and determining whether it is true.
Analytical kind of research is valuable to many people, including students, psychologists, marketers, and others. It aids in determining which advertising initiatives within a firm perform best. In the meantime, medical research and research design determine how well a particular treatment does.
Thus, analytical research can help people achieve their goals while saving lives and money.
Methods of Conducting Analytical Research
Analytical research is the process of gathering, analyzing, and interpreting information to make inferences and reach conclusions. Depending on the purpose of the research and the data you have access to, you can conduct analytical research using a variety of methods. Here are a few typical approaches:
Quantitative research
Numerical data are gathered and analyzed using this method. Statistical methods are then used to analyze the information, which is often collected using surveys, experiments, or pre-existing datasets. Results from quantitative research can be measured, compared, and generalized numerically.
Qualitative research
In contrast to quantitative research, qualitative research focuses on collecting non-numerical information. It gathers detailed information using techniques like interviews, focus groups, observations, or content research. Understanding social phenomena, exploring experiences, and revealing underlying meanings and motivations are all goals of qualitative research.
Mixed methods research
This strategy combines quantitative and qualitative methodologies to grasp a research problem thoroughly. Mixed methods research often entails gathering and evaluating both numerical and non-numerical data, integrating the results, and offering a more comprehensive viewpoint on the research issue.
Experimental research
Experimental research is frequently employed in scientific trials and investigations to establish causal links between variables. This approach entails modifying variables in a controlled environment to identify cause-and-effect connections. Researchers randomly divide volunteers into several groups, provide various interventions or treatments, and track the results.
Observational research
With this approach, behaviors or occurrences are observed and methodically recorded without any outside interference or variable data manipulation . Both controlled surroundings and naturalistic settings can be used for observational research . It offers useful insights into behaviors that occur in the actual world and enables researchers to explore events as they naturally occur.
Case study research
This approach entails thorough research of a single case or a small group of related cases. Case-control studies frequently include a variety of information sources, including observations, records, and interviews. They offer rich, in-depth insights and are particularly helpful for researching complex phenomena in practical settings.
Secondary data analysis
Examining secondary information is time and money-efficient, enabling researchers to explore new research issues or confirm prior findings. With this approach, researchers examine previously gathered information for a different reason. Information from earlier cohort studies, accessible databases, or corporate documents may be included in this.
Content analysis
Content research is frequently employed in social sciences, media observational studies, and cross-sectional studies. This approach systematically examines the content of texts, including media, speeches, and written documents. Themes, patterns, or keywords are found and categorized by researchers to make inferences about the content.
Depending on your research objectives, the resources at your disposal, and the type of data you wish to analyze, selecting the most appropriate approach or combination of methodologies is crucial to conducting analytical research.
Examples of analytical research
Analytical research takes a unique measurement. Instead, you would consider the causes and changes to the trade imbalance. Detailed statistics and statistical checks help guarantee that the results are significant.
For example, it can look into why the value of the Japanese Yen has decreased. This is so that an analytical study can consider “how” and “why” questions.
Another example is that someone might conduct analytical research to identify a study’s gap. It presents a fresh perspective on your data. Therefore, it aids in supporting or refuting notions.
Descriptive vs analytical research
Here are the key differences between descriptive research and analytical research:
Aspect | Descriptive Research | Analytical Research |
Objective | Describe and document characteristics or phenomena. | Analyze and interpret data to understand relationships or causality. |
Focus | “What” questions | “Why” and “How” questions |
Data Analysis | Summarizing information | Statistical research, hypothesis testing, qualitative research |
Goal | Provide an accurate and comprehensive description | Gain insights, make inferences, provide explanations or predictions |
Causal Relationships | Not the primary focus | Examining underlying factors, causes, or effects |
Examples | Surveys, observations, case-control study, content analysis | Experiments, statistical research, qualitative analysis |
The study of cause and effect makes extensive use of analytical research. It benefits from numerous academic disciplines, including marketing, health, and psychology, because it offers more conclusive information for addressing research issues.
QuestionPro offers solutions for every issue and industry, making it more than just survey software. For handling data, we also have systems like our InsightsHub research library.
You may make crucial decisions quickly while using QuestionPro to understand your clients and other study subjects better. Make use of the possibilities of the enterprise-grade research suite right away!
LEARN MORE FREE TRIAL
MORE LIKE THIS
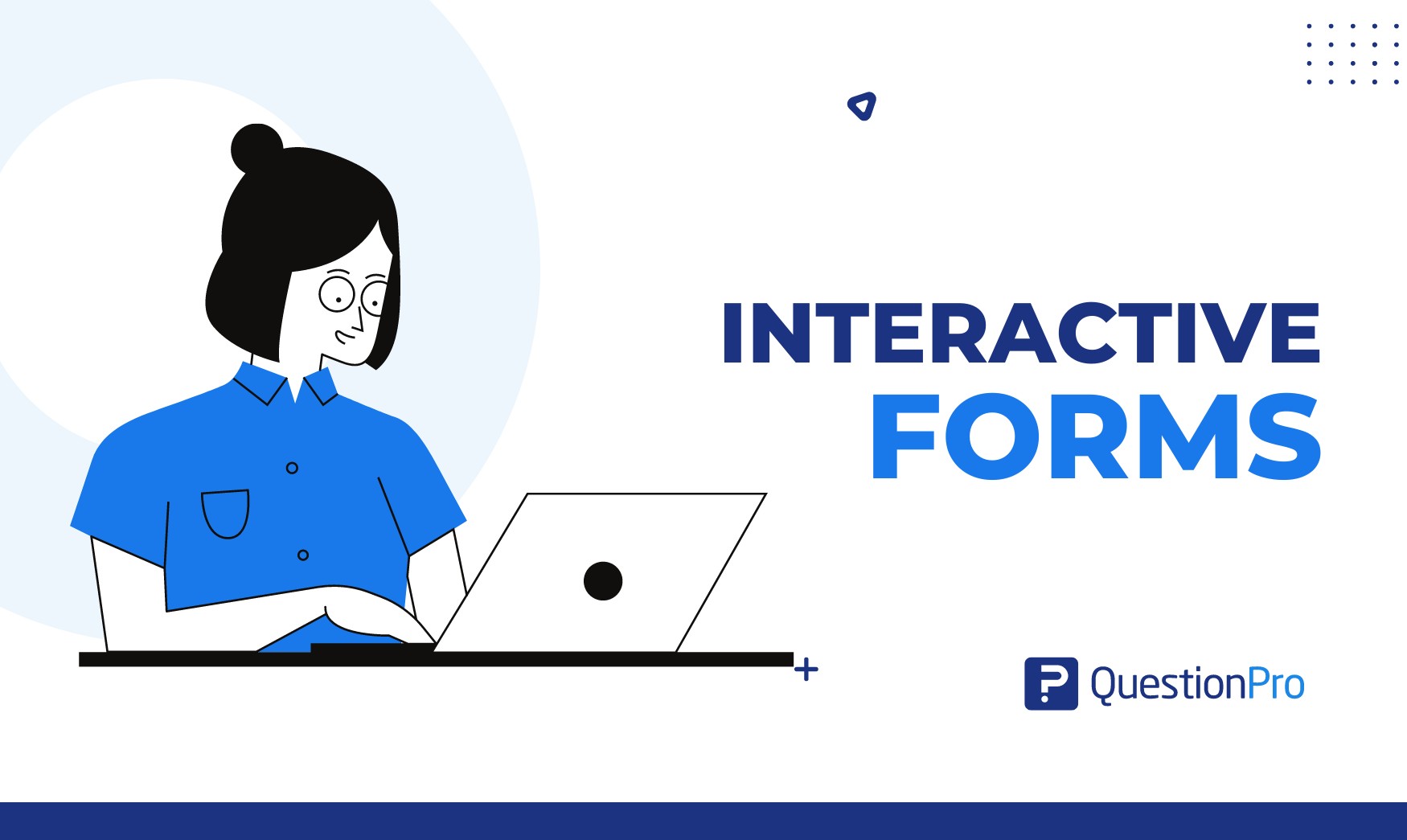
Interactive Forms: Key Features, Benefits, Uses + Design Tips
Sep 4, 2024
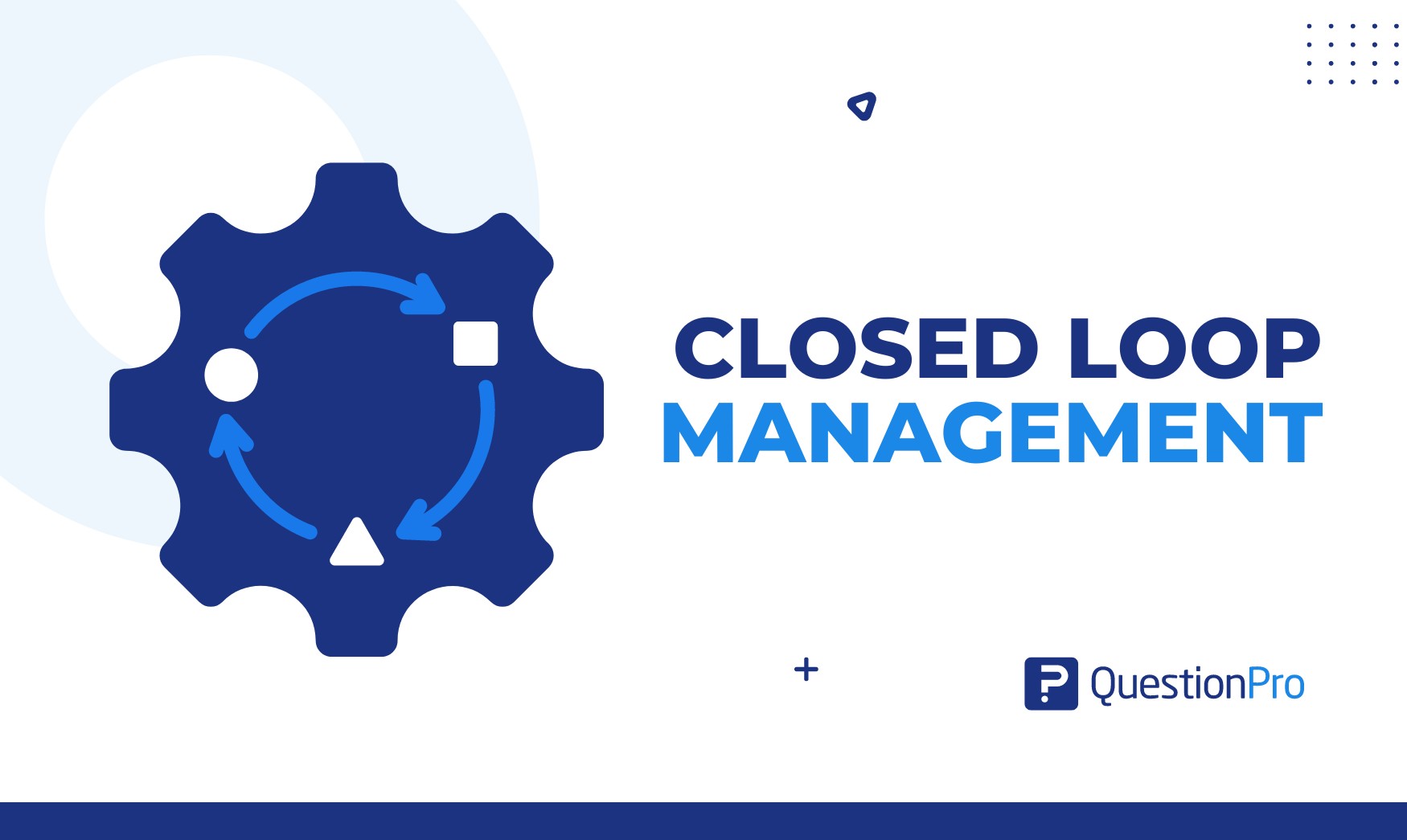
Closed-Loop Management: The Key to Customer Centricity
Sep 3, 2024
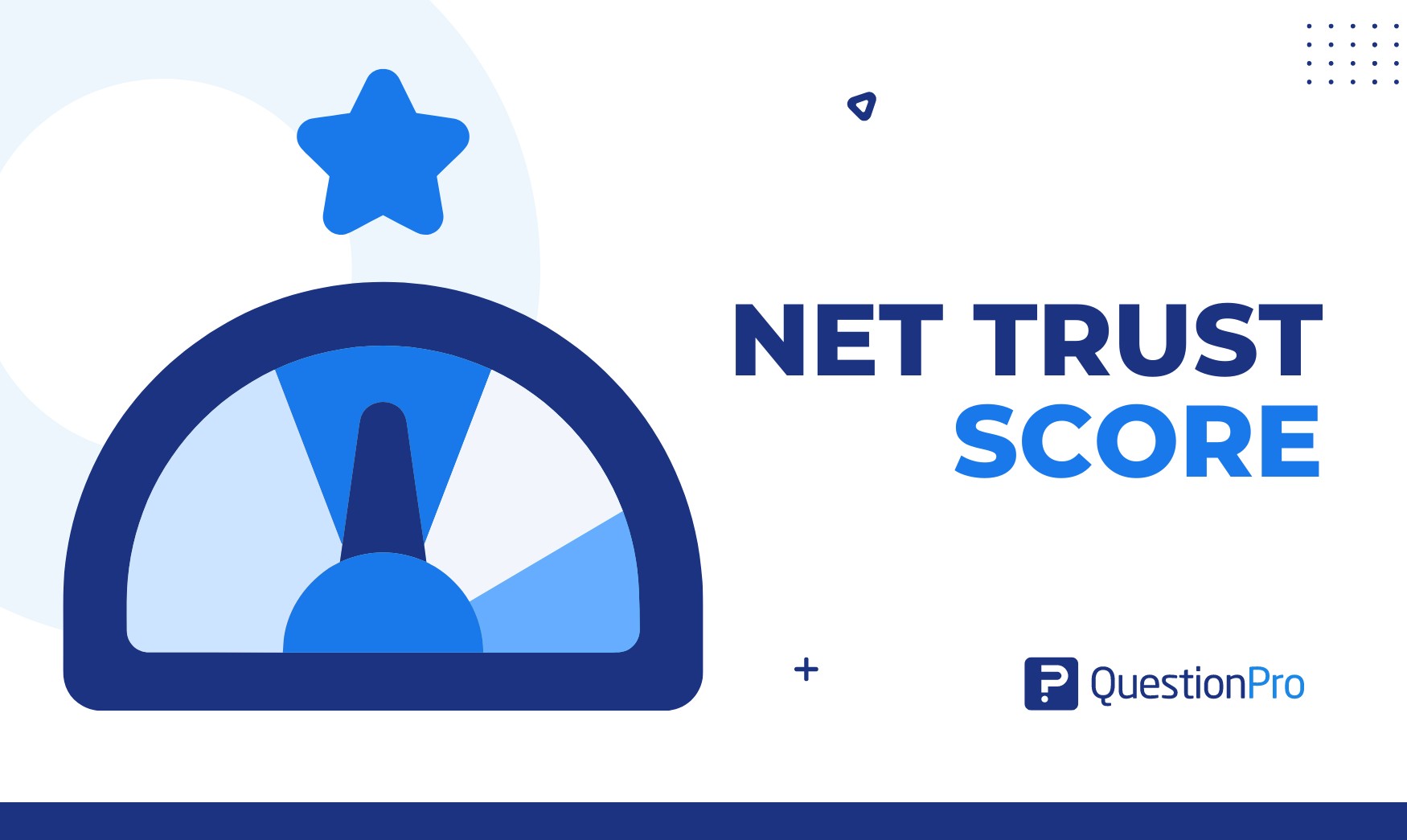
Net Trust Score: Tool for Measuring Trust in Organization
Sep 2, 2024
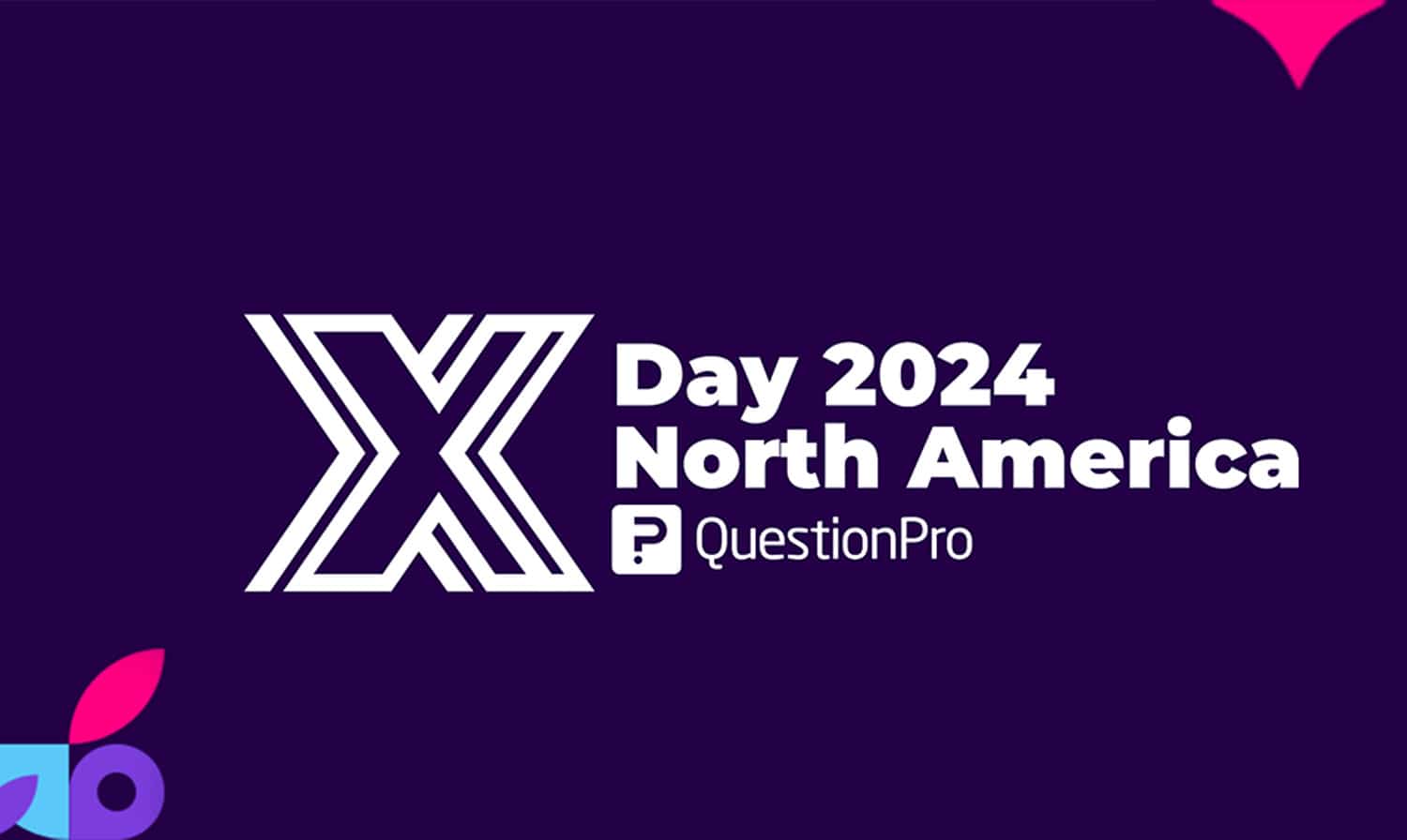
Why You Should Attend XDAY 2024
Aug 30, 2024
Other categories
- Academic Research
- Artificial Intelligence
- Assessments
- Brand Awareness
- Case Studies
- Communities
- Consumer Insights
- Customer effort score
- Customer Engagement
- Customer Experience
- Customer Loyalty
- Customer Research
- Customer Satisfaction
- Employee Benefits
- Employee Engagement
- Employee Retention
- Friday Five
- General Data Protection Regulation
- Insights Hub
- Life@QuestionPro
- Market Research
- Mobile diaries
- Mobile Surveys
- New Features
- Online Communities
- Question Types
- Questionnaire
- QuestionPro Products
- Release Notes
- Research Tools and Apps
- Revenue at Risk
- Survey Templates
- Training Tips
- Tuesday CX Thoughts (TCXT)
- Uncategorized
- What’s Coming Up
- Workforce Intelligence
What are Analytical Study Designs?
- Research Process
- Peer Review
Analytical study designs can be experimental or observational and each type has its own features. In this article, you'll learn the main types of designs and how to figure out which one you'll need for your study.
Updated on September 19, 2022

A study design is critical to your research study because it determines exactly how you will collect and analyze your data. If your study aims to study the relationship between two variables, then an analytical study design is the right choice.
But how do you know which type of analytical study design is best for your specific research question? It's necessary to have a clear plan before you begin data collection. Lots of researchers, sadly, speed through this or don't do it at all.
When are analytical study designs used?
A study design is a systematic plan, developed so you can carry out your research study effectively and efficiently. Having a design is important because it will determine the right methodologies for your study. Using the right study design makes your results more credible, valid, and coherent.
Descriptive vs. analytical studies
Study designs can be broadly divided into either descriptive or analytical.
Descriptive studies describe characteristics such as patterns or trends. They answer the questions of what, who, where, and when, and they generate hypotheses. They include case reports and qualitative studies.
Analytical study designs quantify a relationship between different variables. They answer the questions of why and how. They're used to test hypotheses and make predictions.
Experimental and observational
Analytical study designs can be either experimental or observational. In experimental studies, researchers manipulate something in a population of interest and examine its effects. These designs are used to establish a causal link between two variables.
In observational studies, in contrast, researchers observe the effects of a treatment or intervention without manipulating anything. Observational studies are most often used to study larger patterns over longer periods.
Experimental study designs
Experimental study designs are when a researcher introduces a change in one group and not in another. Typically, these are used when researchers are interested in the effects of this change on some outcome. It's important to try to ensure that both groups are equivalent at baseline to make sure that any differences that arise are from any introduced change.
In one study, Reiner and colleagues studied the effects of a mindfulness intervention on pain perception . The researchers randomly assigned participants into an experimental group that received a mindfulness training program for two weeks. The rest of the participants were placed in a control group that did not receive the intervention.
Experimental studies help us establish causality. This is critical in science because we want to know whether one variable leads to a change, or causes another. Establishing causality leads to higher internal validity and makes results reproducible.
Experimental designs include randomized control trials (RCTs), nonrandomized control trials (non-RCTs), and crossover designs. Read on to learn the differences.
Randomized control trials
In an RCT, one group of individuals receives an intervention or a treatment, while another does not. It's then possible to investigate what happens to the participants in each group.
Another important feature of RCTs is that participants are randomly assigned to study groups. This helps to limit certain biases and retain better control. Randomization also lets researchers pinpoint any differences in outcomes to the intervention received during the trial. RTCs are considered the gold standard in biomedical research and are considered to provide the best kind of evidence.
For example, one RCT looked at whether an exercise intervention impacts depression . Researchers randomly placed patients with depressive symptoms into intervention groups containing different types of exercise (i.e., light, moderate, or strong). Another group received usual medications or no exercise interventions.
Results showed that after the 12-week trial, patients in all exercise groups had decreased depression levels compared to the control group. This means that by using an RCT design, researchers can now safely assume that the exercise variable has a positive impact on depression.
However, RCTs are not without drawbacks. In the example above, we don't know if exercise still has a positive impact on depression in the long term. This is because it's not feasible to keep people under these controlled settings for a long time.
Advantages of RCTs
- It is possible to infer causality
- Everything is properly controlled, so very little is left to chance or bias
- Can be certain that any difference is coming from the intervention
Disadvantages of RCTs
- Expensive and can be time-consuming
- Can take years for results to be available
- Cannot be done for certain types of questions due to ethical reasons, such as asking participants to undergo harmful treatment
- Limited in how many participants researchers can adequately manage in one study or trial
- Not feasible for people to live under controlled conditions for a long time
Nonrandomized controlled trials
Nonrandomized controlled trials are a type of nonrandomized controlled studies (NRS) where the allocation of participants to intervention groups is not done randomly . Here, researchers purposely assign some participants to one group and others to another group based on certain features. Alternatively, participants can sometimes also decide which group they want to be in.
For example, in one study, clinicians were interested in the impact of stroke recovery after being in an enriched versus non-enriched hospital environment . Patients were selected for the trial if they fulfilled certain requirements common to stroke recovery. Then, the intervention group was given access to an enriched environment (i.e. internet access, reading, going outside), and another group was not. Results showed that the enriched group performed better on cognitive tasks.
NRS are useful in medical research because they help study phenomena that would be difficult to measure with an RCT. However, one of their major drawbacks is that we cannot be sure if the intervention leads to the outcome. In the above example, we can't say for certain whether those patients improved after stroke because they were in the enriched environment or whether there were other variables at play.
Advantages of NRS's
- Good option when randomized control trials are not feasible
- More flexible than RCTs
Disadvantages of NRS's
- Can't be sure if the groups have underlying differences
- Introduces risk of bias and confounds
Crossover study
In a crossover design, each participant receives a sequence of different treatments. Crossover designs can be applied to RCTs, in which each participant is randomly assigned to different study groups.
For example, one study looked at the effects of replacing butter with margarine on lipoproteins levels in individuals with cholesterol . Patients were randomly assigned to a 6-week butter diet, followed by a 6-week margarine diet. In between both diets, participants ate a normal diet for 5 weeks.
These designs are helpful because they reduce bias. In the example above, each participant completed both interventions, making them serve as their own control. However, we don't know if eating butter or margarine first leads to certain results in some subjects.
Advantages of crossover studies
- Each participant serves as their own control, reducing confounding variables
- Require fewer participants, so they have better statistical power
Disadvantages of crossover studies
- Susceptible to order effects, meaning the order in which a treatment was given may have an effect
- Carry-over effects between treatments
Observational studies
In observational studies, researchers watch (observe) the effects of a treatment or intervention without trying to change anything in the population. Observational studies help us establish broad trends and patterns in large-scale datasets or populations. They are also a great alternative when an experimental study is not an option.
Unlike experimental research, observational studies do not help us establish causality. This is because researchers do not actively control any variables. Rather, they investigate statistical relationships between them. Often this is done using a correlational approach.
For example, researchers would like to examine the effects of daily fiber intake on bone density . They conduct a large-scale survey of thousands of individuals to examine correlations of fiber intake with different health measures.
The main observational studies are case-control, cohort, and cross-sectional. Let's take a closer look at each one below.
Case-control study
A case-control is a type of observational design in which researchers identify individuals with an existing health situation (cases) and a similar group without the health issue (controls). The cases and the controls are then compared based on some measurements.
Frequently, data collection in a case-control study is retroactive (i.e., backwards in time). This is because participants have already been exposed to the event in question. Additionally, researchers must go through records and patient files to obtain the records for this study design.
For example, a group of researchers examined whether using sleeping pills puts people at risk of Alzheimer's disease . They selected 1976 individuals that received a dementia diagnosis (“cases”) with 7184 other individuals (“controls”). Cases and controls were matched on specific measures such as sex and age. Patient data was consulted to find out how much sleeping pills were consumed over the course of a certain time.
Case-control is ideal for situations where cases are easy to pick out and compare. For instance, in studying rare diseases or outbreaks.
Advantages of case-control studies
- Feasible for rare diseases
- Cheaper and easier to do than an RCT
Disadvantages of case-control studies
- Relies on patient records, which could be lost or damaged
- Potential recall and selection bias
Cohort study (longitudinal)
A cohort is a group of people who are linked in some way. For instance, a birth year cohort is all people born in a specific year. In cohort studies, researchers compare what happens to individuals in the cohort that have been exposed to some variable compared with those that haven't on different variables. They're also called longitudinal studies.
The cohort is then repeatedly assessed on variables of interest over a period of time. There is no set amount of time required for cohort studies. They can range from a few weeks to many years.
Cohort studies can be prospective. In this case, individuals are followed for some time into the future. They can also be retrospective, where data is collected on a cohort from records.
One of the longest cohort studies today is The Harvard Study of Adult Development . This cohort study has been tracking various health outcomes of 268 Harvard graduates and 456 poor individuals in Boston from 1939 to 2014. Physical screenings, blood samples, brain scans and surveys were collected on this cohort for over 70 years. This study has produced a wealth of knowledge on outcomes throughout life.
A cohort study design is a good option when you have a specific group of people you want to study over time. However, a major drawback is that they take a long time and lack control.
Advantages of cohort studies
- Ethically safe
- Allows you to study multiple outcome variables
- Establish trends and patterns
Disadvantages of cohort studies
- Time consuming and expensive
- Can take many years for results to be revealed
- Too many variables to manage
- Depending on length of study, can have many changes in research personnel
Cross-sectional study
Cross-sectional studies are also known as prevalence studies. They look at the relationship of specific variables in a population in one given time. In cross-sectional studies, the researcher does not try to manipulate any of the variables, just study them using statistical analyses. Cross-sectional studies are also called snapshots of a certain variable or time.
For example, researchers wanted to determine the prevalence of inappropriate antibiotic use to study the growing concern about antibiotic resistance. Participants completed a self-administered questionnaire assessing their knowledge and attitude toward antibiotic use. Then, researchers performed statistical analyses on their responses to determine the relationship between the variables.
Cross-sectional study designs are ideal when gathering initial data on a research question. This data can then be analyzed again later. By knowing the public's general attitudes towards antibiotics, this information can then be relayed to physicians or public health authorities. However, it's often difficult to determine how long these results stay true for.
Advantages of cross-sectional studies
- Fast and inexpensive
- Provides a great deal of information for a given time point
- Leaves room for secondary analysis
Disadvantages of cross-sectional studies
- Requires a large sample to be accurate
- Not clear how long results remain true for
- Do not provide information on causality
- Cannot be used to establish long-term trends because data is only for a given time
So, how about your next study?
Whether it's an RCT, a case-control, or even a qualitative study, AJE has services to help you at every step of the publication process. Get expert guidance and publish your work for the world to see.

The AJE Team
See our "Privacy Policy"
Overview of Analytic Studies
Introduction
We search for the determinants of health outcomes, first, by relying on descriptive epidemiology to generate hypotheses about associations between exposures and outcomes. Analytic studies are then undertaken to test specific hypotheses. Samples of subjects are identified and information about exposure status and outcome is collected. The essence of an analytic study is that groups of subjects are compared in order to estimate the magnitude of association between exposures and outcomes.
In their book entitled "Epidemiology Matters" Katherine Keyes and Sandro Galea discuss three fundamental options for studying samples from a population as illustrated in the video below (duration 8:30).
Learning Objectives
After successfully completing this section, the student will be able to:
- Describe the difference between descriptive and scientific/analytic epidemiologic studies in terms of information/evidence provided for medicine and public health.
- Define and explain the distinguishing features of a cohort study.
- Describe and identify the types of epidemiologic questions that can be addressed by cohort studies.
- Define and distinguish among prospective and retrospective cohort studies using the investigator as the point of reference.
- Define and explain the distinguishing features of a case-control study.
- Explain the distinguishing features of an intervention study.
- Identify the study design when reading an article or abstract.
Cohort Type Studies
A cohort is a "group." In epidemiology a cohort is a group of individuals who are followed over a period of time, primarily to assess what happens to them, i.e., their health outcomes. In cohort type studies one identifies individuals who do not have the outcome of interest initially, and groups them in subsets that differ in their exposure to some factor, e.g., smokers and non-smokers. The different exposure groups are then followed over time in order to compare the incidence of health outcomes, such as lung cancer or heart disease. As an example, the Framingham Heart Study enrolled a cohort of 5,209 residents of Framingham, MA who were between the ages of 30-62 and who did not have cardiovascular disease when they were enrolled. These subjects differed from one another in many ways: whether they smoked, how much they smoked, body mass index, eating habits, exercise habits, gender, family history of heart disease, etc. The researchers assessed these and many other characteristics or "exposures" soon after the subjects had been enrolled and before any of them had developed cardiovascular disease. The many "baseline characteristics" were assessed in a number of ways including questionnaires, physical exams, laboratory tests, and imaging studies (e.g., x-rays). They then began "following" the cohort, meaning that they kept in contact with the subjects by phone, mail, or clinic visits in order to determine if and when any of the subjects developed any of the "outcomes of interest," such as myocardial infarction (heart attack), angina, congestive heart failure, stroke, diabetes and many other cardiovascular outcomes.
Over time some subjects eventually began to develop some of the outcomes of interest. Having followed the cohort in this fashion, it was eventually possible to use the information collected to evaluate many hypotheses about what characteristics were associated with an increased risk of heart disease. For example, if one hypothesized that smoking increased the risk of heart attacks, the subjects in the cohort could be sorted based on their smoking habits, and one could compare the subset of the cohort that smoked to the subset who had never smoked. For each such comparison that one wanted to make the cohort could be grouped according to whether they had a given exposure or not, and one could measure and compare the frequency of heart attacks (i.e., the incidence) between the groups. Incidence provides an estimate of risk, so if the incidence of heart attacks is 3 times greater in smokers compared to non-smokers, it suggests an association between smoking and risk of developing a heart attack. (Various biases might also be an explanation for an apparent association. We will learn about these later in the course.) The hallmark of analytical studies, then, is that they collect information about both exposure status and outcome status, and they compare groups to identify whether there appears to be an association or a link.
The Population "At Risk"
From the discussion above, it should be obvious that one of the basic requirements of a cohort type study is that none of the subjects have the outcome of interest at the beginning of the follow-up period, and time must pass in order to determine the frequency of developing the outcome.
- For example, if one wanted to compare the risk of developing uterine cancer between postmenopausal women receiving hormone-replacement therapy and those not receiving hormones, one would consider certain eligibility criteria for the members prior to the start of the study: 1) they should be female, 2) they should be post-menopausal, and 3) they should have a uterus. Among post-menopausal women there might be a number who had had a hysterectomy already, perhaps for persistent bleeding problems or endometriosis. Since these women no longer have a uterus, one would want to exclude them from the cohort, because they are no longer at risk of developing this particular type of cancer.
- Similarly, if one wanted to compare the risk of developing diabetes among nursing home residents who exercised and those who did not, it would be important to test the subjects for diabetes at the beginning of the follow-up period in order to exclude all subjects who already had diabetes and therefore were not "at risk" of developing diabetes.
Eligible subjects have to meet certain criteria to be included as subjects in a study (inclusion criteria). One of these would be that they did not have any of the diseases or conditions that the investigators want to study, i.e., the subjects must be "at risk," of developing the outcome of interest, and the members of the cohort to be followed are sometimes referred to as "the population at risk."
However, at times decisions about who is "at risk" and eligible get complicated.
Example #1: Suppose the outcome of interest is development of measles. There may be subjects who:
- Already were known to have had clinically apparent measles and are immune to subsequent measles infection
- Had sub-clinical cases of measles that went undetected (but the subject may still be immune)
- Had a measles vaccination that conferred immunity
- Had a measles vaccination that failed to confer immunity
In this case the eligibility criteria would be shaped by the specific scientific questions being asked. One might want to compare subjects known to have had clinically apparent measles to those who had not had clinical measles and had not had a measles vaccination. Or, one could take blood sample from all potential subjects in order to measure their antibody titers (levels) to the measles virus.
Example #2: Suppose you are studying an event that can occur more that once, such as a heart attack. Again, the eligibility criteria should be shaped to fit the scientific questions that are being answered. If one were interested in the risk of a first myocardial infarction, then obviously subjects who had already had a heart attack would not be eligible for study. On the other hand, if one were interested in tertiary prevention of heart attacks, the study cohort would include people who had had heart attacks or other clinical manifestations of heart disease, and the outcome of interest would be subsequent significant cardiac events or death.
Prospective and Retrospective Cohort Studies
Cohort studies can be classified as prospective or retrospective based on when outcomes occurred in relation to the enrollment of the cohort.
Prospective Cohort Studies

In a prospective study like the Nurses Health Study baseline information is collected from all subjects in the same way using exactly the same questions and data collection methods for all subjects. The investigators design the questions and data collection procedures carefully in order to obtain accurate information about exposures before disease develops in any of the subjects. After baseline information is collected, subjects in a prospective cohort study are then followed "longitudinally," i.e. over a period of time, usually for years, to determine if and when they become diseased and whether their exposure status changes. In this way, investigators can eventually use the data to answer many questions about the associations between "risk factors" and disease outcomes. For example, one could identify smokers and non-smokers at baseline and compare their subsequent incidence of developing heart disease. Alternatively, one could group subjects based on their body mass index (BMI) and compare their risk of developing heart disease or cancer.
Key Concept: The distinguishing feature of a prospective cohort study is that at the time that the investigators begin enrolling subjects and collecting baseline exposure information, none of the subjects has developed any of the outcomes of interest.
|
Examples of Prospective Cohort Studies
- The Framingham Heart Study Home Page
- The Nurses Health Study Home Page

Pitfall: Note that in these prospective cohort studies a comparison of incidence between the groups can only take place after enough time has elapsed so that some subjects developed the outcomes of interest. Since the data analysis occurs after some outcomes have occurred, some students mistakenly would call this a retrospective study, but this is incorrect. The analysis always occurs after a certain number of events have taken place. The characteristic that distinguishes a study as prospective is that the subjects were enrolled, and baseline data was collected before any subjects developed an outcome of interest.
Retrospective Cohort Studies
In contrast, retrospective studies are conceived after some people have already developed the outcomes of interest. The investigators jump back in time to identify a cohort of individuals at a point in time before they have developed the outcomes of interest, and they try to establish their exposure status at that point in time. They then determine whether the subject subsequently developed the outcome of interest.

Suppose investigators wanted to test the hypothesis that working with the chemicals involved in tire manufacturing increases the risk of death. Since this is a fairly rare exposure, it would be advantageous to use a special exposure cohort such as employees of a large tire manufacturing factory. The employees who actually worked with chemicals used in the manufacturing process would be the exposed group, while clerical workers and management might constitute the "unexposed" group. However, rather than following these subjects for decades, it would be more efficient to use employee health and employment records over the past two or three decades as a source of data. In essence, the investigators are jumping back in time to identify the study cohort at a point in time before the outcome of interest (death) occurred. They can classify them as "exposed" or "unexposed" based on their employment records, and they can use a number of sources to determine subsequent outcome status, such as death (e.g., using health records, next of kin, National Death Index, etc.).
Key Concept: The distinguishing feature of a retrospective cohort study is that the investigators conceive the study and begin identifying and enrolling subjects . |
Retrospective cohort studies like the one described above are very efficient for studying rare or unusual exposures, but there are many potential problems here. Sometimes exposure status is not clear when it is necessary to go back in time and use whatever data is available, especially because the data being used was not designed to answer a health question. Even if it was clear who was exposed to tire manufacturing chemicals based on employee records, it would also be important to take into account (or adjust for) other differences that could have influenced mortality, i.e., confounding factors. For example, it might be important to know whether the subjects smoked, or drank, or what kind of diet they ate. However, it is unlikely that a retrospective cohort study would have accurate information on these many other risk factors.
The video below provides a brief (7:31) explanation of the distinction between retrospective and prospective cohort studies.
Link to a transcript of the video
Intervention Studies (Clinical Trials)
Intervention studies (clinical trials) are experimental research studies that compare the effectiveness of medical treatments, management strategies, prevention strategies, and other medical or public health interventions. Their design is very similar to that of a prospective cohort study. However, in cohort studies exposure status is determined by genetics, self-selection, or life circumstances, and the investigators just observe differences in outcome between those who have a given exposure and those who do not. In clinical trials exposure status (the treatment type) is assigned by the investigators . Ideally, assignment of subjects to one of the comparison groups should be done randomly in order to produce equal distributions of potentially confounding factors. Sometimes a group receiving a new treatment is compared to an untreated group, or a group receiving a placebo or a sham treatment. Sometimes, a new treatment is compared to an untreated group or to a group receiving an established treatment. For more on this topic see the module on Intervention Studies.
In summary, the characteristic that distinguishes a clinical trial from a cohort study is that the investigator assigns the exposure status in a clinical trial, while subjects' genetics, behaviors, and life circumstances determine their exposures in a cohort study.
Key Concept: Common features of both prospective and retrospective cohort studies.
|
Summarizing Data in a Cohort Study
Investigators often use contingency tables to summarize data. In essence, the table is a matrix that displays the combinations of exposure and outcome status. If one were summarizing the results of a study with two possible exposure categories and two possible outcomes, one would use a "two by two" table in which the numbers in the four cells indicate the number of subjects within each of the 4 possible categories of risk and disease status.
For example, consider data from a retrospective cohort study conducted by the Massachusetts Department of Public Health (MDPH) during an investigation of an outbreak of Giardia lamblia in Milton, MA in 2003. The descriptive epidemiology indicated that almost all of the cases belonged to a country club in Milton. The club had an adult swimming pool and a wading pool for toddlers, and the investigators suspected that the outbreak may have occurred when an infected child with a dirty diaper contaminated the water in the kiddy pool. This hypothesis was tested by conducting a retrospective cohort study. The cases of Giardia lamblia had already occurred and had been reported to MDPH via the infectious disease surveillance system (for more information on surveillance, see the Surveillance module). The investigation focused on an obvious cohort - 479 members of the country club who agreed to answer the MDPH questionnaire. The questionnaire asked, among many other things, whether the subject had been exposed to the kiddy pool. The incidence of subsequent Giardia infection was then compared between subjects who been exposed to the kiddy pool and those who had not.
The table below summarizes the findings. A total of 479 subjects completed the questionnaire, and 124 of them indicated that they had been exposed to the kiddy pool. Of these, 16 subsequently developed Giardia infection, but 108 did not. Among the 355 subjects who denied kiddy pool exposure, 14 developed Giardia infection, and the other 341 did not.
|
|
|
|
|
| 16 | 108 | 124 | 16/124 = 12.9% |
| 14 | 341 | 365 | 14/365 = 3,9% |
Organization of the data this way makes it easier to compute the cumulative incidence in each group (12.9% and 3.9% respectively). The incidence in each group provides an estimate of risk, and the groups can be compared in order to estimate the magnitude of association. (This will be addressed in much greater detail in the module on Measures of Association.) One way of quantifying the association is to calculate the relative risk, i.e., dividing the incidence in the exposed group by the incidence in the unexposed group). In this case, the risk ratio is (12.9% / 3.9%) = 3.3. This suggest that subjects who swam in the kiddy pool had 3.3 times the risk of getting Giardia infections compared to those who did not, suggesting that the kiddy pool was the source.
Unanswered Questions
If the kiddy pool was the source of contamination responsible for this outbreak, why was it that:
- Only 16 people exposed to the kiddy pool developed the infection?
- There were 14 Giardia cases among people who denied exposure to the kiddy pool?
Before you look at the answer, think about it and try to come up with a possible explanation.
Likely Explanation
Optional Links of Potential Interest
Link to the 2003 Giardia outbreak
Link to CDC page on Organizing Data

Possible Pitfall: Contingency tables can be oriented in several ways, and this can cause confusion when calculating measures of association.
There is no standard rule about how to set up contingency tables, and you will see them set up in different ways.
- With exposure status in rows and outcome status in columns
- With exposure status in columns and outcome status in rows
- With exposed group first followed by non-exposed group
- With non-exposed group first followed by exposed group
If you aren't careful, these different orientations can result in errors in calculating measures of association. One way to avoid confusion is to always set up your contingency tables in the same way. For example, in these learning modules the contingency tables almost always indicate outcome status in columns listing subjects who have the outcome of interest to the left of subjects who do not have the outcome, and exposure status of the exposed (or most exposed) group is listed in a row above those who are unexposed (or have less exposure).
The table below illustrates this arrangement.
| Those With the Outcome | Those Without the Outcome | Total |
Exposed (or most exposed) |
|
|
|
Non-exposed (or least exposed) |
|
|
|
Case-Control Studies
Cohort studies have an intuitive logic to them, but they can be very problematic when:
- The outcomes being investigated are rare;
- There is a long time period between the exposure of interest and the development of the disease; or
- It is expensive or very difficult to obtain exposure information from a cohort.
In the first case, the rarity of the disease requires enrollment of very large numbers of people. In the second case, the long period of follow-up requires efforts to keep contact with and collect outcome information from individuals. In all three situations, cost and feasibility become an important concern.
A case-control design offers an alternative that is much more efficient. The goal of a case-control study is the same as that of cohort studies, i.e. to estimate the magnitude of association between an exposure and an outcome. However, case-control studies employ a different sampling strategy that gives them greater efficiency. As with a cohort study, a case-control study attempts to identify all people who have developed the disease of interest in the defined population. This is not because they are inherently more important to estimating an association, but because they are almost always rarer than non-diseased individuals, and one of the requirements of accurate estimation of the association is that there are reasonable numbers of people in both the numerators (cases) and denominators (people or person-time) in the measures of disease frequency for both exposed and reference groups. However, because most of the denominator is made up of people who do not develop disease, the case-control design avoids the need to collect information on the entire population by selecting a sample of the underlying population.
Rothman describes the case-control strategy as follows:
"Case-control studies are best understood by considering as the starting point a , which represents a hypothetical study population in which a cohort study might have been conducted. The is the population that gives rise to the cases included in the study. If a cohort study were undertaken, we would define the exposed and unexposed cohorts (or several cohorts) and from these populations obtain denominators for the incidence rates or risks that would be calculated for each cohort. We would then identify the number of cases occurring in each cohort and calculate the risk or incidence rate for each. In a case-control study the same cases are identified and classified as to whether they belong to the exposed or unexposed cohort. Instead of obtaining the denominators for the rates or risks, however, a control group is sampled from the entire source population that gives rise to the cases. Individuals in the control group are then classified into exposed and unexposed categories. The purpose of the control group is to determine the relative size of the exposed and unexposed components of the source population." |
To illustrate this consider the following hypothetical scenario in which the source population is Plymouth County in Massachusetts, which has a total population of 6,647 (hypothetical). Thirteen people in the county have been diagnosed with an unusual disease and seven of them have a particular exposure that is suspected of being an important contributing factor. The chief problem here is that the disease is quite rare.

If I somehow had exposure and outcome information on all of the subjects in the source population and looked at the association using a cohort design, it might look like this:
| Diseased | Non-diseased | Total |
---|---|---|---|
Exposed | 7 | 1,000 | 1,007 |
Non-exposed | 6 | 5,634 | 5,640 |
Therefore, the incidence in the exposed individuals would be 7/1,007 = 0.70%, and the incidence in the non-exposed individuals would be 6/5,640 = 0.11%. Consequently, the risk ratio would be 0.70/0.11=6.52, suggesting that those who had the risk factor (exposure) had 6.5 times the risk of getting the disease compared to those without the risk factor. This is a strong association.
In this hypothetical example, I had data on all 6,647 people in the source population, and I could compute the probability of disease (i.e., the risk or incidence) in both the exposed group and the non-exposed group, because I had the denominators for both the exposed and non-exposed groups.
The problem , of course, is that I usually don't have the resources to get the data on all subjects in the population. If I took a random sample of even 5-10% of the population, I might not have any diseased people in my sample.
An alternative approach would be to use surveillance databases or administrative databases to find most or all 13 of the cases in the source population and determine their exposure status. However, instead of enrolling all of the other 5,634 residents, suppose I were to just take a sample of the non-diseased population. In fact, suppose I only took a sample of 1% of the non-diseased people and I then determined their exposure status. The data might look something like this:
| Diseased | Non-diseased | Total |
---|---|---|---|
Exposed | 7 | 10 | unknown |
Non-exposed | 6 | 56 | unknown |
With this sampling approach I can no longer compute the probability of disease in each exposure group, because I no longer have the denominators in the last column. In other words, I don't know the exposure distribution for the entire source population. However, the small control sample of non-diseased subjects gives me a way to estimate the exposure distribution in the source population. So, I can't compute the probability of disease in each exposure group, but I can compute the odds of disease in the case-control sample.
The Odds Ratio
The odds of disease among the exposed sample are 7/10, and the odds of disease in the non-exposed sample are 6/56. If I compute the odds ratio, I get (7/10) / (5/56) = 6.56, very close to the risk ratio that I computed from data for the entire population. We will consider odds ratios and case-control studies in much greater depth in a later module. However, for the time being the key things to remember are that:
- The sampling strategy for a case-control study is very different from that of cohort studies, despite the fact that both have the goal of estimating the magnitude of association between the exposure and the outcome.
- In a case-control study there is no "follow-up" period. One starts by identifying diseased subjects and determines their exposure distribution; one then takes a sample of the source population that produced those cases in order to estimate the exposure distribution in the overall source population that produced the cases. [In cohort studies none of the subjects have the outcome at the beginning of the follow-up period.]
- In a case-control study, you cannot measure incidence, because you start with diseased people and non-diseased people, so you cannot calculate relative risk.
- The case-control design is very efficient. In the example above the case-control study of only 79 subjects produced an odds ratio (6.56) that was a very close approximation to the risk ratio (6.52) that was obtained from the data in the entire population.
- Case-control studies are particularly useful when the outcome is rare is uncommon in both exposed and non-exposed people.
The Difference Between "Probability" and "Odds"?

- The odds are defined as the probability that the event will occur divided by the probability that the event will not occur.
If the probability of an event occurring is Y, then the probability of the event not occurring is 1-Y. (Example: If the probability of an event is 0.80 (80%), then the probability that the event will not occur is 1-0.80 = 0.20, or 20%.
The odds of an event represent the ratio of the (probability that the event will occur) / (probability that the event will not occur). This could be expressed as follows:
Odds of event = Y / (1-Y)
So, in this example, if the probability of the event occurring = 0.80, then the odds are 0.80 / (1-0.80) = 0.80/0.20 = 4 (i.e., 4 to 1).
- If a race horse runs 100 races and wins 25 times and loses the other 75 times, the probability of winning is 25/100 = 0.25 or 25%, but the odds of the horse winning are 25/75 = 0.333 or 1 win to 3 loses.
- If the horse runs 100 races and wins 5 and loses the other 95 times, the probability of winning is 0.05 or 5%, and the odds of the horse winning are 5/95 = 0.0526.
- If the horse runs 100 races and wins 50, the probability of winning is 50/100 = 0.50 or 50%, and the odds of winning are 50/50 = 1 (even odds).
- If the horse runs 100 races and wins 80, the probability of winning is 80/100 = 0.80 or 80%, and the odds of winning are 80/20 = 4 to 1.
NOTE that when the probability is low, the odds and the probability are very similar.
On Sept. 8, 2011 the New York Times ran an article on the economy in which the writer began by saying "If history is a guide, the odds that the American economy is falling into a double-dip recession have risen sharply in recent weeks and may even have reached 50 percent." Further down in the article the author quoted the economist who had been interviewed for the story. What the economist had actually said was, "Whether we reach the technical definition [of a double-dip recession] I think is probably close to 50-50."
Question: Was the author correct in saying that the "odds" of a double-dip recession may have reached 50 percent?
Key Concept: In a study that is designed and conducted as a case-control study, you cannot calculate incidence. Therefore, you cannot calculate risk ratio or risk difference. You can only calculate an odds ratio. However, in certain situations a case-control study is the only feasible study design. |
Which Study Design Is Best?
Decisions regarding which study design to use rest on a number of factors including::
- Uncommon Outcome: If the outcome of interest is uncommon or rare, a case-control study would usually be best.
- Uncommon Exposure: When studying an uncommon exposure, the investigators need to enroll an adequate number of subjects who have that exposure. In this situation a cohort study is best.
- Ethics of Assigning Subjects to an Exposure: If you wanted to study the association between smoking and lung cancer, It wouldn't be ethical to conduct a clinical trial in which you randomly assigned half of the subjects to smoking.
- Resources: If you have limited time, money, and personnel to gather data, it is unlikely that you will be able to conduct a prospective cohort study. A case-control study or a retrospective cohort study would be better options. The best one to choose would be dictated by whether the outcome was rare or the exposure of interest was rare.
There are some situations in which more than one study design could be used.
Smoking and Lung Cancer: For example, when investigators first sought to establish whether there was a link between smoking and lung cancer, they did a study by finding hospital subjects who had lung cancer and a comparison group of hospital patients who had diseases other than cancer. They then compared the prior exposure histories with respect to smoking and many other factors. They found that past smoking was much more common in the lung cancer cases, and they concluded that there was an association. The advantages to this approach were that they were able to collect the data they wanted relatively quickly and inexpensively, because they started with people who already had the disease of interest.
The short video below provides a nice overview of epidemiological studies.

However, there were several limitations to the study they had done. The study design did not allow them to measure the incidence of lung cancer in smokers and non-smokers, so they couldn't measure the absolute risk of smoking. They also didn't know what other diseases smoking might be associated with, and, finally, they were concerned about some of the biases that can creep into this type of study.
As a result, these investigators then initiated another study. They invited all of the male physicians in the United Kingdom to fill out questionnaires regarding their health status and their smoking status. They then focused on the healthy physicians who were willing to participate, and the investigators mailed follow-up questionnaires to them every few years. They also had a way of finding out the cause of death for any subjects who became ill and died. The study continued for about 50 years. Along the way the investigators periodically compared the incidence of death among non-smoking physicians and physicians who smoked small, moderate or heavy amounts of tobacco.
These studies were useful, because they were able to demonstrate that smokers had an increased risk of over 20 different causes of death. They were also able to measure the incidence of death in different categories, so they knew the absolute risk for each cause of death. Of course, the downside to this approach was that it took a long time, and it was very costly. So, both a case-control study and a prospective cohort study provided useful information about the association between smoking and lung cancer and other diseases, but there were distinct advantages and limitations to each approach.
Hepatitis Outbreak in Marshfield, MA
In 2004 there was an outbreak of hepatitis A on the South Shore of Massachusetts. Over a period of a few weeks there were 20 cases of hepatitis A that were reported to the MDPH, and most of the infected persons were residents of Marshfield, MA. Marshfield's health department requested help in identifying the source from MDPH. The investigators quickly performed descriptive epidemiology. The epidemic curve indicated a point source epidemic, and most of the cases lived in the Marshfield area, although some lived as far away as Boston. They conducted hypothesis-generating interviews, and taken together, the descriptive epidemiology suggested that the source was one of five or six food establishments in the Marshfield area, but it wasn't clear which one. Consequently, the investigators wanted to conduct an analytic study to determine which restaurant was the source. Which study design should have been conducted? Think about the scenario, and then open the "Quiz Me" below and choose your answer.
Link to more on the hepatitis outbreak
Case-control studies are particularly efficient for rare diseases because they begin by identifying a sufficient number of diseased people (or people have some "outcome" of interest) to enable you to do an analysis that tests associations. Case-control studies can be done in just about any circumstance, but they are particularly useful when you are dealing with rare diseases or disease for which there is a very long latent period, i.e. a long time between the causative exposure and the eventual development of disease.
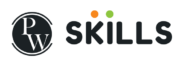
Data Analysis Techniques in Research – Methods, Tools & Examples
Varun Saharawat is a seasoned professional in the fields of SEO and content writing. With a profound knowledge of the intricate aspects of these disciplines, Varun has established himself as a valuable asset in the world of digital marketing and online content creation.
Data analysis techniques in research are essential because they allow researchers to derive meaningful insights from data sets to support their hypotheses or research objectives.
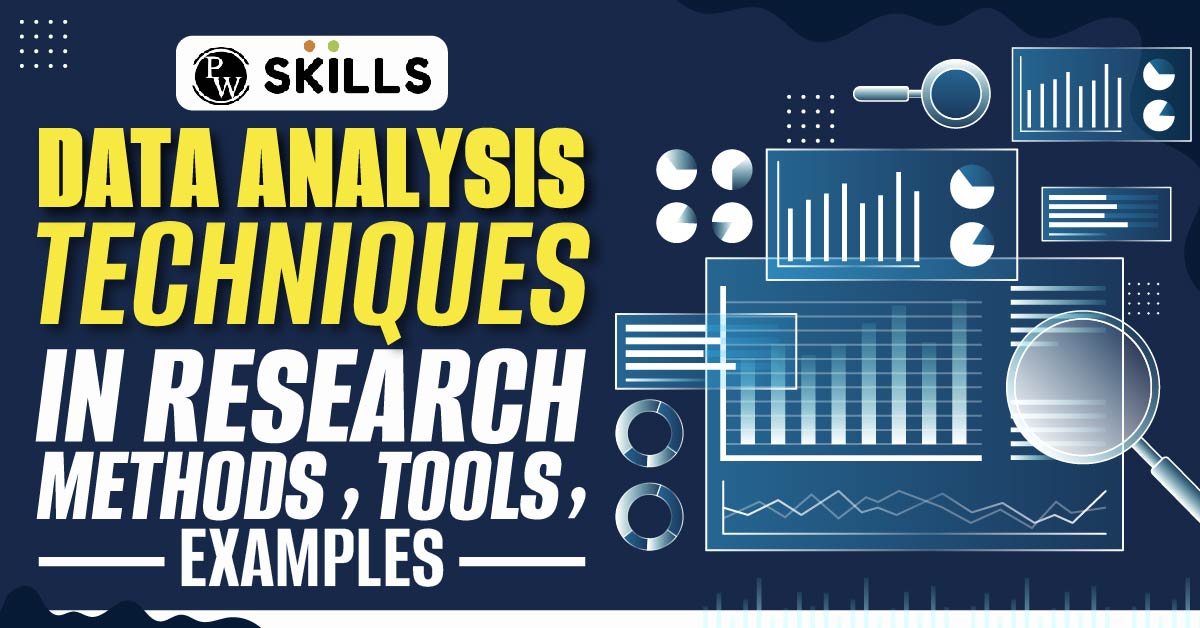
Data Analysis Techniques in Research : While various groups, institutions, and professionals may have diverse approaches to data analysis, a universal definition captures its essence. Data analysis involves refining, transforming, and interpreting raw data to derive actionable insights that guide informed decision-making for businesses.
A straightforward illustration of data analysis emerges when we make everyday decisions, basing our choices on past experiences or predictions of potential outcomes.
If you want to learn more about this topic and acquire valuable skills that will set you apart in today’s data-driven world, we highly recommend enrolling in the Data Analytics Course by Physics Wallah . And as a special offer for our readers, use the coupon code “READER” to get a discount on this course.
Table of Contents
What is Data Analysis?
Data analysis is the systematic process of inspecting, cleaning, transforming, and interpreting data with the objective of discovering valuable insights and drawing meaningful conclusions. This process involves several steps:
- Inspecting : Initial examination of data to understand its structure, quality, and completeness.
- Cleaning : Removing errors, inconsistencies, or irrelevant information to ensure accurate analysis.
- Transforming : Converting data into a format suitable for analysis, such as normalization or aggregation.
- Interpreting : Analyzing the transformed data to identify patterns, trends, and relationships.
Types of Data Analysis Techniques in Research
Data analysis techniques in research are categorized into qualitative and quantitative methods, each with its specific approaches and tools. These techniques are instrumental in extracting meaningful insights, patterns, and relationships from data to support informed decision-making, validate hypotheses, and derive actionable recommendations. Below is an in-depth exploration of the various types of data analysis techniques commonly employed in research:
1) Qualitative Analysis:
Definition: Qualitative analysis focuses on understanding non-numerical data, such as opinions, concepts, or experiences, to derive insights into human behavior, attitudes, and perceptions.
- Content Analysis: Examines textual data, such as interview transcripts, articles, or open-ended survey responses, to identify themes, patterns, or trends.
- Narrative Analysis: Analyzes personal stories or narratives to understand individuals’ experiences, emotions, or perspectives.
- Ethnographic Studies: Involves observing and analyzing cultural practices, behaviors, and norms within specific communities or settings.
2) Quantitative Analysis:
Quantitative analysis emphasizes numerical data and employs statistical methods to explore relationships, patterns, and trends. It encompasses several approaches:
Descriptive Analysis:
- Frequency Distribution: Represents the number of occurrences of distinct values within a dataset.
- Central Tendency: Measures such as mean, median, and mode provide insights into the central values of a dataset.
- Dispersion: Techniques like variance and standard deviation indicate the spread or variability of data.
Diagnostic Analysis:
- Regression Analysis: Assesses the relationship between dependent and independent variables, enabling prediction or understanding causality.
- ANOVA (Analysis of Variance): Examines differences between groups to identify significant variations or effects.
Predictive Analysis:
- Time Series Forecasting: Uses historical data points to predict future trends or outcomes.
- Machine Learning Algorithms: Techniques like decision trees, random forests, and neural networks predict outcomes based on patterns in data.
Prescriptive Analysis:
- Optimization Models: Utilizes linear programming, integer programming, or other optimization techniques to identify the best solutions or strategies.
- Simulation: Mimics real-world scenarios to evaluate various strategies or decisions and determine optimal outcomes.
Specific Techniques:
- Monte Carlo Simulation: Models probabilistic outcomes to assess risk and uncertainty.
- Factor Analysis: Reduces the dimensionality of data by identifying underlying factors or components.
- Cohort Analysis: Studies specific groups or cohorts over time to understand trends, behaviors, or patterns within these groups.
- Cluster Analysis: Classifies objects or individuals into homogeneous groups or clusters based on similarities or attributes.
- Sentiment Analysis: Uses natural language processing and machine learning techniques to determine sentiment, emotions, or opinions from textual data.
Also Read: AI and Predictive Analytics: Examples, Tools, Uses, Ai Vs Predictive Analytics
Data Analysis Techniques in Research Examples
To provide a clearer understanding of how data analysis techniques are applied in research, let’s consider a hypothetical research study focused on evaluating the impact of online learning platforms on students’ academic performance.
Research Objective:
Determine if students using online learning platforms achieve higher academic performance compared to those relying solely on traditional classroom instruction.
Data Collection:
- Quantitative Data: Academic scores (grades) of students using online platforms and those using traditional classroom methods.
- Qualitative Data: Feedback from students regarding their learning experiences, challenges faced, and preferences.
Data Analysis Techniques Applied:
1) Descriptive Analysis:
- Calculate the mean, median, and mode of academic scores for both groups.
- Create frequency distributions to represent the distribution of grades in each group.
2) Diagnostic Analysis:
- Conduct an Analysis of Variance (ANOVA) to determine if there’s a statistically significant difference in academic scores between the two groups.
- Perform Regression Analysis to assess the relationship between the time spent on online platforms and academic performance.
3) Predictive Analysis:
- Utilize Time Series Forecasting to predict future academic performance trends based on historical data.
- Implement Machine Learning algorithms to develop a predictive model that identifies factors contributing to academic success on online platforms.
4) Prescriptive Analysis:
- Apply Optimization Models to identify the optimal combination of online learning resources (e.g., video lectures, interactive quizzes) that maximize academic performance.
- Use Simulation Techniques to evaluate different scenarios, such as varying student engagement levels with online resources, to determine the most effective strategies for improving learning outcomes.
5) Specific Techniques:
- Conduct Factor Analysis on qualitative feedback to identify common themes or factors influencing students’ perceptions and experiences with online learning.
- Perform Cluster Analysis to segment students based on their engagement levels, preferences, or academic outcomes, enabling targeted interventions or personalized learning strategies.
- Apply Sentiment Analysis on textual feedback to categorize students’ sentiments as positive, negative, or neutral regarding online learning experiences.
By applying a combination of qualitative and quantitative data analysis techniques, this research example aims to provide comprehensive insights into the effectiveness of online learning platforms.
Also Read: Learning Path to Become a Data Analyst in 2024
Data Analysis Techniques in Quantitative Research
Quantitative research involves collecting numerical data to examine relationships, test hypotheses, and make predictions. Various data analysis techniques are employed to interpret and draw conclusions from quantitative data. Here are some key data analysis techniques commonly used in quantitative research:
1) Descriptive Statistics:
- Description: Descriptive statistics are used to summarize and describe the main aspects of a dataset, such as central tendency (mean, median, mode), variability (range, variance, standard deviation), and distribution (skewness, kurtosis).
- Applications: Summarizing data, identifying patterns, and providing initial insights into the dataset.
2) Inferential Statistics:
- Description: Inferential statistics involve making predictions or inferences about a population based on a sample of data. This technique includes hypothesis testing, confidence intervals, t-tests, chi-square tests, analysis of variance (ANOVA), regression analysis, and correlation analysis.
- Applications: Testing hypotheses, making predictions, and generalizing findings from a sample to a larger population.
3) Regression Analysis:
- Description: Regression analysis is a statistical technique used to model and examine the relationship between a dependent variable and one or more independent variables. Linear regression, multiple regression, logistic regression, and nonlinear regression are common types of regression analysis .
- Applications: Predicting outcomes, identifying relationships between variables, and understanding the impact of independent variables on the dependent variable.
4) Correlation Analysis:
- Description: Correlation analysis is used to measure and assess the strength and direction of the relationship between two or more variables. The Pearson correlation coefficient, Spearman rank correlation coefficient, and Kendall’s tau are commonly used measures of correlation.
- Applications: Identifying associations between variables and assessing the degree and nature of the relationship.
5) Factor Analysis:
- Description: Factor analysis is a multivariate statistical technique used to identify and analyze underlying relationships or factors among a set of observed variables. It helps in reducing the dimensionality of data and identifying latent variables or constructs.
- Applications: Identifying underlying factors or constructs, simplifying data structures, and understanding the underlying relationships among variables.
6) Time Series Analysis:
- Description: Time series analysis involves analyzing data collected or recorded over a specific period at regular intervals to identify patterns, trends, and seasonality. Techniques such as moving averages, exponential smoothing, autoregressive integrated moving average (ARIMA), and Fourier analysis are used.
- Applications: Forecasting future trends, analyzing seasonal patterns, and understanding time-dependent relationships in data.
7) ANOVA (Analysis of Variance):
- Description: Analysis of variance (ANOVA) is a statistical technique used to analyze and compare the means of two or more groups or treatments to determine if they are statistically different from each other. One-way ANOVA, two-way ANOVA, and MANOVA (Multivariate Analysis of Variance) are common types of ANOVA.
- Applications: Comparing group means, testing hypotheses, and determining the effects of categorical independent variables on a continuous dependent variable.
8) Chi-Square Tests:
- Description: Chi-square tests are non-parametric statistical tests used to assess the association between categorical variables in a contingency table. The Chi-square test of independence, goodness-of-fit test, and test of homogeneity are common chi-square tests.
- Applications: Testing relationships between categorical variables, assessing goodness-of-fit, and evaluating independence.
These quantitative data analysis techniques provide researchers with valuable tools and methods to analyze, interpret, and derive meaningful insights from numerical data. The selection of a specific technique often depends on the research objectives, the nature of the data, and the underlying assumptions of the statistical methods being used.
Also Read: Analysis vs. Analytics: How Are They Different?
Data Analysis Methods
Data analysis methods refer to the techniques and procedures used to analyze, interpret, and draw conclusions from data. These methods are essential for transforming raw data into meaningful insights, facilitating decision-making processes, and driving strategies across various fields. Here are some common data analysis methods:
- Description: Descriptive statistics summarize and organize data to provide a clear and concise overview of the dataset. Measures such as mean, median, mode, range, variance, and standard deviation are commonly used.
- Description: Inferential statistics involve making predictions or inferences about a population based on a sample of data. Techniques such as hypothesis testing, confidence intervals, and regression analysis are used.
3) Exploratory Data Analysis (EDA):
- Description: EDA techniques involve visually exploring and analyzing data to discover patterns, relationships, anomalies, and insights. Methods such as scatter plots, histograms, box plots, and correlation matrices are utilized.
- Applications: Identifying trends, patterns, outliers, and relationships within the dataset.
4) Predictive Analytics:
- Description: Predictive analytics use statistical algorithms and machine learning techniques to analyze historical data and make predictions about future events or outcomes. Techniques such as regression analysis, time series forecasting, and machine learning algorithms (e.g., decision trees, random forests, neural networks) are employed.
- Applications: Forecasting future trends, predicting outcomes, and identifying potential risks or opportunities.
5) Prescriptive Analytics:
- Description: Prescriptive analytics involve analyzing data to recommend actions or strategies that optimize specific objectives or outcomes. Optimization techniques, simulation models, and decision-making algorithms are utilized.
- Applications: Recommending optimal strategies, decision-making support, and resource allocation.
6) Qualitative Data Analysis:
- Description: Qualitative data analysis involves analyzing non-numerical data, such as text, images, videos, or audio, to identify themes, patterns, and insights. Methods such as content analysis, thematic analysis, and narrative analysis are used.
- Applications: Understanding human behavior, attitudes, perceptions, and experiences.
7) Big Data Analytics:
- Description: Big data analytics methods are designed to analyze large volumes of structured and unstructured data to extract valuable insights. Technologies such as Hadoop, Spark, and NoSQL databases are used to process and analyze big data.
- Applications: Analyzing large datasets, identifying trends, patterns, and insights from big data sources.
8) Text Analytics:
- Description: Text analytics methods involve analyzing textual data, such as customer reviews, social media posts, emails, and documents, to extract meaningful information and insights. Techniques such as sentiment analysis, text mining, and natural language processing (NLP) are used.
- Applications: Analyzing customer feedback, monitoring brand reputation, and extracting insights from textual data sources.
These data analysis methods are instrumental in transforming data into actionable insights, informing decision-making processes, and driving organizational success across various sectors, including business, healthcare, finance, marketing, and research. The selection of a specific method often depends on the nature of the data, the research objectives, and the analytical requirements of the project or organization.
Also Read: Quantitative Data Analysis: Types, Analysis & Examples
Data Analysis Tools
Data analysis tools are essential instruments that facilitate the process of examining, cleaning, transforming, and modeling data to uncover useful information, make informed decisions, and drive strategies. Here are some prominent data analysis tools widely used across various industries:
1) Microsoft Excel:
- Description: A spreadsheet software that offers basic to advanced data analysis features, including pivot tables, data visualization tools, and statistical functions.
- Applications: Data cleaning, basic statistical analysis, visualization, and reporting.
2) R Programming Language :
- Description: An open-source programming language specifically designed for statistical computing and data visualization.
- Applications: Advanced statistical analysis, data manipulation, visualization, and machine learning.
3) Python (with Libraries like Pandas, NumPy, Matplotlib, and Seaborn):
- Description: A versatile programming language with libraries that support data manipulation, analysis, and visualization.
- Applications: Data cleaning, statistical analysis, machine learning, and data visualization.
4) SPSS (Statistical Package for the Social Sciences):
- Description: A comprehensive statistical software suite used for data analysis, data mining, and predictive analytics.
- Applications: Descriptive statistics, hypothesis testing, regression analysis, and advanced analytics.
5) SAS (Statistical Analysis System):
- Description: A software suite used for advanced analytics, multivariate analysis, and predictive modeling.
- Applications: Data management, statistical analysis, predictive modeling, and business intelligence.
6) Tableau:
- Description: A data visualization tool that allows users to create interactive and shareable dashboards and reports.
- Applications: Data visualization , business intelligence , and interactive dashboard creation.
7) Power BI:
- Description: A business analytics tool developed by Microsoft that provides interactive visualizations and business intelligence capabilities.
- Applications: Data visualization, business intelligence, reporting, and dashboard creation.
8) SQL (Structured Query Language) Databases (e.g., MySQL, PostgreSQL, Microsoft SQL Server):
- Description: Database management systems that support data storage, retrieval, and manipulation using SQL queries.
- Applications: Data retrieval, data cleaning, data transformation, and database management.
9) Apache Spark:
- Description: A fast and general-purpose distributed computing system designed for big data processing and analytics.
- Applications: Big data processing, machine learning, data streaming, and real-time analytics.
10) IBM SPSS Modeler:
- Description: A data mining software application used for building predictive models and conducting advanced analytics.
- Applications: Predictive modeling, data mining, statistical analysis, and decision optimization.
These tools serve various purposes and cater to different data analysis needs, from basic statistical analysis and data visualization to advanced analytics, machine learning, and big data processing. The choice of a specific tool often depends on the nature of the data, the complexity of the analysis, and the specific requirements of the project or organization.
Also Read: How to Analyze Survey Data: Methods & Examples
Importance of Data Analysis in Research
The importance of data analysis in research cannot be overstated; it serves as the backbone of any scientific investigation or study. Here are several key reasons why data analysis is crucial in the research process:
- Data analysis helps ensure that the results obtained are valid and reliable. By systematically examining the data, researchers can identify any inconsistencies or anomalies that may affect the credibility of the findings.
- Effective data analysis provides researchers with the necessary information to make informed decisions. By interpreting the collected data, researchers can draw conclusions, make predictions, or formulate recommendations based on evidence rather than intuition or guesswork.
- Data analysis allows researchers to identify patterns, trends, and relationships within the data. This can lead to a deeper understanding of the research topic, enabling researchers to uncover insights that may not be immediately apparent.
- In empirical research, data analysis plays a critical role in testing hypotheses. Researchers collect data to either support or refute their hypotheses, and data analysis provides the tools and techniques to evaluate these hypotheses rigorously.
- Transparent and well-executed data analysis enhances the credibility of research findings. By clearly documenting the data analysis methods and procedures, researchers allow others to replicate the study, thereby contributing to the reproducibility of research findings.
- In fields such as business or healthcare, data analysis helps organizations allocate resources more efficiently. By analyzing data on consumer behavior, market trends, or patient outcomes, organizations can make strategic decisions about resource allocation, budgeting, and planning.
- In public policy and social sciences, data analysis is instrumental in developing and evaluating policies and interventions. By analyzing data on social, economic, or environmental factors, policymakers can assess the effectiveness of existing policies and inform the development of new ones.
- Data analysis allows for continuous improvement in research methods and practices. By analyzing past research projects, identifying areas for improvement, and implementing changes based on data-driven insights, researchers can refine their approaches and enhance the quality of future research endeavors.
However, it is important to remember that mastering these techniques requires practice and continuous learning. That’s why we highly recommend the Data Analytics Course by Physics Wallah . Not only does it cover all the fundamentals of data analysis, but it also provides hands-on experience with various tools such as Excel, Python, and Tableau. Plus, if you use the “ READER ” coupon code at checkout, you can get a special discount on the course.
For Latest Tech Related Information, Join Our Official Free Telegram Group : PW Skills Telegram Group
Data Analysis Techniques in Research FAQs
What are the 5 techniques for data analysis.
The five techniques for data analysis include: Descriptive Analysis Diagnostic Analysis Predictive Analysis Prescriptive Analysis Qualitative Analysis
What are techniques of data analysis in research?
Techniques of data analysis in research encompass both qualitative and quantitative methods. These techniques involve processes like summarizing raw data, investigating causes of events, forecasting future outcomes, offering recommendations based on predictions, and examining non-numerical data to understand concepts or experiences.
What are the 3 methods of data analysis?
The three primary methods of data analysis are: Qualitative Analysis Quantitative Analysis Mixed-Methods Analysis
What are the four types of data analysis techniques?
The four types of data analysis techniques are: Descriptive Analysis Diagnostic Analysis Predictive Analysis Prescriptive Analysis
- 10 Best Companies For Data Analysis Internships 2024
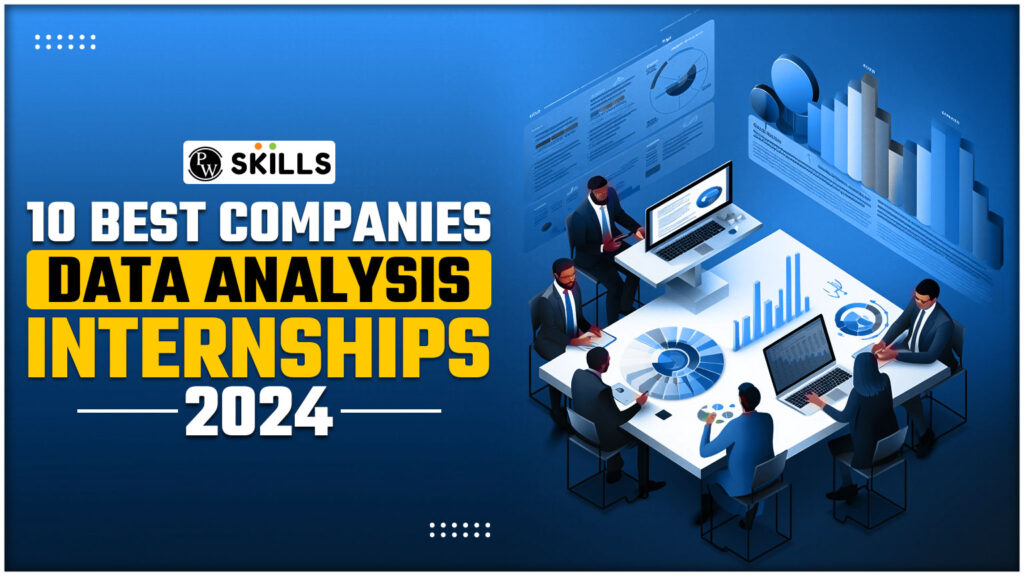
This article will help you provide the top 10 best companies for a Data Analysis Internship which will not only…
- Top Best Big Data Analytics Classes 2024
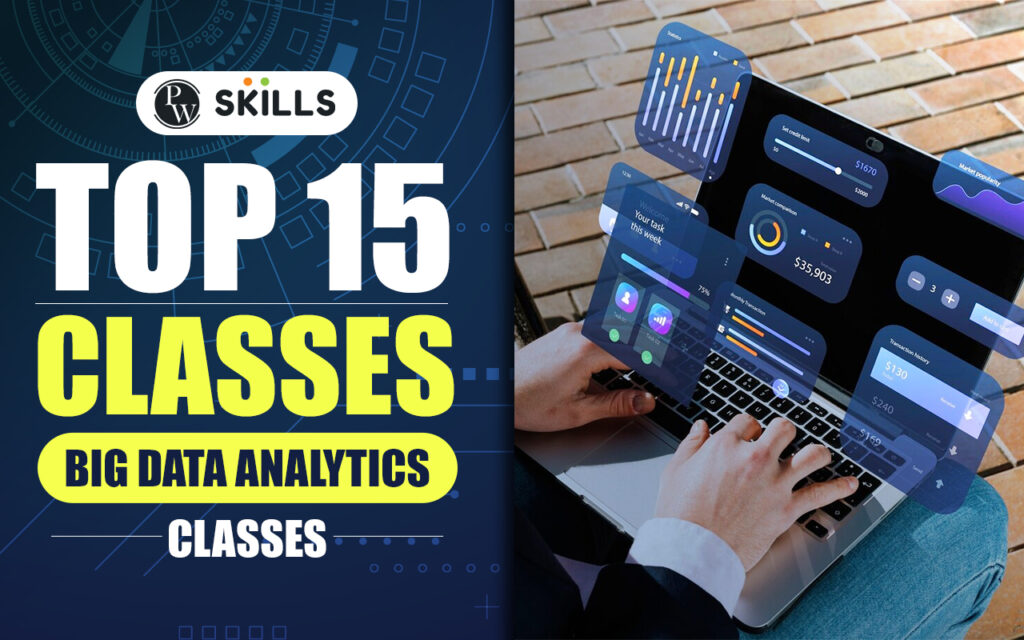
Many websites and institutions provide online remote big data analytics classes to help you learn and also earn certifications for…
- Data Analyst Roadmap 2024: Responsibilities, Skills Required, Career Path
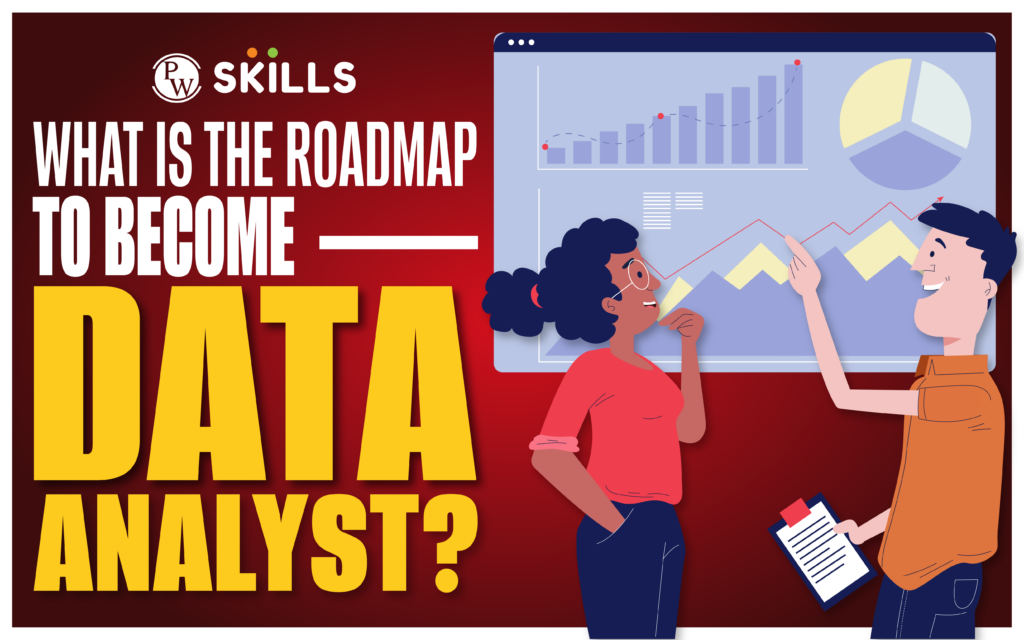
Data Analyst Roadmap: The field of data analysis is booming and is very rewarding for those with the right skills.…
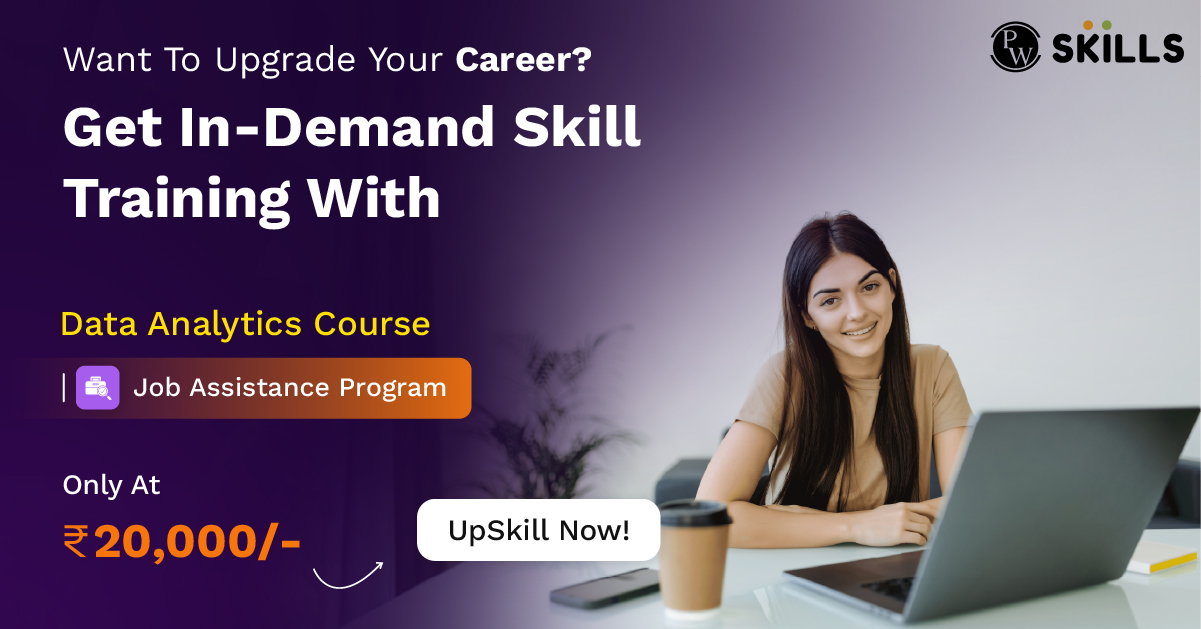
Related Articles
- The Best Data And Analytics Courses For Beginners
- Best Courses For Data Analytics: Top 10 Courses For Your Career in Trend
- BI & Analytics: What’s The Difference?
- Predictive Analysis: Predicting the Future with Data
- Graph Analytics – What Is it and Why Does It Matter?
- How to Analysis of Survey Data: Methods & Examples
- Google Data Analytics Professional Certificate Review, Cost, Eligibility
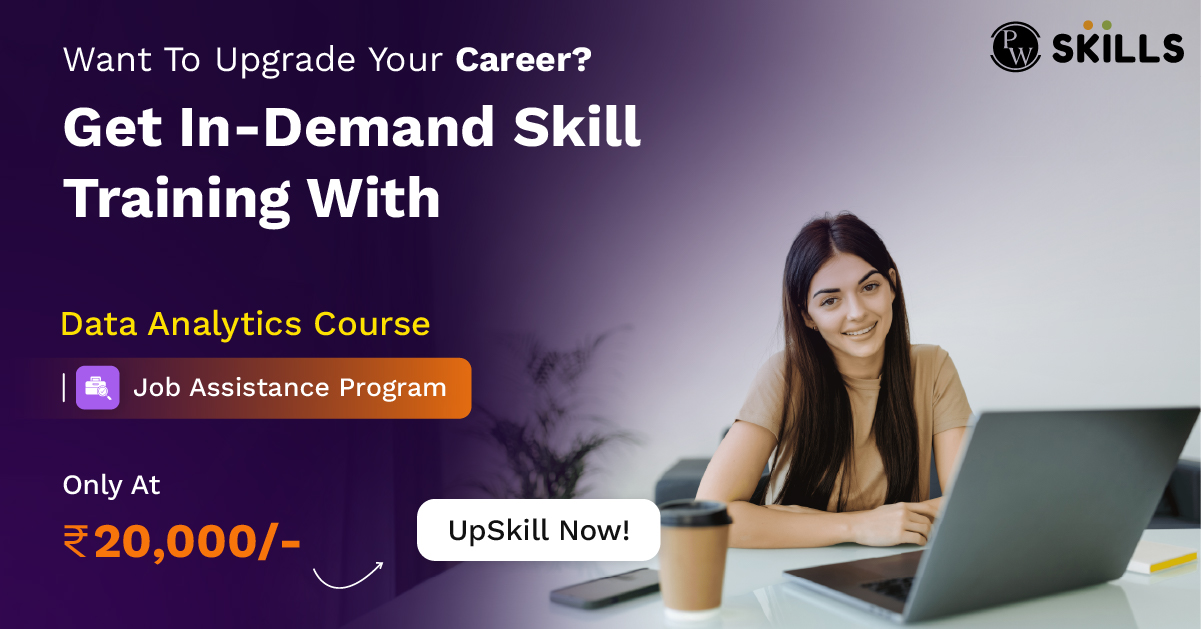
Child Care and Early Education Research Connections
Data analysis.
Different statistics and methods used to describe the characteristics of the members of a sample or population, explore the relationships between variables, to test research hypotheses, and to visually represent data are described. Terms relating to the topics covered are defined in the Research Glossary .
Descriptive Statistics
Tests of Significance
Graphical/Pictorial Methods
Analytical techniques.
Descriptive statistics can be useful for two purposes:
To provide basic information about the characteristics of a sample or population. These characteristics are represented by variables in a research study dataset.
To highlight potential relationships between these characteristics, or the relationships among the variables in the dataset.
The four most common descriptive statistics are:
Proportions, Percentages and Ratios
Measures of central tendency, measures of dispersion, measures of association.
One of the most basic ways of describing the characteristics of a sample or population is to classify its individual members into mutually exclusive categories and counting the number of cases in each of the categories. In research, variables with discrete, qualitative categories are called nominal or categorical variables. The categories can be given numerical codes, but they cannot be ranked, added, or multiplied. Examples of nominal variables include gender (male, female), preschool program attendance (yes, no), and race/ethnicity (White, African American, Hispanic, Asian, American Indian). Researchers calculate proportions, percentages and ratios in order to summarize the data from nominal or categorical variables and to allow for comparisons to be made between groups.
Proportion —The number of cases in a category divided by the total number of cases across all categories of a variable.
Percentage —The proportion multiplied by 100 (or the number of cases in a category divided by the total number of cases across all categories of a value times 100).
Ratio —The number of cases in one category to the number of cases in a second category.
A researcher selects a sample of 100 students from a Head Start program. The sample includes 20 White children, 30 African American children, 40 Hispanic children and 10 children of mixed-race/ethnicity.
Proportion of Hispanic children in the program = 40 / (20+30+40+10) = .40.
Percentage of Hispanic children in the program = .40 x 100 = 40%.
Ratio of Hispanic children to White children in the program = 40/20 = 2.0, or the ratio of Hispanic to White children enrolled in the Head Start program is 2 to 1.
Proportions, percentages and ratios are used to summarize the characteristics of a sample or population that fall into discrete categories. Measures of central tendency are the most basic and, often, the most informative description of a population's characteristics, when those characteristics are measured using an interval scale. The values of an interval variable are ordered where the distance between any two adjacent values is the same but the zero point is arbitrary. Values on an interval scale can be added and subtracted. Examples of interval scales or interval variables include household income, years of schooling, hours a child spends in child care and the cost of child care.
Measures of central tendency describe the "average" member of the sample or population of interest. There are three measures of central tendency:
Mean —The arithmetic average of the values of a variable. To calculate the mean, all the values of a variable are summed and divided by the total number of cases.
Median —The value within a set of values that divides the values in half (i.e. 50% of the variable's values lie above the median, and 50% lie below the median).
Mode —The value of a variable that occurs most often.
The annual incomes of five randomly selected people in the United States are $10,000, $10,000, $45,000, $60,000, and $1,000,000.
Mean Income = (10,000 + 10,000 + 45,000 + 60,000 + 1,000,000) / 5 = $225,000.
Median Income = $45,000.
Modal Income = $10,000.
The mean is the most commonly used measure of central tendency. Medians are generally used when a few values are extremely different from the rest of the values (this is called a skewed distribution). For example, the median income is often the best measure of the average income because, while most individuals earn between $0 and $200,000 annually, a handful of individuals earn millions.
Measures of dispersion provide information about the spread of a variable's values. There are three key measures of dispersion:
Range is simply the difference between the smallest and largest values in the data. Researchers often report simply the values of the range (e.g., 75 – 100).
Variance is a commonly used measure of dispersion, or how spread out a set of values are around the mean. It is calculated by taking the average of the squared differences between each value and the mean. The variance is the standard deviation squared.
Standard deviation , like variance, is a measure of the spread of a set of values around the mean of the values. The wider the spread, the greater the standard deviation and the greater the range of the values from their mean. A small standard deviation indicates that most of the values are close to the mean. A large standard deviation on the other hand indicates that the values are more spread out. The standard deviation is the square root of the variance.
Five randomly selected children were administered a standardized reading assessment. Their scores on the assessment were 50, 50, 60,75 and 90 with a mean score of 65.
Range = 90 - 50 = 40.
Variance = [(50 - 65)2 + (50 - 65)2 + (60 - 65)2 + (75 - 65)2 + (90 - 65)2] / 5 = 300.
Standard Deviation = Square Root (150,540,000,000) = 17.32.
Skewness and Kurtosis
The range, variance and standard deviation are measures of dispersion and provide information about the spread of the values of a variable. Two additional measures provide information about the shape of the distribution of values.
Skew is a measure of whether some values of a variable are extremely different from the majority of the values. Skewness refers to the tendency of the values of a variable to depart from symmetry. A distribution is symmetric if one half of the distribution is exactly equal to the other half. For example, the distribution of annual income in the U.S. is skewed because most people make between $0 and $200,000 a year, but a handful of people earn millions. A variable is positively skewed (skewed to the right) if the extreme values are higher than the majority of values. A variable is negatively skewed (skewed to the left) if the extreme values are lower than the majority of values. In the example of students' standardized test scores, the distribution is slightly positively skewed.
Kurtosis measures how outlier-prone a distribution is. Outliers are values of a variable that are much smaller or larger than most of the values found in a dataset. The kurtosis of a normal distribution is 0. If the kurtosis is different from 0, then the distribution produces outliers that are either more extreme (positive kurtosis) or less extreme (negative kurtosis) than are produced by the normal distribution.
Measures of association indicate whether two variables are related. Two measures are commonly used:
Chi-square test of independence
Correlation
Chi-Square test of independence is used to evaluate whether there is an association between two variables. (The chi-square test can also be used as a measure of goodness of fit, to test if data from a sample come from a population with a specific distribution, as an alternative to Anderson-Darling and Kolmogorov-Smirnov goodness-of-fit tests.)
It is most often used with nominal data (i.e., data that are put into discrete categories: e.g., gender [male, female] and type of job [unskilled, semi-skilled, skilled]) to determine whether they are associated. However, it can also be used with ordinal data.
Assumes that the samples being compared (e.g., males, females) are independent.
Tests the null hypothesis of no difference between the two variables (i.e., type of job is not related to gender).
To test for associations, a chi-square is calculated in the following way: Suppose a researcher wants to know whether there is a relationship between gender and two types of jobs, construction worker and administrative assistant. To perform a chi-square test, the researcher counts the number of female administrative assistants, the number of female construction workers, the number of male administrative assistants, and the number of male construction workers in the data. These counts are compared with the number that would be expected in each category if there were no association between job type and gender (this expected count is based on statistical calculations). The association between the two variables is determined to be significant (the null hypothesis is rejected), if the value of the chi-square test is greater than or equal to the critical value for a given significance level (typically .05) and the degrees of freedom associated with the test found in a chi-square table. The degrees of freedom for the chi-square are calculated using the following formula: df = (r-1)(c-1) where r is the number of rows and c is the number of columns in a contingency or cross-tabulation table. For example, the critical value for a 2 x 2 table with 1 degree of freedom ([2-1][2-1]=1) is 3.841.
Correlation coefficient is used to measure the strength and direction of the relationship between numeric variables (e.g., weight and height).
The most common correlation coefficient is the Pearson's product-moment correlation coefficient (or simply Pearson's r ), which can range from -1 to +1.
Values closer to 1 (either positive or negative) indicate that a stronger association exists between the two variables.
A positive coefficient (values between 0 and 1) suggests that larger values of one of the variables are accompanied by larger values of the other variable. For example, height and weight are usually positively correlated because taller people tend to weigh more.
A negative association (values between 0 and -1) suggests that larger values of one of the variables are accompanied by smaller values of the other variable. For example, age and hours slept per night are often negatively correlated because older people usually sleep fewer hours per night than younger people.
The findings reported by researchers are typically based on data collected from a single sample that was drawn from the population of interest (e.g., a sample of children selected from the population of children enrolled in Head Start or Early Head Start). If additional random samples of the same size were drawn from this population, the estimated percentages and means calculated using the data from each of these other samples might differ by chance somewhat from the estimates produced from one sample. Researchers use one of several tests to evaluate whether their findings are statistically significant.
Statistical significance refers to the probability or likelihood that the difference between groups or the relationship between variables observed in statistical analyses is not due to random chance (e.g., that differences between the average scores on a measure of language development between 3- and 4-year-olds are likely to be “real” rather than just observed in this sample by chance). If there is a very small probability that an observed difference or relationship is due to chance, the results are said to reach statistical significance. This means that the researcher concludes that there is a real difference between two groups or a real relationship between the observed variables.
Significance tests and the associated p- value only tell us how likely it is that a statistical result (e.g., a difference between the means of two or more groups, or a correlation between two variables) is due to chance. The p-value is the probability that the results of a statistical test are due to chance. In the social and behavioral sciences, a p-value less than or equal to .05 is usually interpreted to mean that the results are statistically significant (that the statistical results would occur by chance 5 times or fewer out of 100), although sometimes researchers use a p-value of .10 to indicate whether a result is statistically significant. The lower the p-value, the less likely a statistical result is due to chance. Lower p-values are therefore a more rigorous criteria for concluding significance.
Researchers use a variety of approaches to test whether their findings are statistically significant or not. The choice depends on several factors, including the number of groups being compared, whether the groups are independent from one another, and the type of variables used in the analysis. Three widely used tests are the t-test, F-test, and Chi-square test.
Three of the more widely used tests of statistical significance are described briefly below.
Chi-Square test is used when testing for associations between categorical variables (e.g., differences in whether a child has been diagnosed as having a cognitive disability by gender or race/ethnicity). It is also used as a goodness-of-fit test to determine whether data from a sample come from a population with a specific distribution.
t-test is used to compare the means of two independent samples (independent t-test), the means of one sample at different times (paired sample t-test) or the mean of one sample against a known mean (one sample t-test). For example, when comparing the mean assessment scores of boys and girls or the mean scores of 3- and 4-year-old children, an independent t-test would be used. When comparing the mean assessment scores of girls only at two time points (e.g., fall and spring of the program year) a paired t-test would be used. A one sample t-test would be used when comparing the mean scores of a sample of children to the mean score of a population of children. The t- test is appropriate for small sample sizes (less than 30) although it is often used when testing group differences for larger samples. It is also used to test whether correlation and regression coefficients are significantly different from zero.
F-test is an extension of the t-test and is used to compare the means of three or more independent samples (groups). The F-test is used in Analysis of Variance (ANOVA) to examine the ratio of the between groups to within groups variance. It is also used to test the significance of the total variance explained by a regression model with multiple independent variables.
Significance tests alone do not tell us anything about the size of the difference between groups or the strength of the association between variables. Because significance test results are sensitive to sample size, studies with different sample sizes with the same means and standard deviations would have different t statistics and p values. It is therefore important that researchers provide additional information about the size of the difference between groups or the association and whether the difference/association is substantively meaningful.
See the following for additional information about descriptive statistics and tests of significance:
Descriptive analysis in education: A guide for researchers (PDF)
Basic Statistics
Effect Sizes and Statistical Significance
Summarizing and Presenting Data
There are several graphical and pictorial methods that enhance understanding of individual variables and the relationships between variables. Graphical and pictorial methods provide a visual representation of the data. Some of these methods include:
Line graphs
Scatter plots.
Geographical Information Systems (GIS)
Bar charts visually represent the frequencies or percentages with which different categories of a variable occur.
Bar charts are most often used when describing the percentages of different groups with a specific characteristic. For example, the percentages of boys and girls who participate in team sports. However, they may also be used when describing averages such as the average boys and girls spend per week participating in team sports.
Each category of a variable (e.g., gender [boys and girls], children's age [3, 4, and 5]) is displayed along the bottom (or horizontal or X axis) of a bar chart.
The vertical axis (or Y axis) includes the values of the statistic on that the groups are being compared (e.g., percentage participating in team sports).
A bar is drawn for each of the categories along the horizontal axis and the height of the bar corresponds to the frequency or percentage with which that value occurs.
A pie chart (or a circle chart) is one of the most commonly used methods for graphically presenting statistical data.
As its name suggests, it is a circular graphic, which is divided into slices to illustrate the proportion or percentage of a sample or population that belong to each of the categories of a variable.
The size of each slice represents the proportion or percentage of the total sample or population with a specific characteristic (found in a specific category). For example, the percentage of children enrolled in Early Head Start who are members of different racial/ethnic groups would be represented by different slices with the size of each slice proportionate to the group's representation in the total population of children enrolled in the Early Head Start program.
A line graph is a type of chart which displays information as a series of data points connected by a straight line.
Line graphs are often used to show changes in a characteristic over time.
It has an X-axis (horizontal axis) and a Y axis (vertical axis). The time segments of interest are displayed on the X-axis (e.g., years, months). The range of values that the characteristic of interest can take are displayed along the Y-axis (e.g., annual household income, mean years of schooling, average cost of child care). A data point is plotted coinciding with the value of the Y variable plotted for each of the values of the X variable, and a line is drawn connecting the points.
Scatter plots display the relationship between two quantitative or numeric variables by plotting one variable against the value of another variable
The values of one of the two variables are displayed on the horizontal axis (x axis) and the values of the other variable are displayed on the vertical axis (y axis)
Each person or subject in a study would receive one data point on the scatter plot that corresponds to his or her values on the two variables. For example, a scatter plot could be used to show the relationship between income and children's scores on a math assessment. A data point for each child in the study showing his or her math score and family income would be shown on the scatter plot. Thus, the number of data points would equal the total number of children in the study.
Geographic Information Systems (GIS)
A Geographic Information System is computer software capable of capturing, storing, analyzing, and displaying geographically referenced information; that is, data identified according to location.
Using a GIS program, a researcher can create a map to represent data relationships visually. For example, the National Center for Education Statistics creates maps showing the characteristics of school districts across the United States such as the percentage of children living in married couple households, median family incomes and percentage of population that speaks a language other than English. The data that are linked to school district location come from the American Community Survey.
Display networks of relationships among variables, enabling researchers to identify the nature of relationships that would otherwise be too complex to conceptualize.
See the following for additional information about different graphic methods:
Graphical Analytic Techniques
Geographic Information Systems
Researchers use different analytical techniques to examine complex relationships between variables. There are three basic types of analytical techniques:
Regression Analysis
Grouping methods, multiple equation models.
Regression analysis assumes that the dependent, or outcome, variable is directly affected by one or more independent variables. There are four important types of regression analyses:
Ordinary least squares (OLS) regression
OLS regression (also known as linear regression) is used to determine the relationship between a dependent variable and one or more independent variables.
OLS regression is used when the dependent variable is continuous. Continuous variables, in theory, can take on any value with a range. For example, family child care expenses, measured in dollars, is a continuous variable.
Independent variables may be nominal, ordinal or continuous. Nominal variables, which are also referred to as categorical variables, have two or more non-numeric or qualitative categories. Examples of nominal variables are children's gender (male, female), their parents' marital status (single, married, separated, divorced), and the type of child care children receive (center-based, home-based care). Ordinal variables are similar to nominal variables except it is possible to order the categories and the order has meaning. For example, children's families’ socioeconomic status may be grouped as low, middle and high.
When used to estimate the associations between two or more independent variables and a single dependent variable, it is called multiple linear regression.
In multiple regression, the coefficient (i.e., standardized or unstandardized regression coefficient for each independent variable) tells you how much the dependent variable is expected to change when that independent variable increases by one, holding all the other independent variables constant.
Logistic regression
Logistic regression (or logit regression) is a special form of regression analysis that is used to examine the associations between a set of independent or predictor variables and a dichotomous outcome variable. A dichotomous variable is a variable with only two possible values, e.g. child receives child care before or after the Head Start program day (yes, no).
Like linear regression, the independent variables may be either interval, ordinal, or nominal. A researcher might use logistic regression to study the relationships between parental education, household income, and parental employment and whether children receive child care from someone other than their parents (receives nonparent care/does not receive nonparent care).
Hierarchical linear modeling (HLM)
Used when data are nested. Nested data occur when several individuals belong to the same group under study. For example, in child care research, children enrolled in a center-based child care program are grouped into classrooms with several classrooms in a center. Thus, the children are nested within classrooms and classrooms are nested within centers.
Allows researchers to determine the effects of characteristics for each level of nested data, classrooms and centers, on the outcome variables. HLM is also used to study growth (e.g., growth in children’s reading and math knowledge and skills over time).
Duration models
Used to estimate the length of time before a given event occurs or the length of time spent in a state. For example, in child care policy research, duration models have been used to estimate the length of time that families receive child care subsidies.
Sometimes referred to as survival analysis or event history analysis.
Grouping methods are techniques for classifying observations into meaningful categories. Two of the most common grouping methods are discriminant analysis and cluster analysis.
Discriminant analysis
Identifies characteristics that distinguish between groups. For example, a researcher could use discriminant analysis to determine which characteristics identify families that seek child care subsidies and which identify families that do not.
It is used when the dependent variable is a categorical variable (e.g., family receives child care subsidies [yes, no], child enrolled in family care [yes, no], type of child care child receives [relative care, non-relative care, center-based care]). The independent variables are interval variables (e.g., years of schooling, family income).
Cluster analysis
Used to classify similar individuals together. It uses a set of measured variables to classify a sample of individuals (or organizations) into a number of groups such that individuals with similar values on the variables are placed in the same group. For example, cluster analysis would be used to group together parents who hold similar views of child care or children who are suspended from school.
Its goal is to sort individuals into groups in such a way that individuals in the same group (cluster) are more similar to each other than to individuals in other groups.
The variables used in cluster analysis may be nominal, ordinal or interval.
Multiple equation modeling, which is an extension of regression, is used to examine the causal pathways from independent variables to the dependent variable. For example, what are the variables that link (or explain) the relationship between maternal education (independent variable) and children's early reading skills (dependent variable)? These variables might include the nature and quality of mother-child interactions or the frequency and quality of shared book reading.
There are two main types of multiple equation models:
Path analysis
Structural equation modeling
Path analysis is an extension of multiple regression that allows researchers to examine multiple direct and indirect effects of a set of variables on a dependent, or outcome, variable. In path analysis, a direct effect measures the extent to which the dependent variable is influenced by an independent variable. An indirect effect measures the extent to which an independent variable's influence on the dependent variable is due to another variable.
A path diagram is created that identifies the relationships (paths) between all the variables and the direction of the influence between them.
The paths can run directly from an independent variable to a dependent variable (e.g., X→Y), or they can run indirectly from an independent variable, through an intermediary, or mediating, variable, to the dependent variable (e.g. X1→X2→Y).
The paths in the model are tested to determine the relative importance of each.
Because the relationships between variables in a path model can become complex, researchers often avoid labeling the variables in the model as independent and dependent variables. Instead, two types of variables are found in these models:
Exogenous variables are not affected by other variables in the model. They have straight arrows emerging from them and not pointing to them.
Endogenous variables are influenced by at least one other variable in the model. They have at least one straight arrow pointing to them.
Structural equation modeling (SEM)
Structural equation modeling expands path analysis by allowing for multiple indicators of unobserved (or latent) variables in the model. Latent variables are variables that are not directly observed (measured), but instead are inferred from other variables that are observed or directly measured. For example, children's school readiness is a latent variable with multiple indicators of children's development across multiple domains (e.g., children's scores on standardized assessments of early math and literacy, language, scores based on teacher reports of children's social skills and problem behaviors).
There are two parts to a SEM analysis. First, the measurement model is tested. This involves examining the relationships between the latent variables and their measures (indicators). Second, the structural model is tested in order to examine how the latent variables are related to one another. For example, a researcher might use SEM to investigate the relationships between different types of executive functions and word reading and reading comprehension for elementary school children. In this example, the latent variables word reading and reading comprehension might be inferred from a set of standardized reading assessments and the latent variables cognitive flexibility and inhibitory control from a set of executive function tasks. The measurement model of SEM allows the researcher to evaluate how well children's scores on the standardized reading assessments combine to identify children's word reading and reading comprehension. Assuming that the results of these analyses are acceptable, the researcher would move on to an evaluation of the structural model, examining the predicted relationships between two types of executive functions and two dimensions of reading.
SEM has several advantages over traditional path analysis:
Use of multiple indicators for key variables reduces measurement error.
Can test whether the effects of variables in the model and the relationships depicted in the entire model are the same for different groups (e.g., are the direct and indirect effects of parent investments on children's school readiness the same for White, Hispanic and African American children).
Can test models with multiple dependent variables (e.g., models predicting several domains of child development).
See the following for additional information about multiple equation models:
Finding Our Way: An Introduction to Path Analysis (Streiner)
An Introduction to Structural Equation Modeling (Hox & Bechger) (PDF)

An official website of the United States government
The .gov means it’s official. Federal government websites often end in .gov or .mil. Before sharing sensitive information, make sure you’re on a federal government site.
The site is secure. The https:// ensures that you are connecting to the official website and that any information you provide is encrypted and transmitted securely.
- Publications
- Account settings
Preview improvements coming to the PMC website in October 2024. Learn More or Try it out now .
- Advanced Search
- Journal List
- BMJ Open Access

Analytical studies: a framework for quality improvement design and analysis
Conducting studies for learning is fundamental to improvement. Deming emphasised that the reason for conducting a study is to provide a basis for action on the system of interest. He classified studies into two types depending on the intended target for action. An enumerative study is one in which action will be taken on the universe that was studied. An analytical study is one in which action will be taken on a cause system to improve the future performance of the system of interest. The aim of an enumerative study is estimation, while an analytical study focuses on prediction. Because of the temporal nature of improvement, the theory and methods for analytical studies are a critical component of the science of improvement.
Introduction: enumerative and analytical studies
Designing studies that make it possible to learn from experience and take action to improve future performance is an essential element of quality improvement. These studies use the now traditional theory established through the work of Fisher, 1 Cox, 2 Campbell and Stanley, 3 and others that is widely used in biomedicine research. These designs are used to discover new phenomena that lead to hypothesis generation, and to explore causal mechanisms, 4 as well as to evaluate efficacy and effectiveness. They include observational, retrospective, prospective, pre-experimental, quasiexperimental, blocking, factorial and time-series designs.
In addition to these classifications of studies, Deming 5 defined a distinction between analytical and enumerative studies which has proven to be fundamental to the science of improvement. Deming based his insight on the distinction between these two approaches that Walter Shewhart had made in 1939 as he helped develop measurement strategies for the then-emerging science of ‘quality control.’ 6 The difference between the two concepts lies in the extrapolation of the results that is intended, and in the target for action based on the inferences that are drawn.
A useful way to appreciate that difference is to contrast the inferences that can be made about the water sampled from two different natural sources ( figure 1 ). The enumerative approach is like the study of water from a pond. Because conditions in the bounded universe of the pond are essentially static over time, analyses of random samples taken from the pond at a given time can be used to estimate the makeup of the entire pond. Statistical methods, such as hypothesis testing and CIs, can be used to make decisions and define the precision of the estimates.

Environment in enumerative and analytical study. Internal validity diagram from Fletcher et al . 7
The analytical approach, in contrast, is like the study of water from a river. The river is constantly moving, and its physical properties are changing (eg, due to snow melt, changes in rainfall, dumping of pollutants). The properties of water in a sample from the river at any given time may not describe the river after the samples are taken and analysed. In fact, without repeated sampling over time, it is difficult to make predictions about water quality, since the river will not be the same river in the future as it was at the time of the sampling.
Deming first discussed these concepts in a 1942 paper, 8 as well as in his 1950 textbook, 9 and in a 1975 paper used the enumerative/analytical terminology to characterise specific study designs. 5 While most books on experimental design describe methods for the design and analysis of enumerative studies, Moen et al 10 describe methods for designing and learning from analytical studies. These methods are graphical and focus on prediction of future performance. The concept of analytical studies became a key element in Deming's ‘system of profound knowledge’ that serves as the intellectual foundation for improvement science. 11 The knowledge framework for the science of improvement, which combines elements of psychology, the Shewhart view of variation, the concept of systems, and the theory of knowledge, informs a number of key principles for the design and analysis of improvement studies:
- Knowledge about improvement begins and ends in experimental data but does not end in the data in which it begins.
- Observations, by themselves, do not constitute knowledge.
- Prediction requires theory regarding mechanisms of change and understanding of context.
- Random sampling from a population or universe (assumed by most statistical methods) is not possible when the population of interest is in the future.
- The conditions during studies for improvement will be different from the conditions under which the results will be used. The major source of uncertainty concerning their use is the difficulty of extrapolating study results to different contexts and under different conditions in the future.
- The wider the range of conditions included in an improvement study, the greater the degree of belief in the validity and generalisation of the conclusions.
The classification of studies into enumerative and analytical categories depends on the intended target for action as the result of the study:
- Enumerative studies assume that when actions are taken as the result of a study, they will be taken on the material in the study population or ‘frame’ that was sampled.
More specifically, the study universe in an enumerative study is the bounded group of items (eg, patients, clinics, providers, etc) possessing certain properties of interest. The universe is defined by a frame, a list of identifiable, tangible units that may be sampled and studied. Random selection methods are assumed in the statistical methods used for estimation, decision-making and drawing inferences in enumerative studies. Their aim is estimation about some aspect of the frame (such as a description, comparison or the existence of a cause–effect relationship) and the resulting actions taken on this particular frame. One feature of an enumerative study is that a 100% sample of the frame provides the complete answer to the questions posed by the study (given the methods of investigation and measurement). Statistical methods such as hypothesis tests, CIs and probability statements are appropriate to analyse and report data from enumerative studies. Estimating the infection rate in an intensive care unit for the last month is an example of a simple enumerative study.
- Analytical studies assume that the actions taken as a result of the study will be on the process or causal system that produced the frame studied, rather than the initial frame itself. The aim is to improve future performance.
In contrast to enumerative studies, an analytical study accepts as a given that when actions are taken on a system based on the results of a study, the conditions in that system will inevitably have changed. The aim of an analytical study is to enable prediction about how a change in a system will affect that system's future performance, or prediction as to which plans or strategies for future action on the system will be superior. For example, the task may be to choose among several different treatments for future patients, methods of collecting information or procedures for cleaning an operating room. Because the population of interest is open and continually shifts over time, random samples from that population cannot be obtained in analytical studies, and traditional statistical methods are therefore not useful. Rather, graphical methods of analysis and summary of the repeated samples reveal the trajectory of system behaviour over time, making it possible to predict future behaviour. Use of a Shewhart control chart to monitor and create learning to reduce infection rates in an intensive care unit is an example of a simple analytical study.
The following scenarios give examples to clarify the nature of these two types of studies.
Scenario 1: enumerative study—observation
To estimate how many days it takes new patients to see all primary care physicians contracted with a health plan, a researcher selected a random sample of 150 such physicians from the current active list and called each of their offices to schedule an appointment. The time to the next available appointment ranged from 0 to 180 days, with a mean of 38 days (95% CI 35.6 to 39.6).
This is an enumerative study, since results are intended to be used to estimate the waiting time for appointments with the plan's current population of primary care physicians.
Scenario 2: enumerative study—hypothesis generation
The researcher in scenario 1 noted that on occasion, she was offered an earlier visit with a nurse practitioner (NP) who worked with the physician being called. Additional information revealed that 20 of the 150 physicians in the study worked with one or more NPs. The next available appointment for the 130 physicians without an NP averaged 41 days (95% CI 39 to 43 days) and was 18 days (95% CI 18 to 26 days) for the 20 practices with NPs, a difference of 23 days (a 56% shorter mean waiting time).
This subgroup analysis suggested that the involvement of NPs helps to shorten waiting times, although it does not establish a cause–effect relationship, that is, it was a ‘hypothesis-generating’ study. In any event, this was clearly an enumerative study, since its results were to understand the impact of NPs on waiting times in the particular population of practices. Its results suggested that NPs might influence waiting times, but only for practices in this health plan during the time of the study. The study treated the conditions in the health plan as static, like those in a pond.
Scenario 3: enumerative study—comparison
To find out if administrative changes in a health plan had increased member satisfaction in access to care, the customer service manager replicated a phone survey he had conducted a year previously, using a random sample of 300 members. The percentage of patients who were satisfied with access had increased from 48.7% to 60.7% (Fisher exact test, p<0.004).
This enumerative comparison study was used to estimate the impact of the improvement work during the last year on the members in the plan. Attributing the increase in satisfaction to the improvement work assumes that other conditions in the study frame were static.
Scenario 4: analytical study—learning with a Shewhart chart
Each primary care clinic in a health plan reported its ‘time until the third available appointment’ twice a month, which allowed the quality manager to plot the mean waiting time for all of the clinics on Shewhart charts. Waiting times had been stable for a 12-month period through August, but the manager then noted a special cause (increase in waiting time) in September. On stratifying the data by region, she found that the special cause resulted from increases in waiting time in the Northeast region. Discussion with the regional manager revealed a shortage of primary care physicians in this region, which was predicted to become worse in the next quarter. Making some temporary assignments and increasing physician recruiting efforts resulted in stabilisation of this measure.
Documenting common and special cause variation in measures of interest through the use of Shewhart charts and run charts based on judgement samples is probably the simplest and commonest type of analytical study in healthcare. Such charts, when stable, provide a rational basis for predicting future performance.
Scenario 5: analytical study—establishing a cause–effect relationship
The researcher mentioned in scenarios 1 and 2 planned a study to test the existence of a cause–effect relationship between the inclusion of NPs in primary care offices and waiting time for new patient appointments. The variation in patient characteristics in this health plan appeared to be great enough to make the study results useful to other organisations. For the study, she recruited about 100 of the plan's practices that currently did not use NPs, and obtained funding to facilitate hiring NPs in up to 50 of those practices.
The researcher first explored the theories on mechanisms by which the incorporation of NPs into primary care clinics could reduce waiting times. Using important contextual variables relevant to these mechanisms (practice size, complexity, use of information technology and urban vs rural location), she then developed a randomised block, time-series study design. The study had the power to detect an effect of a mean waiting time of 5 days or more overall, and 10 days for the major subgroups defined by levels of the contextual variables. Since the baseline waiting time for appointments varied substantially across practices, she used the baseline as a covariate.
After completing the study, she analysed data from baseline and postintervention periods using stratified run charts and Shewhart charts, including the raw measures and measures adjusted for important covariates and effects of contextual variables. Overall waiting times decreased 12 days more in practices that included NPs than they did in control practices. Importantly, the subgroup analyses according to contextual variables revealed conditions under which the use of NPs would not be predicted to lead to reductions in waiting times. For example, practices with short baseline waiting times showed little or no improvement by employing NPs. She published the results in a leading health research journal.
This was an analytical study because the intent was to apply the learning from the study to future staffing plans in the health plan. She also published the study, so its results would be useful to primary care practices outside the health plan.
Scenario 6: analytical study—implementing improvement
The quality-improvement manager in another health plan wanted to expand the use of NPs in the plan's primary care practices, because published research had shown a reduction in waiting times for practices with NPs. Two practices in his plan already employed NPs. In one of these practices, Shewhart charts of waiting time by month showed a stable process averaging 10 days during the last 2 years. Waiting time averaged less than 7 days in the second practice, but a period when one of the physicians left the practice was associated with special causes.
The quality manager created a collaborative among the plan's primary care practices to learn how to optimise the use of NPs. Physicians in the two sites that employed NPs served as subject matter experts for the collaborative. In addition to making NPs part of their care teams, participating practices monitored appointment supply and demand, and tested other changes designed to optimise response to patient needs. Thirty sites in the plan voluntarily joined the collaborative and hired NPs. After 6 months, Shewhart charts indicated that waiting times in 25 of the 30 sites had been reduced to less than 7 days. Because waiting times in these practices had been stable over a considerable period of time, the manager predicted that future patients would continue to experience reduced times for appointments. The quality manger began to focus on a follow-up collaborative among the backlog of 70 practices that wanted to join.
This project was clearly an analytical study, since its aim was specifically to improve future waiting-time performance for participating sites and other primary care offices in the plan. Moreover, it focused on learning about the mechanisms through which contextual factors affect the impact of NPs on primary care office functions, under practice conditions that (like those in a river) will inevitably change over time.
Statistical theory in enumerative studies is used to describe the precision of estimates and the validity of hypotheses for the population studied. But since these statistical methods provide no support for extrapolation of the results outside the population that was studied, the subject experts must rely on their understanding of the mechanisms in place to extend results outside the population.
In analytical studies, the standard error of a statistic does not address the most important source of uncertainty, namely, the change in study conditions in the future. Although analytical studies need to take into account the uncertainty due to sampling, as in enumerative studies, the attributes of the study design and analysis of the data primarily deal with the uncertainty resulting from extrapolation to the future (generalisation to the conditions in future time periods). The methods used in analytical studies encourage the exploration of mechanisms through multifactor designs, contextual variables introduced through blocking and replication over time.
Prior stability of a system (as observed in graphic displays of repeated sampling over time, according to Shewhart's methods) increases belief in the results of an analytical study, but stable processes in the past do not guarantee constant system behaviour in the future. The next data point from the future is the most important on a graph of performance. Extrapolation of system behaviour to future times therefore still depends on input from subject experts who are familiar with mechanisms of the system of interest, as well as the important contextual issues. Generalisation is inherently difficult in all studies because ‘whereas the problems of internal validity are solvable within the limits of the logic of probability statistics, the problems of external validity are not logically solvable in any neat, conclusive way’ 3 (p. 17).
The diverse activities commonly referred to as healthcare improvement 12 are all designed to change the behaviour of systems over time, as reflected in the principle that ‘not all change is improvement, but all improvement is change.’ The conditions in the unbounded systems into which improvement interventions are introduced will therefore be different in the future from those in effect at the time the intervention is studied. Since the results of improvement studies are used to predict future system behaviour, such studies clearly belong to the Deming category of analytical studies. Quality improvement studies therefore need to incorporate repeated measurements over time, as well as testing under a wide range of conditions (2, 3 and 10). The ‘gold standard’ of analytical studies is satisfactory prediction over time.
Conclusions and recommendations
In light of these considerations, some important principles for drawing inferences from improvement studies include 10 :
- The analysis of data, interpretation of that analysis and actions taken as a result of the study should be closely tied to the current knowledge of experts about mechanisms of change in the relevant area. They can often use the study to discover, understand and evaluate the underlying mechanisms.
- The conditions of the study will be different from the future conditions under which the results will be used. Assessment by experts of the magnitude of this difference and its potential impact on future events should be an integral part of the interpretation of the results of the intervention.
- Show all the data before aggregation or summary.
- Plot the outcome data in the order in which the tests of change were conducted and annotate with information on the interventions.
- Use graphical displays to assess how much of the variation in the data can be explained by factors that were deliberately changed.
- Rearrange and subgroup the data to study other sources of variation (background and contextual variables).
- Summarise the results of the study with appropriate graphical displays.
Because these principles reflect the fundamental nature of improvement—taking action to change performance, over time, and under changing conditions—their application helps to bring clarity and rigour to improvement science.
Acknowledgments
The author is grateful to F Davidoff and P Batalden for their input to earlier versions of this paper.
Competing interests: None.
Provenance and peer review: Not commissioned; externally peer reviewed.

Qualitative Data Analysis Methods 101:
The “big 6” methods + examples.
By: Kerryn Warren (PhD) | Reviewed By: Eunice Rautenbach (D.Tech) | May 2020 (Updated April 2023)
Qualitative data analysis methods. Wow, that’s a mouthful.
If you’re new to the world of research, qualitative data analysis can look rather intimidating. So much bulky terminology and so many abstract, fluffy concepts. It certainly can be a minefield!
Don’t worry – in this post, we’ll unpack the most popular analysis methods , one at a time, so that you can approach your analysis with confidence and competence – whether that’s for a dissertation, thesis or really any kind of research project.
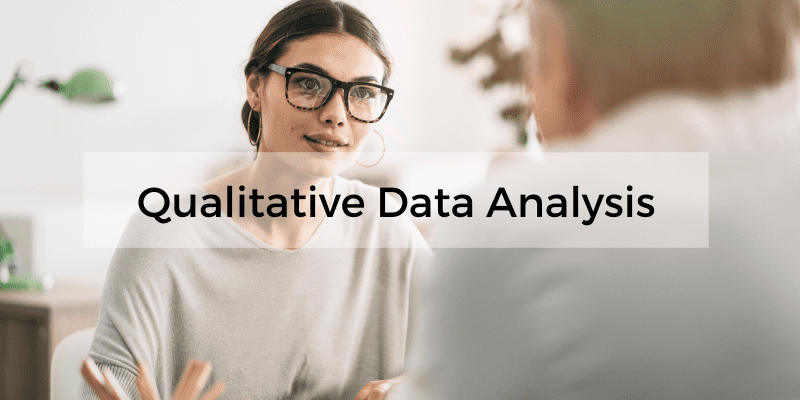
What (exactly) is qualitative data analysis?
To understand qualitative data analysis, we need to first understand qualitative data – so let’s step back and ask the question, “what exactly is qualitative data?”.
Qualitative data refers to pretty much any data that’s “not numbers” . In other words, it’s not the stuff you measure using a fixed scale or complex equipment, nor do you analyse it using complex statistics or mathematics.
So, if it’s not numbers, what is it?
Words, you guessed? Well… sometimes , yes. Qualitative data can, and often does, take the form of interview transcripts, documents and open-ended survey responses – but it can also involve the interpretation of images and videos. In other words, qualitative isn’t just limited to text-based data.
So, how’s that different from quantitative data, you ask?
Simply put, qualitative research focuses on words, descriptions, concepts or ideas – while quantitative research focuses on numbers and statistics . Qualitative research investigates the “softer side” of things to explore and describe , while quantitative research focuses on the “hard numbers”, to measure differences between variables and the relationships between them. If you’re keen to learn more about the differences between qual and quant, we’ve got a detailed post over here .
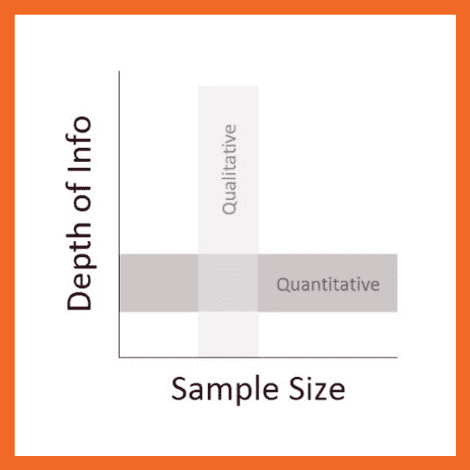
So, qualitative analysis is easier than quantitative, right?
Not quite. In many ways, qualitative data can be challenging and time-consuming to analyse and interpret. At the end of your data collection phase (which itself takes a lot of time), you’ll likely have many pages of text-based data or hours upon hours of audio to work through. You might also have subtle nuances of interactions or discussions that have danced around in your mind, or that you scribbled down in messy field notes. All of this needs to work its way into your analysis.
Making sense of all of this is no small task and you shouldn’t underestimate it. Long story short – qualitative analysis can be a lot of work! Of course, quantitative analysis is no piece of cake either, but it’s important to recognise that qualitative analysis still requires a significant investment in terms of time and effort.
Need a helping hand?
In this post, we’ll explore qualitative data analysis by looking at some of the most common analysis methods we encounter. We’re not going to cover every possible qualitative method and we’re not going to go into heavy detail – we’re just going to give you the big picture. That said, we will of course includes links to loads of extra resources so that you can learn more about whichever analysis method interests you.
Without further delay, let’s get into it.
The “Big 6” Qualitative Analysis Methods
There are many different types of qualitative data analysis, all of which serve different purposes and have unique strengths and weaknesses . We’ll start by outlining the analysis methods and then we’ll dive into the details for each.
The 6 most popular methods (or at least the ones we see at Grad Coach) are:
- Content analysis
- Narrative analysis
- Discourse analysis
- Thematic analysis
- Grounded theory (GT)
- Interpretive phenomenological analysis (IPA)
Let’s take a look at each of them…
QDA Method #1: Qualitative Content Analysis
Content analysis is possibly the most common and straightforward QDA method. At the simplest level, content analysis is used to evaluate patterns within a piece of content (for example, words, phrases or images) or across multiple pieces of content or sources of communication. For example, a collection of newspaper articles or political speeches.
With content analysis, you could, for instance, identify the frequency with which an idea is shared or spoken about – like the number of times a Kardashian is mentioned on Twitter. Or you could identify patterns of deeper underlying interpretations – for instance, by identifying phrases or words in tourist pamphlets that highlight India as an ancient country.
Because content analysis can be used in such a wide variety of ways, it’s important to go into your analysis with a very specific question and goal, or you’ll get lost in the fog. With content analysis, you’ll group large amounts of text into codes , summarise these into categories, and possibly even tabulate the data to calculate the frequency of certain concepts or variables. Because of this, content analysis provides a small splash of quantitative thinking within a qualitative method.
Naturally, while content analysis is widely useful, it’s not without its drawbacks . One of the main issues with content analysis is that it can be very time-consuming , as it requires lots of reading and re-reading of the texts. Also, because of its multidimensional focus on both qualitative and quantitative aspects, it is sometimes accused of losing important nuances in communication.
Content analysis also tends to concentrate on a very specific timeline and doesn’t take into account what happened before or after that timeline. This isn’t necessarily a bad thing though – just something to be aware of. So, keep these factors in mind if you’re considering content analysis. Every analysis method has its limitations , so don’t be put off by these – just be aware of them ! If you’re interested in learning more about content analysis, the video below provides a good starting point.
QDA Method #2: Narrative Analysis
As the name suggests, narrative analysis is all about listening to people telling stories and analysing what that means . Since stories serve a functional purpose of helping us make sense of the world, we can gain insights into the ways that people deal with and make sense of reality by analysing their stories and the ways they’re told.
You could, for example, use narrative analysis to explore whether how something is being said is important. For instance, the narrative of a prisoner trying to justify their crime could provide insight into their view of the world and the justice system. Similarly, analysing the ways entrepreneurs talk about the struggles in their careers or cancer patients telling stories of hope could provide powerful insights into their mindsets and perspectives . Simply put, narrative analysis is about paying attention to the stories that people tell – and more importantly, the way they tell them.
Of course, the narrative approach has its weaknesses , too. Sample sizes are generally quite small due to the time-consuming process of capturing narratives. Because of this, along with the multitude of social and lifestyle factors which can influence a subject, narrative analysis can be quite difficult to reproduce in subsequent research. This means that it’s difficult to test the findings of some of this research.
Similarly, researcher bias can have a strong influence on the results here, so you need to be particularly careful about the potential biases you can bring into your analysis when using this method. Nevertheless, narrative analysis is still a very useful qualitative analysis method – just keep these limitations in mind and be careful not to draw broad conclusions . If you’re keen to learn more about narrative analysis, the video below provides a great introduction to this qualitative analysis method.
QDA Method #3: Discourse Analysis
Discourse is simply a fancy word for written or spoken language or debate . So, discourse analysis is all about analysing language within its social context. In other words, analysing language – such as a conversation, a speech, etc – within the culture and society it takes place. For example, you could analyse how a janitor speaks to a CEO, or how politicians speak about terrorism.
To truly understand these conversations or speeches, the culture and history of those involved in the communication are important factors to consider. For example, a janitor might speak more casually with a CEO in a company that emphasises equality among workers. Similarly, a politician might speak more about terrorism if there was a recent terrorist incident in the country.
So, as you can see, by using discourse analysis, you can identify how culture , history or power dynamics (to name a few) have an effect on the way concepts are spoken about. So, if your research aims and objectives involve understanding culture or power dynamics, discourse analysis can be a powerful method.
Because there are many social influences in terms of how we speak to each other, the potential use of discourse analysis is vast . Of course, this also means it’s important to have a very specific research question (or questions) in mind when analysing your data and looking for patterns and themes, or you might land up going down a winding rabbit hole.
Discourse analysis can also be very time-consuming as you need to sample the data to the point of saturation – in other words, until no new information and insights emerge. But this is, of course, part of what makes discourse analysis such a powerful technique. So, keep these factors in mind when considering this QDA method. Again, if you’re keen to learn more, the video below presents a good starting point.
QDA Method #4: Thematic Analysis
Thematic analysis looks at patterns of meaning in a data set – for example, a set of interviews or focus group transcripts. But what exactly does that… mean? Well, a thematic analysis takes bodies of data (which are often quite large) and groups them according to similarities – in other words, themes . These themes help us make sense of the content and derive meaning from it.
Let’s take a look at an example.
With thematic analysis, you could analyse 100 online reviews of a popular sushi restaurant to find out what patrons think about the place. By reviewing the data, you would then identify the themes that crop up repeatedly within the data – for example, “fresh ingredients” or “friendly wait staff”.
So, as you can see, thematic analysis can be pretty useful for finding out about people’s experiences , views, and opinions . Therefore, if your research aims and objectives involve understanding people’s experience or view of something, thematic analysis can be a great choice.
Since thematic analysis is a bit of an exploratory process, it’s not unusual for your research questions to develop , or even change as you progress through the analysis. While this is somewhat natural in exploratory research, it can also be seen as a disadvantage as it means that data needs to be re-reviewed each time a research question is adjusted. In other words, thematic analysis can be quite time-consuming – but for a good reason. So, keep this in mind if you choose to use thematic analysis for your project and budget extra time for unexpected adjustments.
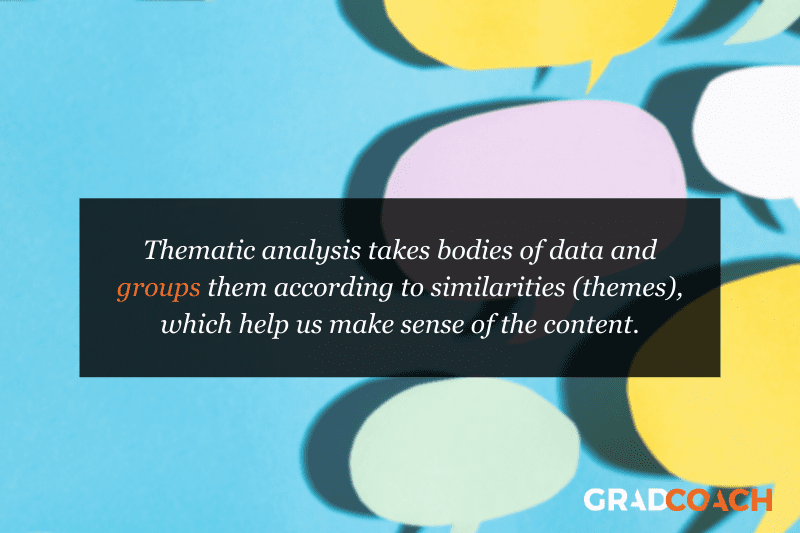
QDA Method #5: Grounded theory (GT)
Grounded theory is a powerful qualitative analysis method where the intention is to create a new theory (or theories) using the data at hand, through a series of “ tests ” and “ revisions ”. Strictly speaking, GT is more a research design type than an analysis method, but we’ve included it here as it’s often referred to as a method.
What’s most important with grounded theory is that you go into the analysis with an open mind and let the data speak for itself – rather than dragging existing hypotheses or theories into your analysis. In other words, your analysis must develop from the ground up (hence the name).
Let’s look at an example of GT in action.
Assume you’re interested in developing a theory about what factors influence students to watch a YouTube video about qualitative analysis. Using Grounded theory , you’d start with this general overarching question about the given population (i.e., graduate students). First, you’d approach a small sample – for example, five graduate students in a department at a university. Ideally, this sample would be reasonably representative of the broader population. You’d interview these students to identify what factors lead them to watch the video.
After analysing the interview data, a general pattern could emerge. For example, you might notice that graduate students are more likely to read a post about qualitative methods if they are just starting on their dissertation journey, or if they have an upcoming test about research methods.
From here, you’ll look for another small sample – for example, five more graduate students in a different department – and see whether this pattern holds true for them. If not, you’ll look for commonalities and adapt your theory accordingly. As this process continues, the theory would develop . As we mentioned earlier, what’s important with grounded theory is that the theory develops from the data – not from some preconceived idea.
So, what are the drawbacks of grounded theory? Well, some argue that there’s a tricky circularity to grounded theory. For it to work, in principle, you should know as little as possible regarding the research question and population, so that you reduce the bias in your interpretation. However, in many circumstances, it’s also thought to be unwise to approach a research question without knowledge of the current literature . In other words, it’s a bit of a “chicken or the egg” situation.
Regardless, grounded theory remains a popular (and powerful) option. Naturally, it’s a very useful method when you’re researching a topic that is completely new or has very little existing research about it, as it allows you to start from scratch and work your way from the ground up .
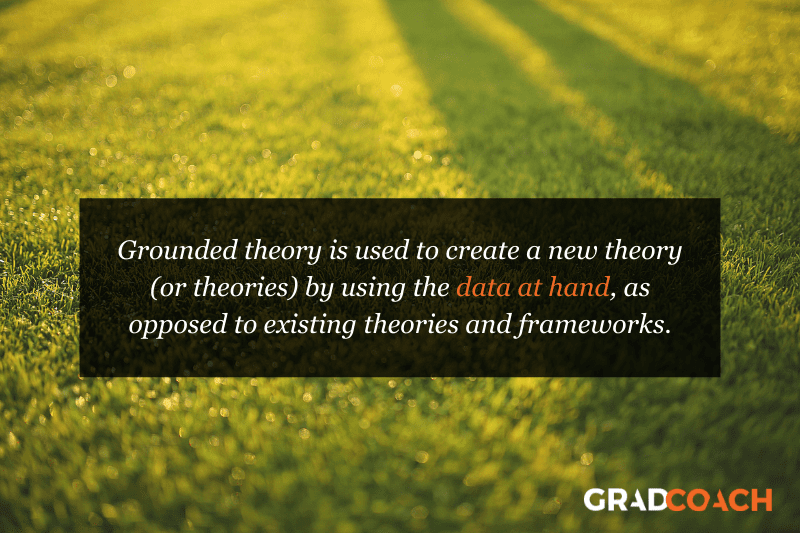
QDA Method #6: Interpretive Phenomenological Analysis (IPA)
Interpretive. Phenomenological. Analysis. IPA . Try saying that three times fast…
Let’s just stick with IPA, okay?
IPA is designed to help you understand the personal experiences of a subject (for example, a person or group of people) concerning a major life event, an experience or a situation . This event or experience is the “phenomenon” that makes up the “P” in IPA. Such phenomena may range from relatively common events – such as motherhood, or being involved in a car accident – to those which are extremely rare – for example, someone’s personal experience in a refugee camp. So, IPA is a great choice if your research involves analysing people’s personal experiences of something that happened to them.
It’s important to remember that IPA is subject – centred . In other words, it’s focused on the experiencer . This means that, while you’ll likely use a coding system to identify commonalities, it’s important not to lose the depth of experience or meaning by trying to reduce everything to codes. Also, keep in mind that since your sample size will generally be very small with IPA, you often won’t be able to draw broad conclusions about the generalisability of your findings. But that’s okay as long as it aligns with your research aims and objectives.
Another thing to be aware of with IPA is personal bias . While researcher bias can creep into all forms of research, self-awareness is critically important with IPA, as it can have a major impact on the results. For example, a researcher who was a victim of a crime himself could insert his own feelings of frustration and anger into the way he interprets the experience of someone who was kidnapped. So, if you’re going to undertake IPA, you need to be very self-aware or you could muddy the analysis.
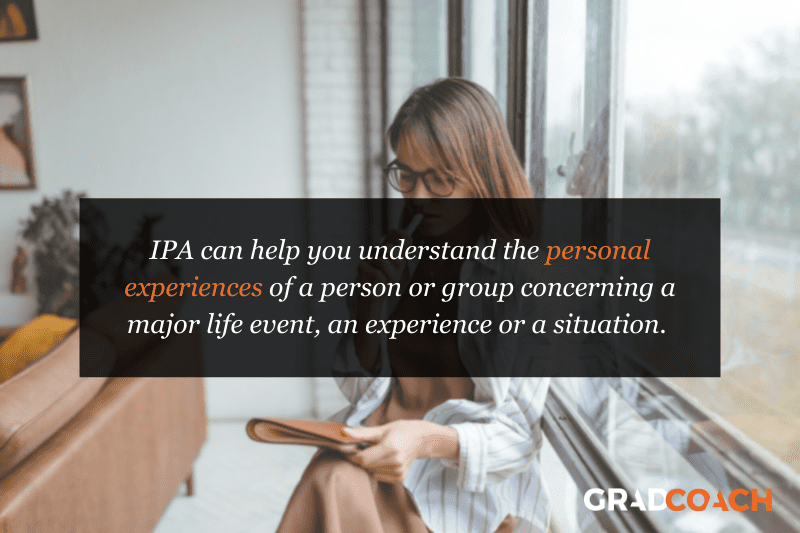
How to choose the right analysis method
In light of all of the qualitative analysis methods we’ve covered so far, you’re probably asking yourself the question, “ How do I choose the right one? ”
Much like all the other methodological decisions you’ll need to make, selecting the right qualitative analysis method largely depends on your research aims, objectives and questions . In other words, the best tool for the job depends on what you’re trying to build. For example:
- Perhaps your research aims to analyse the use of words and what they reveal about the intention of the storyteller and the cultural context of the time.
- Perhaps your research aims to develop an understanding of the unique personal experiences of people that have experienced a certain event, or
- Perhaps your research aims to develop insight regarding the influence of a certain culture on its members.
As you can probably see, each of these research aims are distinctly different , and therefore different analysis methods would be suitable for each one. For example, narrative analysis would likely be a good option for the first aim, while grounded theory wouldn’t be as relevant.
It’s also important to remember that each method has its own set of strengths, weaknesses and general limitations. No single analysis method is perfect . So, depending on the nature of your research, it may make sense to adopt more than one method (this is called triangulation ). Keep in mind though that this will of course be quite time-consuming.
As we’ve seen, all of the qualitative analysis methods we’ve discussed make use of coding and theme-generating techniques, but the intent and approach of each analysis method differ quite substantially. So, it’s very important to come into your research with a clear intention before you decide which analysis method (or methods) to use.
Start by reviewing your research aims , objectives and research questions to assess what exactly you’re trying to find out – then select a qualitative analysis method that fits. Never pick a method just because you like it or have experience using it – your analysis method (or methods) must align with your broader research aims and objectives.
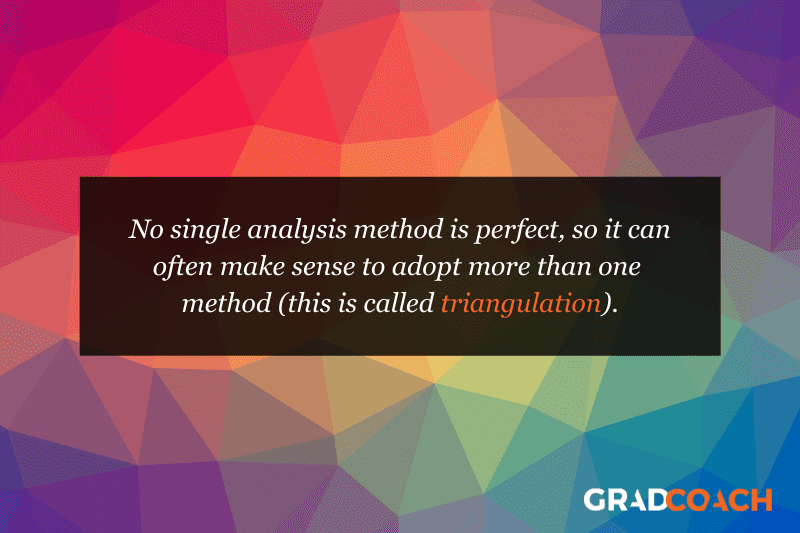
Let’s recap on QDA methods…
In this post, we looked at six popular qualitative data analysis methods:
- First, we looked at content analysis , a straightforward method that blends a little bit of quant into a primarily qualitative analysis.
- Then we looked at narrative analysis , which is about analysing how stories are told.
- Next up was discourse analysis – which is about analysing conversations and interactions.
- Then we moved on to thematic analysis – which is about identifying themes and patterns.
- From there, we went south with grounded theory – which is about starting from scratch with a specific question and using the data alone to build a theory in response to that question.
- And finally, we looked at IPA – which is about understanding people’s unique experiences of a phenomenon.
Of course, these aren’t the only options when it comes to qualitative data analysis, but they’re a great starting point if you’re dipping your toes into qualitative research for the first time.
If you’re still feeling a bit confused, consider our private coaching service , where we hold your hand through the research process to help you develop your best work.
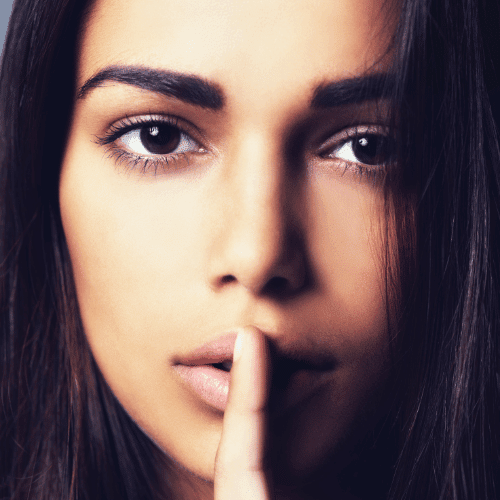
Psst... there’s more!
This post was based on one of our popular Research Bootcamps . If you're working on a research project, you'll definitely want to check this out ...
87 Comments
This has been very helpful. Thank you.
Thank you madam,
Thank you so much for this information
I wonder it so clear for understand and good for me. can I ask additional query?
Very insightful and useful
Good work done with clear explanations. Thank you.
Thanks so much for the write-up, it’s really good.
Thanks madam . It is very important .
thank you very good
Great presentation
This has been very well explained in simple language . It is useful even for a new researcher.
Great to hear that. Good luck with your qualitative data analysis, Pramod!
This is very useful information. And it was very a clear language structured presentation. Thanks a lot.
Thank you so much.
very informative sequential presentation
Precise explanation of method.
Hi, may we use 2 data analysis methods in our qualitative research?
Thanks for your comment. Most commonly, one would use one type of analysis method, but it depends on your research aims and objectives.
You explained it in very simple language, everyone can understand it. Thanks so much.
Thank you very much, this is very helpful. It has been explained in a very simple manner that even a layman understands
Thank nicely explained can I ask is Qualitative content analysis the same as thematic analysis?
Thanks for your comment. No, QCA and thematic are two different types of analysis. This article might help clarify – https://onlinelibrary.wiley.com/doi/10.1111/nhs.12048
This is my first time to come across a well explained data analysis. so helpful.
I have thoroughly enjoyed your explanation of the six qualitative analysis methods. This is very helpful. Thank you!
Thank you very much, this is well explained and useful
i need a citation of your book.
Thanks a lot , remarkable indeed, enlighting to the best
Hi Derek, What other theories/methods would you recommend when the data is a whole speech?
Keep writing useful artikel.
It is important concept about QDA and also the way to express is easily understandable, so thanks for all.
Thank you, this is well explained and very useful.
Very helpful .Thanks.
Hi there! Very well explained. Simple but very useful style of writing. Please provide the citation of the text. warm regards
The session was very helpful and insightful. Thank you
This was very helpful and insightful. Easy to read and understand
As a professional academic writer, this has been so informative and educative. Keep up the good work Grad Coach you are unmatched with quality content for sure.
Keep up the good work Grad Coach you are unmatched with quality content for sure.
Its Great and help me the most. A Million Thanks you Dr.
It is a very nice work
Very insightful. Please, which of this approach could be used for a research that one is trying to elicit students’ misconceptions in a particular concept ?
This is Amazing and well explained, thanks
great overview
What do we call a research data analysis method that one use to advise or determining the best accounting tool or techniques that should be adopted in a company.
Informative video, explained in a clear and simple way. Kudos
Waoo! I have chosen method wrong for my data analysis. But I can revise my work according to this guide. Thank you so much for this helpful lecture.
This has been very helpful. It gave me a good view of my research objectives and how to choose the best method. Thematic analysis it is.
Very helpful indeed. Thanku so much for the insight.
This was incredibly helpful.
Very helpful.
very educative
Nicely written especially for novice academic researchers like me! Thank you.
choosing a right method for a paper is always a hard job for a student, this is a useful information, but it would be more useful personally for me, if the author provide me with a little bit more information about the data analysis techniques in type of explanatory research. Can we use qualitative content analysis technique for explanatory research ? or what is the suitable data analysis method for explanatory research in social studies?
that was very helpful for me. because these details are so important to my research. thank you very much
I learnt a lot. Thank you
Relevant and Informative, thanks !
Well-planned and organized, thanks much! 🙂
I have reviewed qualitative data analysis in a simplest way possible. The content will highly be useful for developing my book on qualitative data analysis methods. Cheers!
Clear explanation on qualitative and how about Case study
This was helpful. Thank you
This was really of great assistance, it was just the right information needed. Explanation very clear and follow.
Wow, Thanks for making my life easy
This was helpful thanks .
Very helpful…. clear and written in an easily understandable manner. Thank you.
This was so helpful as it was easy to understand. I’m a new to research thank you so much.
so educative…. but Ijust want to know which method is coding of the qualitative or tallying done?
Thank you for the great content, I have learnt a lot. So helpful
precise and clear presentation with simple language and thank you for that.
very informative content, thank you.
You guys are amazing on YouTube on this platform. Your teachings are great, educative, and informative. kudos!
Brilliant Delivery. You made a complex subject seem so easy. Well done.
Beautifully explained.
Thanks a lot
Is there a video the captures the practical process of coding using automated applications?
Thanks for the comment. We don’t recommend using automated applications for coding, as they are not sufficiently accurate in our experience.
content analysis can be qualitative research?
THANK YOU VERY MUCH.
Thank you very much for such a wonderful content
do you have any material on Data collection
What a powerful explanation of the QDA methods. Thank you.
Great explanation both written and Video. i have been using of it on a day to day working of my thesis project in accounting and finance. Thank you very much for your support.
very helpful, thank you so much
The tutorial is useful. I benefited a lot.
This is an eye opener for me and very informative, I have used some of your guidance notes on my Thesis, I wonder if you can assist with your 1. name of your book, year of publication, topic etc., this is for citing in my Bibliography,
I certainly hope to hear from you
Submit a Comment Cancel reply
Your email address will not be published. Required fields are marked *
Save my name, email, and website in this browser for the next time I comment.
- Print Friendly

An official website of the United States government
Here’s how you know
Official websites use .gov A .gov website belongs to an official government organization in the United States.
Secure .gov websites use HTTPS A lock ( Lock A locked padlock ) or https:// means you’ve safely connected to the .gov website. Share sensitive information only on official, secure websites.
JavaScript appears to be disabled on this computer. Please click here to see any active alerts .
- Analytical Examples
This section presents five (5) examples illustrating the use of data analysis to support different types of evidence. Each example provides details about the analysis technique used and the type of evidence supported. These include:
- Spatial Co-occurrence with Regional Reference Sites (Example 1).
- Verified Prediction: Predicting Environmental Conditions from Biological Observations (Example 2).
- Stressor-Response Relationships from Field Observations (Example 3).
- Stressor-Response Relationships from Laboratory Studies (Example 4).
- Verified Prediction with Traits (Example 5).
Example 1. Spatial Co-occurrence with Regional Reference Sites
Introduction, analysis and results, how do i score this evidence.
We would like to determine whether stream temperatures observed at an Oregon test site are higher than those at regional reference sites. If temperatures at the test site are higher than reference expectations, then we can conclude that increased temperature spatially co-occurs with the observed impairment. Conversely, temperatures at the test site that are comparable to temperatures at regional reference sites would suggest that increased temperature does not spatially co-occur with the observed impairment.
Analytical Techniques Used
- Scatterplots
- Regression Analysis
Controlling for Natural Variability
Type of evidence supported.
- Spatial/Temporal Co-occurrence
The Oregon Department of Environment Quality (ORDEQ) deployed continuous temperature monitors in streams from 1997-2002. These temperature monitors recorded hourly temperature measurement which were then summarized as seven day average maximum temperatures in degrees C (7DAMT). Sites were also characterized by the geographic location (latitude and longitude), elevation, and catchment area. Reference sites were designated in Oregon based on land use characteristics.
Scatter plots are first used to examine the variation of stream temperature with different natural factors. The factors that are chosen (e.g., elevation, geographic location) must not be associated with local human activities. This initial data exploration suggests that stream temperature in reference sites are inversely related with both elevation and latitude (Figure 1). Next, regression analysis is used to model stream temperature as a function of elevation and latitude.

Both elevation and latitude are statistically significant (p < 0.05) predictors of stream temperature. The model explains approximately half of the overall variability in stream temperature. This model can be used to predict the reference expectations for stream temperature at other sites. That is, the reference expectation for temperature can be calculated as follows:
t = 76.6 - 0.0019E - 1.36L where t is the stream temperature, E is the elevation of the site in feet, and L is the latitude of the site in decimal degrees.
Now, suppose a biologically impaired test site of interest is located at a latitude of 43 degrees N and an elevation of 1000 ft. We monitored stream temperature at this site and found that the seven day average maximum temperature at the site was 22 °C. Temperature is listed as a candidate cause of impairment at this site, and so we would like to know whether stream temperature at the site is elevated relative to the regional reference conditions. The reference expectation for stream temperature can be predicted as follows,
t = 76.6 - 0.0019(1000) - 1.36(43) which gives a predicted reference temperature of 16.4 degrees. Most statistical software will also provide prediction intervals at a specified probability. For this case, 95% prediction intervals around the mean value are 11.4 and 21.4 degrees. Hence, the observed temperature is greater than temperatures we would expect for 95% of reference samples collected at the same elevation and latitude, suggesting that stream temperature is indeed elevated at the test site. We would conclude that at this test site, elevated stream temperature co-occurs with the biological impairment.
The CADStat Regression Prediction tool performs all of these calculations, and also determines whether conditions at test sites are within the range of experience of the set of reference sites.
Elevated temperatures co-occurs with the biological impairment so we would score this evidence as +.
Example 2. Verified Prediction: Predicting Environmental Conditions from Biological Observations
Inference model development and validation, site assessment.
We would like to determine whether observed changes in the macroinvertebrate assemblage composition at a test site in Oregon is consistent with a hypothesis that temperature has increased at the site. That is, if increased temperature is a stressor at the test site, we predict that the temperature inferred from the impaired macroinvertebrate assemblage is higher than expected. For this example, we establish our expectations for the inferred temperature using a set of regional reference sites.
- Predicting Environmental Conditions from Biological Observations
- Verified Predictions
Macroinvertebrate samples and temperature measurements were collected from small streams across the western United States by the U.S. EPA Environmental Monitoring and Assessment Program.
The Oregon Department of Environment Quality (ORDEQ) deployed continuous temperature monitors in streams from 1997-2002. These temperature monitors recorded hourly temperature measurements that were summarized as seven-day average maximum temperatures (7DAMT). Macroinvertebrate samples were also collected from these sites. Sites were characterized by the geographic location (latitude and longitude), elevation, and catchment area. Reference sites were designated in Oregon based on land use characteristics.

The accuracy with which the EMAP models predicted Oregon stream temperatures was assessed by plotting temperature inferred from the macroinvertebrate assemblage versus directly measured mean temperature (Figure 2). Agreement between inferred and directly measured temperatures was strong.
- See Spatial Co-occurrence with Regional Reference Sites
The factors that are chosen for the predictive model (e.g., elevation, geographic location) must not be associated with human activities. This initial data exploration suggested that stream temperature in reference sites varies with both elevation and latitude (Figure 3).

where t i is the stream temperature, E is the elevation of the site in feet, and L is the latitude of the site in decimal degrees.
Since the inference model seemed to provide accurate predictions of stream temperature, inferred temperature can be used to inform the verified prediction type of evidence. That is, we hypothesize that if temperature is the cause of impairment then temperatures inferred from the impaired macroinvertebrate assemblage will be higher than expected.
At the biologically impaired test site of interest we collected a macroinvertebrate sample and used the EMAP inference models to infer temperature at the test site as 21°C based on the macroinvertebrate assemblage. The biologically impaired site is located at an elevation of 1000 feet and latitude of 43° North. The expected inferred stream temperature at the site is predicted using the regression relationship developed from regional reference conditions,
t i = 50.3 - 0.0013 (1000) - 0.82( 43)
which gives a predicted reference inferred temperature of 13.7°C. 95% prediction intervals around this mean value are 10.5°C and 17.2°C, so the EMAP inferred temperature of 21°C, based on the collected macroinvertebrate assemblage, is well outside the predicted range of 95% of inferred temperatures at similar reference sites. This finding suggests that inferred stream temperature is indeed elevated at the test site. Hence, the macroinvertebrate assemblage at the test site is one that is characteristic of much warmer streams than we would expect for a stream at this elevation and latitude. At this test site, we have verified our prediction that the observed macroinvertebrate assemblage is consistent with temperatures being higher than expected.
The CADStat PECBO and Regression Prediction tools perform all the calculations described in this example.
Predictions of increased biologically-inferred temperatures have been verified (+).
- Yuan LL (2007) Maximum likelihood method for predicting environmental conditions from assemblage composition: The R package bio.infer. Journal of Statistical Software 22: Article 3.
Example 3. Stressor-Response Relationships from Field Observations
We would like to determine whether water quality variables in Long Creek, Maine (U.S. EPA 2007) are associated with three observed changes in the aquatic invertebrate community relative to the reference stream: a decrease in Ephemeroptera, Plecoptera and Trichoptera (EPT) richness; an increase in percent non-insect taxa; and a shift towards increased pollution tolerance, estimated using Hilsenhoff's Biotic Index (HBI) (Hilsenhoff 1987, 1988).
- Causal Analysis of Biological Impairment in Long Creek
- Correlation Analysis
- Stressor-Response Relationships from the Field
In this example, we present analyses relevant to two candidate causes, ionic strength (measured using specific conductivity), and zinc. If specific conductivity (or zinc) is not associated with the biological responses in the expected direction, this evidence would weaken the argument for ionic strength (or zinc) being a cause of the observed biological changes. Conversely, if specific conductivity (or zinc) is associated with the biological responses in the expected direction, this evidence would somewhat support the argument that ionic strength (or zinc) is the cause of the observed changes.
These associations can provide only weak support for a causal argument because other stressors may be correlated with increased conductivity (or zinc), and are not controlled for in this analysis. For this reason, it is important to conduct this analysis for as many of the candidate causes as possible.
Biological and water chemistry data from 8 sites along Long Creek and a similar but unimpaired reference stream, are used in this example.
Biological metrics were calculated from macroinvertebrate rockbag samples deployed throughout the study area beginning August 5-6, 1999, for a period of 32 days, following standard Maine Department of Environmental Protection (MEDEP) protocol (Davies and Tsomides 2002).
Water chemistry measurements of conductivity and zinc were made from baseflow water samples collected by MEDEP on three days in August 2000. Methods and analyses are described in MEDEP (2002). Here, the analysts assume that the differences in the collection dates for biological samples (1999) and for water chemistry samples (2000) did not affect observed relationships. Ideally, additional data would be collected as a follow-up to validate this assumption.
The data were analyzed using scatter plots (Figure 4). The project team interpreted the scatter plots by looking for linear and curvilinear trends in the data. Because only one data point from each site was available, the plots were not used to make judgments about individual sites or stream reaches. Instead, the plots were used to characterize trends across the two watersheds.
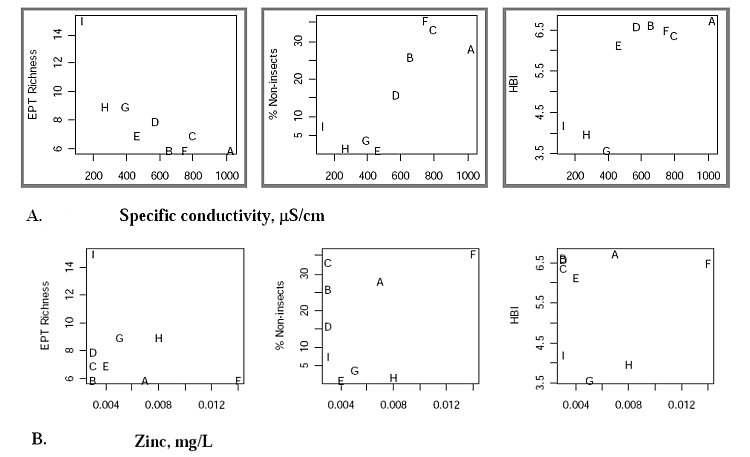
The visual interpretation of the scatterplots was supplemented with correlation coefficients (Table 1). Correlation coefficients were not evaluated for significance because of the small sample size and pseudo-replication of sites. Rather, consistent correlations of relatively large magnitude for all three biological responses were considered by the analysts to provide some support for ionic strength as a candidate cause. When evaluating this evidence, it is worth noting again that both analyses hinge on the assumption that samples of water chemistry taken in August 2000 are similar to exposures experienced by organisms in August 1999.
Specific conductivity | Zinc | |
---|---|---|
EPT Richness | -0.86 | -0.21 |
% non-insects | 0.78 | 0.026 |
HBI | 0.78 | -0.15 |
Associations between specific conductivity and all three biological responses were apparent and in the expected direction. We would score this evidence as + for each of the biological responses.
There were no clear associations between zinc and any of the three biological responses. We would score this evidence as - for each of the biological responses.
- Davies SP, Tsomides L (2002) Methods for biological sampling and analysis of Maine's rivers and streams . Maine Department of Environmental Protection, Augusta ME. DEP LW0387-B2002.
- Hilsenhoff WL (1987) An improved biotic index of organic stream pollution. Great Lakes Entomologist 20:31-39.
- Hilsenhoff WL (1988) Rapid field assessment of organic pollution with a family level biotic index. Journal of the North American Benthological Society 7(1):65-68.
- MEDEP (2002) A biological, physical, and chemical assessment of two urban streams in southern Maine: Long Creek and Red Brook. Maine Department of Environmental Protection, Augusta ME. DEP-LW0572.
- U.S. EPA (2007) Causal Analysis of Biological Impairment in Long Creek: A Sandy-Bottomed Stream in Coastal Southern Maine . U.S. Environmental Protection Agency, Office of Research and Development, National Center for Environmental Assessment, Washington DC. EPA-600-R-06-065F.
Example 4. Stress or-Response Relationships from Laboratory Studies
Laboratory toxicity data.
In this example, we ask whether organisms in Long Creek, Maine (U.S. EPA 2007) are exposed to a candidate cause (zinc) at quantities or frequencies sufficient to induce observed biological effects. We use results from laboratory studies to evaluate whether zinc in the water column under base flow conditions reached concentrations that could explain the observed decrease in Ephemeroptera, Plecoptera and Trichoptera (EPT) richness. The comparison of laboratory and field data can be performed in two ways.
- Most commonly, effective concentrations from laboratory data are compared to ambient concentrations at the affected site. If zinc concentrations associated with similar types of effects in the laboratory are similar to or lower than concentrations that have been shown to occur at the affected site, this would provide evidence that zinc concentrations are high enough to cause the effects.
- Species Sensitivity Distributions
- Stressor-Response Relationships from Laboratory Studies
Conversely, if zinc concentrations associated with similar types of effects in the laboratory are much higher than those at the affected site, then the case for zinc would be weakened. Either some other stressor is the cause of the observed decline, or zinc is acting jointly with another cause to produce the effect.
- We can also compare the magnitude of effects observed at the site with the magnitude of effects observed in the laboratory at concentrations equal to ambient concentrations. If the magnitude of effects at the site are much greater than would be predicted from the laboratory concentration-response relationship, then we would conclude that either zinc concentrations are not high enough to have caused the effects, or the laboratory organisms or endpoints are not as sensitive as the organisms or responses at the affected site. If magnitude of effects observed at the site is approximately equal to those predicted from the laboratory concentration-response relationship, then this would support the argument that zinc is the cause of the effects. Finally, if the magnitude of effects observed at the site is much less than predicted from laboratory studies, we would conclude that some physical factor (e.g., dissolved organic matter) or some biological process (e.g., replacement of sensitive insect species by tolerant species) may be reducing the effect in the field.
This example uses summaries of laboratory toxicity test results and compares these summaries with data from the site.
Two approaches were used to summarize laboratory results. First, U.S. EPA's chronic criterion value for zinc was used to represent sublethal effects and effects of extended exposures. The chronic criterion value for zinc at 100 mg/L hardness (as CaCO 3 ) is 0.12 mg/L . A chronic value for an EPT insect would be preferable, but none were available.
- ECOTOX Database
It was necessary to generate SSDs with data for total metals because greater than 90% of freshwater metals data in ECOTOX are reported as total metals. Free ion or dissolved metal concentrations would be more appropriate indicators of actual toxic exposure and be more relevant to the dissolved metal concentrations reported for Long Creek. However, this is a relatively minor problem, because nearly all metals in laboratory tests are dissolved.
SSDs were generated using LC 50 data. Since an LC 50 is a concentration that kills half of the organisms in a test population, one would expect to observe a reduction in the abundance of some species when water concentrations equal the LC 50 for that species. Data used in generating SSDs do not represent specific species present at the study area. Toxicity data are generally not available for site-specific taxa due to the diversity of species occurring in the wild and the need to perform toxicity tests with well characterized organisms.
Biological and water chemistry data from two sites along Long Creek are used in this example. EPT richness was calculated from macroinvertebrate rockbag samples deployed throughout the study area beginning August 5-6 1999, following standard Maine Department of Environmental Protection (MEDEP) protocol (Davies and Tsomides 2002).
Baseflow water samples were collected by MEDEP on three days in August 2000. Methods and analyses are described in MEDEP (2002).
The laboratory results were compared to site data by graphically comparing the proportion of decrease in EPT richness, relative to the reference site, and impaired site zinc concentrations. In addition, the SSD was used to identify 0.087 as a benchmark concentration of 10% at which 10% of species would be expected to experience lethal effects.
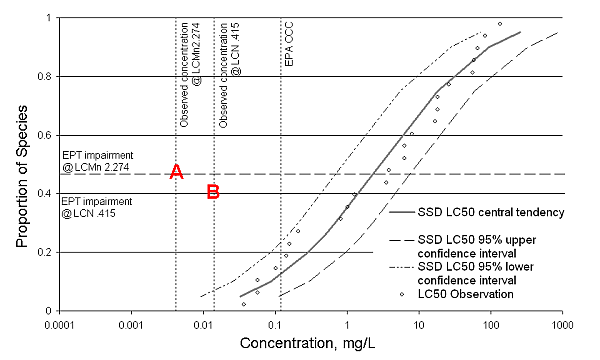
- The organisms and endpoints measured in the laboratory are relevant to EPT richness.
- The laboratory exposures are relevant to the exposures encountered by organisms in the field.
- Measured baseflow concentrations of zinc in August 2000 were similar to unmeasured concentrations in August 1999.
How do I score this evidence?
Measured concentrations are all below the EPA criterion continuous concentration. The measured concentrations at the site fall below the 10% benchmark derived from the SSD. Points corresponding to the observed impairment occur at concentrations below the lower confidence limits on the SSD curve. This weakens the case that zinc caused the observed decreases in EPT, giving a score of - (one minus).
- Davies SP, Tsomides L (2002) Methods for biological sampling and analysis of Maine's rivers and streams . Maine Department of Environmental Protection, Augusta ME. DEP LW0387-B2002.
- U.S. EPA (2007) Causal Analysis of Biological Impairment in Long Creek: A Sandy-Bottomed Stream in Coastal Southern Maine . U.S. Environmental Protection Agency, Office of Research and Development, National Center for Environmental Assessment, Washington DC. EPA-600-R-06-065F.
Example 5. Verified Prediction with Traits
In causal analysis we find that trait information is well suited to a type of evidence called verified prediction, where the knowledge of a cause's mode of action permits prediction and subsequent confirmation of previously unobserved effects. In this application, we would predict changes in the occurrence of different traits we would expect to occur if a particular stressor was present and causing biological effects. If we found that these traits do indeed occur at the impaired site, our prediction is verified and the causal hypothesis is supported by that evidence.
Analytical approaches range from basic comparisons of measurements to more formal statistical tests (see page on establishing differences from expectations). Incorporating predictions of traits into causal analysis is an area of active research, and so we present a hypothetical example below.
Existing information about the relationship between a trait and environmental gradients can be used to predict how the occurrence of a trait will differ between the test site and reference expectations. The occurrence of a trait in a community from test site is compared with a community from a reference site. If the predicted occurance of a is supported, the result would support a claim of verified prediction.
We illustrate this with an example of clinger relative richness and sediment in streams across the eastern United States. Existing literature indicates that the relative richness of clingers decreases with increased bedded sediment (Figure 5, Pollard and Yuan 2010).
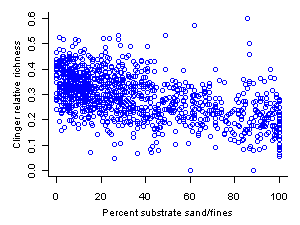
- See Interpreting Statistics to determine the confidence intervals
If the predicted pattern is observed (here, if the test site had fewer clingers than the reference site), the type of evidence "verified prediction" is scored as supported (+). If multiple predictions were verified or if the predictions were highly specific, the evidence may be convincing (+++).
- Abell R, Thieme ML, Revenga C, Bryer M, Kottelat M, Bogutskaya N, Coad B, Mandrak N, Balderas SC, Bussing W, Stiassny MLJ, Skelton P, Allen GR, Unmack P, Naseka A, Ng R, Sindorf N, Robertson J, Armijo E, Higgins JV, Heibel TJ, Wikramanayake E, Olson D, Lopez HL, Reis RE, Lundberg JG, Sabaj Perez MH, Petry P (2009) Freshwater ecoregions of the world: a new map of biogeographic units for freshwater biodiversity conservation. BioScience 58:403-414.
- Pollard AI, Yuan LL (2010) Assessing the consistency of response metrics of the invertebrate benthos: a comparison of trait- and identity-based measures. Freshwater Biology 55:1420-1429.
- CADDIS Home
- Volume 1: Stressor Identification
- Volume 2: Sources, Stressors and Responses
- Worksheet Examples
- State Examples
- Case Studies
- Volume 4: Data Analysis
- Volume 5: Causal Databases

10 Examples: What Are Analytical Skills?
Analytical skills are cognitive abilities that allow you to process, evaluate, and interpret complex information. These skills allow you to make data-driven decisions and solve problems effectively. In today’s fast-paced and data-driven world, having strong analytical skills is essential to excel in both personal and professional endeavors.
There are several components to analytical skills, such as critical thinking, data and information analysis, problem-solving, and decision-making. These components work in tandem to help you analyze various factors, uncover patterns or trends, and draw logical conclusions based on available data.
Here are some examples of analytical skills:
- Critical thinking: The ability to objectively evaluate information and form a reasoned judgment.
- Data analysis: The process of collecting, organizing, interpreting, and presenting data.
- Problem-solving: The capacity to identify issues, analyze potential solutions, and implement the most effective course of action.
- Decision-making: The process of choosing the most appropriate option among various alternatives based on relevant information.
- Research: The skill to gather information on a specific topic, interpret it and draw conclusions.
To showcase your analytical skills in a job application, emphasize instances where you have used these abilities to achieve positive results. Include metrics or specific examples that demonstrate the impact of your actions.
1. Critical thinking: “Analyzed complex data sets and objectively evaluated information to form a reasoned judgment, resulting in a 10% increase in sales revenue.”
2. Data analysis: “Utilized advanced data analysis techniques to collect, organize, interpret, and present data, resulting in a 20% reduction in operating costs.”
3. Problem-solving: “Identified issues in the production process, analyzed potential solutions, and implemented the most effective course of action, resulting in a 15% increase in productivity.”
4. Decision-making: “Made informed decisions by choosing the most appropriate option among various alternatives based on relevant information, resulting in a 25% increase in customer satisfaction.”
5. Research: “Conducted extensive research on market trends and customer preferences, interpreted the data, and drew conclusions that informed the development of new products, resulting in a 30% increase in sales.”
Related: Top Transferable Skills Every Company Wants
Analytical Skills Examples
Research and data analysis.
In your research and data analysis efforts, you can showcase your analytical skills by gathering relevant information, processing it, and drawing conclusions from the findings. For example:
- Conducting market research to identify trends and patterns
- Analyzing data to determine effectiveness of an advertising campaign
- Utilizing statistical software to evaluate data and make predictions
Critical Thinking
Critical thinking involves analyzing information, considering alternative viewpoints, and making informed decisions. Examples of using critical thinking skills include:
- Evaluating the pros and cons before making a decision
- Recognizing potential pitfalls or inconsistencies in a plan
- Identifying and questioning assumptions in an argument
Problem-Solving
Problem-solving requires identifying issues, generating potential solutions, and selecting the most appropriate course of action. Some examples of problem-solving skills in action are:
- Troubleshooting technical issues by systematically examining components
- Resolving customer complaints by finding mutually beneficial solutions
- Implementing new processes to increase efficiency and reduce errors
Communication
Effective communication is a vital analytical skill, as it enables you to convey your findings and ideas to others. Through clear and concise presentations, you can demonstrate your ability to:
- Summarize complex data in easy-to-understand formats
- Explain your thought process while reaching a decision
- Collaborate with team members to formulate plans and solve problems
Analytical Skills Examples for Different Industries
Analytical skills in marketing: resume paragraph example.
“I possess strong analytical skills that allow me to understand consumer behavior and trends. I have experience utilizing statistical analysis to identify patterns in customer preferences and target campaigns effectively. This knowledge has allowed me to segment audiences, set priorities, and optimize marketing strategies, resulting in increased ROI and customer engagement.”
Analytical Skills in Finance: Resume Paragraph Example
“With my financial analytical skills, I am able to manage budgets, analyze balance sheets, and forecast revenue growth. I have experience utilizing financial models to assess investment opportunities, evaluate profitability, and perform risk assessments. This skill set has enabled me to make informed decisions that impact my organization’s financial health, resulting in increased profitability and stability.”
Analytical Skills in Sales: Resume Paragraph Example
“My analytical skills allow me to interpret sales data, identify trends, and forecast future demand. I have experience planning targeted sales strategies, allocating resources efficiently, and increasing overall productivity in the industry, resulting in increased sales revenue.”
Analytical Skills in Website Management: Resume Paragraph Example
“I possess strong analytical skills that allow me to analyze user behavior and site performance to optimize the user experience. I have experience tracking website metrics and probabilities to identify areas for improvement, drive more traffic, and engage users more effectively.”
Analytical Skills in Science and Research: Resume Paragraph Example
“I possess essential analytical skills for designing experiments, interpreting data, and drawing informed conclusions. I have experience critically analyzing research findings and challenging existing models to drive innovation and advancements in my field.”
Demonstrating Analytical Skills
To showcase your analytical skills in your resume, include them in the Skills section as bullet points. Be specific, mentioning the particular analytical abilities you excel in. For instance:
- Data analysis
- Critical thinking
- Problem-solving
Next, incorporate your analytical skills within your Work Experience section. Use action verbs and quantify your accomplishments wherever possible. Here’s an example:
- “Analyzed market trends to increase sales by 20% in Q3”
Cover Letter
Your cover letter offers an opportunity to provide context and examples of how you’ve utilized your analytical skills in the past. Choose a specific experience or project to discuss, and demonstrate how your skills contributed to its success. For example:
“In my previous role as a Market Analyst at X Company, I employed my data analysis skills to identify business growth opportunities. I assessed customer feedback and sales data, allowing us to better target our marketing efforts and resulting in a 15% increase in customer satisfaction.”
Job Interview
During the job interview, be prepared to provide concrete examples of how you’ve applied your analytical skills. Use the STAR (Situation, Task, Action, Result) method to describe a particular scenario in which you demonstrated your abilities:
- Situation : Explain the context or challenge you faced
- Task : Describe the goal you were trying to achieve
- Action : Express the specific steps you took, emphasizing your analytical skills
- Result : Share the positive outcome achieved
For example:
“In my last position as a Financial Analyst, I was tasked with identifying cost-saving measures for our department. I meticulously reviewed budget reports and discovered discrepancies in vendor billing. By negotiating new contracts, we managed to save the company $50,000 annually.”
Developing and Enhancing Analytical Skills
Improving critical and analytical thinking.
To improve your critical and analytical thinking skills, start by questioning assumptions and evaluating the source of information. Expand your knowledge base by reading diverse materials and participating in discussions with individuals who have different perspectives. Utilize activities such as puzzles, brainteasers, and strategy games to challenge your brain further. Also, think critically about your own beliefs and decisions to foster self-awareness, humility, and open-mindedness.
Problem-Solving Techniques
Effective problem-solving techniques include breaking down complex issues into smaller, more manageable components and analyzing each independently. This approach allows you to systematically address challenges step-by-step. Additionally, brainstorm various potential solutions, considering both conventional and unconventional ideas. After identifying possible options, evaluate the pros and cons of each, and select the most viable ones to implement.
Related: Top Problem-Solving Skills for Today’s Job Market
The Role of Soft and Hard Skills
As you develop your analytical skills, it’s important to understand the roles of both soft and hard skills. Soft skills pertain to your interpersonal, communication, and collaborative abilities, which contribute to your overall effectiveness in the workplace. On the other hand, hard skills or technical skills refer to the specific capabilities you possess, such as programming, data analysis, or expertise in a particular software.
A well-rounded professional should have a combination of both soft and hard skills. To effectively analyze data, interpret findings, and solve complex problems, you need not only the technical expertise but also the communication and relationship-building skills to work with others.
In the context of analytical skills, examples of soft skills include critical thinking, problem-solving, and adaptability. These abilities allow you to see beyond the numbers, identify patterns, and anticipate how changes in one area may affect another. Additionally, communication and collaboration skills are key for working in a team setting, understanding different perspectives, and finding the best solution. Related: What Are Soft Skills? (and How to Showcase Them)
Examples of hard skills related to analytical skills include data processing, statistical analysis, and experience with analytical tools like Excel or SQL. These technical abilities enable you to gather, process, and analyze data more efficiently and accurately, helping you produce valuable insights for your team and organization. Related: Technical Skills Examples for Resume and List of 21 Important Technical Skills (with Examples)
To showcase your analytical skills in a job application, consider mentioning specific instances where you applied your analytical abilities, such as solving a complex issue or improving a process through data-driven insights. Provide examples that demonstrate your proficiency in relevant technical tools or software.
The Importance of Analytical Skills in the Workplace
As an employee, your ability to process and interpret information allows you to make well-informed decisions, spot trends, and tackle complex problems.
One of the key aspects of analytical skills is decision-making . In any job, your ability to make sound decisions in a timely manner will contribute to your success. By breaking down complex information and identifying patterns, you can draw from a rich pool of knowledge and make confident choices that benefit both your team and your organization.
As you hone your analytical skills, you’ll understand more effectively how to process the deluge of information present in today’s work environment. Whether you’re dealing with data, reports, or research, your ability to extract meaningful insights will allow you to add value to projects and deliver results that have a tangible impact.
Related: Effective Decision Making Process: 7 Steps with Examples
Frequently Asked Questions
What are some common examples of analytical skills.
Some common examples of analytical skills include: problem-solving, critical thinking, data analysis, decision-making, systems thinking, research, attention to detail, and forecasting. These skills allow you to effectively gather, interpret, and apply information to understand complex situations and make well-informed decisions.
How do you demonstrate analytical skills in a job interview?
During a job interview, you can demonstrate your analytical skills by:
- Sharing specific examples of how you used analytical skills to solve a problem or make a decision in your past work experiences.
- Highlighting projects or tasks where you had to analyze data, identify patterns, and derive conclusions.
- Discussing tools and techniques you have used for data analysis, such as spreadsheets, statistical software, or analytical frameworks.
- Explaining your thought process in real-time when answering situational or problem-solving interview questions.
What are the key differences between analytical skills and critical thinking?
Analytical skills involve techniques for gathering, organizing, interpreting, and drawing conclusions from data and information, while critical thinking is a broader skill that includes the ability to question assumptions, evaluate arguments, and make informed judgments based on evidence and sound reasoning.
How do analytical skills benefit workplace performance?
Analytical skills can improve workplace performance by:
- Enhancing decision-making processes, leading to more informed and effective choices.
- Identifying patterns and trends in data that can inform future planning or strategies.
- Improving troubleshooting and problem-solving abilities, helping to resolve issues more efficiently.
- Increasing innovation and creativity by encouraging systematic exploration of ideas and synthesis of new insights.
Which professions require strong analytical skills?
Professions that often require strong analytical skills include: data analysts, finance professionals, business analysts, marketers, economists, scientists, engineers, healthcare professionals, and project managers. However, analytical skills can be valuable assets in virtually any industry and role, as they are crucial for problem-solving and effective decision-making.
What are some effective ways to develop and enhance analytical skills?
To develop and enhance your analytical skills, consider the following:
- Engaging in activities that require data analysis, such as working on projects, participating in clubs or organizations, or volunteering in relevant fields.
- Taking courses or attending workshops on subjects like statistics, logic, data visualization, and related topics.
- Practicing problem-solving techniques, such as breaking down complex issues into smaller components or using models and frameworks to guide your thinking.
- Seeking feedback on your work and learning from experience, as well as observing and learning from professionals with strong analytical skills.
- 8 Examples: Top Problem Solving Skills
- 37 Analytical Skills Self Evaluation Comments Examples
- Self Evaluation Examples [Complete Guide]
Pitch N Hire
Request a demo
Get personalized job alerts matching your skills and preferences.

- One-Click Apply to Jobs
- Set Your Job Alerts
- Personalized Job Recommendations
- Build your Resume
- Give Assessments & Earn Profile Badges
- Track Your Job Applications
Everything You Need To Know About Analytical Research
Written By : Pitch N Hire
Wed May 01 2024

Research is vital in any field. It helps in finding out information about various subjects. It is a systematic process of collecting data, documenting critical information, analyzing data, and interpreting it. It employs different methodologies to perform various tasks. Its main task is to collect, compose and analyze data on the subject matter. It can be defined as the process of creating new knowledge or applying existing knowledge to invent new concepts.
Research methods are classified into different categories based on the methods, nature of the study, purpose, and research design. Based on the nature of the study, research is classified into two parts- descriptive research and analytical research. This article will cover the subject matter of analytical research.
Now, you must be thinking about what is analytical research. It is that kind of research in which secondary data are used to critically examine the study. Researchers used already existing information for research analysis. Different types of analytical research designs are used for critically evaluating the information extracted from the data of the existing research.
Effect of Analytical Studies on Education Trails
Students, research scholars, doctors, psychologists, etc. take the help of analytical research for taking out important information for their research studies. It helps in adding new concepts and ideas to the already produced material. Various kinds of analytical research designs are used to add value to the study material. It is conducted using various methods such as literary research, public opinion, meta-analysis, scientific trials, etc.
When you come across a question of what is analytical research, you can define it as a tool that is used to add reliability to the work. This is generally conducted to provide support to an idea or hypothesis. It employs critical thinking to extract the small details. This helps in building big assumptions about the subject matter or the material of the study. It emphasizes comprehending the cause-effect relationship between variables.
Analytical Research Designs
All-in-one Hiring OS
Revolutionizing Interviews, Hiring, and Job Opportunities

The first is cohort studies and the second is a case-control study. In cohort studies, people of different groups with different levels of exposure are observed over time to analyze the occurrence of an outcome. It is a forward-direction and prospective kind of study. It is easier to determine the outcome risk among unexposed and exposed groups.
It resembles the experimental design. Whereas in case-control studies, researchers enlist two groups, cases, and controls, and then bring out the history of exposure of each group. It is a backward-direction and retrospective study. It consumes less time and is comparatively cheaper than cohort studies. It is the primary study design that is used to examine the relationship between a particular exposure and an outcome.
Methods of Conducting Analytical Research
Analytical Research saves time, money, and lives and helps in achieving objectives effectively. It can be conducted using the following methods:
Literary Research
Literary Research is one of the methods of conducting analytical research. It means finding new ideas and concepts from already existing literary work. It requires you to invent something new, a new way of interpreting the already available information to discuss it. It is the backbone of various research studies. Its function is to find out all the literary information, preserve them with different methodologies and analyze them. It provides hypotheses in the already existing research and also helps in analyzing modern-day research. It helps in analyzing unsolved or doubtful theories.
Meta-Analysis Research
Meta-Analysis is an epidemiological, formal, and quantitative research design that helps in the systematic assessment of previous research results to develop a conclusion about the body of research. It is a subset of systematic reviews. It analyzes the strength of the evidence. It helps in examining the variability or heterogeneity. It includes a quantitative review of the body of literature. It is PRISMA and its aim is to identify the existence of effects, finding the negative and positive kinds of effects. Its results can improve the accuracy of the estimates of effects.
Scientific Trials
Scientific Trials research is conducted on people. It is of two types, observational studies and the second is clinical traits. It finds new possibilities for clinical traits. It aims to find out medical strategies. It also helps in determining whether medical treatment and devices are safe it not. It searches for a better way of treating, diagnosing, screening, and treatment of the disease. It is a scientific study that involves 4 stages. It is conducted to find if a new treatment method is safe, effective, and efficient in people or not.
It aims to examine or analyze surgical, medical, and behavioral interventions. There are different types of scientific trials such as cohort studies, case-control studies, treatment trials, cross-sectional studies, screening trials, pilot trials, prevention trials, etc.
Analytical Research is that kind of research that utilizes the already available data for extracting information. Its main aim is to divide a topic or a concept into smaller pieces to understand it in a better way and then assemble those parts in a way that is understandable by you. You can conduct analytical research by using the methods discussed in the article. It involves ex-ante research. It means analyzing the phenomenon.
It is of different types such as historical research, philosophical research, research synthesis, and reviews. Also, it intends to comprehend the causal relation between phenomena. It works within the limited variables and involves in-depth research and analysis of the available data.
Therefore, it is crucial for any data because it adds relevance to it and makes it authentic. It supports and validates a hypothesis. It helps companies in making quick and effective decision-making about the product and services provided by them.
Related Articles
- Siebel interview questions
- Spec work for graphic designers
- How Do Hiring Software Systems Improve The Recruitment Process?
- An Ultimate Guide to Candidate Management System
- Best Applicant Tracking System (ATS) Providers In The USA
- Candidate Tracking System: What Does It Include?
Let our experts elevate your hiring journey. Message us and unlock potential. We'll be in touch.
- Applicant Tracking System (ATS)
- Interview & Assessment
- SEO Services
- Content Marketing Services
- Social Media Marketing Services
- Software Testing Services
- Web Development Services
- UI / UX Services
- Mobile Development Services
- Permanent Staffing Services
- Contract Staffing Services
Related Posts
For any queries to privacy concerns, please contact us at [email protected]
Begin a seamless recruiting journey today.
How it works
Transform your enterprise with the scalable mindsets, skills, & behavior change that drive performance.
Explore how BetterUp connects to your core business systems.
We pair AI with the latest in human-centered coaching to drive powerful, lasting learning and behavior change.
Build leaders that accelerate team performance and engagement.
Unlock performance potential at scale with AI-powered curated growth journeys.
Build resilience, well-being and agility to drive performance across your entire enterprise.
Transform your business, starting with your sales leaders.
Unlock business impact from the top with executive coaching.
Foster a culture of inclusion and belonging.
Accelerate the performance and potential of your agencies and employees.
See how innovative organizations use BetterUp to build a thriving workforce.
Discover how BetterUp measurably impacts key business outcomes for organizations like yours.
Daring Leadership Institute: a groundbreaking partnership that amplifies Brené Brown's empirically based, courage-building curriculum with BetterUp’s human transformation platform.

- What is coaching?
Learn how 1:1 coaching works, who its for, and if it's right for you.
Accelerate your personal and professional growth with the expert guidance of a BetterUp Coach.
Types of Coaching
Navigate career transitions, accelerate your professional growth, and achieve your career goals with expert coaching.
Enhance your communication skills for better personal and professional relationships, with tailored coaching that focuses on your needs.
Find balance, resilience, and well-being in all areas of your life with holistic coaching designed to empower you.
Discover your perfect match : Take our 5-minute assessment and let us pair you with one of our top Coaches tailored just for you.
Find your coach
-1.png)
Research, expert insights, and resources to develop courageous leaders within your organization.
Best practices, research, and tools to fuel individual and business growth.
View on-demand BetterUp events and learn about upcoming live discussions.
The latest insights and ideas for building a high-performing workplace.
- BetterUp Briefing
The online magazine that helps you understand tomorrow's workforce trends, today.
Innovative research featured in peer-reviewed journals, press, and more.
Founded in 2022 to deepen the understanding of the intersection of well-being, purpose, and performance
We're on a mission to help everyone live with clarity, purpose, and passion.
Join us and create impactful change.
Read the buzz about BetterUp.
Meet the leadership that's passionate about empowering your workforce.

For Business
For Individuals

What are analytical skills? Examples and how to level up
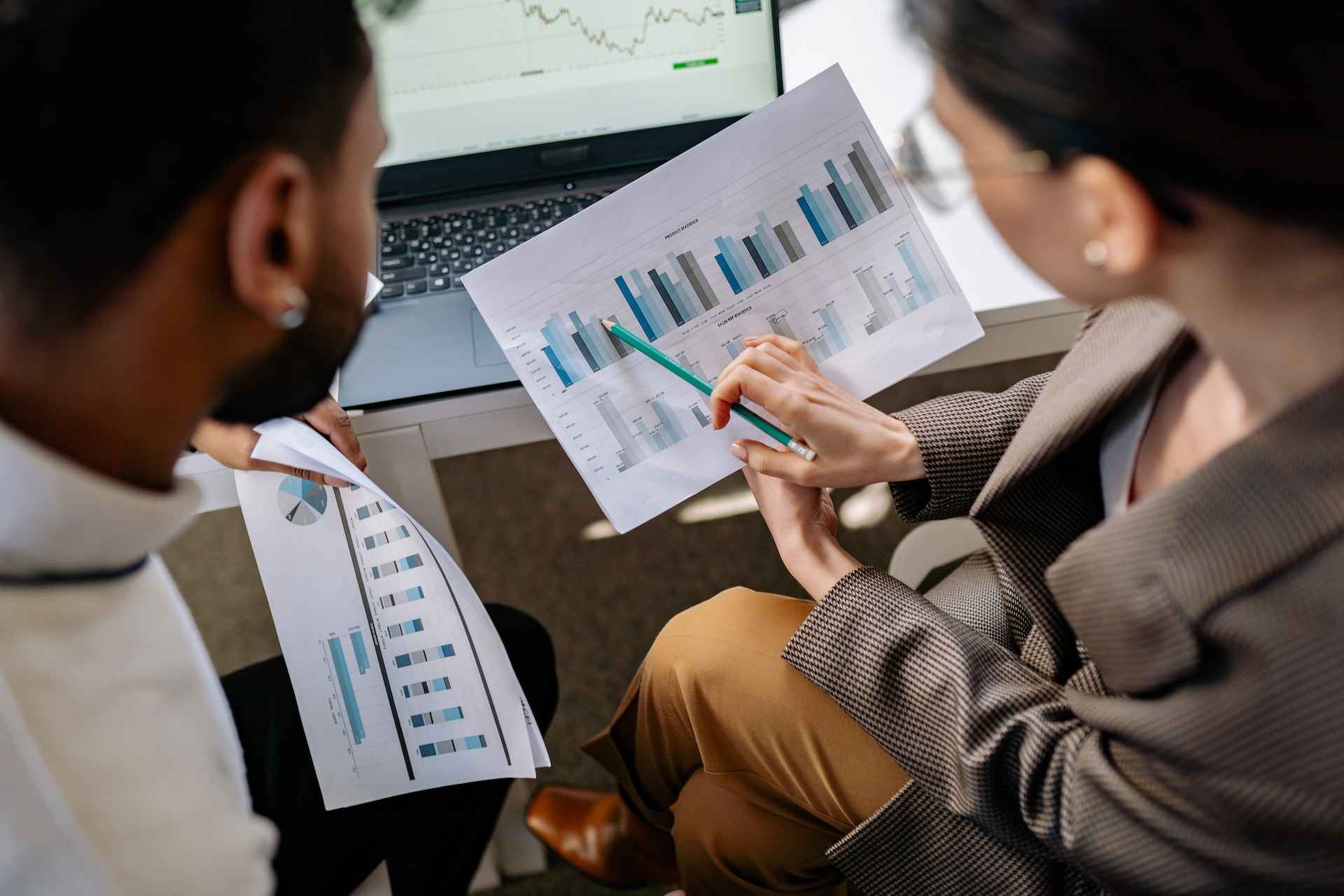
Jump to section
What are analytical skills?
Why are analytical skills important, 9 analytical skills examples, how to improve analytical skills, how to show analytical skills in a job application, the benefits of an analytical mind.
With market forecasts, performance metrics, and KPIs, work throws a lot of information at you.
If you want to stay ahead of the curve, not only do you have to make sense of the data that comes your way — you need to put it to good use. And that requires analytical skills.
You likely use analytical thinking skills every day without realizing it, like when you solve complex problems or prioritize tasks . But understanding the meaning of analysis skills in a job description, why you should include them in your professional development plan, and what makes them vital to every position can help advance your career.
Analytical skills, or analysis skills, are the ones you use to research and interpret information. Although you might associate them with data analysis, they help you think critically about an issue, make decisions , and solve problems in any context. That means anytime you’re brainstorming for a solution or reviewing a project that didn’t go smoothly, you’re analyzing information to find a conclusion. With so many applications, they’re relevant for nearly every job, making them a must-have on your resume.
Analytical skills help you think objectively about information and come to informed conclusions. Positions that consider these skills the most essential qualification grew by 92% between 1980 and 2018 , which shows just how in-demand they are. And according to Statista, global data creation will grow to more than 180 zettabytes by 2025 — a number with 21 zeros. That data informs every industry, from tech to marketing.
Even if you don’t interact with statistics and data on the job, you still need analytical skills to be successful. They’re incredibly valuable because:
- They’re transferable: You can use analysis skills in a variety of professional contexts and in different areas of your life, like making major decisions as a family or setting better long-term personal goals.
- They build agility: Whether you’re starting a new position or experiencing a workplace shift, analysis helps you understand and adapt quickly to changing conditions.
- They foster innovation: Analytical skills can help you troubleshoot processes or operational improvements that increase productivity and profitability.
- They make you an attractive candidate: Companies are always looking for future leaders who can build company value. Developing a strong analytical skill set shows potential employers that you’re an intelligent, growth-oriented candidate.
If the thought of evaluating data feels unintuitive, or if math and statistics aren’t your strong suits, don’t stress. Many examples of analytical thinking skills don’t involve numbers. You can build your logic and analysis abilities through a variety of capacities, such as:
1. Brainstorming
Using the information in front of you to generate new ideas is a valuable transferable skill that helps you innovate at work . Developing your brainstorming techniques leads to better collaboration and organizational growth, whether you’re thinking of team bonding activities or troubleshooting a project roadblock. Related skills include benchmarking, diagnosis, and judgment to adequately assess situations and find solutions.
2. Communication
Becoming proficient at analysis is one thing, but you should also know how to communicate your findings to your audience — especially if they don’t have the same context or experience as you. Strong communication skills like public speaking , active listening , and storytelling can help you strategize the best ways to get the message out and collaborate with your team . And thinking critically about how to approach difficult conversations or persuade someone to see your point relies on these skills.
3. Creativity
You might not associate analysis with your creativity skills, but if you want to find an innovative approach to an age-old problem, you’ll need to combine data with creative thinking . This can help you establish effective metrics, spot trends others miss, and see why the most obvious answer to a problem isn’t always the best. Skills that can help you to think outside the box include strategic planning, collaboration, and integration.

4. Critical thinking
Processing information and determining what’s valuable requires critical thinking skills . They help you avoid the cognitive biases that prevent innovation and growth, allowing you to see things as they really are and understand their relevance. Essential skills to turn yourself into a critical thinker are comparative analysis, business intelligence, and inference.
5. Data analytics
When it comes to large volumes of information, a skilled analytical thinker can sort the beneficial from the irrelevant. Data skills give you the tools to identify trends and patterns and visualize outcomes before they impact an organization or project’s performance. Some of the most common skills you can develop are prescriptive analysis and return on investment (ROI) analysis.
6. Forecasting
Predicting future business, market, and cultural trends better positions your organization to take advantage of new opportunities or prepare for downturns. Business forecasting requires a mix of research skills and predictive abilities, like statistical analysis and data visualization, and the ability to present your findings clearly.
7. Logical reasoning
Becoming a logical thinker means learning to observe and analyze situations to draw rational and objective conclusions. With logic, you can evaluate available facts, identify patterns or correlations, and use them to improve decision-making outcomes. If you’re looking to improve in this area, consider developing inductive and deductive reasoning skills.
8. Problem-solving
Problem-solving appears in all facets of your life — not just work. Effectively finding solutions to any issue takes analysis and logic, and you also need to take initiative with clear action plans . To improve your problem-solving skills , invest in developing visualization , collaboration, and goal-setting skills.
9. Research
Knowing how to locate information is just as valuable as understanding what to do with it. With research skills, you’ll recognize and collect data relevant to the problem you’re trying to solve or the initiative you’re trying to start. You can improve these skills by learning about data collection techniques, accuracy evaluation, and metrics.

You don’t need to earn a degree in data science to develop these skills. All it takes is time, practice, and commitment. Everything from work experience to hobbies can help you learn new things and make progress. Try a few of these ideas and stick with the ones you enjoy:
1. Document your skill set
The next time you encounter a problem and need to find solutions, take time to assess your process. Ask yourself:
- What facts are you considering?
- Do you ask for help or research on your own? What are your sources of advice?
- What does your brainstorming process look like?
- How do you make and execute a final decision?
- Do you reflect on the outcomes of your choices to identify lessons and opportunities for improvement?
- Are there any mistakes you find yourself making repeatedly?
- What problems do you constantly solve easily?
These questions can give insight into your analytical strengths and weaknesses and point you toward opportunities for growth.
2. Take courses
Many online and in-person courses can expand your logical thinking and analysis skills. They don’t necessarily have to involve information sciences. Just choose something that trains your brain and fills in your skills gaps .
Consider studying philosophy to learn how to develop your arguments or public speaking to better communicate the results of your research. You could also work on your hard skills with tools like Microsoft Excel and learn how to crunch numbers effectively. Whatever you choose, you can explore different online courses or certification programs to upskill.
3. Analyze everything
Spend time consciously and critically evaluating everything — your surroundings, work processes, and even the way you interact with others. Integrating analysis into your day-to-day helps you practice. The analytical part of your brain is like a muscle, and the more you use it, the stronger it’ll become.
After reading a book, listening to a podcast, or watching a movie, take some time to analyze what you watched. What were the messages? What did you learn? How was it delivered? Taking this approach to media will help you apply it to other scenarios in your life.
If you’re giving a presentation at work or helping your team upskill , use the opportunity to flex the analytical side of your brain. For effective teaching, you’ll need to process and analyze the topic thoroughly, which requires skills like logic and communication. You also have to analyze others’ learning styles and adjust your teachings to match them.
5. Play games
Spend your commute or weekends working on your skills in a way you enjoy. Try doing logic games like Sudoku and crossword puzzles during work breaks to foster critical thinking. And you can also integrate analytical skills into your existing hobbies. According to researcher Rakesh Ghildiyal, even team sports like soccer or hockey will stretch your capacity for analysis and strategic thinking .
6. Ask questions
According to a study in Tr ends in Cognitive Sciences, being curious improves cognitive function , helping you develop problem-solving skills, retention, and memory. Start speaking up in meetings and questioning the why and how of different decisions around you. You’ll think more critically and even help your team find breakthrough solutions they otherwise wouldn’t.
7.Seek advice
If you’re unsure what analytical skills you need to develop, try asking your manager or colleagues for feedback . Their outside perspective offers insight you might not find within, like patterns in. And if you’re looking for more consistent guidance, talking to a coach can help you spot weaknesses and set goals for the long term.

8. Pursue opportunities
Speak to your manager about participating in special projects that could help you develop and flex your skills. If you’d like to learn about SEO or market research, ask to shadow someone in the ecommerce or marketing departments. If you’re interested in business forecasting, talk to the data analysis team. Taking initiative demonstrates a desire to learn and shows leadership that you’re eager to grow.

Shining a spotlight on your analytical skills can help you at any stage of your job search. But since they take many forms, it’s best to be specific and show potential employers exactly why and how they make you a better candidate. Here are a few ways you can showcase them to the fullest:
1. In your cover letter
Your cover letter crafts a narrative around your skills and work experience. Use it to tell a story about how you put your analytical skills to use to solve a problem or improve workflow. Make sure to include concrete details to explain your thought process and solution — just keep it concise. Relate it back to the job description to show the hiring manager or recruiter you have the qualifications necessary to succeed.
2. On your resume
Depending on the type of resume you’re writing, there are many opportunities to convey your analytical skills to a potential employer. You could include them in sections like:
- Professional summary: If you decide to include a summary, describe yourself as an analytical person or a problem-solver, whichever relates best to the job posting.
- Work experience: Describe all the ways your skill for analysis has helped you perform or go above and beyond your responsibilities. Be sure to include specific details about challenges and outcomes related to the role you’re applying for to show how you use those skills.
- Skills section: If your resume has a skill-specific section, itemize the analytical abilities you’ve developed over your career. These can include hard analytical skills like predictive modeling as well as interpersonal skills like communication.
3. During a job interview
As part of your interview preparation , list your professional accomplishments and the skills that helped along the way, such as problem-solving, data literacy, or strategic thinking. Then, pull them together into confident answers to common interview questions using the STAR method to give the interviewer a holistic picture of your skill set.
Developing analytical skills isn’t only helpful in the workplace. It’s essential to life. You’ll use them daily whenever you read the news, make a major purchase, or interact with others. Learning to critically evaluate information can benefit your relationships and help you feel more confident in your decisions, whether you’re weighing your personal budget or making a big career change .
Understand Yourself Better:
Big 5 Personality Test
Elizabeth Perry, ACC
Elizabeth Perry is a Coach Community Manager at BetterUp. She uses strategic engagement strategies to cultivate a learning community across a global network of Coaches through in-person and virtual experiences, technology-enabled platforms, and strategic coaching industry partnerships. With over 3 years of coaching experience and a certification in transformative leadership and life coaching from Sofia University, Elizabeth leverages transpersonal psychology expertise to help coaches and clients gain awareness of their behavioral and thought patterns, discover their purpose and passions, and elevate their potential. She is a lifelong student of psychology, personal growth, and human potential as well as an ICF-certified ACC transpersonal life and leadership Coach.
20 examples of development opportunities that can level up your career
A roadmap for career development: how to set your course, create a networking plan in 7 easy steps, discover how to get noticed by upper management at work, are you being passed over for a promotion here’s what to do, what business acumen is and 9 ways to develop it, 8 examples for setting professional development goals at work, professional development is for everyone (we’re looking at you), how to pursue jobs versus careers to achieve different goals, how to develop critical thinking skills, why we're facing a crisis of imagination, and how to overcome it, 10 essential business skills that make an impact on your career, what are hard skills & examples for your resume, use a personal swot analysis to discover your strengths and weaknesses, 17 essential transferable skills to boost your job search, critical thinking is the one skillset you can't afford not to master, what are metacognitive skills examples in everyday life, stay connected with betterup, get our newsletter, event invites, plus product insights and research..
3100 E 5th Street, Suite 350 Austin, TX 78702
- Platform Overview
- Integrations
- Powered by AI
- BetterUp Lead™
- BetterUp Manage™
- BetterUp Care®
- Sales Performance
- Diversity & Inclusion
- Case Studies
- Why BetterUp?
- About Coaching
- Find your Coach
- Career Coaching
- Communication Coaching
- Personal Coaching
- News and Press
- Leadership Team
- Become a BetterUp Coach
- BetterUp Labs
- Center for Purpose & Performance
- Leadership Training
- Business Coaching
- Contact Support
- Contact Sales
- Privacy Policy
- Acceptable Use Policy
- Trust & Security
- Cookie Preferences
Longitudinal studies of leadership development: a scoping review
- Open access
- Published: 30 August 2024
Cite this article
You have full access to this open access article
- Felipe Senna Cotrim ORCID: orcid.org/0009-0008-9820-3434 1 &
- Jorge Filipe Da Silva Gomes ORCID: orcid.org/0000-0003-0694-2229 1 , 2
Although various reviews about leadership development (LD) have been published in recent years, no one has attempted to systematically review longitudinal LD studies, which is arguably the most appropriate way to study LD (Day, Leadership Quarterly, 22 (3), 561–571, 2011). In this way, the focus of the present scoping review is to understand how true longitudinal LD studies have been investigated and what inconsistencies exist, primarily from a methodological perspective. Only business contexts and leadership-associated outcomes are considered. To achieve this, ample searches were performed in five online databases from 1900 to 2021 that returned 1023 articles after the removal of duplicates. Additionally, subject experts were consulted, reference lists of key studies were cross-checked, and handsearch of leading leadership journals was performed. A subsequent and rigorous inclusion process narrowed the sample down to 19 articles. The combined sample contains 2,776 participants (67% male) and 88 waves of data (average of 4.2). Evidence is mapped according to participants, setting, procedures, outcomes, analytical approach, and key findings. Despite many strengths, a lack of context diversity and qualitative designs are noticed. A thematic analysis indicates that LD authors are focused on measuring status, behavioral, and cognitive aspects. Implications for knowledge and future research paths are discussed.
Similar content being viewed by others
Responsible leadership: a mapping of extant research and future directions.
Leadership in Organizations: State of the Art with Emphasis on Measurement Instruments
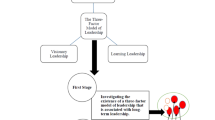
An Organization’s Success and a Three-Factor Model of Leadership: Evidence from Harvard University
Explore related subjects.
- Artificial Intelligence
Avoid common mistakes on your manuscript.
Introduction
Even though many literature reviews about leadership development (LD) have been published in recent years (e.g., Vogel, Reichard, Batistic, & Cerne, 2020; Lacerenza et al., 2017 ; Day et al., 2014 ), no one has attempted to systematically review longitudinal LD studies, let alone true longitudinal studies, which is arguably the most appropriate way to study LD (Day, 2011 ). True longitudinal is operationalized in the present study as research involving three or more phases of data collection (Ployhart & Vandenberg, 2010 ), since pretest-posttest designs can be limited when it comes to measuring change (Rogosa et al., 1982 ). In addition to the focus on studies using multiple waves of data, the particular interest here is in the underlying methodological choices of those studies. The goal is not only to map elements such as concepts, strategy, participants, settings, analytical approaches and tools, but also to make gaps and inconsistencies more evident in the hope of advancing the science of LD.
The current study relies on the assumption that longitudinal methods are the most appropriate way to study LD as the field was categorized as “inherently longitudinal” (Day, 2011 ). These arguments are partly motivated by the idea that the leader development process is an ongoing and lifelong journey (Day et al., 2009 ), which, in turn, indicates why cross-sectional methods would be less suited. By inspecting the term “leadership development”, it is noted that it refers not only to the science of leadership, but also the science of development, which is concerned with measuring change over time. The development side is underexplored, but the focus should be on both parts of the equation (Day et al., 2014 ). As Day ( 2024 ) recently puts it: “We need a separate field of leader and leadership development apart from the voluminous leadership literature because of the development component” (p. 213). Despite referring to leadership and development as a science above, it seems worth acknowledging that they can be seen as an art too (Ladkin & Taylor, 2010 ). The art of leadership is described by Springborg ( 2010 ) as staying present with one’s senses instead of quickly jumping to conclusions. This line of thinking suggests that practicing the art of leadership means relying on intuition, awareness, and feeling. This is potentially relevant as the complexity of the world cannot be completely understood from scientific operationalizations alone, arts-based practices relate differently with complexity, allowing novel ways of responding to it (Ladkin & Taylor, 2010 ).
Considering the preceding paragraphs, the present research question can be expressed as: how are true longitudinal studies of LD being investigated and what inconsistencies exist, primarily from a methodological perspective? To help answer this question, a scoping review was chosen, a type of systematic review that is most suitable when the goal is to map evidence and identify gaps in knowledge (Tricco et al., 2018 ), and not to understand the effectiveness of specific interventions, which is the job of a traditional systematic review (Munn et al., 2018 ). Researchers suggest that scoping reviews should be as comprehensive as possible (Arksey & O’Malley, 2005 ), thus the process of including articles involved searching multiple online databases, identifying gray literature, cross-checking reference lists of key studies, and handsearching leading leadership journals. Only articles written in English language were admitted. Significant time was spent building a subsequent search strategy and a pre-determined inclusion criteria was followed to arrive at the final sample. The search and inclusion process follows the procedures of the PRISMA statement, the preferred reporting items for systematic reviews and meta-analysis (Moher et al., 2009 ), and particularly the PRISMA extension for scoping reviews (PRISMA-ScR) (Tricco et al., 2018 ).
Nineteen studies were further analyzed out of 1,236 identified. A large table (Table 1 ) is presented in the results section mapping the most important methodological information. As recommended (Tricco et al., 2018 ), a thematic analysis is conducted too, followed by a discussion about the emergent themes in longitudinal LD.
Literature review
Leader and leadership development.
Using 2,390 primary works and 78,178 secondary ones, a recent bibliometric review (Vogel et al., 2020 ) maps the LD field in two interesting ways: through a historiography and a co-citation analysis. Historiography indicates that LD originated in actual organizational challenges and needs around 1989 and then transitioned to theory building around 2004 pulled by authentic leadership development scholars. The co-citation analysis indicates that seminal theories in leadership, motivation and learning highly influenced the field, which, in turn, shifted its focus to developmental interventions and processes as well as theoretical frameworks and intra-person developmental efforts such as identity construction (Vogel et al., 2020 ). Still on a broader level, by reviewing 25 years of LD contributions, Day et al. ( 2014 ) explains why LD is young compared to the centenary field of leadership. The former is, by definition, interested in change (development), and the latter, for a significant time of history, has focused on traits, which are harder to change, though not impossible (Bleidorn et al., 2019 ).
Individuals have predisposed levels of leadership ability (Arvey et al., 2007 ) and researchers have been especially interested in intelligence (Judge et al., 2004 ) and personality (Judge et al., 2002 ). Even though genetics will always play a part, leadership training works even more than previously thought regarding reactions, learning, transfer, and actual results, as shown by a meta-analysis (Lacerenza et al., 2017 ).
Instead of training, McCall ( 2004 ) argues that experiences are at the heart of LD. The challenges associated with experiences is that it is not simple to offer the right experiences to the right executives and that they vary in developmental potential due to contextual circumstances and individual differences. Six years later, McCall ( 2010 ) reinforces his argument, suggesting that companies should bet on what is potentially the most powerful developer of leaders: experience. Within the scope of experiences, some scholars are making the case for “consciousness-raising experiences” in leadership development (Mirvis, 2008 ). They are designed for the mind and heart and characterized by the focus on self, others, and society. Another relevant and more common type of experience in life is education. Evidence from almost half a million students from 600 institutions highlights that leadership knowledge as well as opportunities for application of learned principles are related with an increase in leadership capacity upon conclusion of higher education (Johnson & Routon, 2024 ).
Experiences and trainings are naturally more focused on developing skills and competencies, but some authors understand that these sometimes loosely connected leadership skills should be integrated to a leader identity (Lord & Hall, 2005 ). Indeed, identity has become a more popular aspect of LD (Epitropaki et al., 2017 ) and empirical investigations claim that leader identity is associated with leader effectiveness (Day & Sin, 2011 ).
Day ( 2000 ) makes the important distinction between leader development (developing individuals) and leadership development (developing the collective). In the present work, the use of “LD” incorporates both leader and leadership development. Drawing on this idea, The Center for Creative Leadership defines leader development as “the expansion of a person’s capacity to be effective in leadership roles and processes (Van Velsor et al., 2010 , p. 2)” and leadership development as “the expansion of a collective’s capacity to produce direction, alignment, and commitment (Van Velsor et al., 2010 , p. 20)”. Respecting these distinctions and contributions, Day and Dragoni ( 2015 ) review theoretical and practical arguments and suggest proximal and distal outcomes to indicate whether leadership is developing from an individual level and a team level. For instance, on the individual level, leadership self-efficacy and leader identity are proximal indicators while dynamic skills and meaning-making structures are distal. Regarding the team level, psychological safety and team learning are proximal indicators while collective leadership capacity are distal ones.
LD is also greatly associated with mentoring across publications, for instance, it increases leadership self-efficacy, which, in turn, predicts leader performance (Lester et al., 2011 ), and it also promotes the development of a leader identity (Muir, 2014 ). Interestingly, the effect of mentoring is not only beneficial to mentees in terms of developing (transformational) leadership, but also to mentors (Chun et al., 2012 ). Similarly, a recent study shows that mentors can develop their leader identity and self-efficacy as a result of a mentoring process (Ayoobzadeh & Boies, 2020 ). In the same vein, coaching has been established as an important LD topic (Day, 2000 ). A systematic review shows several methodological challenges associated with executive coaching, but list many evidence-based benefits of the practice in relation to the coachee (e.g. better leadership skills), the organization, and the coach (Athanasopoulou & Dopson, 2018 ).
Feedback seems to be another popular theme within the LD literature, especially 360-degree feedback (Atwater & Waldman, 1998 ), a practice associated with enhanced management competence in corporate environments (Bailey & Fletcher, 2002 ). Within an MBA context, peer feedback decreased self-ratings of leadership competence three and six months later, an effect that was stronger for women than men, suggesting that women align their self-ratings with peer ratings while men have a tendency to inflate their self-images (Mayo et al., 2012 ). Seifert and Yukl ( 2010 ) contribute to the literature by demonstrating that two feedback interventions enhance leader effectiveness compared to only one intervention. Even though a recent meta-analysis related the use of 360-degree feedback during leadership training to higher results compared to single-source feedback, it is also linked to lower levels of learning and transfer (Lacerenza et al., 2017 ). For example, receiving negative feedback from multiple sources could obstruct improvement because it may threaten one’s self-view. These results can be considered thought provoking given how 360 feedback is popular and sometimes taken for granted by organizations.
Longitudinal research
Despite some very early records of longitudinal research overviewing the history and the fundamentals of this methodology, Rajulton ( 2001 ) says that it was not until the 1920s that more significant longitudinal studies started to be found, allowing the science of development and growth to be advanced.
An early definition of longitudinal research is given by Baltes ( 1968 ), he contrasts longitudinal and cross-sectional research and defines the former as observing one sample at different measurement points (pp. 146–147). Ployhart and Vandenberg ( 2010 ) take a step back, they discern between the terms static and dynamic before attempting to define longitudinal research, they relate the former with cross-sectional methods and the latter with longitudinal ones. Similarly, Rajulton ( 2001 ) states that cross-sectional information is concerned with status, and longitudinal information deals with progress and change in status.
However, one interesting definition offered by Taris ( 2000 ) is that longitudinal research happens when “data are collected for the same set of research units for (but not necessarily at) two or more occasions, in principle allowing for intra-individual comparison across time” (pp. 1–2). Additionally, Ployhart and Vandenberg ( 2010 ) focus on the quantity of observations when they say that longitudinal research is “research emphasizing the study of change and containing at minimum three repeated observations (although more than three is better) on at least one of the substantive constructs of interest” (p. 97). Acknowledging the two previous definitions and its weaknesses, Wang et al. ( 2017 ) argue that longitudinal research is not necessarily focused on intra-individual analysis and cite examples where two waves of data collection is an appropriate procedure (e.g., prospective design), thus claiming an alternative definition: “longitudinal research is simply research where data are collected over a meaningful span of time” (p. 3).
Although definitions and tools seem to be improving in the past years, it was not always like this. Reflecting on the challenging past decades for the reliability of longitudinal research, particularly the 1960s and 1970s, Singer and Willett ( 2003 ) said that although scientists had always been fascinated with the study of change, it was only after the 1980s that the subject could be studied well due to new methodological tools and models developed.
Given the analytical problems at the time, Rogosa et al. ( 1982 ) clarifies misconceptions about measuring change, especially in terms of the pretest-posttest design, and encourage researchers to use multiple waves of data. They claim that “two waves of data are better than one, but not much better” (p. 744). Contrary to the thinking expressed in previous decades, Rogosa and Willett ( 1983 ) demonstrate the reliability of difference scores, which are typically used in two-wave designs, in the measurement of change for some cases (e.g., individual growth), though they do not claim the score to have high reliability in general.
Coming from an education and psychological perspective, Willett ( 1989 ) demonstrates that significant increases in the reliability of individual growth measures can be harnessed by incrementing data collection with a few additional waves of information beyond two. Aware of the methodological problems and the current conversation, Chan ( 1998 ) proposed an integrative approach to analyze change focused on the organizational context embodying longitudinal mean and covariance structures analysis (LMACS) and multiple indicator latent growth modeling (MLGM). He expressed his ideas in a less technical way, which facilitated the progress of the field.
Ployhart and Vandenberg ( 2010 ) raise key theoretical, methodological, and analytical questions when it comes to developing and evaluating longitudinal research in management. And using a panel discussion format, Wang et al. ( 2017 ) build on the same structure with the purpose of helping researchers make informed decisions in a non-technical way.
Longitudinal leadership development research
A pioneer initiative of longitudinal LD studies is the Management Progress Study (MPS) initiated by the Bell System (AT&T) in 1956 with the purpose of analyzing the growth, mostly in terms of status, of 422 men (Bray, 1964 ). Interesting follow ups were conducted after 8 and 20 years making this project one of the most popular field researches in management development (Day, 2011 ).
Attempting to longitudinally analyze a new generation of executives in 1977, A. Howard and D. Bray launched the Management Continuity Study (MCS). This ambitious project replicates many aspects of the MPS, but it also addresses weaknesses such as the lack of representation of women and different ethnicities (Howard & Bray, 1988 ). The MCS sample was used by many other longitudinal scholars to obtain stimulating insights, for instance, how successful male and female executives deal with power (Jacobs & McClelland, 1994 ), and the influence of college experiences on progress and performance (Howard, 1986 ).
In parallel with these two major longitudinal efforts, an Eastern perspective contributes significantly to the field of longitudinal LD. The Japanese Career Progress Study originated in 1972 is a sample of 85 male college graduates starting their careers at a leading Japanese department store chain who were followed up after 7 years (Wakabayashi & Graen, 1984 ) and 13 years (Wakabayashi et al., 1988 ) mostly in terms of promotion, salary, and performance. The multilevel and mixed-method approach with multiple waves of data revealed, in aggregation, that the organizational assessment of management potential of newcomers, the quality of exchange with superiors, and their early job performance predicted speed of promotion, total annual salary, and annual bonus on the seventh and thirteenth year of tenure. Wakabayashi et al. ( 1988 ), in a summarizing tone, state that the first three years of employment are critical when it comes to later career progress and leadership status up to 13 years.
After these pioneers, more LD longitudinal works started to emerge. Perhaps the biggest contribution to the area is the publication of a special issue in 2011 by the Leadership Quarterly . Authors of the referred issue promote important discussions and advance thought-provoking insights. In particular, the importance of true longitudinal studies, the ones involving three of more waves of data collection (Day, 2011 ), as well as the benefits of analyzing leadership through a long-lens approach (Murphy & Johnson, 2011 ). Specifically, the special issue explored childhood and adolescence factors. For instance, Gottfried et al. ( 2011 ) studied the motivational roots of leadership and found that children and teenagers with higher academic intrinsic motivation are more likely to want to lead as adults. Similarly, Guerin et al. ( 2011 ) found that adolescent extraversion predicts leadership potential over a decade later in adulthood with the relationship being fully mediated by adult social skills. Furthermore, the special issue explored family aspects in relation to LD. Oliver et al. ( 2011 ) are the first to connect family environment in childhood to adulthood leadership. Specifically, they found that a supportive and stimulating family atmosphere led to transformational leadership qualities in adulthood through positive self-concept. Li et al. ( 2011 ) detected that higher family socioeconomic status negatively influences leader advancement for females. The opposite was observed for males.
Apart from the larger longitudinal efforts mentioned above, many independent LD studies that rely on their own longitudinal samples contributed significantly to the field too. They vary greatly in settings and concepts, but some early important contributions seem to be Atwater et al.‘s ( 1999 ) demonstration that military leader emergence and leader effectiveness can be predicted by individual differences such as cognitive ability, physical fitness, and prior influence experience. Focused on the followers instead of the leaders, Dvir et al. ( 2002 ) suggest that transformational leadership training leads to followers’ development and performance. Also, executives’ competence, judged by self and others, significantly improves after multi-rater multi-source feedback (Bailey & Fletcher, 2002 ).
Other notable contributions involve the influence of self-regulation training on LD (Yeow & Martin, 2013 ), mentoring as a tool to develop not only the mentee (Lester et al., 2011 ), but also the mentor (Chun et al., 2012 ), and more unorthodox views such as dark personality traits and performance (Harms et al., 2011 ). However, some authors seem to be not only focused on behavioral, but also cognitive change (e.g., leader identity). Day and Sin ( 2011 ) claim that individuals with a strong leader identity are more effective across time. By using a university sample, Miscenko et al. ( 2017 ) propose that leader identity develops in a J-shaped pattern and that leader identity development is associated with leadership skills development. On the other hand, high-potential executives seem to develop leader identity in a linear and progressive way (Kragt & Day, 2020 ).
Methodology
Type of review and sources of evidence.
Despite being more widely seen, systematic reviews are best suited to approach specific questions addressing effectiveness, appropriateness, meaningfulness, and feasibility of particular interventions (Munn et al., 2018 ), and given this study’s broader research question, a scoping review was chosen. This method is usually defined as a mapping process (Arksey & O’Malley, 2005 ) or a system for synthesizing evidence (Levac et al., 2010 ). More recently, it was described as a “systematic way to map evidence on a topic and identify main concepts, theories, sources, and knowledge gaps” (Tricco et al., 2018 , p. 467). Despite the differences, both types of reviews are quite related, Moher et al. ( 2015 ) even see them as part of the same “family”.
The execution of each step of the current review was guided by the methodology initially laid out by Arksey and O’Malley ( 2005 ) and by the PRISMA extension for scoping reviews (PRISMA-ScR) and its corresponding checklist (Tricco et al., 2018 ). Following recommendations that a scoping review should be as comprehensive as possible (Arksey & O’Malley, 2005 ), different sources were used: (1) Online databases were searched (e.g., Web of Science, Scopus); (2) gray literature was identified (e.g., subject experts were consulted); (3) reference lists of key studies were cross-checked; and (4) handsearch of leading leadership journals was performed.
Search strategy for online databases: building search strings and identifying databases
Significant time was spent building the search strings for the present work as this is seen as a wise choice to improve search efficiency (Denyer & Tranfield, 2009 ). According to Arksey and O’Malley ( 2005 ) the process starts by having the research question in mind and identifying the key concepts that are present, in this case, longitudinal , leadership , and development . Based on this initial process, synonyms for each concept were identified. For instance, since the term “leadership” can be often substituted in the literature by management, executive, supervisory, and potentially others, these variations were added to the search string. Similarly, the term “development” can be substituted by training, program, intervention, and potentially others, thus these variations were incorporated as well.
In addition to identifying synonyms, this search strategy took into consideration some other concepts that seem to be highly associated with LD such as coaching, mentoring, and 360-feedback (Day, 2000 ). Hence, these terms plus their variations were incorporated. Finally, the search strategies and the specific keywords of past LD systematic reviews were screened (e.g. Collins & Holton, 2004 ; Lacerenza et al., 2017 ; Vogel et al., 2020 ) to verify any potential blind spots concerning the terms to be used here. In practical terms, seven different search strings were necessary to capture the process described. The first search string is completely detailed as follows and the remaining search strings are available in Appendix A .
Search 1: longitudinal AND (“leader* development” OR “manage* development” OR “executive development” OR “supervisory development” OR “team development” OR “human resource$ development”) .
The search strategy and the definition of keywords were verified by a professional librarian at ISEG – University of Lisbon. Feedback and other suggestions were given over a one-hour videocall in March of 2021.
One additional decision when it comes to the search strategy is identifying the databases to be used. Systematic review guidelines seem confident that authors must search more than one database (Liberati et al., 2009 ), others generally suggest that two or more are enough (Petticrew & Roberts, 2008 ), but little guidance is available for precisely deciding when to stop the searches, especially in the context of scoping reviews in social sciences instead of systematic reviews in medical sciences (e.g., Chilcott et al., 2003 ).
Considering this situation, searches started in a highly ambitious way in terms of quantity of databases and search restrictions (e.g., filters), and were iteratively pondered according to the reality of executing the work given the colossal volume of data for two authors with limited resources to go through. The described strategy seems aligned with both earlier (Arksey & O’Malley, 2005 ) and more recent recommendations (Peters et al., 2020 ) for authors writing scoping reviews as it is thought that comprehensiveness should be framed within the constraints of time and resources available to the authors. In this way, five databases were used: Web of Science, PsycARTICLES, Ebsco’s Business Source Complete, JSTOR, and Elsevier’s Scopus. The databases were mostly hand curated based on relevancy for LD. In other words, WoS has been extensively used by authors published in high-caliber leadership journals such as the Leadership Quarterly , and on some cases it is the only source of information (Vogel et al., 2020 ). PsycARTICLES seems unavoidable in psychological research, and it is found in most reviews at top-ranked journals interested in LD such as the Journal of Applied Psychology , for instance. Business Source Complete, Scopus, and JSTOR went through a similar curation process in addition to being well-known and comprehensive sources of information across social sciences disciplines.
Inclusion criteria
Three essential criteria served as pre-requisites for document inclusion in light of the research question.
Method: Is it a true longitudinal study (three or more waves of data) as opposed to a cross-sectional or a pretest-posttest one?
Context: Is the work approaching a business context? This study is interested in understanding longitudinal contributions to LD within a “business context”, which is an umbrella term created to incorporate for-profit and nonprofit companies, public organizations, and graduate students associated with management (e.g., MBA, executive education) or closely related areas (e.g., economics, organizational psychology). In this way, numerous LD studies involving sports, healthcare, and military contexts were naturally excluded from the final sample.
Concepts and measures: Is the study actually measuring change in terms of LD? Only results incorporating LD as a primary variable were considered. In this way, the authors were interested in analyzing leadership-related outcomes (e.g., leadership efficacy, leader identity), and not more distant concepts (e.g., job performance).
Only documents from 1900 until 2021 in English language were considered. Even though LD was not a formal research area in the early or mid-1900s, when the field “all years” is selected before a search in most databases, the range set by default starts in 1900. For clarification purposes, the earliest study analyzed in the present work dates to 1986.
On a more technical note, different filters according to the database at hand were used to refine the results (e.g., subject area, document type). As an example, the present research is not interested in LD in the sports space or document types such as editorials or reviews, thus filters were used to aid this refinement process. This whole procedure is consistent with the idea proposed by Levac et al. ( 2010 ) that the inclusion and exclusion criteria should be iterative and adapted based on the challenges identified.
Additional sources of information
Almost all the way through the screening execution, the authors of this study learned that scoping review researchers are encouraged to explore other sources of information apart from databases (Arksey & O’Malley, 2005 ; Peters et al., 2020 ). As a result, three à posteriori procedures were used to add evidence: (1) identifying gray literature through contacting subject experts, (2) cross-checking reference lists of important studies, and (3) handsearching key bibliographies and journals. Although the standard procedure for systematic reviews is to include articles from additional sources before the start of the screening process (Liberati et al., 2009 ), it is believed that the inverted execution does not threat the soundness of this work since adding and subtracting results before or after cannot affect the final sum and considering the iterative nature of scoping reviews (Levac et al., 2010 ). The only unfortunate implication observed was an extra load of work given the necessity to do an additional round of screening instead of screening all in once.
When it comes to consulting subject-matter experts, a list of a dozen high-level names was put together (e.g., D. Day, J. Antonakis, C. Lacerenza, L. Dragoni, R. Reichard) and the individual email outreach was executed in June of 2022. The email text to the list of authors included a brief personal introduction, the reason for contact and descriptions of the request, and a gratitude note for the impact of their work on this author’s academic journey.
Despite some prompt and friendly replies from high-caliber authors, including D. Day, who is considered a seminal scholar in LD, and also J. Antonakis, who was the chief editor of the Leadership Quarterly journal at the time of contact, no gray documents could have been added for multiple reasons varying from email bounces, no replies, replies from authors with no suggestions in mind, or irrelevant suggestions for this particular research question.
In addition to the step above, reference lists of key studies were cross-checked. First, pivotal review studies in LD (e.g., Day et al., 2014 ; Lacerenza et al., 2017 ) had their reference lists analyzed. Then, selected articles were further evaluated and selected based on screening of title, keywords, abstracts, and, ultimately, full-text analysis.
Finally, handsearching, a legitimate process in systematic literature reviews (Liberati et al., 2009 ), including scoping reviews (Tricco et al., 2018 ), was performed. Eight journals labeled “dominant” based on a co-citation analysis of LD (Vogel et al., 2020 ) were handsearched as an additional attempt to locate relevant evidence. The Academy of Management Review was part of this list, but naturally excluded from this process as no empirical works would have been found there, so the seven journals analyzed were Leadership Quarterly , Journal of Applied Psychology , Academy of Management Learning & Education , Personnel Psychology , Leadership , Journal of Organizational Behavior , and Journal of Management.
In terms of execution, central terms for the present research question (e.g., leadership development, longitudinal) were typed into the general search boxes of these journals and the list of results were scanned. Documents indicating good fit were further analyzed via screening of abstract and keywords, and full text. When searching the Leadership Quarterly journal, particular attention was devoted to a special issue published in 2011 centered on longitudinal leadership development studies (volume 22, issue 3). The handsearch process generated results as two articles that would not have been found otherwise were included in the sample for respecting the determined criteria (Cherniss et al., 2010 ; Dragoni et al., 2014 ).
Data charting process
Referred to as “data extraction” in systematic reviews, data charting (Arksey & O’Malley, 2005 ) is the process of extracting information from the sample in a scoping review. Even though any information can be charted in practice, researchers ideally should obtain pieces of information that help answer the research question (Levac et al., 2010 ). Given this ponderation and the research question at hand, a data charting framework was created to keep a consistent extraction standard across studies.
Nature of variables (e.g., quantitative, qualitative).
Research strategy (e.g., experiment, survey).
Participants (e.g., sample size, gender distribution).
Setting (e.g., industry, company information).
Intervention (e.g., program characteristics).
Research procedures (e.g., comparator, waves of data).
Outcome measures (e.g., variables, instruments).
Analytical approach (e.g., strategy, techniques).
Despite the primary focus on methodological choices of longitudinal LD studies, it was judged important to also chart the key findings of each study given the underlying motivation of the present research to contribute to the longitudinal LD field. A separate table (Table 2 ) was created to map this information. The data charting process took place with the assistance of Microsoft Excel.
Search results
Taking into consideration the search strategy and the inclusion criteria described previously, the WoS database returned 673 results. PsycARTICLES, in turn, retrieved 84 results. Next, Ebsco’s Business Source Complete returned 332 documents. JSTOR found 49 articles. Lastly, Elsevier’s Scopus retrieved 98 results. In total, 1236 documents were found. After removal of duplicates, a total of 1023 articles were screened given the determined criteria. The screening of titles, abstracts, and keywords removed 810 works, and screening the full text removed another 196 works, resulting in 17 included studies. À posteriori inclusion based on conversations with LD experts and handsearch of bibliographies and journals added another two documents, confirming a final sample of 19 articles. This whole process is illustrated by the flow chart below (Fig. 1 ).
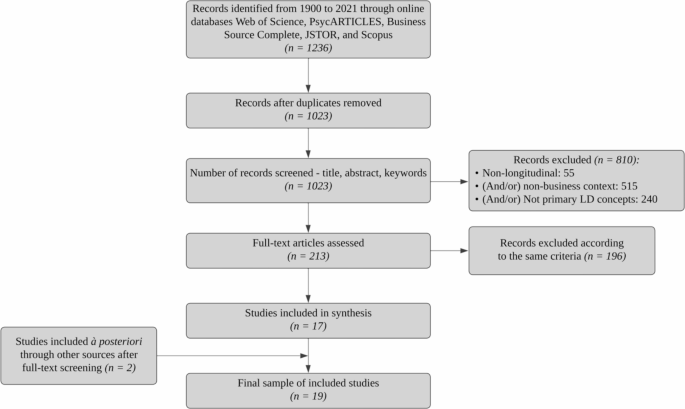
PRISMA flowchart: Search and inclusion process
General characteristics
The table listing the 19 documents and some of their basic characteristics can be found in Appendix B . The works comprise different years, journals, countries, and authors. The first true longitudinal study of LD in a business context was published in 1986 by the Journal of Applied Psychology . One noticeable feature of the table found in Appendix B is the substantial 22-year gap in publications from 1988 to 2010. After 2010, on the other hand, researchers seem to have found more efficient ways to collect longitudinal data, and until 2021, on average 1.42 studies were published every year. Despite the progress, compared to past decades, the number is still quite modest given the importance of true longitudinal studies to the science of LD (Day, 2011 ).
In terms of outlets, eleven different journals represent the sample. The pioneer on the subject and methodology is clearly the Journal of Applied Psychology . The most dominant journal is the Leadership Quarterly with five publications. In terms of countries, the United States lead the list with twelve publications. The United Kingdom has five, Germany and Switzerland have one publication each. Professor D. Day contributes to four articles (2020, 2018, 2017, 2011), which is a considerable achievement given this highly selective sample. Moreover, G. Larson, C. Sandahl, and T. Soderhjelm contributed twice (2017, 2019). All other authors contributed once.
How true longitudinal LD studies have been conducted methodologically and what inconsistencies exist?
The research question is addressed following two recommended stages, a description of the characteristics and a thematic analysis (Levac et al., 2010 ). These two steps are assessed below.
Characteristics
Table 1 helps to address the research question of this study which is to evaluate how true longitudinal studies of LD are being investigated and what inconsistencies exist, primarily from a methodological perspective.
First, in terms of the nature of variables and strategy, the vast majority were quantitative (16), two studies utilized mixed methods, and only one used qualitative data (Andersson, 2010 ). This study’s criteria yielded a majority of experimental and survey strategies. However, archival data, narrative inquiry, observation, and action learning are represented as well.
Collectively, the studies form a sample of 2,776 participants. This number represents respondents that answered all longitudinal measures, thus drop-out participants, who have perhaps answered only the first measure and not the following ones, were not counted. In terms of sex, this combined sample is composed by 67% of males. The more recent studies seem to be more balanced in terms of gender though. In total, 88 waves of data were collected across all studies, resulting in an average of 4.2 waves per study. The maximum value observed is 13 waves of data (Middleton et al., 2019 ). The longest study lasted 20 years between first and last data collection (Howard, 1986 ) and the shortest study lasted 4 weeks (Quigley, 2013 ).
When it comes to the contextual settings, 6 publications researched one single company, 7 authors gathered participants from two or more companies, and 6 studies analyzed business students, mostly MBA students with work experience. The targeted companies, to cite only a few examples, were quite diverse, ranging from a large Australian corporation with more than 200,000 employees (Kragt & Day, 2020 ); to a museum leader development program with global participants (Middleton et al., 2019 ); to a multinational Indian-based IT company (Steele & Day, 2018 ); to middle managers of the headquarters of a regional grocery store chain in the United States. As for business students, the sample includes, among others, a top-ranked MBA program at a Spanish business school (Mayo et al., 2012 ); full-time MBA students at a large American university; and a graduate degree at a Dutch business school (Miscenko et al., 2017 ).
No form of intervention was found in 6 studies. The remaining 13 studies applied different LD trainings that varied in (1) length, ranging from 90 minutes to 145 hours; (2) content focus such as self-regulation, influence, feedback, team effectiveness; and (3) methods like lecture, role-play, discussion, readings, coaching.
By taking a look at the LD outcome measures, it is noticed that the two early studies of the sample, the ones that belong to the 1980s, were preoccupied with measuring some form of status, for instance career progress in terms of speed of promotion, and level of management achieved. After 2010, the focus of analysis changes from status to either cognitive outcomes (leader identity, self-perceived role knowledge) or behavioral outcomes (skills, competencies, efficacy). Established instruments and developed measures are both present.
Changing the conversation to the analytical approach of these works, it seems that it was not until 2011 that more appropriate procedures for longitudinal modelers started to emerge. This raises the question if more true longitudinal studies emerged because of more suitable tools available, or if these new tools were created given the importance to research human development in a longitudinal way.
Before 2011, the sample indicates the use of multiple regression equations, correlation analyses, ANOVAs, and ANCOVAs. After that year, an emergence and consolidation of more sophisticated methods is observed, like random coefficient modeling (RCM), latent growth model (LGM), multilevel modeling (MLM), hierarchical multivariate linear modeling (HMLM). In terms of the software tools used to execute these analyses, SPSS, R, HLM, NLME are highlighted.
Despite the present focus on methodologies, it was judged relevant to additionally chart the key findings of the studies included in this review. Table 2 maps this information chronologically by author.
Themes were driven by the concepts, or the objects of analysis being used by scholars and derived by examining the “LD outcome measure” column of Table 1 as well as the full study. Specifically, a summarized thematic analysis was performed (Braun & Clarke, 2006 ). Variables were grouped together based on similarity. For instance, self-confidence and leadership efficacy are measuring behavioral change, hence a category called “behavioral” was created. Following this line of thinking, variables such as leader identity and self-perceived role knowledge are measuring cognitive change, thus the category “cognitive”. The same process was applied for the status category. After this procedure, the quantity of studies in each category was simply counted. Some studies are measuring more than one dimension, as shown below in Fig. 2 .
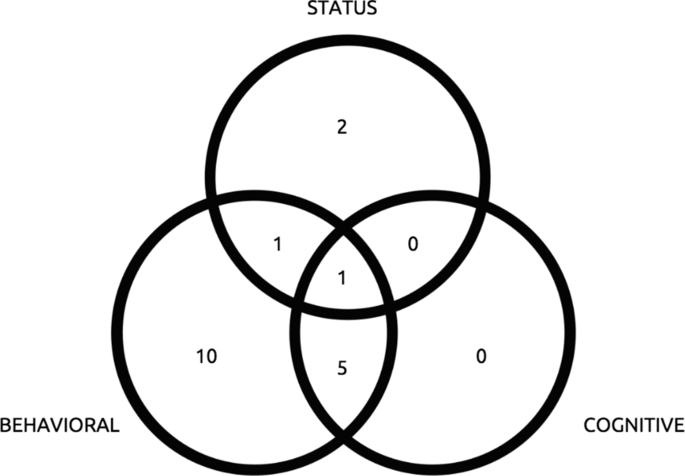
Venn diagram of main themes identified by quantity of studies
As observed, most scholars are, not surprisingly, interested in researching behaviors, maybe because it is an inherent aspect of the organizational behavior field. The behavioral dimension is also the only one to intersect with the other two that emerged. Status outcomes were the primary variable for only two studies. And although no studies analyzed cognitive outcomes alone, researchers seem interested in understanding these factors as it greatly intersects with the behavior sphere. Lastly, only one true longitudinal study of LD measured all three categories (Kragt & Day, 2020 ). Table 3 provides more information based on these themes.
The themes reveal some interesting aspects. First, measuring status as a primary outcome is linked to older publications while the cognitive and behavioral dimensions are more recent concepts of interest. The status dimension is also associated with less waves of data but longer length of study in general. The opposite happens for studies focused on behavioral and cognitive aspects, they are characterized by collecting more waves of data in less time.
Even though the goal of this research is to analyze only business contexts, some diversity is observed in terms of specific setting (e.g., business schools, large companies, partnerships with consultancy firms), and location (e.g., USA, Europe, Australia, Japan, India). Except for India, no developing countries are observed, suggesting a potential research need.
In terms of strategies and interventions, conducting experiments is associated with the more recent studies. A lack is qualitative methods is also noticed. Additionally, the survey strategy is always present across the three themes. No standard regarding the type of intervention is detected, they are mostly trainings with slightly different areas of concentration.
The two studies focusing on status used more general analytic tools such as multiple regression and ANOVA analysis. More sophisticated tools are observed across the other two spheres and their intersections (e.g., LGM, RCM, HLM).
The evidence indicates that the longitudinal LD area is young with the vast majority of studies being published after 2010. The combined sample sums 2,776 participants (67% male) and 88 waves of data. Most of these studies are quantitative, and mostly surveys or experiments. The context, as expected, is very much managerial and composed mostly by large companies and business schools in developed countries. Regarding LD outcomes, three major themes were found, status (e.g., level of leadership attained), behavioral (e.g., leadership effectiveness), and cognitive (e.g., leader identity).
Scoping reviews have the power to map a field of knowledge making gaps more evident (Arksey & O’Malley, 2005 ). In this way, it is not difficult to notice that no developing countries are represented except from India, smaller companies are also not represented, and women are underrepresented as they compose one third of this review’s combined sample. Considering that leadership is highly contextual (Johns, 2006 ), it is understood that, if supported by insights originated from diverse contexts, the field could make significant progress in terms of bridging LD science and practice (Day et al., 2018 ).
Moreover, it is concerning to see almost no qualitative studies in this review. Despite the challenges associated with conducting longitudinal qualitative research in the social sciences (Thomson & Holland, 2003 ), this methodology has the potential to enrich the LD field with deeper insights. One promising path seems to be multiple perspective qualitative longitudinal interviews (MPQLI) (Vogl et al., 2018 ), a framework created to analyze related individuals (e.g., one’s peers, superiors, subordinates) and to deal with complex and voluminous data. Another hopeful avenue of research for LD is through the underdeveloped area of mixed methods longitudinal research (MMLR) (Vogl, 2023 ). The current study has been relying on the assumption that longitudinal designs are the most appropriate way to study LD (Day, 2011 ). Building on this and being more specific, MMLR may be even more appropriate to understand and explain LD given the complementary insights generated (Vogl, 2023 ). However, applying this type of methodology comes with a series of issues as well as high execution effort that need to be taken into consideration by future scholars (Plano Clark et al., 2015 ).
One additional issue associated with longitudinal research is deciding how many waves of data to collect and what is the ideal length of interval between measurement points (Ployhart & Vandenberg, 2010 ). In the present study, it is difficult to recognize any corresponding standard among the experimental studies. Some authors seem to be following the intervention’s length, for instance, Miscenko et al.‘s ( 2017 ) 7-week leadership program collected data at seven weekly time points, but the vast majority of studies do not offer explanations for the choices made. Even though most of these decisions are atheoretical and the ideal time interval is rarely known because it greatly depends on the phenomenon of interest, Wang et al. ( 2017 ) say this is a critical matter because it directly affects the change trajectory. Therefore, the science of longitudinal leadership research could benefit from more information about the decision rationale given the variables at hand. For example, for which kinds of leadership phenomena longer lengths are more valuable and vice versa? How many waves of data would be more suitable according to concept, levels of analysis, or research goals?
Regarding concepts, data shows that scholars are less interested in measuring status-related concepts (e.g., hierarchical level achieved), while behavioral variables are the most popular ones and cognitive variables can be considered emerging. Although each study naturally uses variables that are coherent with their research questions, the three dimensions presented earlier (Fig. 2 ) offer different and valuable perspectives to the development of leaders and leadership, so it is judged beneficial to cross dimensions whenever possible. For example, Kragt and Day ( 2020 ) is the only study that sheds light on status (e.g., promotion), behavior (e.g., managing stress), and cognitive aspects (e.g., leader identity).
As a summary, this paper contributes to theory in several ways. First, through mapping the methods being used to date; second, by identifying inconsistencies and gaps; third, by elaborating on ways in which the leadership field can advance; fourth, by understanding themes in terms of outcome variables; and lastly, through insights for management scholars and practitioners given the exclusive focus on business contexts.
Limitations
The present work is not immune to limitations, as no scientific work is. This study includes documents up to the year 2021, resulting in a three-year gap considering the submission date to this journal. Significant personal circumstances prevented the authors from pursuing publication earlier, so to mitigate this potential limitation, a modest cursory review is presented as described. Searching the Web of Science database from 2022 to 2024 using the seven search strings outlined in Appendix A , a list of 116 documents were gathered. Following the PRISMA-ScR framework (Tricco et al., 2018 ), records were screened (abstract and/or full text) based on the same pre-determined criteria described in the methodology section. Even though 12 records were closely assessed, only 2 peer-reviewed articles respected the parameters. They are identified below followed by a summarized discussion.
“How coaching interactions transform leader identity of young professionals over time” published in the International Journal of Evidence Based Coaching and Mentoring by Hughes and Vaccaro ( 2024 ) was the first record identified. This qualitative exploration utilizing semi-structured interviews before, during and after the coaching experience highlights through narrative inquiry analysis how coaching that is grounded in identity transformation practices are an important mechanism for emerging leaders as they navigate high degrees of professional and personal change in their lives. Despite the small sample size (six coaches), the three-phase data collection can be considered rare in qualitative studies of leadership development, representing a strength.
“Perceived changes in leadership behavior during formal leadership education” published in Public Personnel Management by Sørensen et al. ( 2023 ) was the second record identified. This multilevel three-year study with 62 leaders and 860 respondents found that leadership education has a considerable effect on leadership behaviors when it comes to tasks, relations, and change. Among the highlighted insights is the interesting fact that subordinates rated change in leadership behavior significantly lower compared to superiors and peers.
In addition to the limitations presented so far, scoping reviewers are encouraged to initially conduct the data charting process with at least two scholars working independently (Levac et al., 2010 ) and this was not possible to accomplish in the present study. Although agreeing with the above-mentioned recommendation, it is believed that the findings are not threatened by not executing this step, as the main motivation for it seems to be saving time when it comes to including studies. Thus, the only drawback for the current research was making the data charting process longer than it could have been.
The attempts to include gray literature were restricted to contacting LD subject-experts, which is a valid and effective strategy (Petticrew & Roberts, 2008 ), but there are additional tactics that could potentially lead to a larger sample. One example would be searching online databases for theses and dissertations around the theme. Future studies are encouraged to address that.
The experience of conducting a scoping review was perceived as “too manual”. Despite the confidence in the present results, it is difficult to ensure the inexistence of minor oversights as the process involved multiple Excel documents with dozens of tabs and thousands of lines each. Using a software was unfortunately not an option for the present study, but researchers interested in scoping reviews should consider using one.
The focus of the current review was purposefully restricted to business contexts. Although this is beneficial to the present goal and to obtain more specific insights, it leads to low generalizability power. Including studies from other LD contexts such as healthcare, military, and sports, can offer a good opportunity to learn across disciplines and potentially identify synergies for the benefit of leadership research as a whole.
Future research
Regarding the limitations highlighted above, it is encouraged that LD scholars conducting scoping reviews to focus on working within larger teams of colleagues as some scoping review procedures can be quite lengthy depending on the protocol chosen (e.g., a truly extensive search, data charting). Most of the limitations identified above could have been solved by that. And referring again to how data could not be obtained past 2021 for this study, it is encouraged that researchers engaged with scoping reviews include the most up to date records whenever possible.
Despite the search comprehensiveness demonstrated here, the present sample is relatively small. So, even though it is unknown if a larger sample is possible to achieve given this study’s scope, scholars are still encouraged to try to include more articles. Specifically, through searching more than five online databases, trying to expand the search for gray literature, and, if possible, performing searches in languages in addition to English.
Changing the conversation from the methodology of scoping reviews to the actual methodological contents of the sample, one gap that is easily noticed is the lack of qualitative or mixed-method studies, therefore these designs are encouraged for an enhanced perspective of LD in business contexts. Qualitative research has been growing strong in management science due to the value of their rich insights (Bluhm et al., 2011 ) and it seems that the LD field has plenty of space to leverage this opportunity. This is not to say that more quantitative designs are not needed, but right now it seems that the field can significantly grow from qualitative and mixed-methods contributions.
For sponsored authors or authors with a higher budget and a more numerous team, it would be interesting to conduct a scoping review similar to this one but not restricted to the business context as insights from other fields like health sciences, sports, education, military can help advance the science of LD. It would finally be interesting for a future scoping review of LD to organize the research through levels of analysis, namely intraindividual change, group change, and organizational change.
Even though the most recent studies analyzed by this scoping review worked with more gender balanced samples, male participants are predominant overall, hence future research is encouraged to continue working with a balanced proportion of males and females. Alternatively, all-female samples could leverage new insights as no studies under the current criteria have explored this angle yet. Relatedly, the LD field could unlock novel contributions by going beyond sex in terms of demographic characteristics. For example, age, race, social class, and gender identity are potentially good opportunities to extend knowledge.
The present scoping review intended to understand how true longitudinal studies of LD are being researched and what inconsistencies exist, primarily from a methodological perspective. After a rigorous search process ranging from 1900 to 2021, evidence was extracted from 19 peer-reviewed articles set in business contexts and measuring LD change with at least three waves of data. The current study elucidates gaps, patterns, and inconsistencies in terms of many aspects including nature of data, research strategy, participants, waves of data, concepts, analytical techniques, and key findings. Some observed highlights include the pattern to measure behavioral concepts and the emergent interest in measuring cognitive concepts. The procedures of the most recent works are shorter in length and more numerous in waves of data, the opposite was true a few decades ago. More sophisticated analytical techniques have been used in recent years as the field understands LD as a developmental science and art. However, there is an overreliance on quantitative methods leading to a bright future for qualitative and mixed-methods longitudinal researchers. Given the historical gender imbalance in participants studied (combined sample is 67% male), balanced or all-female samples can lead to original insights.
Search strings used in the five online databases .
Search 1 | longitudinal |
| |
“leader* development” OR “manage* development” OR “executive development” OR “supervisory development” OR “team development” OR “human resource$ development” | |
Search 2 | longitudinal |
| |
“leader* training” OR “manage* training” OR “executive training” OR “supervisory training” OR “team training” OR “human resource$ training” | |
Search 3 | longitudinal |
| |
“leader* program*” OR “manage* program*” OR “executive program*” OR “supervisory program*” OR “team program*” OR “human resource$ program*” | |
Search 4 | longitudinal |
| |
“leader* intervention” OR “manage* intervention” OR “executive intervention” OR “supervisory intervention” OR “team intervention” OR “human resource$ intervention” | |
Search 5 | longitudinal |
| |
“leader* education” OR “manage* education” OR “executive education” OR “supervisory education” OR “team education” OR “human resource$ education” | |
Search 6 | longitudinal |
| |
“leader* building” OR “manage* building” OR “executive building” OR “supervisory building” OR “team building” OR “human resource$ building” | |
Search 7 | longitudinal |
| |
coaching OR mentoring OR “360-degree feedback” OR “multi-source feedback” OR “multi-rater feedback” |
List of selected studies and basic details .
Author | Year | Title | Journal | Editor Country |
---|---|---|---|---|
Howard, Ann | 1986 | College Experiences and Managerial Performance | Journal of Applied Psychology | United States |
Wakabayashi, Mitsuru; Graen, George; Graen, Michael; Graen, Martin | 1988 | Japanese Management Progress: Mobility Into Middle Management | Journal of Applied Psychology | United States |
Seifert, Charles F.; Yukl, Gary | 2010 | Effects of repeated multi-source feedback on the influence behavior and effectiveness of managers: A field experiment | Leadership Quarterly | United States |
Andersson, Thomas | 2010 | Struggles of managerial being and becoming: Experiences from managers’ personal development training | Journal of Management Development | United Kingdom |
Cherniss, Cary Grimm, Laurence G. Liautaud, Jim P. | 2010 | Process-designed training: A new approach for helping leaders develop emotional and social competence | Journal of Management Development | United Kingdom |
Abrell, Carolin; Rowold, Jens; Weibler, Jürgen; Moenninghoff, Martina | 2011 | Evaluation of a Long-Term Transformational Leadership Development Program | Zeitschrift für Personalforschung | Germany |
Day, DV; Sin, HP | 2011 | Longitudinal tests of an integrative model of leader development: Charting and understanding developmental trajectories | Leadership Quarterly | United States |
Mayo, M; Kakarika, M; Pastor, JC; Brutus, S | 2012 | Aligning or inflating your leadership self-image? A longitudinal study of responses to peer feedback in MBA teams | Academy of Management Learning & Education | United States |
Quigley, Narda R. | 2013 | A Longitudinal, Multilevel Study of Leadership Efficacy Development in MBA Teams | Academy of Management Learning & Education | United States |
Yeow, J; Martin, R | 2013 | The role of self-regulation in developing leaders: A longitudinal field experiment | Leadership Quarterly | United States |
Dragoni, Lisa Park, Haeseen Soltis, Jim Forte-Trammell, Sheila | 2014 | Show and tell: How supervisors facilitate leader development among transitioning leaders | Journal of Applied Psychology | United States |
Baron, Louis | 2016 | Authentic leadership and mindfulness development through action learning | Journal of Managerial Psychology | United Kingdom |
Miscenko, Darja; Guenter, Hannes; Day, David V. | 2017 | Am I a leader? Examining leader identity development over time | Leadership Quarterly | United States |
Larsson, G; Sandahl, C; Soderhjelm, T; Sjovold, E; Zander, A | 2017 | Leadership behavior changes following a theory-based leadership development intervention: A longitudinal study of subordinates’ and leaders’ evaluations | Scandinavian Journal of Psychology | United Kingdom |
Steele, Andrea R.; Day, David V. | 2018 | The Role of Self-Attention in Leader Development | Journal of Leadership Studies | United States |
Sandahl C., Larsson G., Lundin J., Söderhjelm T.M. | 2019 | The experiential understanding group-and-leader managerial course: long-term follow-up | Leadership and Organization Development Journal | United Kingdom |
Middleton, ED; Walker, DO; Reichard, RJ | 2019 | Developmental Trajectories of Leader Identity: Role of Learning Goal Orientation | Journal of Leadership & Organizational Studies | United States |
Kragt, D; Day, DV | 2020 | Predicting Leadership Competency Development and Promotion Among High-Potential Executives: The Role of Leader Identity | Frontiers in Psychology | Switzerland |
D’Innocenzo, L; Kukenberger, M; Farro, AC; Griffith, JA | 2021 | Shared leadership performance relationship trajectories as a function of team interventions and members’ collective personalities | Leadership Quarterly | United States |
Data availability
The authors declare that the data is available upon request.
Andersson, T. (2010). Struggles of managerial being and becoming: Experiences from managers’ personal development training. Journal of Management Development, 29 (2), 167–176. https://doi.org/10.1108/02621711011019305
Article Google Scholar
Arksey, H., & O’Malley, L. (2005). Scoping studies: Towards a methodological framework. International Journal of Social Research Methodology: Theory and Practice, 8 (1), 19–32. https://doi.org/10.1080/1364557032000119616
Arvey, R. D., Zhang, Z., Avolio, B. J., & Krueger, R. F. (2007). Developmental and genetic determinants of leadership role occupancy among women. Journal of Applied Psychology, 92 (3), 693–706. https://doi.org/10.1037/0021-9010.92.3.693
Article PubMed Google Scholar
Athanasopoulou, A., & Dopson, S. (2018). A systematic review of executive coaching outcomes: Is it the journey or the destination that matters the most? Leadership Quarterly, 29 (1), 70–88. https://doi.org/10.1016/j.leaqua.2017.11.004
Atwater, L. E., & Waldman, D. (1998). 360 degree feedback and leadership development. Leadership Quarterly, 9 (4), 423–426.
Atwater, L. E., Dionne, S. D., Avolio, B. J., Camobreco, J. F., & Lau, A. W. (1999). A longitudinal study of the leadership development process: Individual differences predicting leader effectiveness. Human Relations, 52 (12), 1543–1562.
Ayoobzadeh, M., & Boies, K. (2020). From mentors to leaders: Leader development outcomes for mentors. Journal of Managerial Psychology, 35 (6), 497–511. https://doi.org/10.1108/JMP-10-2019-0591
Bailey, C., & Fletcher, C. (2002). The impact of multiple source feedback on management development: Findings from a longitudinal study. Journal of Organizational Behavior, 23 (7), 853–867. https://doi.org/10.1002/job.167
Baltes, P. B. (1968). Longitudinal and cross-sectional sequences in the study of age and generation effects. Human Development, 11 , 145–171.
Bleidorn, W., Hill, P. L., Back, M. D., Denissen, J. J. A., Hennecke, M., Hopwood, C. J., Jokela, M., Kandler, C., Lucas, R. E., Luhmann, M., Orth, U., Wagner, J., Wrzus, C., Zimmermann, J., & Roberts, B. (2019). The policy relevance of personality traits. American Psychologist, 74 (9), 1056–1067. https://doi.org/10.1037/amp0000503
Bluhm, D. J., Harman, W., Lee, T. W., & Mitchell, T. R. (2011). Qualitative research in management: A decade of progress. Journal of Management Studies, 48 (8), 1866–1891. https://doi.org/10.1111/j.1467-6486.2010.00972.x
Braun, V., & Clarke, V. (2006). Using thematic analysis in psychology. Qualitative Research in Psychology, 3 (2), 77–101. https://doi.org/10.1191/1478088706qp063oa
Bray, D. W. (1964). The management progress study. American Psychologist, 19 (6), 419–420. https://doi.org/10.1037/h0038616
Chan, D. (1998). The conceptualization and analysis of change over time: An integrative approach incorporating longitudinal mean and covariance structures analysis (LMACS) and multiple indicator latent growth modeling (MLGM). Organizational Research Methods, 1 (4), 421–483. https://doi.org/10.1177/109442819814004
Cherniss, C., Grimm, L. G., & Liautaud, J. P. (2010). Process-designed training: A new approach for helping leaders develop emotional and social competence. Journal of Management Development, 29 (5), 413–431. https://doi.org/10.1108/02621711011039196
Chilcott, J., Brennan, A., Booth, A., Karnon, J., & Tappenden, P. (2003). The role of modelling in prioritising and planning clinical trials. Health Technology Assessment, 7 (23). https://doi.org/10.3310/hta7230
Chun, J. U., Sosik, J. J., & Yun, N. Y. (2012). A longitudinal study of mentor and protégé outcomes in formal mentoring relationships. Journal of Organizational Behavior, 33 , 1071–1094. https://doi.org/10.1002/job
Collins, D. B., & Holton, E. F. (2004). The effectiveness of managerial leadership development programs: A meta-analysis of studies from 1982 to 2001. Human Resource Development Quarterly, 15 (2), 217–248. https://doi.org/10.1002/hrdq.1099
Day, D. V. (2000). Leadership development: A review in context. Leadership Quarterly, 11 (4), 581–613. https://doi.org/10.1080/00336297.1981.10483720
Day, D. V. (2011). Integrative perspectives on longitudinal investigations of leader development: From childhood through adulthood. Leadership Quarterly, 22 (3), 561–571. https://doi.org/10.1016/j.leaqua.2011.04.012
Day, D. V. (2024). Developing leaders and leadership: Principles, practices, and processes. Palgrave MacMillan . https://doi.org/10.1007/978-3-031-59068-9
Day, D. V., & Dragoni, L. (2015). Leadership development: An outcome-oriented review based on time and levels of analyses. Annual Review of Organizational Psychology and Organizational Behavior, 2 , 133–156. https://doi.org/10.1146/annurev-orgpsych-032414-111328
Day, D. V., & Sin, H. P. (2011). Longitudinal tests of an integrative model of leader development: Charting and understanding developmental trajectories. Leadership Quarterly, 22 (3), 545–560. https://doi.org/10.1016/j.leaqua.2011.04.011
Day, D. V., Harrison, M. M., & Halpin, S. M. (2009). An integrative approach to leader development: Connecting adult development, identity, and expertise . Routledge. https://doi.org/10.4324/9780203809525
Book Google Scholar
Day, D. V., Fleenor, J. W., Atwater, L. E., Sturm, R. E., & McKee, R. A. (2014). Advances in leader and leadership development: A review of 25 years of research and theory. Leadership Quarterly, 25 (1), 63–82. https://doi.org/10.1016/j.leaqua.2013.11.004
Day, D., Riggio, R., Conger, J., & Tan, S. (2018). Call for papers - special issue on 21st century leadership development: Bridging science and practice. Leadership Quarterly, 29 (6), I. https://doi.org/10.1016/s1048-9843(18)30810-5
Denyer, D., & Tranfield, D. (2009). Producing a systematic review. In D. A. Buchanan & A. Bryman (Eds.), The SAGE handbook of organizational research methods (pp. 671–689).
Dragoni, L., Park, H., Soltis, J., & Forte-Trammell, S. (2014). Show and tell: How supervisors facilitate leader development among transitioning leaders. Journal of Applied Psychology, 99 (1), 66–86. https://doi.org/10.1037/a0034452
Dvir, T., Eden, D., Avolio, B. J., & Shamir, B. (2002). Impact of transformational leadership on follower development and performance: A field experiment. Academy of Management Journal, 45 (4), 735–744. https://doi.org/10.2307/3069307
Epitropaki, O., Kark, R., Mainemelis, C., & Lord, R. G. (2017). Leadership and followership identity processes: A multilevel review. Leadership Quarterly, 28 (1), 104–129. https://doi.org/10.1016/j.leaqua.2016.10.003
Gottfried, A. E., Gottfried, A. W., Reichard, R. J., Guerin, D. W., Oliver, P. H., & Riggio, R. E. (2011). Motivational roots of leadership: A longitudinal study from childhood through adulthood. Leadership Quarterly, 22 (3), 510–519. https://doi.org/10.1016/j.leaqua.2011.04.008
Guerin, D. W., Oliver, P. H., Gottfried, A. W., Gottfried, A. E., Reichard, R. J., & Riggio, R. E. (2011). Childhood and adolescent antecedents of social skills and leadership potential in adulthood: Temperamental approach/withdrawal and extraversion. Leadership Quarterly, 22 (3), 482–494. https://doi.org/10.1016/j.leaqua.2011.04.006
Harms, P. D., Spain, S. M., & Hannah, S. T. (2011). Leader development and the dark side of personality. Leadership Quarterly, 22 (3), 495–509. https://doi.org/10.1016/j.leaqua.2011.04.007
Howard, A. (1986). College experiences and managerial performance. Journal of Applied Psychology, 71 (3), 530–552. https://doi.org/10.1037/0021-9010.71.3.530
Howard, A., & Bray, D. W. (1988). Managerial lives in transition: Advancing age and changing times . Guilford Press.
Google Scholar
Hughes, A., & Vaccaro, C. (2024). How coaching interactions transform leader identity of young professionals over time. International Journal of Evidence Based Coaching and Mentoring, 22 (1), 130–148. https://doi.org/10.24384/3tw6-r891
Jacobs, R. L., & McClelland, D. C. (1994). Moving up the corporate ladder: A longitudinal study of the leadership motive pattern and managerial success in women and men. Consulting Psychology Journal: Practice and Research, 46 (1), 32–41. https://doi.org/10.1037/1061-4087.46.1.32
Johns, G. (2006). The essential impact of context on organizational behavior. Academy of Management Review, 31 (2), 386–408. https://doi.org/10.5465/AMR.2006.20208687
Johnson, C. D., & Routon, P. W. (2024). Who feels taught to lead? Assessing collegiate leadership skill development. Journal of Leadership Education, 23 (1), 50–65. https://doi.org/10.1108/jole-01-2024-0013
Judge, T. A., Bono, J. E., Ilies, R., & Gerhardt, M. W. (2002). Personality and leadership: A qualitative and quantitative review. Journal of Applied Psychology, 87 (4), 765–780. https://doi.org/10.1037/0021-9010.87.4.765
Judge, T. A., Colbert, A. E., & Ilies, R. (2004). Intelligence and leadership: A quantitative review and test of theoretical propositions. Journal of Applied Psychology, 89 (3), 542–552. https://doi.org/10.1037/0021-9010.89.3.542
Kragt, D., & Day, D. V. (2020). Predicting leadership competency development and promotion among high-potential executives: The role of leader identity. Frontiers in Psychology, 11 (August), 1–16. https://doi.org/10.3389/fpsyg.2020.01816
Lacerenza, C. N., Reyes, D. L., Marlow, S. L., Joseph, D. L., & Salas, E. (2017). Leadership training design, delivery, and implementation: A meta-analysis. Journal of Applied Psychology, 102 (12), 1686–1718. https://doi.org/10.1037/apl0000241.supp
Ladkin, D., & Taylor, S. S. (2010). Leadership as art: Variations on a theme. Leadership, 6 (3), 235–241. https://doi.org/10.1177/1742715010368765
Lester, P. B., Hannah, S. T., Harms, P. D., Vogelgesang, G. R., & Avolio, B. J. (2011). Mentoring impact on leader efficacy development: A field experiment. Academy of Management Learning and Education, 10 (3), 409–429. https://doi.org/10.5465/amle.2010.0047
Levac, D., Colquhoun, H., & O’Brien, K. K. (2010). Scoping studies: Advancing the methodology. Implementation Science, 5 (69), 1–9. https://doi.org/10.1017/cbo9780511814563.003
Li, W. D., Arvey, R. D., & Song, Z. (2011). The influence of general mental ability, self-esteem and family socioeconomic status on leadership role occupancy and leader advancement: The moderating role of gender. Leadership Quarterly, 22 (3), 520–534. https://doi.org/10.1016/j.leaqua.2011.04.009
Liberati, A., Altman, D. G., Tetzlaff, J., Mulrow, C., Gøtzsche, P. C., Ioannidis, J. P. A., Clarke, M., Devereaux, P. J., Kleijnen, J., & Moher, D. (2009). The PRISMA statement for reporting systematic reviews and meta-analyses of studies that evaluate health care interventions: Explanation and elaboration. Journal of Clinical Epidemiology, 62 (10), e1–e34. https://doi.org/10.1016/j.jclinepi.2009.06.006
Lord, R. G., & Hall, R. J. (2005). Identity, deep structure and the development of leadership skill. Leadership Quarterly, 16 (4), 591–615. https://doi.org/10.1016/j.leaqua.2005.06.003
Mayo, M., Kakarika, M., Pastor, J. C., & Brutus, S. (2012). Aligning or inflating your leadership self-image? A longitudinal study of responses to peer feedback in MBA teams. Academy of Management Learning and Education, 11 (4), 631–652. https://doi.org/10.5465/amle.2010.0069
McCall, M. W. (2004). Leadership development through experience. Academy of Management Executive, 18 (3), 127–130. https://doi.org/10.5465/AME.2004.14776183
McCall, M. W. (2010). Recasting leadership development. Industrial and Organizational Psychology, 3 (1), 3–19. https://doi.org/10.1111/j.1754-9434.2009.01189.x
Middleton, E. D., Walker, D. O., & Reichard, R. J. (2019). Developmental trajectories of leader identity: Role of learning goal orientation. Journal of Leadership and Organizational Studies, 26 (4), 495–509. https://doi.org/10.1177/1548051818781818
Mirvis, P. (2008). Executive development through consciousness-raising experiences. Academy of Management Learning and Education, 7 (2), 173–188. https://doi.org/10.5465/AMLE.2008.32712616
Miscenko, D., Guenter, H., & Day, D. V. (2017). Am I a leader? Examining leader identity development over time. Leadership Quarterly, 28 (5), 605–620. https://doi.org/10.1016/j.leaqua.2017.01.004
Moher, D., Liberati, A., Tetzlaff, J., Alttman, D. G., & The PRISMA Group. (2009). Preferred reporting items for systematic reviews and meta-analyses: The PRISMA statement. Annals of Internal Medicine, 151 (4), 264–269.
Moher, D., Stewart, L., & Shekelle, P. (2015). All in the family: Systematic reviews, rapid reviews, scoping reviews, realist reviews, and more. Systematic Reviews, 4 (1), 1–2. https://doi.org/10.1186/s13643-015-0163-7
Article PubMed PubMed Central Google Scholar
Muir, D. (2014). Mentoring and leader identity development: A case study. Human Resource Development Quarterly, 25 (3), 349–379. https://doi.org/10.1002/hrdq
Munn, Z., Peters, M. D. J., Stern, C., Tufanaru, C., McArthur, A., & Aromataris, E. (2018). Systematic review or scoping review? Guidance for authors when choosing between a systematic or scoping review approach. BMC Medical Research Methodology, 18 (143), 1–7. https://doi.org/10.4324/9781315159416
Murphy, S. E., & Johnson, S. K. (2011). The benefits of a long-lens approach to leader development: Understanding the seeds of leadership. Leadership Quarterly, 22 (3), 459–470. https://doi.org/10.1016/j.leaqua.2011.04.004
Oliver, P. H., Gottfried, A. W., Guerin, D. W., Gottfried, A. E., Reichard, R. J., & Riggio, R. E. (2011). Adolescent family environmental antecedents to transformational leadership potential: A longitudinal mediational analysis. Leadership Quarterly, 22 (3), 535–544. https://doi.org/10.1016/j.leaqua.2011.04.010
Peters, M. D. J., Marnie, C., Tricco, A. C., Pollock, D., Munn, Z., Alexander, L., McInerney, P., Godfrey, C. M., & Khalil, H. (2020). Updated methodological guidance for the conduct of scoping reviews. JBI Evidence Synthesis, 18 (10), 2119–2126. https://doi.org/10.11124/JBIES-20-00167
Petticrew, M., & Roberts, H. (2008). Systematic reviews in the social sciences: A practical guide . https://doi.org/10.1002/9780470754887
Plano Clark, V. L., Anderson, N., Wertz, J. A., Zhou, Y., Schumacher, K., & Miaskowski, C. (2015). Conceptualizing longitudinal mixed methods designs: A methodological review of health sciences research. Journal of Mixed Methods Research, 9 (4), 297–319. https://doi.org/10.1177/1558689814543563
Ployhart, R. E., & Vandenberg, R. J. (2010). Longitudinal research: The theory, design, and analysis of change. Journal of Management, 36 (1), 94–120. https://doi.org/10.1177/0149206309352110
Quigley, N. R. (2013). A longitudinal, multilevel study of leadership efficacy development in MBA teams. Academy of Management Learning & Education, 12 (4), 579–602. https://doi.org/10.5465/amle.2011.0524
Rajulton, F. (2001). The fundamentals of longitudinal research: An overview. Canadian Studies in Population, 28 (2), 169. https://doi.org/10.25336/p6w897
Rogosa, D. R., & Willett, J. B. (1983). Demonstrating the reliability of the difference score in the measurement of change. Journal of Educational Measurement, 20 (4), 335–343.
Rogosa, D., Brandt, D., & Zimowski, M. (1982). A growth curve approach to the measurement of change. Psychological Bulletin, 92 (3), 726–748. https://doi.org/10.1037/0033-2909.92.3.726
Seifert, C. F., & Yukl, G. (2010). Effects of repeated multi-source feedback on the influence behavior and effectiveness of managers: A field experiment. Leadership Quarterly, 21 (5), 856–866. https://doi.org/10.1016/j.leaqua.2010.07.012
Singer, J. D., & Willett, J. B. (2003). Applied longitudinal data analysis: Modeling change and event occurrence. In Etica e Politica . Oxford University Press. https://doi.org/10.1093/acprof
Sørensen, P., Hansen, M. B., & Villadsen, A. R. (2023). Perceived changes in leadership behavior during formal leadership education. Public Personnel Management, 52 (2), 170–190. https://doi.org/10.1177/00910260221136085
Springborg, C. (2010). Leadership as art - leaders coming to their senses. Leadership, 6 (3), 243–258. https://doi.org/10.1177/1742715010368766
Steele, A. R., & Day, D. V. (2018). The role of self-attention in leader development. Journal of Leadership Studies, 12 (2), 17–32. https://doi.org/10.1002/jls.21570
Taris, T. W. (2000). A primer in longitudinal data analysis . SAGE Publications Ltd.
Thomson, R., & Holland, J. (2003). Hindsight, foresight and insight: The challenges of longitudinal qualitative research. International Journal of Social Research Methodology: Theory and Practice, 6 (3), 233–244. https://doi.org/10.1080/1364557032000091833
Tricco, A. C., Lillie, E., Zarin, W., O’Brien, K. K., Colquhoun, H., Levac, D., Moher, D., Peters, M. D. J., Horsley, T., Weeks, L., Hempel, S., Akl, E. A., Chang, C., McGowan, J., Stewart, L., Hartling, L., Aldcroft, A., Wilson, M. G., Garritty, C., & Straus, S. E. (2018). PRISMA extension for scoping reviews (PRISMA-ScR): Checklist and explanation. Annals of Internal Medicine, 169 (7), 467–473. https://doi.org/10.7326/M18-0850
Van Velsor, E., McCauley, C. D., & Ruderman, M. N. (2010). The center for creative leadership handbook of leadership development (3rd ed.). Jossey-Bass Inc.
Vogel, B., Reichard, R. J., Batistič, S., & Černe, M. (2020). A bibliometric review of the leadership development field: How we got here, where we are, and where we are headed. The Leadership Quarterly , 101381. https://doi.org/10.1016/j.leaqua.2020.101381
Vogl, S. (2023). Mixed methods longitudinal research. Forum: Qualitative Social Research, 24 (1). https://doi.org/10.17169/fqs-24.1.4012
Vogl, S., Zartler, U., Schmidt, E. M., & Rieder, I. (2018). Developing an analytical framework for multiple perspective, qualitative longitudinal interviews (MPQLI). International Journal of Social Research Methodology, 21 (2), 177–190. https://doi.org/10.1080/13645579.2017.1345149
Wakabayashi, M., & Graen, G. B. (1984). The Japanese career progress study: A 7-year follow-up. Journal of Applied Psychology, 69 (4), 603–614. https://doi.org/10.1037/0021-9010.69.4.603
Wakabayashi, M., Graen, G., Graen, M., & Graen, M. (1988). Japanese management progress: Mobility into middle management. Journal of Applied Psychology, 73 (2), 217–227. https://doi.org/10.1037/0021-9010.73.2.217
Wang, M., Beal, D. J., Chan, D., Newman, D. A., Vancouver, J. B., & Vandenberg, R. J. (2017). Longitudinal research: A panel discussion on conceptual issues, research design, and statistical techniques. Work Aging and Retirement, 3 (1), 1–24. https://doi.org/10.1093/workar/waw033
Willett, J. B. (1989). Some results on reliability for the longitudinal measurement of change: Implications for the design of studies of individual growth. Educational and Psychological Measurement, 49 , 587–602.
Yeow, J. B., & Martin, R. (2013). The role of self-regulation in developing leaders: A longitudinal field experiment. Leadership Quarterly, 24 (5), 625–637. https://doi.org/10.1016/j.leaqua.2013.04.004
Download references
Acknowledgements
Not applicable.
Open access funding provided by FCT|FCCN (b-on). The authors gratefully acknowledge financial support from FCT - Fundaçao para a Ciencia e Tecnologia (Portugal), national funding through research grant UIDB/04521/2020.
Author information
Authors and affiliations.
Lisbon School of Economics and Management (ISEG), University of Lisbon, Rua do Quelhas, 6, Lisboa, 1200-781, Portugal
Felipe Senna Cotrim & Jorge Filipe Da Silva Gomes
Advance/CSG, ISEG, Universidade de Lisboa, Lisbon, Portugal
Jorge Filipe Da Silva Gomes
You can also search for this author in PubMed Google Scholar
Corresponding author
Correspondence to Felipe Senna Cotrim .
Ethics declarations
Informed consent, conflict of interest.
The authors declare that they have no conflict of interest.
Additional information
Publisher’s note.
Springer Nature remains neutral with regard to jurisdictional claims in published maps and institutional affiliations.
Rights and permissions
Open Access This article is licensed under a Creative Commons Attribution 4.0 International License, which permits use, sharing, adaptation, distribution and reproduction in any medium or format, as long as you give appropriate credit to the original author(s) and the source, provide a link to the Creative Commons licence, and indicate if changes were made. The images or other third party material in this article are included in the article’s Creative Commons licence, unless indicated otherwise in a credit line to the material. If material is not included in the article’s Creative Commons licence and your intended use is not permitted by statutory regulation or exceeds the permitted use, you will need to obtain permission directly from the copyright holder. To view a copy of this licence, visit http://creativecommons.org/licenses/by/4.0/ .
Reprints and permissions
About this article
Cotrim, F.S., Da Silva Gomes, J.F. Longitudinal studies of leadership development: a scoping review. Curr Psychol (2024). https://doi.org/10.1007/s12144-024-06567-4
Download citation
Accepted : 12 August 2024
Published : 30 August 2024
DOI : https://doi.org/10.1007/s12144-024-06567-4
Share this article
Anyone you share the following link with will be able to read this content:
Sorry, a shareable link is not currently available for this article.
Provided by the Springer Nature SharedIt content-sharing initiative
- Leadership development
- Scoping review
- Longitudinal studies
- Business context
- Find a journal
- Publish with us
- Track your research
Contacta con nuestros responsables de cada área y resuelve de forma rápida y eficaz tus dudas
Para Títulos Universitarios haz click aquí
Para Másteres y Postgrados haz click aquí
Para Ciclos Formativos haz click aquí
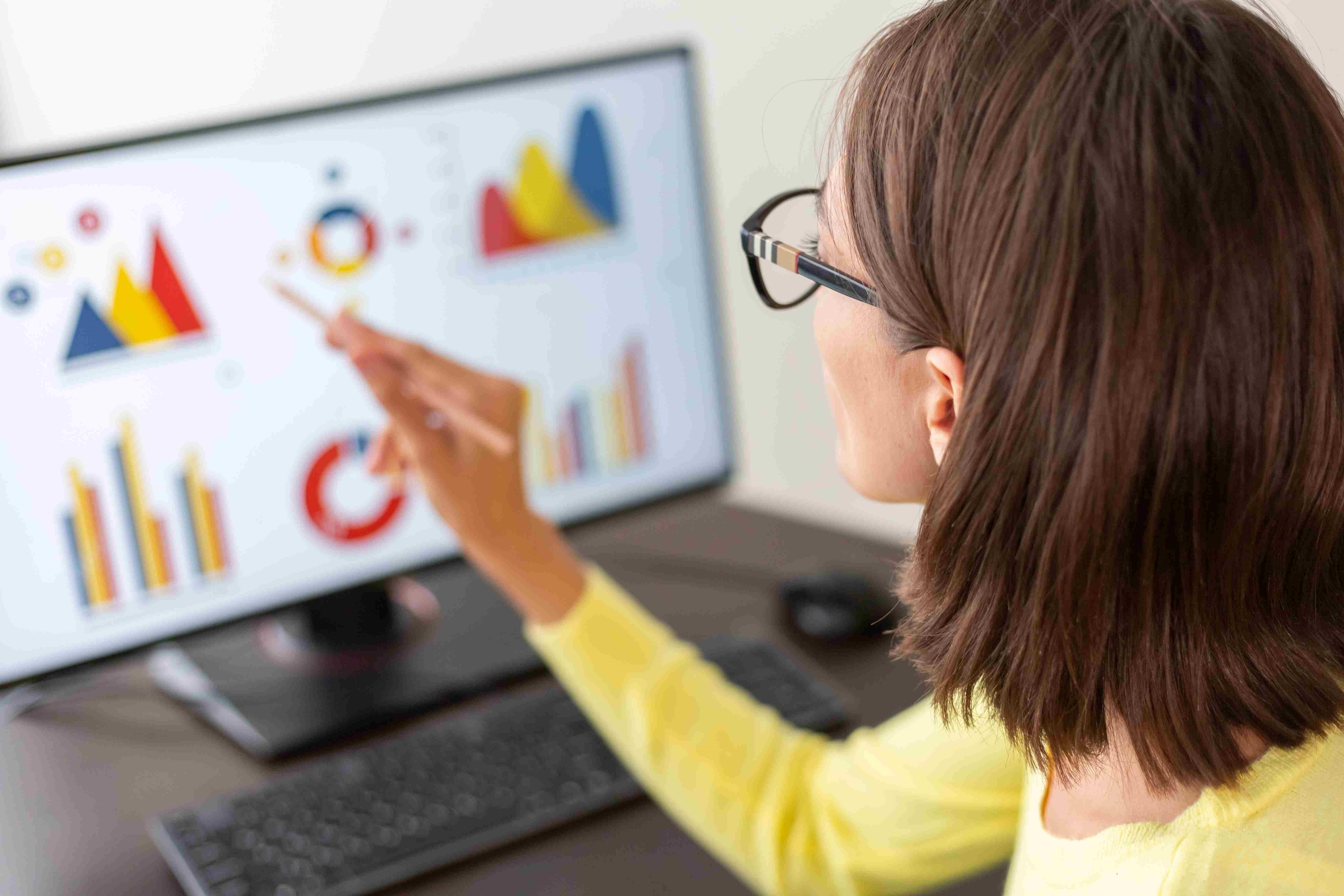
Analytical skills: What they are and examples
- Agosto 2024
- Fecha de publicación
- Marketing y Comunicación
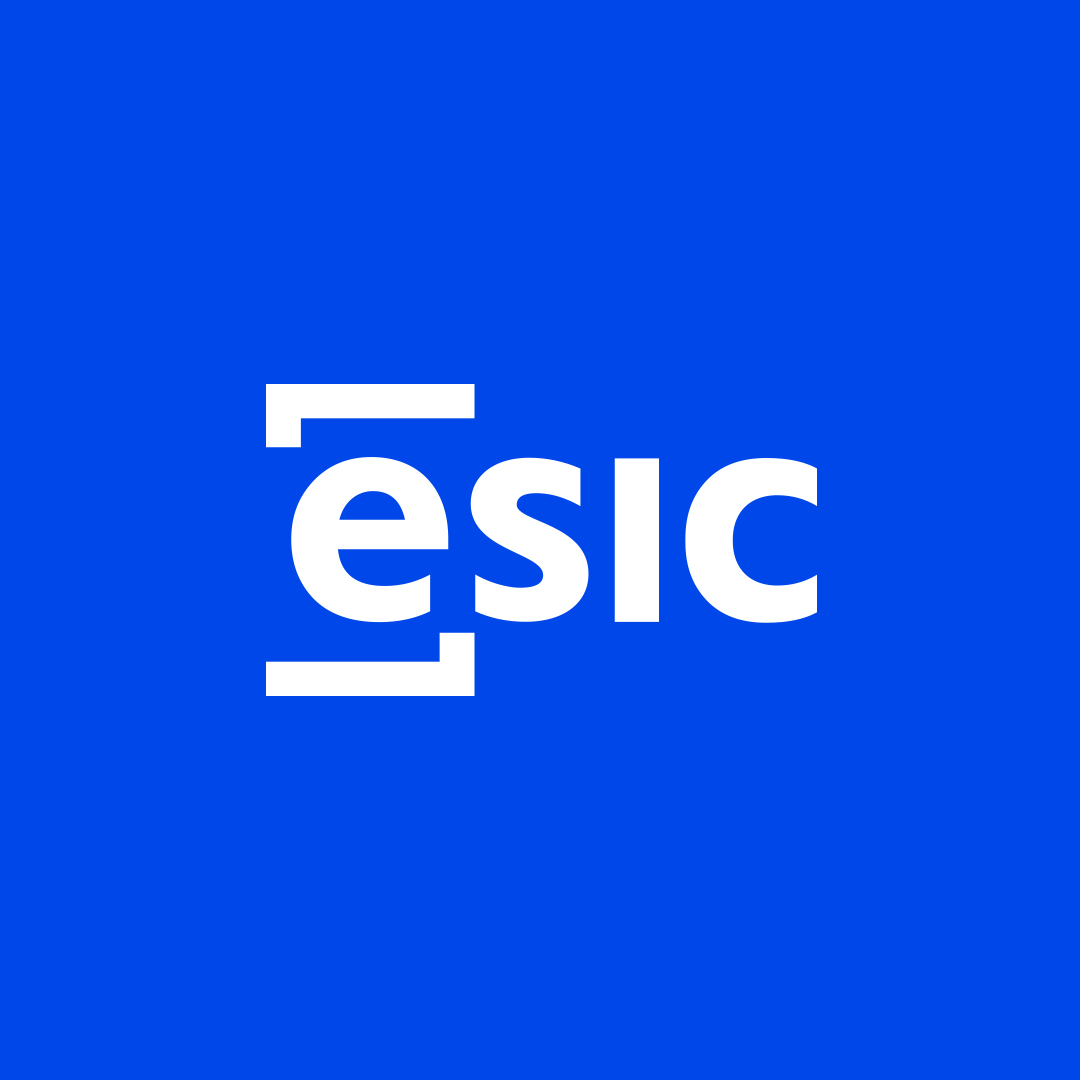
_ESIC Business & Marketing School
ESIC Business & Marketing School.
In the current era of data, analytical skills are indispensable . These abilities enable people to make sense of information, solve problems and choose wisely. This text will discuss what having analytical skills means and why they are important as well as providing examples from various fields.
Additionally, we will show that the ESIC University Master’s Degree in Marketing & Sales Management (GESCO) is the best fit for students who wish to foster their strategic thinking and analytical abilities .
Would you like to study our Master’s Degree in Marketing and Sales Management ? Access for more information.
What do we mean by the term “analytical skills”?
Analytical skills means the potential of a person to amass knowledge , which they then break down into simpler forms or words that can be understood more easily; employing this outcome to solve problems so as to come up with rational decisions supported by evidence rather than baseless opinions; evaluating information critically and drawing logical deductions from it, while using organized research methods such as surveys, among others.
Definition of analytical skills
Analytical skills are defined as different cognitive abilities that allow individuals to process information and draw inferences from it. In simple words, this implies scrutinizing facts, finding patterns and detecting relations between variables, among other things.
They are crucial in areas such as business, science, engineering and technology, where decisions need to be based on evidence.
Examples of analytical skills
Analytical skills are essential for problem-solving, decision-making, and critical thinking in both personal and professional contexts. Bellow we look various examples of analytical skills:
Data analysis
Data analysis refers to the examination of data sets in order to identify patterns, trends and correlations . For example, a marketing analyst might use data analysis methods to determine how successful an advertising campaign recently conducted by the company was. These numbers can also be interpreted so that they provide recommendations for future marketing strategies.
Research
Strong research abilities are an essential part of analytical skills . This means gathering information from different sources, assessing its reliability and then applying it to respond to specific questions or to solve problems.
For instance, a scientist employed in a pharmaceutical company might carry out research to establish how well new drugs work.
Critical thinking
The capacity to think critically involves being able to evaluate information and arguments in a logical manner. Among such skillsets is questioning assumptions, recognizing bias and assessing the credibility of presented evidence.
A project manager exercises critical thinking by evaluating various project proposals based on their feasibility and choosing the most viable one.
Ability to solve problems
Problem-solving is a critical component of analytical skills. This involves identifying problems, generating potential solutions and executing the most appropriate one . By way of illustration, an engineer can use their problem-solving capabilities to diagnose and fix faulty equipment.
Logical thinking
Logical thinking means reaching conclusions based on logical principles and thinking in a structured manner . Such competence becomes important in areas such as computer programming, where it is necessary to have logical steps for creating functional software. For example, during code debugging for program improvement, a software developer may employ logical reasoning.
The importance of analytical skills
Analytical skills are important for a lot of reasons:
Decision-making based on knowledge: these skills enable people to make decisions with data and facts instead of intuition or random guesses.
Problem-solving: this helps identify the real problems and find solutions that can work.
Productivity: analysis can increase productivity by finding areas for improvement and simplifying processes.
Creativity: analytical thinking may also generate creative solutions to problems or different ways of looking at them.
Competitiveness: in business, strong analytical skills foster better strategic planning, as well as market research, which gives an edge over rivals.
If you are interested in delving deeper into analytical skills how to improve your negotiation skills, we encourage you to learn more about our Master’s Degree in Marketing and Sales Management.
If you want to develop your reasoning and evaluation abilities , a Master’s Degree in Marketing and Sales Management is the best decision for you. ESIC University offers such a course, also known as Global Executive Program in Marketing.
The reason behind universal development of the curriculum lies in its multidisciplinary nature, where different areas of study are covered that include strategy formulation and data analysis, among others, which are necessary for dynamic industries like these.
In ESIC’s opinion , what sets apart the GESCO program from others worldwide is its applicability to real life situations, as the lecturers have previously worked in various companies and there are strong relationships with employers. All of this enables students to do more practical, case studies and update their knowledge, thus increasing their understanding of contemporary marketing environment complexities.
Studying the GESCO program provides students with the necessary tools to fill managerial positions in the advertising-sales industry, since this type of environment enhances their training.
Find out more about our Master’s Degree in Marketing and Sales Management
También te puede interesar
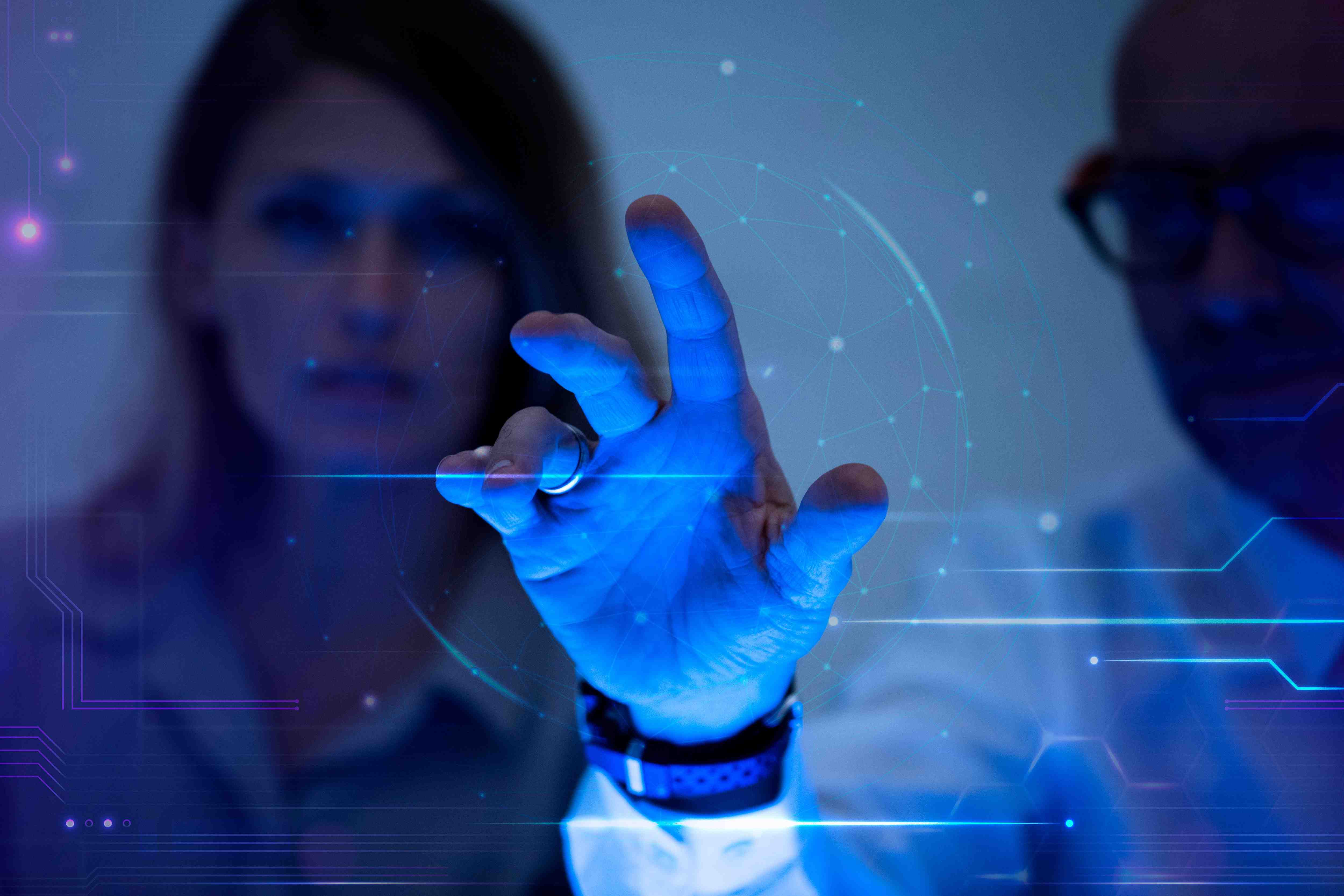
Beneficios de la transformación digital de la Administración pública
Con el avance de la tecnología, las empresas han cambiado y han tenido que adaptarse a las tendencias digitales que han llegado para quedarse. La transformación digital ha irrumpido de lleno en toda...
- Publicado por _ESIC Business & Marketing School
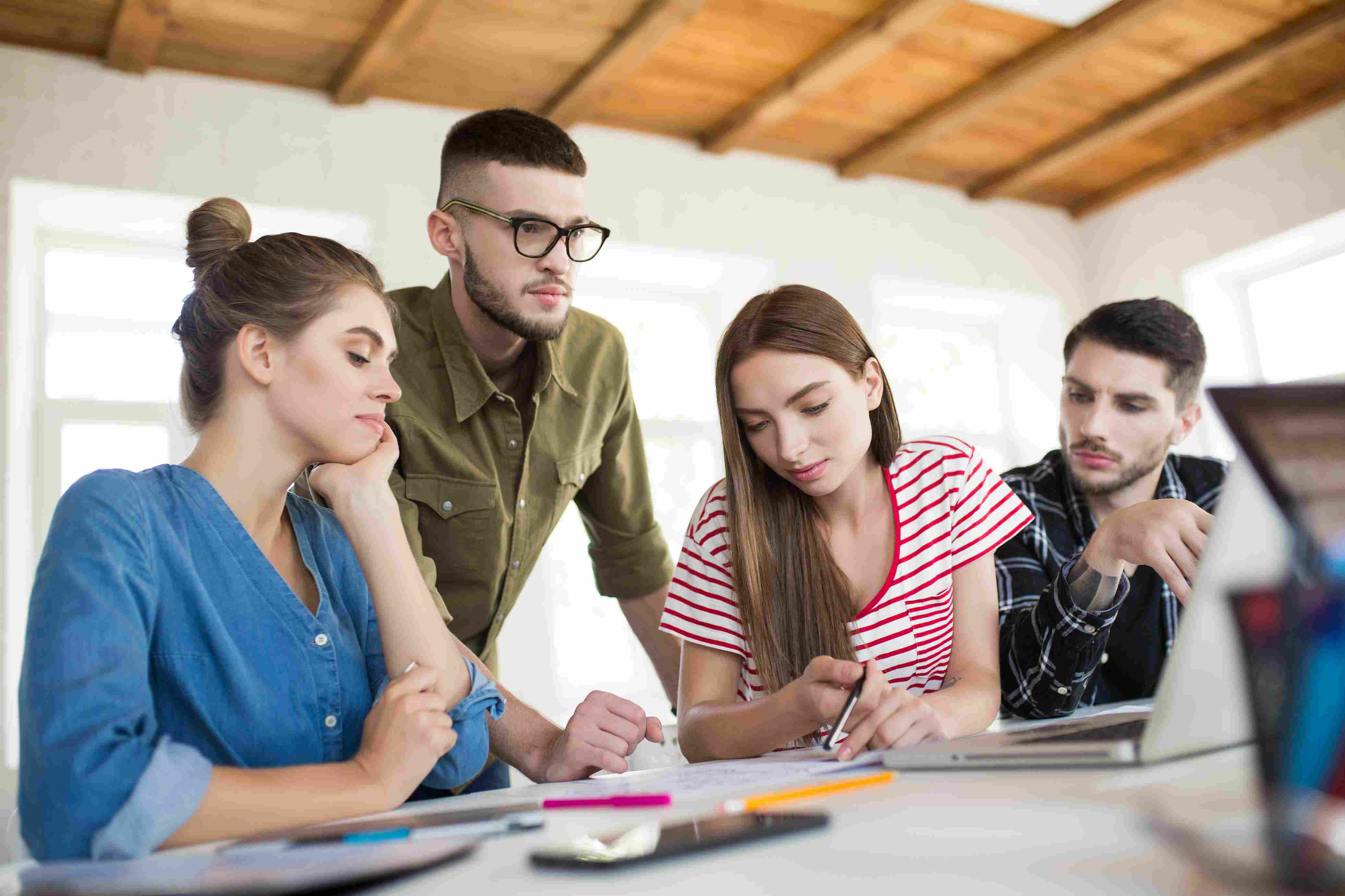
¿Qué es la FCT (Formación en Centros de Trabajo)?
Mundo educativo
No existe mejor formación que la práctica; esto es un hecho, pues pese a todas las ventajas y conocimientos que proporciona la teoría, las prácticas te permiten aprender del día a día de la que ...
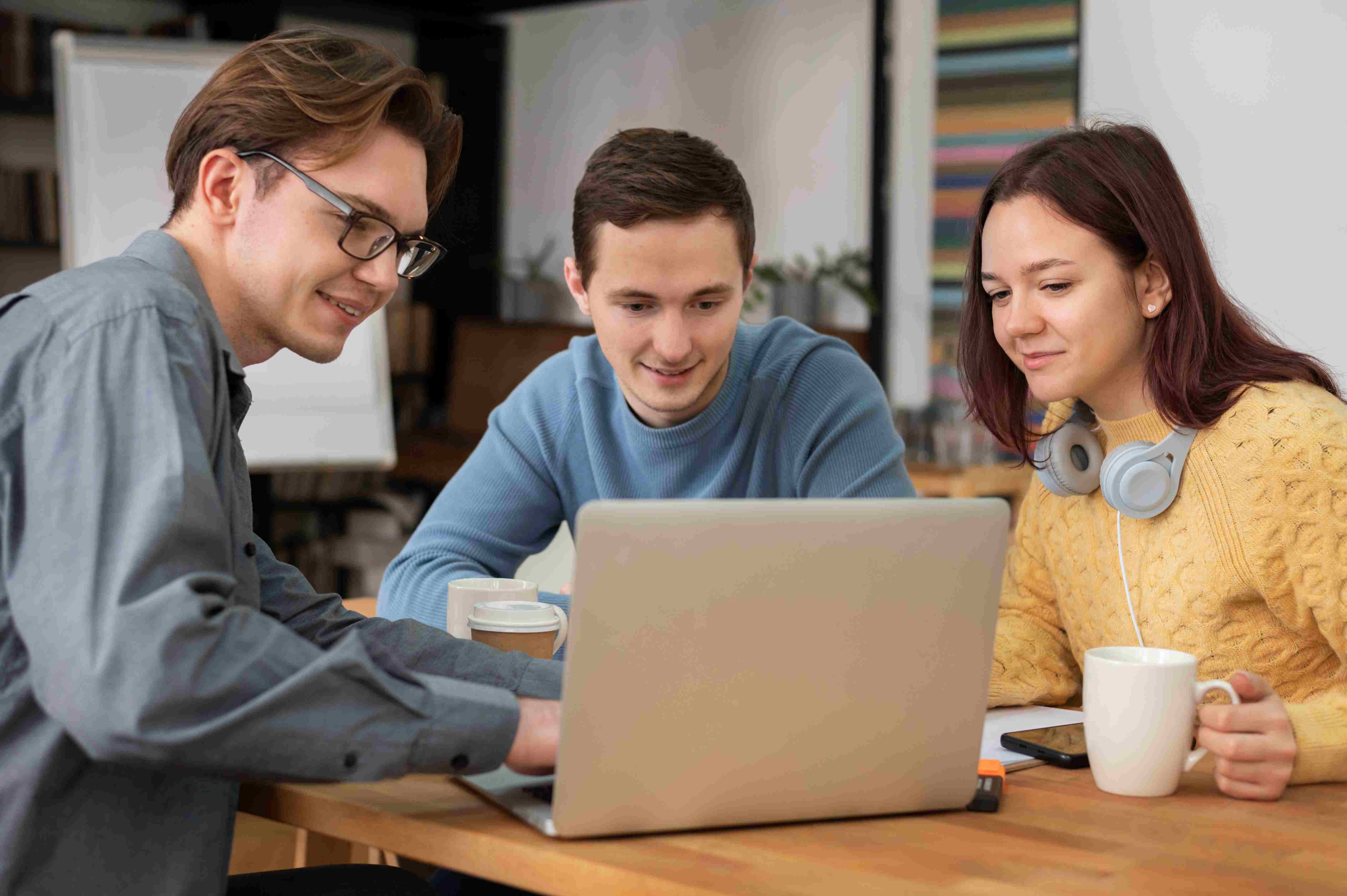
Cómo acceder a la universidad desde un Grado Superior de Formación Profesional
El acceso a la educación superior es una meta compartida por muchos estudiantes que buscan ampliar sus horizontes académicos y profesionales. Aunque la vía tradicional a la universidad es la educac...
- Publicado por ESIC Formación Profesional Superior
¿Quieres que un asesor se ponga en contacto telefónico contigo?
Déjanos tus datos y te llamamos
ESIC Business & Marketing School atenderán tu solicitud de información sobre nuestros servicios formativos. Para esta finalidad y las siguientes, puedes oponerte y acceder, rectificar o suprimir tus datos y ejercitar otros derechos como se indica en nuestra política de privacidad.
SOLICITAR INFORMACIÓN
- Open access
- Published: 02 September 2024
Causal associations of hypothyroidism with frozen shoulder: a two-sample bidirectional Mendelian randomization study
- Bin Chen 1 ,
- Zheng-hua Zhu 1 ,
- Qing Li 2 ,
- Zhi-cheng Zuo 1 &
- Kai-long Zhou 1
BMC Musculoskeletal Disorders volume 25 , Article number: 693 ( 2024 ) Cite this article
Metrics details
Many studies have investigated the association between hypothyroidism and frozen shoulder, but their findings have been inconsistent. Furthermore, earlier research has been primarily observational, which may introduce bias and does not establish a cause-and-effect relationship. To ascertain the causal association, we performed a two-sample bidirectional Mendelian randomization (MR) analysis.
We obtained data on “Hypothyroidism” and “Frozen Shoulder” from Summary-level Genome-Wide Association Studies (GWAS) datasets that have been published. The information came from European population samples. The primary analysis utilized the inverse-variance weighted (IVW) method. Additionally, a sensitivity analysis was conducted to assess the robustness of the results.
We ultimately chose 39 SNPs as IVs for the final analysis. The results of the two MR methods we utilized in the investigation indicated that a possible causal relationship between hypothyroidism and frozen shoulder. The most significant analytical outcome demonstrated an odds ratio (OR) of 1.0577 (95% Confidence Interval (CI):1.0057–1.1123), P = 0.029, using the IVW approach. Furthermore, using the MR Egger method as a supplementary analytical outcome showed an OR of 1.1608 (95% CI:1.0318–1.3060), P = 0.017. Furthermore, the results of our sensitivity analysis indicate that there is no heterogeneity or pleiotropy in our MR analysis. In the reverse Mendelian analysis, no causal relationship was found between frozen shoulders and hypothyroidism.
Our MR analysis suggests that there may be a causal relationship between hypothyroidism and frozen shoulder.
Peer Review reports
Frozen shoulder, also known as adhesive capsulitis, is a common shoulder condition. Patients with frozen shoulder usually experience severe shoulder pain and diffuse shoulder stiffness, which is usually progressive and can lead to severe limitations in daily activities, especially with external rotation of the shoulder joint [ 1 ]. The incidence of the disease is difficult to ascertain because of its insidious onset and the fact that many patients do not choose to seek medical attention. It is estimated to affect about 2% to 5% of the population, with women affected more commonly than men (1.6:1.0) [ 2 , 3 ]. The peak occurrence of frozen shoulder is typically between the ages of 40 and 60, with a positive family history present in around 9.5% of cases [ 4 ]. However, the underlying etiology and pathophysiology of frozen shoulder remains unclear.
The prevalence of frozen shoulder has been reported to be higher in certain diseases such as dyslipidemia [ 5 ], diabetes [ 6 , 7 ], and thyroid disorders [ 4 , 8 ]. The relationship between diabetes and frozen shoulder has been established through epidemiological studies [ 9 , 10 , 11 ]. However, the relationship between thyroid disease and frozen shoulder remains unclear. Thyroid disorders include hyperthyroidism, hypothyroidism, thyroiditis, subclinical hypothyroidism, and others. Previously, some studies reported the connection between frozen shoulders and thyroid dysfunction. However, the conclusions of these studies are not consistent [ 4 , 12 , 13 , 14 , 15 , 16 ]. In addition, these studies are primarily observational and susceptible to confounding variables. Traditional observational studies can only obtain correlations, not exact causal relationships [ 17 ].
MR is a technique that utilizes genetic variants as instrumental variables (IVs) of exposure factors to determine the causal relationship between exposure factors and outcomes [ 17 , 18 ]. MR operates similarly to a randomized controlled trial as genetic variants adhere to Mendelian inheritance patterns and are randomly distributed in the population [ 19 ]. Moreover, alleles remain fixed between individuals and are not influenced by the onset or progression of disease. Consequently, causal inferences derived from MR analyses are less susceptible to confounding and reverse causality biases [ 20 , 21 ]. And with the growing number of GWAS data published by large consortia, MR studies can provide reliable results with a sufficient sample size [ 22 ]. In this study, we performed a two-sample bidirectional MR analysis to evaluate the causal relationship between hypothyroidism and frozen shoulder.
Study design description
The bidirectional MR design, which examines the relationship between hypothyroidism and frozen shoulder, is succinctly outlined in Fig. 1 . Using summary data from Genome-Wide Association Studies (GWAS) datasets, we conducted two MR analyses to explore the potential reciprocal association between hypothyroidism and frozen shoulder. In the reverse MR analyses, Frozen Shoulder was considered as the exposure and Hypothyroidism as the outcome, while the forward MR analyses focused on Hypothyroidism as the exposure. Figure 1 illustrates the key assumptions of the MR analysis.
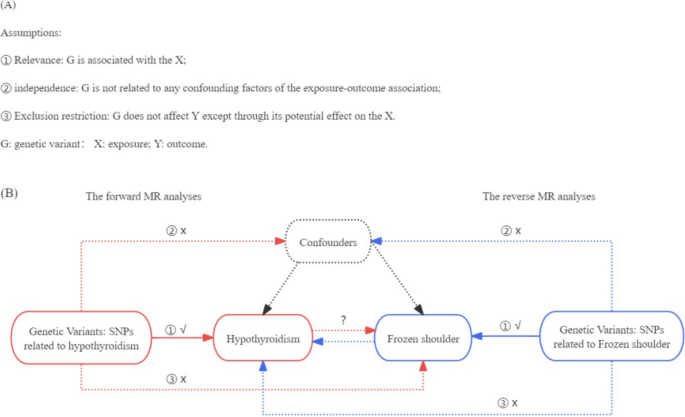
Description of the study design in this bidirectional MR study. A MR analyses depend on three core assumptions. B Research design sketches
Data source
Genetic variants associated with Hypothyroidism were extracted from published Summary-level GWAS datasets provided by the FinnGen Consortium, using the “Hypothyroidism” phenotype in this study. The GWAS included 16380353 subjects, including 22997 cases and 175475 controls. Data for Frozen Shoulder were obtained from the GWAS, which was derived from a European sample [ 23 ]. The frozen shoulder was defined based on the occurrence of one or more International Classification of Disease, 10th Revision (ICD10) codes (as shown in the supplementary material). Our MR study was conducted using publicly available studies or shared datasets and therefore did not require additional ethical statements or consent.
Selection of IV
For MR studies to yield reliable results, they must adhere to three fundamental assumptions [ 24 ], Regarding the IV selection, the following statements hold true: (1) IVs exhibit substantial correlation with exposure factors; (2) IVs do not directly impact outcomes but influence outcomes through exposure; (3) IVs are not correlated with any confounding factors that could influence exposure and outcome. Firstly, we selected single‐nucleotide polymorphisms (SNPs) from the European GWAS that met the genome-wide significance criterion ( p < 5 × 10 –8 ) and were associated with the exposure of interest as potential SNPs. Subsequently, we excluded any selected SNPs that linkage disequilibrium (LD) using the clump function (r 2 = 0.001, kb = 10000). Furthermore, palindromic SNPs and ambiguous SNPs were excluded. These excluded SNPs were not included in subsequent analyses. To evaluate weak instrumental variable effects, we utilized the F-statistic, considering genetic variants with an F-statistic < 10 as weak IVs and excluding them. Then for the second assumption, we needed to manually remove SNPs associated with outcome ( p < 5 × 10 –8 ). For the third assumption, “ IVs are not correlated with any confounding factors that could influence exposure and outcome,” implying that the IVs chosen should not have horizontal pleiotropy. The final set of SNPs meeting these criteria were utilized as IVs in the subsequent MR analysis.
MR analysis
In this study, we evaluated the relationship between hypothyroidism and frozen shoulder using two different MR methods: IVW [ 25 ] and MR-Egger regression [ 26 ]. The Wald ratio for each IV will be meta-analyzed using the IVW approach to investigate the causal relationship. In contrast to the MR-Egger technique, which remains functional even in the presence of invalid IVs, the IVW method assumes that all included genetic variants are valid instrumental variables. Furthermore, MR-Egger incorporates an intercept term to examine potential pleiotropy. If this intercept term equals 0 ( P > 0.05), the results of the MR-Egger regression model closely align with those obtained from IVW; However, if the intercept term deviates significantly from 0 ( P < 0.05), it suggests possible horizontal pleiotropy associated with these IVs. MR-Egger employed as estimation method alongside IVW. Although less efficient, these approaches can provide reliable predictions across a broader range of scenarios.
Sensitivity analysis
We performed a sensitivity analysis to investigate potential horizontal pleiotropy and heterogeneity in our study, aiming to demonstrate the robustness of our findings. Cochran’s Q test was employed to identify possible heterogeneity. Cochran’s Q statistic assessed genetic variant heterogeneity while considering significance at p < 0.05 level and I 2 > 25% as an indication of heterogeneity. on the results, we generated funnel plots. MR-Egger intercept tests were then utilized to estimate horizontal pleiotropy (with presence of an intercept and horizontal pleiotropy considered when p < 0.05). Additionally, a leave-one-out to determine if causality depended on or was influenced by any specific SNP. All statistical analyses were performed using the “TwoSampleMR” packages in R (version 3.6.3, www.r-project.org/ ) [ 27 ].
Instrumental variables
We ultimately chose 39 SNPs as IVs for the final analysis after going through the aforementioned screening process. All IVs had an F-statistic > 10, indicating a low probability of weak IV bias. Comprehensive information on each IV can be found in Appendix 1 .
Mendelian randomization results
According to the outcomes of the two MR techniques we employed for our analysis, hypothyroidism increases the risk factors for developing frozen shoulder. Specifically, as shown in the results of Table 1 , the primary analytical outcome using the IVW method revealed an OR of 1.0577 (95% CI:1.0057–1.1123), P = 0.029. Additionally, employing the MR Egger method secondary analytical outcome resulted in an OR of 1.1608 (95% CI:1.0318–1.3060), P = 0.017. Furthermore, scatter plots (Fig. 2 ) and forest plots (Fig. 3 ) were generated based on the findings of this MR study.
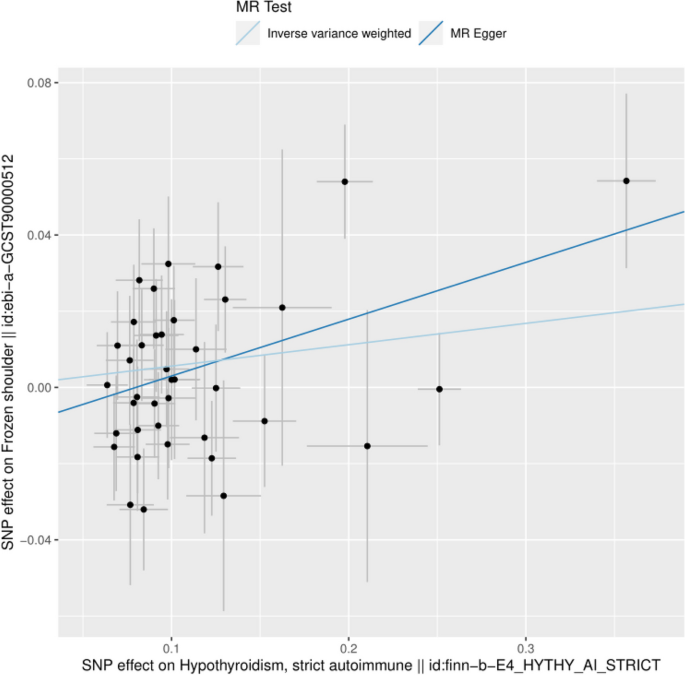
Scatterplot of MR analysis
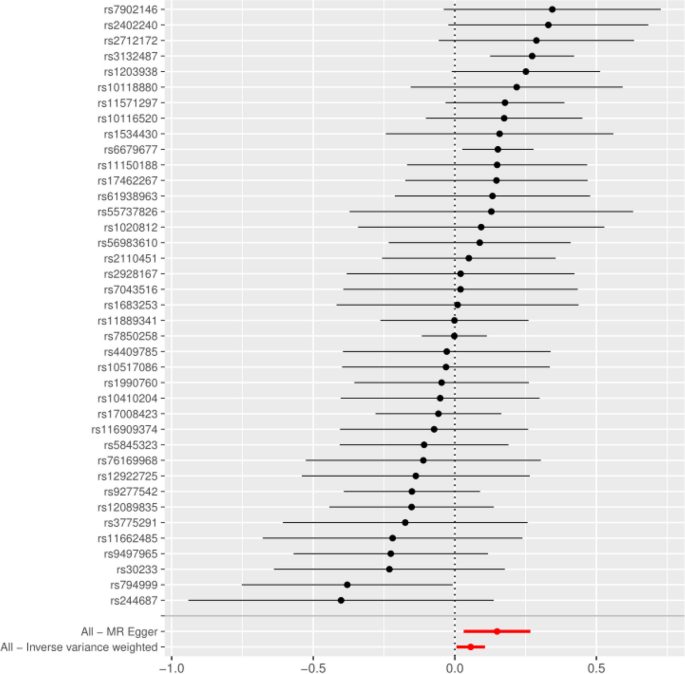
Forest plot of MR analysis
Heterogeneity and sensitivity test
The heterogeneity of causal estimates obtained for each SNP reflects their variability. A lower level of heterogeneity indicates higher reliability of MR estimates. To further validate the dependability of the results, we conducted a sensitivity analysis to examine the heterogeneity in MR. The funnel plots we created are displayed in Fig. 4 together with the results of Cochran’s Q test (Table 2 ), which revealed no heterogeneity in IVs. Additionally, the MR-Egger intercept test results (p = 0.0968) indicated no presence of pleiotropy in our data. Furthermore, the outcomes leave-one-out test demonstrated that causation remained independent and unaffected by any specific SNP (Fig. 5 ).
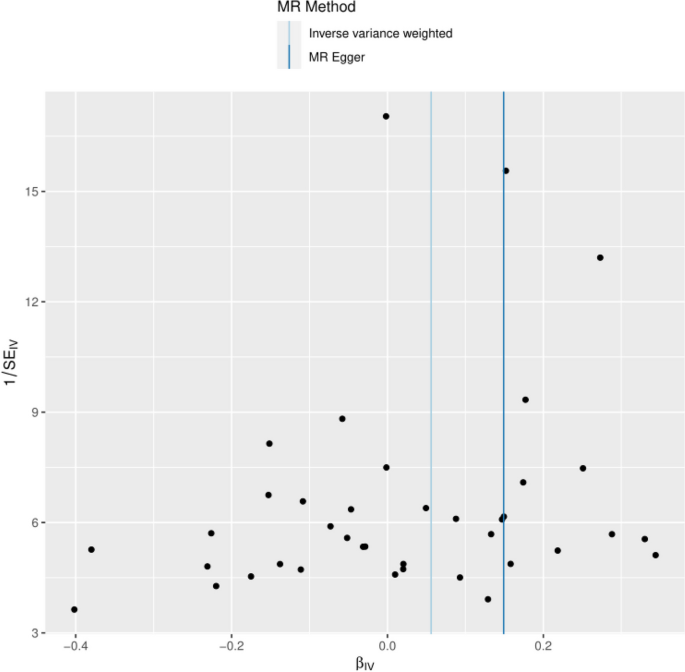
Funnel plot to assess heterogeneity
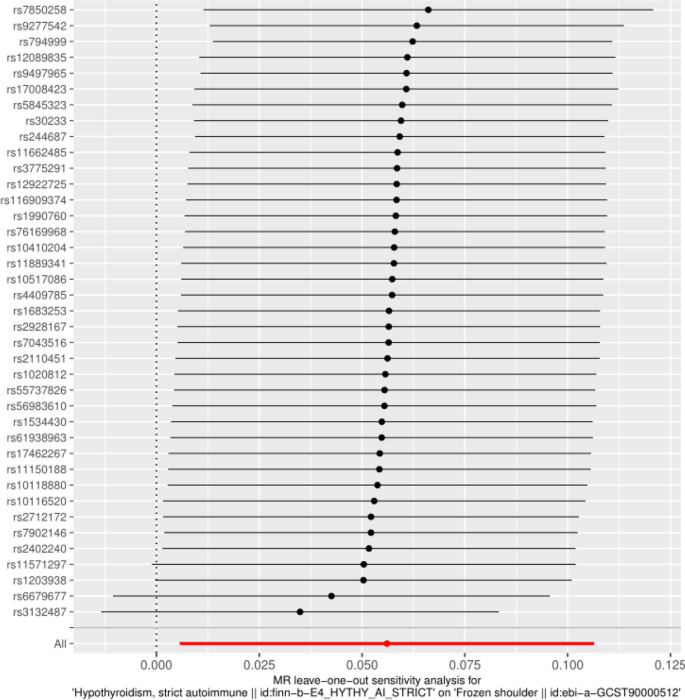
Sensitivity analysis by the leave-one-out method
Reverse Mendelian randomization analysis
In the reverse two-sample MR analysis, frozen shoulder was chosen as the exposure factor, and hypothyroidism as the outcome factor. The same threshold was set, and chain imbalance was eliminated. Finally, four SNPs were included as IVs in the reverse MR analysis. None of the four results from the MR analysis support a causal relationship between genetic susceptibility to frozen shoulder and the risk of hypothyroidism, as shown in Table 3 .
The frequent shoulder ailment known as frozen shoulder is characterized by joint pain and dysfunction. It has a significant negative impact on patient’s quality of life and increases the financial strain on families and society. Frozen shoulder can be caused by various factors, with thyroid disorders being one of them, although the exact causal relationship between them remains unclear.
There is considerable debate over whether hypothyroidism enhances the prevalence of frozen shoulder in the population. Results from Carina Cohen et al. [ 4 ] indicate that thyroid disorders, particularly hypothyroidism and the presence of benign thyroid nodules, significantly contribute to the risk of developing frozen shoulder. These factors increase the likelihood of acquiring the condition by 2.69 times [ 4 ]. A case–control study conducted in China revealed that thyroid disease is associated with an elevated risk of developing frozen shoulder [ 14 ]. Hyung Bin Park et al. also discovered a notable association between subclinical hypothyroidism and frozen shoulder [ 16 ]. Consistent with previous studies, a case–control study from Brazil reported that patients with hypothyroidism were more likely to be diagnosed with frozen shoulder than comparable patients [ 28 ]. However, there are some inconsistencies. Kiera Kingston et al. [ 13 ] discovered hypothyroidism in 8.1% of individuals with adhesive capsulitis; however, this rate was lower than the 10.3% identified in the control population [ 13 ]. Hyung et al. concluded that there was no association between them [ 15 ]. Studies by Chris et al. also questioned the relationship between heart disease, high cholesterol and thyroid disease and frozen shoulder [ 29 ]. All of these studies, we discovered, had poor scores on the evidence-based medicine scale, were vulnerable to a wide range of confounding variables, and carried a number of significant risks of bias. Additionally, conventional observational studies only provide correlations rather than precise causal links.
To overcome this shortcoming, we performed the MR analysis. The results of the two MR methods examined in this study suggest a possible causal relationship between hypothyroidism and frozen shoulder. Importantly, no substantial heterogeneity or pleiotropy was observed in these findings. Our conclusions are similar to those of Deng et al. [ 30 ]. However, our study conducted a reverse Mendelian randomization analysis and had a larger sample size. Several mechanisms may underlie this association. First, fibrosis plays a crucial role in the movement disorders associated with frozen shoulder. Hypothyroidism impairs the synthesis and breakdown of collagen, elastic fibers, and polysaccharides within soft tissues, resulting in tissue edema and fibrosis, contributing to the development of frozen shoulder [ 31 ]. Second, hypothyroidism influences various signaling pathways including growth factors, the extracellular matrix, and calcium signaling, which can impact the differentiation and functionality of osteocytes, leading to bone degeneration and subsequently progressing to frozen shoulder [ 32 ]. Third, hypothyroidism can result in reduced nerve conduction velocity, nerve fiber degeneration, and neuritis, subsequently compromising the sensory and motor functions of nerves and elevating the risk of developing frozen shoulder [ 33 ]. The outcomes of the MR analysis can be used to screen potential risk factors in advance. Accordingly, people with hypothyroidism are more likely to develop frozen shoulder. It is suggested that clinicians should pay attention to the patients with shoulder discomfort when treating hypothyroidism, and provide some ideas for early intervention, which is beneficial to the prognosis of patients.
Our research has some advantages. Firstly, by employing the MR approach, confounding factors and reverse causality were carefully controlled, at least to a large extent. Secondly, our study relied on data derived from previously published GWAS studies, which boasted a substantial sample size and encompassed numerous genetic variants. Moreover, it is worth mentioning that we also used different methods to estimate the impacts, which improves the reliability of our results. However, our MR study still has limitations. First, there may be unobserved pleiotropy beyond vertical pleiotropy. In addition, the samples for this study were all from the European population. Research results based on race may limit their generalizations to other populations. Therefore, large-scale, multi ethnic clinical and basic research may be needed to validate these issues.
With the help of two Mendelian randomization studies, we found that there may be a causal relationship between hypothyroidism and frozen shoulder, and hypothyroidism may be associated with an increased risk of frozen shoulder. However, the exact mechanism remains to be elucidated. More research is required to investigate the underlying mechanisms of this causal relationship.
Availability of data and materials
The datasets used and/or analysed during the current study available from the corresponding author on reasonable request.
Abbreviations
- Mendelian randomization
Genome-Wide Association Studies
Inverse-Variance Weighted
Confidence Interval
Instrumental Variables
Single‐Nucleotide Polymorphisms
Linkage Disequilibrium
Neviaser AS, Neviaser RJ. Adhesive capsulitis of the shoulder. J Am Acad Orthop Surg. 2011;19(9):536–42. https://doi.org/10.5435/00124635-201109000-00004 .
Article PubMed Google Scholar
Hand C, Clipsham K, Rees JL, Carr AJ. Long-term outcome of frozen shoulder. J Shoulder Elbow Surg. 2008;17(2):231–6. https://doi.org/10.1016/j.jse.2007.05.009 .
Hsu JE, Anakwenze OA, Warrender WJ, Abboud JA. Current review of adhesive capsulitis. J Shoulder Elbow Surg. 2011;20(3):502–14. https://doi.org/10.1016/j.jse.2010.08.023 .
Cohen C, Tortato S, Silva OBS, Leal MF, Ejnisman B, Faloppa F. Association between Frozen Shoulder and Thyroid Diseases: Strengthening the Evidences. Rev Bras Ortop (Sao Paulo). 2020;55(4):483–9. https://doi.org/10.1055/s-0039-3402476 .
Sung CM, Jung TS, Park HB. Are serum lipids involved in primary frozen shoulder? A case-control study. J Bone Joint Surg Am. 2014;96(21):1828–33. https://doi.org/10.2106/jbjs.m.00936 .
Huang YP, Fann CY, Chiu YH, Yen MF, Chen LS, Chen HH, et al. Association of diabetes mellitus with the risk of developing adhesive capsulitis of the shoulder: a longitudinal population-based followup study. Arthritis Care Res (Hoboken). 2013;65(7):1197–202. https://doi.org/10.1002/acr.21938 .
Arkkila PE, Kantola IM, Viikari JS, Rönnemaa T. Shoulder capsulitis in type I and II diabetic patients: association with diabetic complications and related diseases. Ann Rheum Dis. 1996;55(12):907–14. https://doi.org/10.1136/ard.55.12.907 .
Article CAS PubMed PubMed Central Google Scholar
Bowman CA, Jeffcoate WJ, Pattrick M, Doherty M. Bilateral adhesive capsulitis, oligoarthritis and proximal myopathy as presentation of hypothyroidism. Br J Rheumatol. 1988;27(1):62–4. https://doi.org/10.1093/rheumatology/27.1.62 .
Article CAS PubMed Google Scholar
Ramirez J. Adhesive capsulitis: diagnosis and management. Am Fam Physician. 2019;99(5):297–300.
PubMed Google Scholar
Wagner S, Nørgaard K, Willaing I, Olesen K, Andersen HU. Upper-extremity impairments in type 1 diabetes: results from a controlled nationwide study. Diabetes Care. 2023;46(6):1204–8. https://doi.org/10.2337/dc23-0063 .
Juel NG, Brox JI, Brunborg C, Holte KB, Berg TJ. Very High prevalence of frozen shoulder in patients with type 1 diabetes of ≥45 years’ duration: the dialong shoulder study. Arch Phys Med Rehabil. 2017;98(8):1551–9. https://doi.org/10.1016/j.apmr.2017.01.020 .
Huang SW, Lin JW, Wang WT, Wu CW, Liou TH, Lin HW. Hyperthyroidism is a risk factor for developing adhesive capsulitis of the shoulder: a nationwide longitudinal population-based study. Sci Rep. 2014;4:4183. https://doi.org/10.1038/srep04183 .
Kingston K, Curry EJ, Galvin JW, Li X. Shoulder adhesive capsulitis: epidemiology and predictors of surgery. J Shoulder Elbow Surg. 2018;27(8):1437–43. https://doi.org/10.1016/j.jse.2018.04.004 .
Li W, Lu N, Xu H, Wang H, Huang J. Case control study of risk factors for frozen shoulder in China. Int J Rheum Dis. 2015;18(5):508–13. https://doi.org/10.1111/1756-185x.12246 .
Park HB, Gwark JY, Jung J, Jeong ST. Association between high-sensitivity C-reactive protein and idiopathic adhesive capsulitis. J Bone Joint Surg Am. 2020;102(9):761–8. https://doi.org/10.2106/jbjs.19.00759 .
Park HB, Gwark JY, Jung J, Jeong ST. Involvement of inflammatory lipoproteinemia with idiopathic adhesive capsulitis accompanying subclinical hypothyroidism. J Shoulder Elbow Surg. 2022;31(10):2121–7. https://doi.org/10.1016/j.jse.2022.03.003 .
Lawlor DA, Harbord RM, Sterne JA, Timpson N, Davey Smith G. Mendelian randomization: using genes as instruments for making causal inferences in epidemiology. Stat Med. 2008;27(8):1133–63. https://doi.org/10.1002/sim.3034 .
Smith GD, Ebrahim S. ‘Mendelian randomization’: can genetic epidemiology contribute to understanding environmental determinants of disease? Int J Epidemiol. 2003;32(1):1–22. https://doi.org/10.1093/ije/dyg070 .
He Y, Zheng C, He MH, Huang JR. The causal relationship between body mass index and the risk of osteoarthritis. Int J Gen Med. 2021;14:2227–37. https://doi.org/10.2147/ijgm.s314180 .
Article PubMed PubMed Central Google Scholar
Evans DM, Davey Smith G. Mendelian randomization: new applications in the coming age of hypothesis-free causality. Annu Rev Genomics Hum Genet. 2015;16:327–50. https://doi.org/10.1146/annurev-genom-090314-050016 .
Burgess S, Butterworth A, Malarstig A, Thompson SG. Use of Mendelian randomisation to assess potential benefit of clinical intervention. BMJ. 2012;345:e7325. https://doi.org/10.1136/bmj.e7325 .
Li MJ, Liu Z, Wang P, Wong MP, Nelson MR, Kocher JP, et al. GWASdb v2: an update database for human genetic variants identified by genome-wide association studies. Nucleic Acids Res. 2016;44(D1):D869–76. https://doi.org/10.1093/nar/gkv1317 .
Green HD, Jones A, Evans JP, Wood AR, Beaumont RN, Tyrrell J, et al. A genome-wide association study identifies 5 loci associated with frozen shoulder and implicates diabetes as a causal risk factor. PLoS Genet. 2021;17(6):e1009577. https://doi.org/10.1371/journal.pgen.1009577 .
Burgess S, Davey Smith G, Davies NM, Dudbridge F, Gill D, Glymour MM, et al. Guidelines for performing Mendelian randomization investigations: update for summer 2023. Wellcome Open Res. 2019;4:186. https://doi.org/10.12688/wellcomeopenres.15555.3 .
Burgess S, Butterworth A, Thompson SG. Mendelian randomization analysis with multiple genetic variants using summarized data. Genet Epidemiol. 2013;37(7):658–65. https://doi.org/10.1002/gepi.21758 .
Bowden J, Del Greco MF, Minelli C, Davey Smith G, Sheehan NA, Thompson JR. Assessing the suitability of summary data for two-sample Mendelian randomization analyses using MR-Egger regression: the role of the I2 statistic. Int J Epidemiol. 2016;45(6):1961–1974. https://doi.org/10.1093/ije/dyw220 .
Hemani G, Zheng J, Elsworth B, Wade KH, Haberland V, Baird D, et al. The MR-Base platform supports systematic causal inference across the human phenome . Elife. 2018;7. https://doi.org/10.7554/eLife.34408 .
Schiefer M, Teixeira PFS, Fontenelle C, Carminatti T, Santos DA, Righi LD, et al. Prevalence of hypothyroidism in patients with frozen shoulder. J Shoulder Elbow Surg. 2017;26(1):49–55. https://doi.org/10.1016/j.jse.2016.04.026 .
Smith CD, White WJ, Bunker TD. The associations of frozen shoulder in patients requiring arthroscopic capsular release. Should Elb. 2012;4(2):87–9. https://doi.org/10.1111/j.1758-5740.2011.00169.x .
Article Google Scholar
Deng G, Wei Y. The causal relationship between hypothyroidism and frozen shoulder: A two-sample Mendelian randomization. Medicine (Baltimore). 2023;102(43):e35650. https://doi.org/10.1097/md.0000000000035650 .
Pandey V, Madi S. Clinical guidelines in the management of frozen shoulder: an update! Indian J Orthop. 2021;55(2):299–309. https://doi.org/10.1007/s43465-021-00351-3 .
Zhu S, Pang Y, Xu J, Chen X, Zhang C, Wu B, et al. Endocrine regulation on bone by thyroid. Front Endocrinol (Lausanne). 2022;13:873820. https://doi.org/10.3389/fendo.2022.873820 .
Baksi S, Pradhan A. Thyroid hormone: sex-dependent role in nervous system regulation and disease. Biol Sex Differ. 2021;12(1):25. https://doi.org/10.1186/s13293-021-00367-2 .
Download references
Acknowledgements
Not applicable.
This study was supported by the Project of State Key Laboratory of Radiation Medicine and Protection, Soochow University (No. GZK12023047).
Author information
Authors and affiliations.
Department of Orthopaedics, The Second Affiliated Hospital of Soochow University, Suzhou, China
Bin Chen, Zheng-hua Zhu, Zhi-cheng Zuo & Kai-long Zhou
State Key Laboratory of Radiation Medicine and Protection, Soochow University, Suzhou, 215123, China
You can also search for this author in PubMed Google Scholar
Contributions
BC: designed research, performed research, collected data, analyzed data, wrote paper. Zh Z, QL and Zc Z: collected data and verification results. Kl Z: designed research and revised article.
Corresponding author
Correspondence to Kai-long Zhou .
Ethics declarations
Ethics approval and consent to participate.
Because the study was based on a public database, did not involve animal or human studies, and was available in the form of open access and anonymous data, Institutional Review Board approval was not required.
Consent for publication
Competing interests.
The authors declare no competing interests.
Additional information
Publisher’s note.
Springer Nature remains neutral with regard to jurisdictional claims in published maps and institutional affiliations.
Supplementary Information
Supplementary material 1., supplementary material 2., rights and permissions.
Open Access This article is licensed under a Creative Commons Attribution-NonCommercial-NoDerivatives 4.0 International License, which permits any non-commercial use, sharing, distribution and reproduction in any medium or format, as long as you give appropriate credit to the original author(s) and the source, provide a link to the Creative Commons licence, and indicate if you modified the licensed material. You do not have permission under this licence to share adapted material derived from this article or parts of it. The images or other third party material in this article are included in the article’s Creative Commons licence, unless indicated otherwise in a credit line to the material. If material is not included in the article’s Creative Commons licence and your intended use is not permitted by statutory regulation or exceeds the permitted use, you will need to obtain permission directly from the copyright holder. To view a copy of this licence, visit http://creativecommons.org/licenses/by-nc-nd/4.0/ .
Reprints and permissions
About this article
Cite this article.
Chen, B., Zhu, Zh., Li, Q. et al. Causal associations of hypothyroidism with frozen shoulder: a two-sample bidirectional Mendelian randomization study. BMC Musculoskelet Disord 25 , 693 (2024). https://doi.org/10.1186/s12891-024-07826-y
Download citation
Received : 03 October 2023
Accepted : 28 August 2024
Published : 02 September 2024
DOI : https://doi.org/10.1186/s12891-024-07826-y
Share this article
Anyone you share the following link with will be able to read this content:
Sorry, a shareable link is not currently available for this article.
Provided by the Springer Nature SharedIt content-sharing initiative
- Frozen shoulder
- Hypothyroidism
BMC Musculoskeletal Disorders
ISSN: 1471-2474
- General enquiries: [email protected]
- Open access
- Published: 28 August 2024
International aid management in Afghanistan’s health sector from the perspective of national and international managers
- Noorullah Rashed 1 , 2 ,
- Hamidreza Shabanikiya 1 , 4 ,
- Leili Alizamani 1 ,
- Jamshid Jamali 3 &
- Fatemeh Kokabisaghi 1 , 4
BMC Health Services Research volume 24 , Article number: 1001 ( 2024 ) Cite this article
Metrics details
The primary purpose of international aid is to promote economic and social development around the world. International aid plays an important role in Afghanistan’s healthcare system. The purpose of this study is to investigate international aid management in Afghanistan’s health sector from the perspectives of national and international managers in 2022 and to provide recommendations for the improvement.
Design/methodology/approach
The study has a cross-sectional design. The study participants were chosen by random sampling. The sample size was determined based on Yaman’s formula at 110. The data collection tool was the questionnaire provided by International Health Partnership and Related Initiatives. The data were analyzed in two descriptive (mean and percentage) and analytical formats. Independent t-test, Mann-Whitney, Kolmogorov-Smirnov tests and Variance analysis were used to examine the relationships between demographic variables and the scores of each dimension.
The average scores given to different dimensions of aid management were as following: 1) the donners’ support of the national health strategy: 48/68 ± 16.14 (49%), 2) the predictable financing: 50/23 ± 16.02 (50%), 3) foreign aid on budget: 55/39 ± 20.15 (55%), 4) strengthening public financial management system: 38/35 ± 19.06 (38%), 5) strengthening the supply and procurement system: 40.97 ± 19.55 (41%), 6) mutual accountability: 46.50 ± 19.26 (46%), 7) technical support and training: 50.24 ± 17.33 (50%), 8) civil society involvement: 35.24 ± 18.61(35%), 9) private sector participation: 36 ± 17.55 (36%), and in total the average score was 44.52 ± 13.27 (44%). The difference between the scores given by two groups of managers was not significant. No meaningful relationship was observed between the total score and any of the demographic variables, but there was a weak relationship between work and management experience and total score. The correlation coefficient showed a statistically significant relationship between the different dimensions of the questionnaire. To sum up, the performance in all dimensions of aid management hardly reached 50%. Donors’ support for the national health strategy was not adequate. There were challenges in evidence-based decision-making, developing national health strategies, control and evaluation, the allocation of resources and use of procurement system. The priorities of donors and government were not always similar and mutual responsibility was lacking. Technical assistance and supporting multilateral cooperation are necessary.
Originality/value
Most studies on foreign aid focused on its effects on economic growth, poverty and investment and not aid management processes. Without proper aid management, parts of resources are wasted and aims of aid programs cannot be achieved. This study investigates aid management in a developing country from the perspectives of two main stakeholders, international and national managers.
Research limitations and implications
Data collection coincided with the change of government in Afghanistan. The situation might be different now. Still, this study provides areas for the improvement of aid management in the studied country. Future studies can build upon the findings of this research and conduct in-depth exploration of areas of aid effectiveness and designing detailed programs of improvement.
Practical implications
Instructions of the Paris Declaration on Aid Effectiveness need to be followed. Particularly, civil society involvement and private sector participation should receive attention. A joint plan for improvement and collaboration of different stakeholders is needed.
Peer Review reports
Introduction
Afghanistan’s history is characterized by internal conflicts and wars which destroyed the economy and country’s infrastructures, including the healthcare system [ 1 ]. Afghanistan is highly dependent on international aid. Dependence on international aid is defined when the aid accounts for at least 10% of the Gross Domestic Product (GDP), and in the absence of this aid, the government cannot perform its main functions [ 2 ]. In 2018, the World Bank estimated that international aid constitutes nearly 40% of Afghanistan’s GDP [ 3 ].
Foreign aid has been effective in improving Afghans’ access to education and health services but still 43% of Afghans do not have access to primary health services and 55% live below the poverty line [ 4 ]. The health financing system in this country is fragile due to high out-of-pocket payment and reliance on donors [ 5 ]. The country’s health sector is financed by 72% out-of-pocket payments, 19.4% donations, 5.1% government budget and 3.5 other sources [ 6 ]. Lack of cooperation between the government and donors on how to spend the aid, political instability, low domestic production and investment, drug mafia, and illiteracy decreased the effectiveness of aid in Afghanistan [ 7 ]. The health of Afghans has improved over the past decade; however, because of poor management of health system, corruption, low quality of health services, lack of monitoring and control, the absence of a comprehensive national policy on universal health services coverage and incomplete implementation of development programs, Afghanistan has the lowest health indicators among the countries in the region [ 4 ].
In recent years, a large amount of aid has been delivered to Afghanistan. There are limited studies addressing aid management and effectiveness in this country. Studies mostly focused on the impact of aid particularly economic effects. Better processes and structures prevent waste of resources that can be used for other priorities. Since international aid plays an important and fundamental role in Afghanistan’s healthcare system, and this system is dependent on it, the way international aid is managed is of great and undeniable importance. The current study examined international aid management in the health sector of Afghanistan from the perspectives of health system managers and donors. It provides areas that require attention of policy makers to increase effectiveness.
Literature review
The main purpose of foreign aid is to reduce poverty and increase economic growth and development in recipient countries [ 8 ]. Official development assistance has increased steadily over the past years. Economic growth is a determinant of social development. Studies showed that public expenditure on health and education, and proper income distribution contributed to human development. Study by Gomanee et al. showed effects of international aid on alleviating poverty and infant mortality [ 9 ].
This is difficult to determine the real impact of foreign aid because development is a multi-dimensional issue that can be influenced by multiple stakeholders. Moreover, the methodology and scope of the assessment can bring different results. Foreign aid effectiveness has been questioned in empirical studies [ 10 ]. In some cases, foreign aid has been remarkably effective. However, there are examples of aid failure [ 11 ]. A study in African recipient countries showed that foreign aid did not influence development growth [ 12 ]. Another study on 33 aid receiving countries showed that 1% increase in the health aid share of GDP reduced the infant mortality rate by 0.18%. It suggested that the proper management of health aid in developing countries can help to improve public health in these countries [ 11 ]. Another study showed that foreign aid had positive effects on reducing poverty. Aid targeted at pro-poor programs such as agriculture, education, health and other social services has been effective [ 13 ].
Aid alone is not enough for achieving sustainable development. It can be effective in countries committed to improving public services and infrastructure and eradicating corruption [ 14 ]. Even though the foreign aid has been increased in recent years, the healthcare resources have not been enough to guarantee everyone’s access to primary healthcare. There is need for more foreign aid and national investment. The aid should be sustainable, predictable and long-lasting to support health promotion plans. The provision of aid-dependent healthcare services will be disrupted if the donors decrease or postpone the aid [ 15 ].
The impact of aid and its effectiveness can be influenced by the way aid is managed. There are many problems in the management of international aid. A large amount of the aid is not received by the recipient government and is spent on unnecessary activities, parallel programs, transaction costs, and donors’ office administration. Some aid programs do not focus on the needs and priorities of the recipient country. In addition to improving the situation of the disadvantaged groups in recipient countries, capacity and infrastructure building, and enhancing health system management, and procurement are necessary. They help health system to become independent in future and better use the resources. Some governments believe that conflicts in policymaking lead to the waste of resources. The donors do not have interest in capacity building [ 15 ]. Chung and Hwang believe that donors should not determine where and how the resources be used but collaborate with the government to assess the population needs and set the priorities [ 10 ].
A study in Syria showed that harmonization of aid and collaboration between stakeholders are perquisites of aid effectiveness. During 2016–2019, the aid to this country has not been harmonized and correlated with humanitarian needs instead aligning more with donor policies [ 16 ]. Another study in Pakistan found that foreign aid has had positive impact on health sector, although in long run, the effect was low. The reason might be that the aid has not been successful in institutional development. If the management of health system does not improve, the aid will create a debate burden [ 17 ]. In Ethiopia, the policy “one plan, one budget, one report” and foundation of country ownership and coordination of health partners, donors and governments resulted in accomplishments in healthcare [ 18 ].
Paris Declaration on International Aid Effectiveness 2005 offers a series of strategies to commit international donors to accountability and increase aid effectiveness. This document invites the developing countries to reduce poverty and improve the performance of institutions and eliminate corruption, and the donors to align with the goals of the recipient governments and cooperate with them, optimize the processes and share information to avoid duplication. Developing countries and donors should focus on the results and be accountable for them. Donors and recipient governments should take an integrated approach to aid effectiveness in policy making to improve quality of foreign aid [ 19 ].
Most studies focused on the effects of aid on economic growth, poverty and investment. The underlying assumption in Paris Declaration was that changes in process such as reducing aid fragmentation could increase the impact of aid. The Global Partnership for Effective Development Cooperation 2011 suggests the collaboration of governments, donors, private sector and civil society. Without proper management, international aid cannot help decreasing inequality and promoting development [ 20 ]. Therefore, it is necessary to study aid effectiveness, processes and management.
Data and method
Data and sample size.
This cross-sectional, descriptive and analytical study was conducted in 2022. The research population was the managers of health sector, both public and private, and international institutions based in Herat province of Afghanistan. The participants were chosen by random sampling. Due to the lack of similar studies, the sample size was determined based on Yaman’s formula and considering an error of 5% and the population size of 180, that made 110 people.
The inclusion criteria were at least two years of work experience in the health sector or international organizations. Incomplete questionnaires (more than 50% of the items have not been answered) were excluded from the study.
The data collection tool was the standard questionnaire of the International Health Partnership and Related Initiatives. It constitutes nine main dimensions, including donors’ support for the national health strategy, predictable financing, foreign aid on budget, public finance management system, procurement system, mutual accountability, technical support and training, civil society engagement and private sector participation, each of which has a number of subcategories and a total of 30 questions [ 21 ]. Due to the lack of an Afghan version of this questionnaire, it was translated to local language by two language experts. The content validity of the questionnaire was qualitatively assessed by 5 experts in the health sector in Afghanistan. The ambiguous items were corrected. The internal consistency of the questionnaire was evaluated by consulting 30 healthcare personnel. The stability, balance and homogeneity of the questions were measured through test and retest with the same people and calculating Cronbach’s alpha. The value of Cronbach’s alpha was 0.963, which is an acceptable value and shows the reliability of the tool.
Methodology
The data of this descriptive and analytical study was collected by self-administered approach. Descriptive studies (similar to this one) provide a detailed understanding of a phenomenon, while they might have limited generalizability and potential bias.
Questionnaires were presented to the study participants in person or by phone and email. All methods were performed in accordance with the relevant guidelines and regulations. In this study, the questions were scored from 1 to 5 (very poor to very good). The data was analyzed in two descriptive (mean and percentage) and analytical formats in SPSS. Independent variables were gender, education, managerial level and years of work experience. Scores given to each dimension of aid effectiveness were the dependent variables. Independent t-test (for data with normal distribution) or Mann-Whitney test (for data with non-normal distribution) were used to examine the relationships of the scores of dimensions and independent variables such as gender. Variance analysis was used to examine the relationships of scores and multivariate variables (such as education, age, work experience). Variance analysis shows the data’s volatility and consistency, which can impact the interpretations of the results. The normality of data distribution of quantitative variables was evaluated using the Kolmogorov-Smirnov test. It is used when there are two samples coming from two populations that can be different. The significance level of the tests was considered 5%.
Descriptive statistics
The average age of the study participants was 42.81 ± 8.36, the average work experience was 14.65 ± 6.06 years, and the average management experience was 10.25 ± 5. In addition, 96 people (87.3%) were men, 48.6% had a bachelor’s degree, and 41.3% a master’s degree. 73 people (67%) were middle-ranked managers. 74 people (67.3%) worked with international organizations and 85.5% completed a training course related to international aid. The knowledge of 15.1% of participants on international aid management was at average level. 64.5% of the participants received information about aid management at their workplace. 71 respondent (66.4%) studied medical and health programs (Table 1 ).
Empirical findings
The results of the survey showed that the highest scores were for foreign aid on budget (39/55 ± 20.15), technical support and training (42/50 ± 17.33), and predictable financing (23/50 ± 16.02) and the lowest score was in the field of civil society participation (35.24 ± 18.61) (Table 2 ). The performance in all dimensions of aid management hardly reached 50%.
More details about the dimensions of evaluation are provided in Table 3 . According to this table, the scores in all dimensions were in the range of 30–56. The lowest scores belonged to civil society participation. In general, the scores were very low and proved that all areas of aid management need improvement.
According to Table 4 , the managers of Afghanistan’s health sector and international organizations based in this country gave the lowest scores to the participation of civil society and the private sector in international aid programs, and the highest scores to considering the foreign aid in the budget. They had similar opinions about different dimensions of international aid management (Table 4 ).
The relationships between independent variables (gender and education) and the scores of different dimensions of aid management showed no meaningful difference. There were no changes in the dependent variable due the manipulation of these two independent variables. However, between managerial level and work experience with the scores, there was week relationship (Table 5 ).
The correlation coefficient showed that between the different dimensions of the questionnaire, there were meaningful relationships which mean the variables change together in the same direction. This indicates the strength of the linear relationship between variables. (Table 6 ).
In this cross-sectional study, international aid management in health sector of Afghanistan has been investigated from the perspective of the managers of health facilities and international organizations based in Herat province in 2022. The average age of study participants was 42.81 ± 36.8, the average work experience was 14.65 ± 6.06 years, and management experience of 10.25 ± 5.83 years. The majority of participants were men, had a bachelor’s degree and worked in middle management positions. A large number of participants worked with international organizations and mostly completed training course related to international aid management. Most of the participants were medical and health graduates. One third of them had fair knowledge about international aid management. The majority acquired the knowledge through work experience.
The managers of Afghanistan’s health system and international organizations believed that the management of international aid in health system of this country was at average level (score: 44.52 ± 13.27 (44% achievement).The performance was better in the dimension of aid on budget (55%) and the lowest was related to civil participation (36%). A study by the Organization for Economic Cooperation and Development (OECD) in 34 aid recipient countries showed that all countries were lagging behind the goals set in the Paris Declaration and needed more efforts and cooperation to improve the situation [ 22 ]. A study conducted in 2009 on the effectiveness of international aid in Afghanistan showed that the conditions in this country brough about challenges for the effectiveness of aid. These include: persistent insecurity, lack of national and international capacity, multiple and often inconsistent programs, ambiguous goals, unclear lines between military, humanitarian, and development interventions, widespread corruption, and lack of coordination among donors [ 23 ].
Donors’ support for the national health strategy was not adequate in Afghanistan (score: 50/23 ± 16.02 (50% achievement). There are challenges in developing national health strategies, control and evaluation of health services, evidence based decision-making and the use of national frameworks. A study in 2020, which investigated the impact of international aid on the growth of Afghanistan’s economy, found factors such as the non-cooperation of the Afghan government and donor countries as an obstacle to aid effectiveness. According to this study, in Afghanistan, there is neither an efficient and effective government institution, nor there are appropriate strategies on the use of international aid [ 24 ]. Similarly, the study on the international aid effectiveness in Ethiopia showed that the aid was scattered and there was no coordination between donors and the government and mutual accountability [ 25 ]. A study conducted on international aid dependence and political agreements in Afghanistan showed that aid was usually allocated based on the preferences of the donors rather than the priorities of the recipient country. Aid has largely focused on short-term goals, hindering medium- and long-term progress. Moreover, the aid may not be under the control of the recipient country [ 2 ]. Studies on foreign aid in other countries, including Nepal, showed that lack of attention to national preferences disrupted proper response to people’s needs [ 26 ].
Sometimes, the priorities are defined at global, regional or multi-country programs and often they are not completely aligned with national policies [ 27 ]. According to the World Health Organization (WHO), doners and the recipient countries might have different views on population needs [ 28 ]. Donors have different histories, experiences, and ideas that affect the projects they prefer to support. Sometimes, the lack of coordination and insularity greatly reduce the effectiveness of aid. For example, there are many international institutions and non-governmental organizations operating in Mali. Each of them has its own strategy, values, culture and work process. Acting in isolation and not integrating the goals with the national policies and structure and the lack of cooperation between the private and public sectors have reduced the effectiveness of aid in recent years [ 29 ]. In the allocation of the aid, the less considered issues are usually the goals of the recipient country [ 30 ]. The lack of coordination between donors is the most important challenge of aid management. Sustainable and effective change depends on the institutionalization of all policies at the local level [ 31 ]. A study by the African Development Bank in 2011 showed that the conflict of interests, weakness of the structures and the lack of capacity were the main challenges of international aid effectiveness. Short-term perspectives disrupt long term development plans [ 32 ].
The predictability of financing received an average score (55/39 ± 20.15 (55% achievement)) in this study which shows that the distribution of health financial resources, allocating aid based on the predetermined plans, and financing health centers through government’s long-term budget and the knowledge of the government on international donors’ programs are problematic. In a study by the Asian Development Bank in 2011, the predictability of development cooperation in Asian countries was evaluated at 78%, which was higher than Afghanistan [ 32 ]. To increase the predictability, it is necessary to have a comprehensive and transparent information system. A case study on international aid effectiveness in health sector of Ethiopia showed that no systematic and comprehensive data on the flow of aid was available [ 25 ]. In a study investigating the management of international aid in a developing country showed that transparency was an important indicator for identifying the problems, weaknesses and gaps in various areas of economic development. The study concluded that it is necessary to increase the involvement of interest groups in formulating strategies and policies [ 33 ].
According to the study participants, about 55% of international aid was placed in national budget. The donors set different strategies in this regard. For example, Italy recognizes the full ownership of the country’s health and medical institutions and gives the responsibility to implement the interventions to the local authorities in Afghanistan [ 34 ]. In contrast, spending a large part of Germany’s aid outside the Afghan government’s system has weakened the government and harmed the accountability of aid recipient institutions [ 35 ]. Similarly, conflicting programs or overlapping projects implemented by different donors reduced the effectiveness of aid according to Albanians [ 33 ]. In Africa, international aid does not flow through the government’s budget system, and is spent by non-governmental organizations or individuals. Local governments do not have enough information about the resources and projects [ 36 ]. Another study on the flow of aid in programs to fight tuberculosis, AIDS and malaria showed that there was no coherence between aid at the national level; aid was not flexible and a small part of it entered the government budget [ 27 ]. In a study that examined international aid management in Ethiopia, it was found that the government played an important role in coordinating international aid. In this country, there are specific national health programs in which the role of international aid is clear [ 25 ].
According to the respondents of this study, strengthening the financial management system of the public sector was not a priority for the donors (achieving 38% of the standard). WHO, in coordination with all key stakeholders in Afghanistan, helps to increase overall resources for health and improve the effectiveness of the investments [ 37 ]. However, the study by Dastan et al. about the determinants of financial protection in the health sector of Afghanistan showed that there was an urgent need to strengthen the overall health financing system in order to promote public health in this country [ 38 ]. Besharat Hossein reviewed the effects of international aid in Bangladesh and said the aid had little effectiveness due to the limited capacity of Bangladeshi institutions. If the government reforms its institutions and policies, foreign aid can contribute more effectively to the national economy [ 39 ]. In another study conducted by the United Nations Conference on Trade and Development (UNCTAD) on international aid allocated to less developed countries, found that donors’ financial resources can be hardly tracked due to the lack of a financial information system. The absence of transparency in spending resources reduced the donors’ trust [ 31 ]. A study in Sri Lanka showed that inefficiency of financial resources and weak institutions made foreign aid ineffective. In addition to effective policies, proper monitoring system supported by donors, and preventing the misuse of resources are needed [ 40 ].
Strengthening the supply system of the recipient country is an important part of aid management. It was scored 40.97 ± 19.55 (41% achievement). In Afghanistan, this aspect has not received enough attention. The donors’ support and use of the national procurement system need improvement. A study on the pros and cons of foreign aid in Albania indicated that donors were reluctant to use Albania’s public procurement systems. Strategic agreements between donors and the government, and forming working groups were suggested to adjust the aid flow [ 41 ]. The study of the Asian Development Bank on aid recipient countries showed that 47% of the aid flows through the public procurement systems. Further coordination between governments and donors is necessary [ 32 ]. The results of this study are similar to the present study.
In the current study, mutual responsibility of the donors and the government was not optimum (score:46.50 ± 19.26 (46% achievement)). There should be an evaluation system agreed with two parties. According to the report of the OECD, the mutual accountability in Afghanistan is a serious challenge, especially since the government and the donors insist on their own political goals, which creates an atmosphere of distrust and makes the implementation of programs difficult [ 22 ]. Asian Development Bank in 2011 indicated that countries were scored 54% in establishing mutual accountability and supporting the government in achieving its goals [ 32 ]. A study on foreign aid policy and its effect on Nepal’s growth showed that the capacity of country’s economy to implement programs was less than satisfactory due to the lack of proper information system and regular monitoring [ 42 ]. In Nigeria, the donors needed to monitor the implementation of plans and effective use of foreign aid. Without making political, economic and institutional reforms, the massive influx of foreign aid will be futile [ 43 ]. A review of foreign aid in Africa in 2012 concluded that responsible governance in this continent is a key to economic development [ 44 ].
Technical support and training help the recipient countries to better contribute in implementing the programs. Considering technical assistance in national programs and health strategies and supporting multilateral cooperation are necessary. The score of technical support in this study was 50.24 ± 17.33 (50%). The study of the Asian Development Bank showed that 45% of the donors paid attention to capacity building and education in recipient countries [ 32 ]. The Geneva Conference 2018 addressed the development of infrastructure and sustainable development in developing countries. The Kabul Conference 2010 focused on the rule of law and good governance and development. The International Monetary Fund supported establishing flexible and sustainable systems for health in Afghanistan [ 45 ]. In recent years, the spending on improving health sector management and policymaking has increased significantly. The aid focused on strengthening the health system through capacity building and planning [ 46 ]. In the absence of a proper support system, the aid is spent on daily affairs and does not lead to the transfer of technology and enhancing the capabilities of the country [ 47 ].
According to the WHO, low salaries and inappropriate working conditions discouraged the few skilled managers and entrepreneurs to participate in international aid projects in Afghanistan. The shortage of female healthcare providers is evident in this country [ 28 ]. The United States Agency for International Development (USAID) launched a midwifery training program to increase the number of female health workers and give women more access to necessary care. USAID created a system for monitoring and supported national diseases information system [ 48 ]. A study showed the need for skilled and knowledgeable managers committed to national values, and teamwork to determine priorities and establish a strong monitoring system. Unbalanced distribution of resources, lack of coordination, unnecessary costs, low efficiency and the lack of infrastructure are among the challenges of the country’s reconstruction process [ 49 ]. There have been various studies on the effectiveness of training provided by donors. The program of transferring technical skills to Afghan government employees by Germany has not been successful enough due to the lack of a monitoring system. Trained employees would not like to work in government facilities due to low wages. After acquiring the necessary skills, they are attracted to non-governmental organizations. Enhancing aid effectiveness requires a change in human resources strategies and enhancing security [ 35 ].
Civil society involvement in health sector programs and development is essential. The society should be empowered by receiving information, technical support and opportunities to participate. The Ministry of Health and the World Bank play important roles in supporting healthcare projects through non-governmental organizations [ 50 ]. However, this study showed that civil participation was not adequate (score: 35.24 ± 18.61, (35% achievement). A study in Albania concluded that the technical assistance and capacity building provided by donors and increasing the awareness of the civil society were among the benefits of aid assistance [ 41 ]. in Nepal, civil participation in country’s development is a challenge. Similar to Afghanistan, this country has religious and linguistic diversity, which together with its uneven terrain and inefficient government acts as an obstacle to national unity for growth [ 42 ]. Civil society needs information to participate in aid management. This information should be understood and analyzed by the civil society and encourage cooperation [ 51 ]. According to OECD, non-governmental organizations and the private sector are weak in developing countries. Lack of capacity hiders them to play their role in the development of the country [ 22 ].
Private sector participation received the lowest score (36 ± 17.55 (36% achievement), among different dimensions of aid management in Afghanistan. Private sector participation in the development and implementation of health sector policies needs donors’ support, information, and financial and technical assistance. The donors can achieve the goals of aid with the support of the private sector and the government. Because of people’s lack of trust to the government administrative system and the desire to achieve tangible results, the private sector compete with government organizations in attracting donated resources, but still they are depended on the support of the government. Some countries, such as the Netherlands, make financial support subject to allocating a part of the aid budget to non-governmental organizations. But, in low-income countries, this organizations do not have enough skills, information and power to cooperate with donors [ 52 ].
In recent years, the private sector has grown in Afghanistan. The government is determined to develop a solid policy framework and establish institutions and systems aimed at ensuring higher quality private services and a long-term and sustainable role for the private sector. Afghanistan is at the beginning of privatization; evidence shows that the Ministry of Health can promote a more efficient and effective private sector [ 53 ]. Based on the report of the UNCTAD, if donors cooperate with the private sector and civil society to set priorities and implement programs, the aid can be effective [ 31 ].
The performance in all dimensions of aid management hardly reached 50%. The managers of Afghanistan’s health sector and international organizations based in this country believed that international aid management in Afghanistan’s health sector needs to be improved. The standards of the Paris Declaration on Aid Effectiveness could be helpful in this regard. According to the studied managers, the best dimension of aid management was the inclusion of international aid in government budget. However, civil society involvement and the private sector participation in planning and implementing aid programs was not satisfactory.
This study showed the areas of aid management that needs improvement in Afghanistan. According to the results, in order to improve international aid management, it is necessary to improve the resources management with the cooperation of international donors, to strengthen health planning, and to develop an effective administrative and management system. Promoting transparency, accountability, and fighting against corruption are the perquisites of aid effectiveness. Economic and social development and investment in infrastructure and cooperation between the government and donors and the private sector will improve public governance. Finding ways to reduce the dependence of the health sector on international aid will be a sustainable solution. The government of Afghanistan should determine the needs of its population and direct the aid towards the priorities of the country which cannot be achieved with government budget.
Study limitations and future studies guidelines
Data collection coincided with the change of government in Afghanistan. The participants of the study stated that due to the extensive changes in administrative and management structures and unclear processes, their opinions addressed the situation before the changes in 2021. Still, this study provides areas for the improvement of aid management in the studied country. Future studies can build upon the findings of this research and conduct in-depth exploration of areas of aid effectiveness and designing detailed programs of improvement. A joint plan for improvement and collaboration of different stakeholders is needed.
Data availability
Data are not publicly available to preserve individuals’ privacy.
Abbreviations
Gross Domestic Product
Organization for Economic Cooperation and Development
United States Agency for International Development
World Health Organization
United Nations Conference on Trade and Development
Frost A, Wilkinson M, Boyle P, Patel P, Sullivan R. An assessment of the barriers to accessing the Basic Package of Health Services (BPHS) in Afghanistan: was the BPHS a success? Globalization Health. 2016;12(1):1–11.
Article Google Scholar
Bizhan N. Building legitimacy and state capacity in protracted fragility: The case of Afghanistan. Available at SSRN 3166985. 2018.
Cooper R. Aid dependency and political settlements in Afghanistan. 2018.
Afghanistan TD. The state of Afghanistan’s health system. http://www.dailyafghanistan.com/2021 [.
Zeng W, Kim C, Archer L, Sayedi O, Jabarkhil MY, Sears K. Assessing the feasibility of introducing health insurance in Afghanistan: a qualitative stakeholder analysis. BMC Health Serv Res. 2017;17(1):1–9.
Health Financing. Strategy 2019–2023. Islamic Republic of Afghanistan Ministry of Public Health General Directorate of Policy. Planning, and International Relations Health Economics and Financing Directorate; 2019.
ÇEVİK S. The impact of International Aid on Economic Growth of Afghanistan. LAÜ Sosyal Bilimler Dergisi. 2021;11(2):99–114.
Google Scholar
Izadkhasti H. The effects of Foreign Aid on Government fiscal behavior in selected developing Countries., in Department of Economic 2008. University of Esfahan; 2008.
Gomanee K, Girma S, Morrissey O. Aid, public spending and human welfare: evidence from quantile regressions. J Int Development: J Dev Stud Association. 2005;17(3):299–309.
Chung D, Hwang J. An Economic and Social Impact of International Aid at National Level: application of spatial panel model. World. 2022;3(3):575–85.
Ekanayake E, Chatrna D. The effect of foreign aid on economic growth in developing countries. J Int Bus Cult Stud. 2010;3:1.
Loxley J, Sackey HA. Aid effectiveness in Africa. Afr Dev Rev. 2008;20(2):163–99.
Mahembe E, Odhiambo NM. Foreign aid and poverty reduction: a review of international literature. Cogent Social Sci. 2019;5(1):1625741.
Adamu PA. The impact of foreign aid on economic growth in ECOWAS countries: A simultaneous-equation model. WIDER Working Paper; 2013.
WHO. Health Systems Financing: the Path to Universal Coverage. 2010.
Alkhalil M, Ekzayez A, Meagher K, Al Aref M, Turkmani R, Abbara A, et al. An analysis of humanitarian and health aid harmonisation over a decade (2011–2019) of the Syrian conflict. medRxiv. 2024;202404:17–24305968.
Anwar A, Khan G, Anwar M. Impact of Foreign Aid on Health Sector of Pakistan. Global Econ Rev. 2020;4:1–10.
Teshome SB, Hoebink P. Aid, ownership, and coordination in the health sector in Ethiopia. Dev Stud Res. 2018;5(sup1):S40–55.
OECD. The Paris Declaration on Aid Effectiveness. 2005 https://www.oecd.org/dac/effectiveness/parisdeclarationandaccraagendaforaction.htm2005 [.
Janus H, Marschall P, Öhler H. Bridging the gaps: an integrated approach to assessing aid effectiveness. German Dev Inst. 2020;12.
International Health Partnership. Progress in the international health partnership and related initiatives; performance report. 2016.
OECD, SURVEY ON MONITORING THE PARIS DECLARATION OVERVIEW OF THE RESULTS. OECD. 2006;8(2):130.
Roberts RE, editor. Reflections on the Paris Declaration and Aid Effectiveness in Afghanistan2009.
Abbasi IaMR. Iran’s financial aid to Afghanistan: its goals and economic effects Faslnama E. Rawabet E Khareji. 2011;3(3):195–229.
Alemu G. A case study on aid effectiveness in Ethiopia. Wolfensohn Center for Development; 2009.
Karkee R, Comfort J. NGOs, foreign aid, and development in Nepal. Front Public Health. 2016;4:177.
Article PubMed PubMed Central Google Scholar
Piva P, Dodd R. Where did all the aid go? An in-depth analysis of increased health aid flows over the past 10 years. Bull World Health Organ. 2009;87:930–9.
Diabré Z, Lovelace CF, Norberg C, editors. International development assistance and health: the report of working group 6 of the Commission on Macroeconomics and Health2002.
Dante I, Gautier JF, Marouani MA, Raffinot M, Mali. Dev Policy Rev. 2003;21(2):217–34.
Riddell RC. Does foreign aid really work? OUP Oxford; 2008.
UNCTAD secretariat. THE LEAST DEVELOPED COUNTRIE, REPORT. 2000.
ADB. Aid Effectiveness Report 2011 Overall Achievements on Paris Declaration Commitments. 2011.
Zeneli M, Reci A, Harxhi G. External assistance and Albanian citizens perceptions about effectiveness of coordination. Mediterranean J Social Sci. 2014;5(3):103.
Fund G. Afghanistan Country overview description https://data.theglobalfund.org/location/AFG/overview?components=Tuberculosis 2022 [.
Totakhail ML. Foreign Aid and Economic Development in Afghanistan. Unpublished Doctoral dissertation) The University of Erfurt. 2011.
Helleiner G. External conditionality, local ownership and development. Toronto, University of Toronto; 2000. pp. 82–97.
World Health. Organization WHO Afghanistan country office, 2019. Switzerland: Geneva; 2019.
Dastan I, Abbasi A, Arfa C, Hashimi MN, Alawi SMK. Measurement and determinants of financial protection in health in Afghanistan. BMC Health Serv Res. 2021;21(1):1–15.
Hosseinzadeh BA. Historical background of Afghanistan’s health system https://www.bbc.com/persian/afghanistan/2009/04/090407_hestory_of_helth_afghanistan2021 [.
Madhusanka M. The effect of foreign aids on economic growth of Sri Lanka. Int J Innovative Sci Res Technol. 2021;6(1):114–8.
Reci A, editor. Editor advantages and disadvantages of foreign assistance in Albania. Forum Scientiae Oeconomia; 2014.
Bista R. Foreign aid policy and its growth effect in Nepal. EconoQuantum. 2006;3(1):109–41.
Mbah S, Amassoma D. The linkage between foreign aid and economic growth in Nigeria. Int J Economic Practices Theor. 2014;4(6):1007–17.
Akinola AO. Foreign aids in Africa: from realities to contradictions. Int J Soc Econ. 2012;22(4).
International Monetary Fund. Afghanistan Country overview description https://data.theglobalfund.org/location/AFG/overview?components=Tuberculosis2022 [.
Karyda K, Moka D. Afghanistan: the changing state of its Health System and the contribution of NGOs and the International Community. Int J Social Sci Res Rev. 2022;5(10):160–76.
Sengupta A. International Aid and Access to Health Products. Health action international. 2012:11.
USAID, Health. https://www.usaid.gov/afghanistan/health.2022 [.
Ramyar SJ. Foreign Aid and Socio-Economic Development (Case Study: Afghanistan), in Social sciences. Ferdowsi University of Mashhad; 2019. p. 252.
Walraven G, Yousofzai Y, Mirzazada S. The World Bank’s health funding in Afghanistan. Lancet. 2021;398(10306):1128.
Article PubMed Google Scholar
Aid B. Survey on Monitoring the Paris Declaration. Making Aid more effective by 2010. Paris: Organisation for Economic Co-operation and Development; 2008.
Rogerson A, Hewitt A, Waldenburg D. The International Aid System 2005–2010: Forces For and Ag or and Ag or and Against Change. 2004.
Cross HE, Sayedi O, Irani L, Archer LC, Sears K, Sharma S. Government stewardship of the for-profit private health sector in Afghanistan. Health Policy Plann. 2017;32(3):338–48.
Download references
Received by Mashhad University of Medical Sciences, Iran.
Author information
Authors and affiliations.
Department of Management Sciences and Health Economy, School of Heath, Mashhad University of Medical Sciences, Mashhad, Iran
Noorullah Rashed, Hamidreza Shabanikiya, Leili Alizamani & Fatemeh Kokabisaghi
Health Network, Herat, Afghanistan
Noorullah Rashed
Department of Biostatistics, School of Heath, Mashhad University of Medical Sciences, Mashhad, Iran
Jamshid Jamali
Social Determinants of Health Research Center, School of Heath, Mashhad University of Medical Sciences, Mashhad, Iran
Hamidreza Shabanikiya & Fatemeh Kokabisaghi
You can also search for this author in PubMed Google Scholar
Contributions
FK designed the study and supervised it; NR: collected data and wrote the report; HSH and JJ: designed methods and analysis; LA: wrote the paper;
Corresponding author
Correspondence to Fatemeh Kokabisaghi .
Ethics declarations
Consent for publication.
Not applicable.
Competing interests
The authors declare no competing interests.
Ethics approval and consent to participate
The study protocol received the code of ethics from the Graduate Education Committee of Mashhad University of Medical Sciences, Iran (code of ethics: IR.MUMS.REC.1400.372). Informed consent was acquired from all participants after explaining the purpose of the research, and answering their questions. They could withdraw from participating in the research at any time. The principles of confidentiality and research ethics were followed.
Ethical guidelines
All methods were performed in accordance with the relevant guidelines and regulations.
Additional information
Publisher’s note.
Springer Nature remains neutral with regard to jurisdictional claims in published maps and institutional affiliations.
Rights and permissions
Open Access This article is licensed under a Creative Commons Attribution-NonCommercial-NoDerivatives 4.0 International License, which permits any non-commercial use, sharing, distribution and reproduction in any medium or format, as long as you give appropriate credit to the original author(s) and the source, provide a link to the Creative Commons licence, and indicate if you modified the licensed material. You do not have permission under this licence to share adapted material derived from this article or parts of it. The images or other third party material in this article are included in the article’s Creative Commons licence, unless indicated otherwise in a credit line to the material. If material is not included in the article’s Creative Commons licence and your intended use is not permitted by statutory regulation or exceeds the permitted use, you will need to obtain permission directly from the copyright holder. To view a copy of this licence, visit http://creativecommons.org/licenses/by-nc-nd/4.0/ .
Reprints and permissions
About this article
Cite this article.
Rashed, N., Shabanikiya, H., Alizamani, L. et al. International aid management in Afghanistan’s health sector from the perspective of national and international managers. BMC Health Serv Res 24 , 1001 (2024). https://doi.org/10.1186/s12913-024-11260-0
Download citation
Received : 06 February 2024
Accepted : 27 June 2024
Published : 28 August 2024
DOI : https://doi.org/10.1186/s12913-024-11260-0
Share this article
Anyone you share the following link with will be able to read this content:
Sorry, a shareable link is not currently available for this article.
Provided by the Springer Nature SharedIt content-sharing initiative
- Afghanistan
- International aid
- Foreign aid
- Healthcare system
- International Health Partnership and related initiatives
BMC Health Services Research
ISSN: 1472-6963
- General enquiries: [email protected]
Thank you for visiting nature.com. You are using a browser version with limited support for CSS. To obtain the best experience, we recommend you use a more up to date browser (or turn off compatibility mode in Internet Explorer). In the meantime, to ensure continued support, we are displaying the site without styles and JavaScript.
- View all journals
- Explore content
- About the journal
- Publish with us
- Sign up for alerts
- Open access
- Published: 28 August 2024
A new multi-analytical procedure for radiocarbon dating of historical mortars
- Sara Calandra 1 ,
- Emma Cantisani 2 ,
- Claudia Conti 3 ,
- Barbara Salvadori 2 ,
- Serena Barone 4 , 5 ,
- Lucia Liccioli 4 ,
- Mariaelena Fedi 4 ,
- Teresa Salvatici 1 ,
- Andrea Arrighetti 6 ,
- Fabio Fratini 2 &
- Carlo Alberto Garzonio 1
Scientific Reports volume 14 , Article number: 19979 ( 2024 ) Cite this article
Metrics details
The overarching challenge of this research is setting up a procedure to select the most appropriate fraction from complex, heterogeneous materials such as historic mortars in case of radiocarbon dating. At present, in the international community, there is not a unique and fully accepted way of mortar sample preparation to systematically obtain accurate results. With this contribution, we propose a strategy for selecting suitable mortar samples for radiocarbon dating of anthropogenic calcite in binder or lump. A four-step procedure is proposed: (I) good sampling strategies along with architectural and historical surveys; (II) mineralogical, petrographic, and chemical characterization of mortars to evaluate the feasibility of sample dating; (III) a non-destructive multi-analytical characterization of binder-rich portions to avoid geogenic calcite contamination; (IV) carbonate micro-sample preparation and accelerator mass spectrometer (AMS) measurements. The most innovative feature of the overall procedure relies on the fact that, in case of positive validation in step III, exactly the same material is treated and measured in step IV. The paper aims to apply this procedure to the ancient mortar of the Florentine historical building (Trebbio Castle), selecting micro-samples suitable for dating in natural hydraulic mortars. The discussion of the mortar dating results with the historical-archaeological hypotheses provided significant insights into the construction history of the building.
Similar content being viewed by others
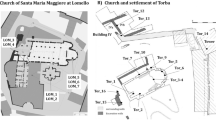
Integrated multi-analytical screening approach for reliable radiocarbon dating of ancient mortars
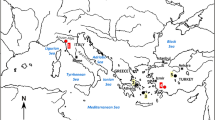
An innovative approach for provenancing ancient white marbles: the contribution of x-ray diffraction to disentangling the origins of Göktepe and Carrara marbles
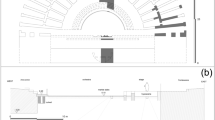
Early exploitation of Neapolitan pozzolan ( pulvis puteolana ) in the Roman theatre of Aquileia, Northern Italy
Introduction.
Radiocarbon dating is one of the most widely used dating techniques in the field of archaeology and Cultural Heritage 1 . This technique is used to typically date organic finds (such as charcoal, wood, bone, or textiles) but also inorganic materials 2 , such as carbonate compounds, i.e., lead white 3 , 4 . Mortar is an artificial product which has been prepared and used by humans since ancient times, mainly consisting of a binder, some aggregates and possible additives.
In mortar and plaster samples, plant remains, such as charcoal, vegetal, and straw fragments, are the most dated fraction with radiocarbon method ( 14 C), as reported in literature 5 , 6 , 7 . Other approach concerns the dating with Optically Stimulated Luminescence (OSL) of quartz and feldspar aggregates 8 , 9 , 10 .
In addition, among possible applications to inorganic carbon-based materials, the use of 14 C method for dating ancient mortars was proposed as early as in the 1960s, applying the method to the inorganic binder 11 , 12 , 13 . In mortars, the inorganic radiocarbon-datable component is calcite, which is formed by the reaction of calcium hydroxide with atmospheric CO 2 during the setting of the material (the so-called anthropogenic calcite). Air-hardening mortars are the most suitable for dating because they set and harden incorporating atmospheric CO 2 . However, since mortars are heterogeneous materials, other sources of C, which may contaminate the 14 C concentration, can be present in the mortar samples.
Contaminations can be due to the presence of:
unburned carbonate of stone used for the production of lime and carbonate aggregate present in the mixture (geogenic calcite). These two sources make the sample older than expected;
(re)crystallized secondary calcium carbonates and products of delayed hardening (so-called secondary calcite). Secondary calcite forms after the initial hardening of the mortar, thus, causing an apparent rejuvenation of the sample.
Moreover, the type of binder of the mortar sample may not be quite ideal, as there are historical mortars with not totally air lime binder.
Selection of the datable fraction and elimination of potential contamination is a challenge for the international radiocarbon community 14 , 15 , 16 , 17 . Despite numerous efforts as evidenced by the extensive literature in the field carried out by the scientific community, an analytical workflow for characterization and dating of inorganic fraction of mortars has not been established.
This paper aims at discussing our sample selection procedure for radiocarbon dating of historic mortars, from the preliminary comprehensive characterization of the material to the sample preparation for the 14 C-AMS measurement. Archaeological and historical survey coupling with accurate sampling, and then in-depth minero-petrographic and chemical characterization of the mortars are the first two steps (Step I and II), respectively. Separation of binder from the aggregate coupled with characterization of the separated carbonate fractions is mandatory (Step III). Proactive identification of the origin of calcite allows for the reduction of the possible contamination risk, thus obtaining accurate 14 C measurement by AMS (Step IV).
As far as Step II is concerned, a multi-analytical characterization procedure of the mortar fragments, i.e. optical and electron microscopy (OM, SEM–EDS), X-ray diffraction on powders (XRPD), thermogravimetric analysis (TGA), infrared spectroscopy (FTIR) was designed.
In Step III, a non-destructive, original approach capable of identifying the origin of calcite (geogenic and anthropogenic calcite) was explored using XRPD, OM-cathodoluminescence (OM-CL), ATR-FTIR, micro-Raman. A new experimental set-up for the collection of CO 2 evolving from the selected calcite was installed, by integrating an acidification reactor into our so-called Lilliput graphitization reactors, which are optimized for microgram-sized samples 18 , 19 . The graphitization line is used to obtain graphite samples whose residual 14 C abundance is measured by AMS.
The procedure was validated in architectural contexts, such as the Tuscany historical building (Trebbio Castle in Florentine surroundings).
Analytical procedure
Step i: sampling and the issue of chronology questions.
Accurate dating of mortar in masonry requires a comprehensive approach involving collaboration between experts in mortar analysis, archaeologists and architects who understand wall stratigraphy 20 , 21 . Precise sampling is crucial and begins with well-defined research questions related to chronology, along with documentation and historical research and analysis of the masonry 22 (Fig. 1 , step I). For example, if the aim is to determine the age of construction, it is important to avoid areas of repair or renovation. However, if the focus is on determining the period in which the building was in use, these repaired or renovated areas may be of greater importance. Mortar between stone blocks is likely to be original if the stone block overlies the mortar; on the other hand, if the mortar protrudes beyond the stone block, this indicates a later intervention. Care should be taken when selecting samples from the ground, i.e. from collapsed ruins, as these may have been transported or weathered, thus being their association with the event to be dated not accurate.
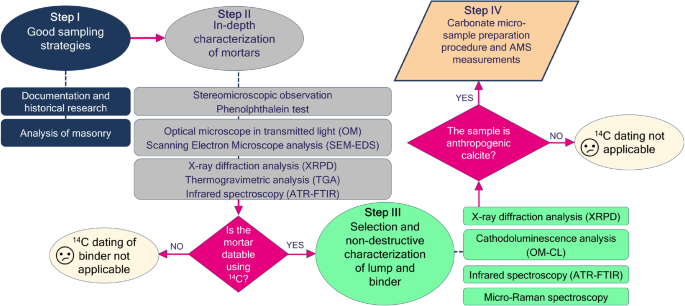
Graphical representation of the new multi-analytical procedure for radiocarbon dating of historical mortars.
Mismatches in the dating results can arise due to different factors, such as mortar constituents (the type of binder and aggregate) or environmental factors (the state of preservation, which may be due to e.g. recrystallization and delayed hardening).
For instance, bedding mortars or core mortars are generally less altered over time than plaster and are less exposed to the external environmental parameters 23 .
To minimize secondary carbon sources, sampling sites should be carefully selected, favoring areas that are less exposed to weathering over exterior surfaces 20 , 21 . Analyzing samples from greater depths and intermediate heights helps to mitigate the influence of ambient water, which can introduce younger samples through rainfall or surface water or obvious aging effects from dissolved geological carbonates in groundwater and soil moisture.
As the slaked lime (Ca(OH) 2 ) absorbs CO 2 from the atmosphere, the setting and hardening process starts from the surface and progresses inwards. Delayed hardening in the inner parts of thick walls can lead to inaccuracies in dating results 24 . Optimal samples should be taken at a depth close to the wall surface, deep enough to avoid problems with the surface, but not too deep to have problems with delayed hardening. If carbonate aggregates are present, careful sampling is essential to limit dispersion.
In summary, careful sampling and consideration of various factors are crucial for successful 14 C dating results in mortar. These considerations and methods contribute to the robustness and reliability of mortar dating in archaeological investigations.
The evaluation of the degree of carbonation of the mortar with the phenolphthalein test is the first mandatory characterization step. Phenolphthalein indicates the presence of calcium hydroxide in the mortar. A sample that is not fully carbonated must be excluded for 14 C dating. The test can be carried out in situ on the masonry or in the laboratory on a sample.
Step II: analytical procedure to characterize mortars for dating
To characterize mortars and select those materials that can be suitable for dating purposes, it is essential to determine the composition of all the constituents of the mixture, their relative amounts (binder/aggregate ratio—B/A), the nature of binder and aggregates, the constituents within the binder, as well as the degree of carbonation. In fact, the information we can get from different analytical techniques can give us hints about the manufacturing process of the materials. In particular, it can suggest us whether the basic conditions for applying radiocarbon dating are respected and can support us to choose the best approach to select the most appropriate fraction to be dated. For example, the aforementioned B/A ratio allows us to understand how much material we have to sample to get enough mass at the end of the selection procedure.
For a comprehensive characterization, several investigations must be performed, each useful in reconstructing the overall picture and providing key information to select or exclude material for dating (Fig. 1 , step II). The complementarity of multiple investigations is crucial for an accurate and full understanding of the material. Indeed, the investigations make it possible to determine the relative chronology of different construction phases within a building or site 25 , 26 . Here following the summarized description of the analytical techniques proposed for characterization.
Thin-section observation of mortar under an OM in transmitted light provides essential insights into the nature of binder, aggregate and lumps 19 , 27 .
For the binder, OM provides information on the texture (micritic, microsparitic, sparitic), the mineralogical composition, the birefringence colors, the structure and the interactions with the aggregate. Moreover, OM allows us to classify the binder as: air lime, natural hydraulic lime, air lime with addition of pozzolanic materials (i.e. cocciopesto , volcanic ash and clay minerals) and modern hydraulic binder 28 , 29 .
The description of the aggregate is crucial for the evaluation of contamination sources, taking into account mineralogical composition, particle size distribution, amount of binder with respect to aggregate (B/A) ratio, macroporosity and alteration products.
Petrography is also beneficial for the identification of lumps and organic fragments in the mortar. OM observation make it possible to recognize the type of lumps and distinguish between residues of stones used to make binders and binder residues.
The observation of lumps with OM allows us to recognize their types and origins, achieving information on the rock used to produce lime as well as suggestions on production technologies 27 .
Petrographic observation contributes to assess the uniformity of the binder and to identify zones of different crystallinity due to partial recrystallization by circulating water. Sources of contamination, such as recrystallization of calcite and carbonate aggregates, can lead to exclude samples from 14 C dating 21 .
Modern binders should be eliminated from dating, since the dating principle is not applicable to these types of binders. Particular attention should be paid to magnesium binders 30 . The 14 C dating outcomes may be affected by the presence of much younger 14 C, due to the properties of minerals produced upon carbonation (such as magnesite and nesquehonite).
XRPD analysis of bulk samples includes the mineralogical composition of both the binder and the aggregate, which can be integrated with the identified phases in thin sections. Single lumps and binder-rich portions can be also analyzed. All these data yield crucial information, revealing whether the mortar is non-carbonated (portlandite), if the sample contains magnesium lime (brucite, hydromagnesite, magnesite), or if the binder exhibits hydraulic properties 31 (tobermorite, hydrogarnet), or if secondary reactions occurred which lead to the formation of new phases (gypsum, hydrotalcite, hydrocalumite). The presence of these latter two phases in mortar binders strongly influences the radiocarbon dating of lime mortars, because of their high (CO 3 ) 2– anion capture capability 32 , 33 . The presence of gypsum indicates that the binder has altered, suggesting an open system and therefore a context subject to contamination from the external environment 34 .
Observations under the optical microscope can be further enhanced and supplemented by SEM–EDS which combines microscopy and X-ray spectroscopy to obtain detailed information on the morphology and elemental composition of mortar constituents. Semi-quantitative elemental analysis is useful for: (1) estimating the provenance of raw material through the analysis of residues of stones used for lime production; (2) obtaining information on the hydraulic index (HI) 35 and the overall composition of the binder, including the possible presence of Ca and Mg based binder, and of silico-aluminates ferriferous phases; (3) evaluating changes in elemental composition within reaction rims areas; (4) characterizing lumps, especially if they have a heterogeneous texture; (5) achieving micro-chemical information about the aggregate and providing hypothesis on its provenance.
TGA is used in the analysis of historical mortars for evaluating hydraulic behavior; it involves subjecting a sample to controlled temperature changes while measuring its mass as a function of temperature. TGA serves for characterization of binder materials (air binder, hydraulic binder, gypsum, etc.) 36 , 37 . Moreover, the TGA results can be integrated with the HI value calculated from punctual micro-chemical analyses carried out with SEM-EDS 38 .
Step III: Selection and characterization of the powder for the screening of CaCO 3 origin
Upon assessing that the sample exhibits datable characteristics, as a consequence of all the analyses performed in Step II, the following process involves the selection and further characterization of the carbonate fraction. The binder calcite has the same chemical composition as burned carbonate rocks or carbonate aggregates, but different textural, isotopic signatures and mechanical properties.
A mechanical separation of binder-rich bulk and lump was performed, starting from a selection under stereomicroscope. For bulk samples, a portion enriched with binder and lumps is separated, then sieved to 63 µm and lightly crushed.
Our approach aims at finding non-destructive techniques able to determine the origin of the calcite in the powder samples selected for dating (Fig. 1 , step III). Non-destructive techniques allow the preservation of the sample mass so that the same sample can be subjected to several analytical procedures and treated for 14 C analysis (Fig. 1 , step IV).
The different origin of carbonates (geogenic and anthropogenic) can be detected by the different distortions in the lattice structure within small crystallites. In principle, different types of calcite interact with electromagnetic radiation in a way that depends on the atomic arrangement. FTIR and Raman spectroscopies can be used to identify short-range order at the molecular level. In addition, CL analysis, which is conventionally used to assess the origin of calcite, in our approach is combined with ATR-FTIR and micro-Raman.
The most important advantage in this non-destructive approach is that the exactly same powder is analyzed in OM-CL, ATR-FTIR and micro-Raman; and if the sample is mainly constituted by anthropogenic calcite, it is used for step IV.
CL is a petrographic technique which represents an additional way of examining thin sections or powder samples of carbonate specimens 39 . The phenomenon of CL of mortars has been discussed since 1997 and has been used in numerous studies to evaluate the origin of carbonates 13 , 40 , 41 . Different densities and distribution of atomic defects in the calcite crystal structure serve as markers to identify the origin of calcite. Considering this principle, geogenic calcite and anthropogenic calcite may have different luminescence intensities due to the different formation process.
The phenomenon can be easily observed with petrographic microscopes equipped for CL analysis (OM-CL), this instrumentation is relatively inexpensive and easy to use. For the non-destructive analysis of powders, we used OM-CL. The disadvantage of this technique lies in the resulting color hues, especially when multiple emissions of the same powder result in a composite hue. Typically, a qualitative analysis was performed, just attributing “hues” to the different observed colors (see for example tile red, dull purple, brown, dark brown, grey, dull grey and black). In such a framework, interpretation of data could be influenced by the operator him/herself 20 , 42 , 43 . This problem can be solved by combining several analytical techniques to obtain a validated and unambiguous result.
In the context of mortar dating, spectroscopy has already been used to distinguish the origins of calcite. As demonstrated in previous studies 44 , 45 , 46 conventional Fourier transform infrared spectroscopy in transmission mode with KBr pellets can be employed for rapid sample analysis, using the heights of v 2 and v 4 bands.
In order to use non-destructive analysis and preserve the sample for further analysis and dating, ATR-FTIR was tested on samples with known composition and origin to establish whether this mode could lead to the same results as the FTIR technique with KBr pellet 47 .
Since it has been shown that differences in grinding degree affect peak widths and relative heights of carbonate archaeological materials 34 , 48 , samples with same preparation procedures were analyzed to replicate the typical pre-treatment that might be carried out on unknown samples for dating purposes.
The distinct trend lines highlight the systematic differences in v 2 versus v 4 peak heights in ATR-FTIR mode for calcites formed through various processes. Two trend lines were created (geogenic and anthropogenic calcites), which can help to determine the origins of unknown samples, offering preliminary insights into their formation. The ability to discern calcite origins through the ATR technique is particularly advantageous in the field of mortar dating, as powdered samples can be collected and reused for dating if they contain anthropogenic calcite.
- Micro-Raman
Micro-Raman spectroscopy is a valuable tool for the characterization of mortars, enabling high lateral resolution analysis of the mineral phases of aggregates and binder components 49 , 50 . So far, some studies have demonstrated that micro-Raman spectroscopy can be successfully used to estimate the content of cations (Mg 2+ , Fe 2+ and Mn 2+ ) in carbonates, as the vibrational frequencies of the translational (T) and librational (L) modes of carbonates are significantly related to their cation composition 51 , 52 . Raman spectroscopy has been used to investigate variations in atomic bonding in biogenic calcite crystals and to distinguish the degree of crystallinity of calcium carbonate in biological materials by assessing the frequencies and width of the v 1 and v 4 bands 53 . Raman analysis of CaCO 3 polymorphs in 54 found that the amorphous calcium carbonate exhibits a broad peak in the lattice mode region (below 400 cm −1 ) and that the most prominent band associated with the carbonate ion at around 1085 cm −1 , which appears as broader and significantly less intense than usual, slightly shifts towards lower wavenumbers.
We carried out a study to determine the origin of calcite using micro-Raman spectroscopy. The potential to distinguish between geogenic and anthropogenic calcite using micro-Raman spectroscopy was established for the first time by the authors 55 .
Raman spectroscopy and statistical methods have shown that the anthropogenic calcite samples exhibit a broadening of the L, v 1 and v 4 bands (calculated from FWHMs) compared to geogenic calcite samples.
Structural disorder within the calcite crystals or the presence of low crystalline order is reflected in relatively broad FWHM values and wavenumber shifts. The wider and shifted toward lower wavenumber is the spectral band, the lower the crystallinity within the mineral.
The influencing parameters (including band position, band intensity, the area covered by the bands and the FWHM values of L, v 4 and v 1 ) for distinguishing the origins of calcite were successfully identified and they can be used to determine the origin of calcite in unknown samples intended for dating.
The potential of micro-Raman on distinguishing different calcite domains was also confirmed by Toffolo et al. 56 . In this paper, the micro-Raman analyses were performed on petrographic thin sections in archaeological lime samples.
Step IV: carbonate micro-sample preparation and AMS measurements
The limited sample material due to the high possible level of heterogeneity of the mortars, the sample loss during the characterization step and the highly selective pre-treatment process, motivates us to use the micro-sample 14 C preparation.
In this framework, the so-called Lilliput graphitization line at the LABEC laboratory in Florence, one of the laboratories of CHNet, the INFN network for Cultural Heritage, was integrated with a reaction chamber designed for the extraction of CO 2 from carbonates (Fig. 1 , step IV). The Lilliput line is particularly useful in the case of mortar treatment, because it allows managing samples as small as only 50 µg of carbon, well below the limit of the “traditional” larger samples of about 700 µg 18 , 57 . Such small processed masses provided the possibility to investigate the feasibility of dating even individual lumps of binder in mortar samples.
Typical processing masses for mortar samples are:—approx. 2.5 mg in the case of lump; —approx. 5 mg in the case of bulk mortar.
Acid dissolution and Lilliput graphitization reactors
The extraction of C from the selected inorganic fraction of the mortar is carried out by acid dissolution. The carbonate sample, mechanically separated and previously characterized with non-destructive techniques, is treated with H 3 PO 4 in the acidification line.
For bulk samples, 2 evolving CO 2 fractions are usually collected per sample: the first in a few seconds (0–10/30 s) and the second thereafter (10/30–60 s). The selected shortened reaction time is intended to avoid the risk of geological contamination, at least in the first fraction, as contaminants may still be present despite mechanical separation. In the case of lump samples, a fraction from the first few seconds of the reaction is collected without the risk of contaminants reacting with the acid.
The CO 2 extracted from the acidification line is then cryogenically transferred into the graphitization chamber using liquid nitrogen. The amount of CO 2 collected is monitored by pressure measurements. Typically, about 100 mbar of CO 2 is collected for each sample; this pressure basically corresponds to about 50 µg of graphite at the end of the reaction given the inner volume of the Lilliput reaction chambers. The graphitization reaction occurs on small copper inserts previously prepared with Fe catalyser pressed on them and is triggered at 600 °C in presence of H 2 excess; the reaction produces water, which is trapped within the cold finger. After the graphitization process, the copper inserts with the graphite deposited on them are mounted in specially modified aluminum holders that fit into the ion source of the accelerator to measure the radiocarbon concentration.
Results and discussion
Application of the procedure on historical building: trebbio castle.
The analytical procedure for dating was applied to mortar samples from the walls of the tower of the Trebbio Castle, one of the most important and significant examples of aristocratic villas owned by the Medici in the area around Florence (Mugello) 58 (Fig. 2 ).
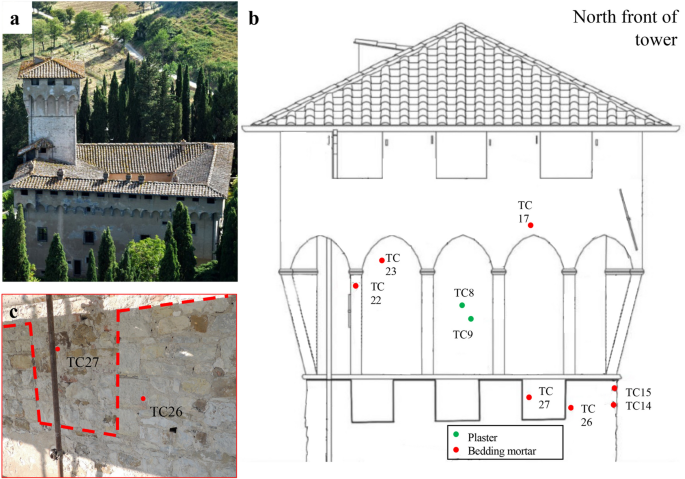
Trebbio Castle: building ( a ); sampling on the North side, perspective drawing (by Teresa Salvatici and Sara Calandra) ( b ), and masonry ( c ).
The building was investigated through the building archaeological approach, which identifies the stratigraphic units of the building, and then associated with written or other sources, allowing the formulation of hypotheses about the construction phases of the masonry 59 .
Based on this methodology, four main construction phases from the thirteenth century to the first decades of the seventeenth century, were identified:
Phase 1 (before 14th cent.): the presence of a square tower is documented. The tower was partially rebuilt and its original structure is only visible in the lower part.
Phase 2 (14th cent.): addition of storeys to the pre-existing tower with improved masonry and crenellated walls, characterized by different building construction techniques than the previous phase.
Phase 3 (1420–1433): addition of new storeys to the tower and complete renovation of the upper structure, including corbels and wider walkways, attributed to Michelozzo (as reported written source in 60 ).
Phase 4 (post 1433): modern and contemporary restorations, including mortar sealing, reconstruction and rectification of structural problems to restore a late medieval appearance. Some restoration works were performed following the numerous historical earthquakes that affected the Mugello area 58 .
The chronology of the building phases is the result of a combination of stratigraphic studies, written sources and the chronotypological abacus of Mugello masonries 58 .
The archaeological reading of the masonry and the resulting hypotheses about the construction phases formed the basis for the selection of the mortar sampling points. A total of 27 bedding mortar and plaster samples were collected using a hammer and chisel ( Supplementary Table 1 ).
The comprehensive minero-petrographic characterization was performed on all mortar samples ( Supplementary Table 2 ).
Mineralogical composition analysis of bulk mortars by XRPD revealed the presence of calcite, quartz, feldspar (plagioclase and k-feldspar), lizardite and micas. Calcite can be referred to the binder, lime lumps or fragments of aggregate; quartz, feldspars, lizardite and micas can be related to the aggregate. Gypsum was recorded only in TC19 and 24, probably due to the sulphation phenomena of the binder 34 , Supplementary Fig. 1 .
From the petrographic observation, these mortars are made of natural hydraulic binder, obtained by firing of marly limestone (Alberese limestone, Monte Morello Formation), diffusely employed in Florentine area 61 . The aggregate exhibits a heterogeneous composition, utilizing sandy sediments from local watercourses. Finer sands (< 400 µm) predominantly consist of single crystals of quartz, feldspars, spathic calcite, while coarser fractions contain fragments of arenaceous rocks, serpentinites, and Alberese limestone. Rare fragments of cocciopesto were also found.
Since the raw materials used are the same and the production technologies are similar, no minero-petrographic criteria were identified to differentiate samples belonging to different construction stages. Within the same construction stages, different characteristics are found in the mix-design (i.e., B/A, grain size distribution).
For radiocarbon application we focused on the binder aspect, aggregate composition and the presence of lump. So, the binder in the plaster samples (TC1-11, Supplementary Fig. 2a, b ) has undergone some chemical alteration due to the dissolution and slow recrystallization of calcite by the circulating moisture in the masonry. This process can develop in specific areas of the samples, e.g. in pores and along fractures (referred to as secondary calcite) or change the entire texture of the binder (referred to as partially binder recrystallization). This prevented us from selecting these samples for dating, causing an apparent rejuvenation of the sample 62 .
As for the bedding mortars, the binder is better preserved. Care in the preparation of the mixtures is evident, considering careful selection of aggregates and a consistently high binder content. However, even these samples exhibit characteristics that allow the selection of only certain samples for dating: samples superficially collected, those with non-homogeneous carbonation processes (heterogeneous texture ranges from microsparitic to sparitic in TC12, 13, 14, 15, 16, 18, 22, 24, 25, Supplementary Fig. 2c, d ), or binder recrystallization (as in TC12, 13, 14, 15, 16, 17, 18, 19, 21, 22, 24, 25), those showing gypsum in XRPD (as in TC19, 24), and those with almost exclusively carbonate aggregate (TC20, 23; Supplementary Fig. 2e ) have been excluded. Heterogeneous texture can be due to delayed carbonation processes or binder dissolution-recrystallization 63 , in fact, in most samples the two features are combined.
Within bedding mortars, we focused on two samples that could provide key insights into the historical attribution of construction phases and with mineralogical-petrographic characteristics which are more suitable for dating. These samples come from the crenellated masonry, TC26, and the infill masonry, TC27 (see in Fig. 2 c). The samples show complete carbonation through phenolphthalein test. On initial macroscopic examination, sample TC26 appears to have a compact mortar with few fractures and a hazel coloration. Millimetre-sized lumps of varying coloration, from white to yellowish, are visible. The mortar sample TC27 is compact and has a hazel color. Millimetre-sized lumps of yellowish to white hues can also be observed.
The main mineralogical and petrographic characteristics of these two samples are listed in Table 1 .
Petrographic observations of TC26 and TC27 reveal the presence of a binder with homogeneous structure and micritic texture with small dark inclusions. Lumps are present, referring to both unmixed binder and unburned limestone.
The aggregate exhibits a heterogeneous composition and bimodal grain size distribution, consisting of abundant carbonate rock fragments (limestone and calcarenites), sandstone, serpentinite and crystal of quartz, feldspars, and calcite ( Supplementary Fig. 3 ). TC26 and TC27 differ in: the B/A, 1/3 and 1/2, respectively, and for the coarser aggregate grain size in sample TC27 (0.7–1 mm).
SEM–EDS analyses on the binder showed significant variability in SiO 2 and CaO content, along with the presence of different types of lumps, confirming the use of Alberese limestone. A comprehensive study of lumps, combining OM, OM-CL, and SEM–EDS analyses in the same area, revealed that also the texture of lumps is heterogeneous (Fig. 3 ). They exhibit a similar texture to the binder in OM, appearing brick-red in CL, and SEM analysis indicates a CaO and SiO 2 + Al 2 O 3 + Fe 2 O 3 composition comparable to that of the binder. SEM–EDS analysis of the thin sections indicates that only small amounts of Mg are present (less than 1.8%). To gain further insight into the binder composition, SEM–EDS microanalyses were carried out on polished thin sections of both binder and lime lumps. The micro-chemical composition of lime lumps and binder is reported in Table 3 . In addition, the hydraulicity index (HI) was calculated using Boynton's formula 35 (Table 2 ). TC26 exhibits an HI of 0.16 ± 0.05, TC27 shows an HI of 0.20 ± 0.08, classifying as weakly hydraulic.
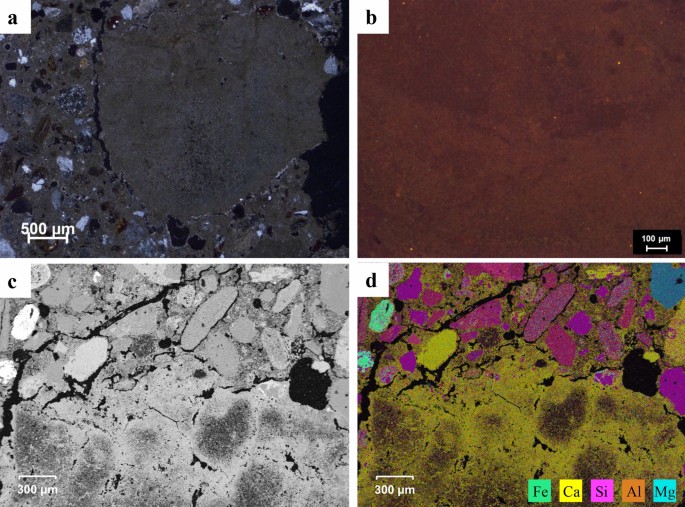
OM ( a ), OM-CL ( b ), SEM–EDS ( c , d ) analyses on lime lump. In ( c ), BS image of a detail of the lump. In ( d ), SEM–EDS map layered on the previous area.
HI results are compared with TGA analyses performed on 3 portions of binder-rich mortar per sample.
The hydraulic water (%) originating from the hydraulic components varies between 7.02% and 8.89%, while the CO 2 decomposition from air lime binder is between 27.0% and 31.9%. The results of SEM–EDS are in agreement with those of TGA and show that the mortars have slightly hydraulic behavior ( Supplementary Fig. 4 ).
Being carbonate aggregates abundant, to avoid possible contamination, we decided to focus on lumps for dating. Four lumps were selected for TC26 samples (labelled as TC26L1, L2, L3, and L4), and four for samples TC27 (labelled as TC27L1, L2, L3 and L4) (Fig. 4 a).
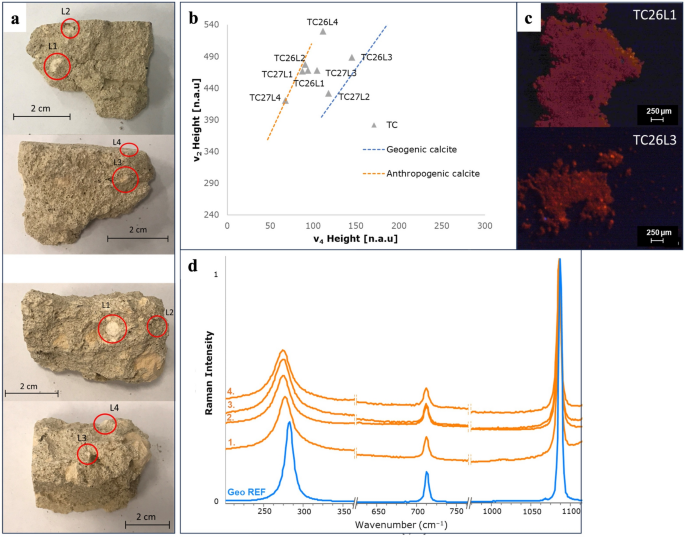
Results of non-destructive techniques on powders ( b - d ) and lump selection points ( a ). Plot of ν 2 and ν 4 with typical trend lines of geogenic and anthropogenic calcites obtained by ATR-FTIR and TC lump samples ( b ); OM-CL photomicrographs of lump powders: an anthropogenic sample (TC26L1) and a geogenic sample (TC26L3) ( c ); comparison among individual Raman spectra of carbonate samples: geogenic calcite (in blue, a reference sample) and anthropogenic calcite of TC samples (1: TC26L1; 2: TC26L2; 3: TC26L4; 4: TC27L1) ( d ).
XRPD analyses were conducted on the powdered lump samples after sieving to determine mineralogical composition (Table 3 ); OM-CL, ATR-FTIR and micro-Raman analyses (Fig. 4 b–d) were performed to assess the origin of the calcite.
XRPD analysis on lumps showed that the primary component is calcite, as expected.
TC26L1, TC26L2, TC26L4, TC27L1 and TC27L4 exhibit red-brown luminescence, which is consistent with their position on the anthropogenic calcite trend in ATR-FTIR, classifying them as pyrogenic carbonate. However, TC26L3, TC27L2, and TC27L3 exhibit orange CL and geogenic trends in ATR-FTIR, confirming that these lumps consist of geogenic calcite. ATR-FTIR detected a broad band centered at 1080 cm –1 , attributable to ν as (Si–O–Si) and ascribed to amorphous silicates 64 , likely originating from the calcination of stone rich in silicate components (e.g., clay minerals) ( Supplementary Fig. 4 ).
Micro-Raman analyses, conducted on TC26L1, TC26L2, TC26L4, TC27L1, TC27L4, show a Raman shift of L and v 1 bands toward lower wavenumber, along with their higher FWHM values, which is also observed for the v 4 band (Table 3 ). The micro-Raman results definitively confirm the data collected by other techniques. The observed values are typical of anthropogenic calcite.
Based on the result of the characterization process discussed in the previous sections, lumps TC26L1, TC26L2, TC26L4 and TC27L1 were chosen to be suitable for dating. The reaction times, along with the masses of the graphitized samples, are listed in Table 4 . The reaction time of 30 s was chosen since, having assessed the anthropogenic origin of the calcite, the risk of contaminants reacting with the acid and the sample mass were low. The AMS results are reported in Table 4 .
When samples belonging to the same fragment or construction phase have consistent radiocarbon concentration between each other, a weighted average can be calculated to obtain a more precise result. Indeed, the lumps TC26L1, TC26L2, and TC26L4 from the same mortar portion exhibit consistent radiocarbon concentrations. The results of the weighted average of the three radiocarbon concentrations and the corresponding conventional radiocarbon age are also reported in Table 4 .
The calibrated age for samples TC26L1 + TC26L2 + TC26L4 results from the measured conventional radiocarbon age (Fig. 5 ).
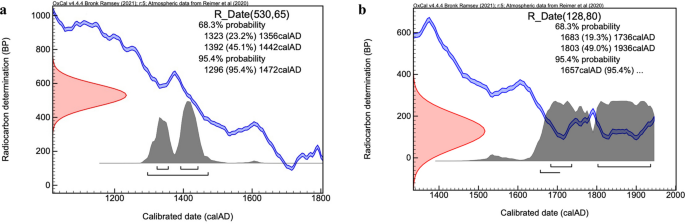
Calibrated age of the TC samples: TC26L1 + TC26L2 + TC26L4 ( a ); TC27L1 ( b ).
Although the calibrated age of the TC26 lump samples spans two of the phases identified in the archaeological analysis of the tower (phases 2 and 3), the characteristics of the masonry where the sample was taken suggest an interpretation of the 14 C results as more likely within the middle of the fourteenth century (phase 2).
The dating results for sample TC27L1 are presented in Table 4 . Given the conventional radiocarbon age measured (Fig. 5 ), sample TC27L1 is considered modern. A discrepancy can be observed between the assumed chronology and the measurement of the radiocarbon concentration. Based on the historical-archaeological hypothesis, it is assumed that the masonry dates from the middle of the fifteenth century (phase 3).
However, this discrepancy could be related to the extensive joint sealing of the upper part of the tower. The combination of the calibrated age and the historical information; allow us to formulate a specific interpretative hypothesis and attribute this operation to the restoration season following the seismic events that affected the Mugello area between the mid-15th and mid-seventeenth centuries 58 . After this intense and destructive earthquake period, intensive restoration and reconstruction activities were carried out on all the Medici properties in the area (e.g. Cafaggiolo, the Fortezza di San Martino, the town of Scarperia). Sample TC27, being associable to the modern phase, could have intercepted one of these activities.
The comparison between the radiocarbon dating and the archaeological results offered two new interpretations. On the one hand, the 14 C dating for sample TC26 yielded a range of dates that included the fourteenth and fifteenth centuries, while the chronotypology of the masonry of Mugello allows us to shift the focus to the first one. TC27, on the other hand, it was the 14 C dating that provided new interpretative approaches to the historical-archaeological data and made it possible to identify specific restoration interventions carried out in the modern period on a masonry that in the written source dates to the early fifteenth century 60 .
The proposed multistep procedure is demonstrated to be a successful approach for selecting a suitable mortar sample for radiocarbon dating. Multi-analytical approach (OM, SEM–EDS, XRPD, TGA) has been proven to permit the selection of the most suitable mortar to be dated. Non-destructive analyses (XRPD, OM-CL, ATR-FTIR and Micro-Raman) of selected specific mortar portions, such as binder-rich or lump samples (mechanical separation), enable the characterization of the calcite origin (differentiation between anthropogenic and geogenic calcite) with the perspective of reusing the samples for following analyses and dating.
The acidification line to extract CO 2 was coupled to the so-called Lilliput graphitization line, for dealing with small carbonate samples (2.5 mg lump and 5.0 mg bulk).
Our procedure has been successfully applied to single lumps collected from Florentine natural hydraulic mortars to date Trebbio Castle construction phases.
The new proposed multi-analytical procedure allowed us to discard most of the samples, identifying problems that could affect their correct dating (step II, III). Consequently, the preparation and the following AMS measurements (step IV) focused on just those micro-samples that had been found suitable as the result of characterization in step III, exploiting the same material. The results obtained from the comparison of the mortars with the historical-archaeological hypothesis provided relevant insights into the construction history of the building.
This paper aims at emphasizing that it is only through a well-structured analytical procedure that it is possible to select suitable samples and approach for the dating of traditional historical mortars.
Carbonation test
The phenolphthalein test (standardized by UNI EN 14630, 2007) is carried out using a 1% solution of phenolphthalein in ethyl alcohol. Applied to the surface of the freshly cut sample.
Optical microscope
The Axioscope A.1 Zeiss transmitted light polarizing optical microscope, connected to a digital video camera, allowed for the acquisition of sample images in thin sections, which were processed using AxioVision software. The acquired images were further analyzed to obtain information on the morphological and morphometric characteristics of the samples using the ImageJ program.
X-ray powder diffraction (XRPD)
The mineralogical composition was analyzed using a Philips X’Pert PRO X-ray powder diffractometer (XRPD) with a Cu anticathode (wavelength λ = 1.54 Å). The instrument operated at a current intensity of 30 mA and a voltage of 40 kV. The 2θ range explored was between 3 and 70° with a step size of 0.02° and a total time per pattern of 16 min 27 s. XRPD analyses were conducted on both powder bulk samples and specific lumps.
Scanning electron microscopy with energy-dispersive X-ray spectroscopy (SEM–EDS)
The ZEISS EVO MA 15 SEM–EDS with a tungsten filament and an energy-dispersive X-ray spectroscopy (EDS) analytical system, Oxford Ultimax 40 (with a resolution of 127 eV @5.9 keV and an area of 40 mm 2 ), was utilized for semi-quantitative microchemical and morphological analyses. These analyses were conducted on thin sections (prepared after carbon-metallized pretreatment) taken from both the binder and lumps areas, as well as from powder samples. The operational settings were as follows: an acceleration potential of 15 kV, a beam current of 500 pA, a working distance of 9–8.5 mm, a live time of 20 s to achieve an acquisition rate of at least 600,000 counts using Co standard, a process time of 4 for point analyses, and a pixel dwell time of 500 µs for map acquisition with a resolution of 1024 × 768 pixels. The microanalysis employed the Aztec 5.0 SP1 software, implementing the XPP matrix correction scheme. This process utilized purchased standard elements for calculations, enabling “standard-less” quantitative analysis. Constant analytical conditions, such as filament emission, were monitored through numerous analyses of a Co metallic standard.
Thermo-gravimetric analysis (TGA)
Thermogravimetric analyses (TGA) were carried out on historical mortar samples using a Perkin Elmer Pyris 6 system and Netzsch TG 209 F3 Tarsus. Fragments from each sample were mechanically broken down using a porcelain pestle, and the portion passing through a sieve with 63 µm openings (ISO R 565 Series) was selected as a binder-rich specimen. About 5 mg of the sample was used for TGA, and the analysis was conducted within the temperature range of 110–1000 °C. The samples were dried using silica gel as a desiccant at room temperature for a minimum of one week. The TGA experiments were performed in open alumina crucibles, with a heating rate of 10 °C min −1 , and a nitrogen gas flow of 30 mL min −1 .
Cathodoluminescence (OM-CL)
Optical microscope cathodoluminescence (OM-CL) analysis was conducted using the CL8100 MK5 model by Cambridge Image Technology Ltd., coupled with a Leica DM2700P polarization optical microscope. The microscope is equipped with a high-sensitivity 12 MP Leica Flexcam C1 camera and dedicated LAS X software, enabling the acquisition of digital images in various formats.
Fourier transform infrared spectroscopy (ATR-FTIR)
FTIR spectra were collected with a portable Bruker Optics ALPHA FT-IR Spectrometer equipped with SiC Globar source and a DTGS detector. The powdered samples were analyzed using a Platinum ATR single reflection diamond module collecting 24 scans, in the 4000–400 cm −1 spectral range, with a resolution of 4 cm −1 . The spectra were processed using OPUS 7.2 software (Bruker Optics GmbH, Ettlingen, Germany) and Spectragryph 1.2.15. Instrument was used in the laboratory of ISPC-CNR (Institute of Heritage Science in Sesto Fiorentino), Italy.
Micro-Raman spectroscopy
A Renishaw InVia Raman spectrometer, characterized by high resolution, was utilized in combination with a Leica DMLM microscope. The experiments involved employing a 785 nm excitation line, a 50 × long working distance objective (NA 0.5), a spectral resolution better than 1 cm −1 , and a theoretical laser spot diameter of 1.9 μm. The laser operated at a power of 80 mW, and each spectrum was acquired over a period of 5 s. Our focus was primarily on the low-to-medium region of the spectral range, specifically collecting data within the range of 100–1400 cm −1 .
Data availability
All data generated or analysed during this study are included in this published article [and its supplementary information files ].
Hajdas, I. et al. Radiocarbon dating. Nat. Rev. Methods Primers 1 (1), 62. https://doi.org/10.1038/s43586-021-00058-7 (2021).
Article CAS Google Scholar
Urbanová, P., Boaretto, E. & Artioli, G. The state-of-the-art of dating techniques applied to ancient mortars and binders: A review. Radiocarbon 62 (3), 503–525. https://doi.org/10.1017/RDC.2020.43 (2020).
Hendriks, L. et al. Selective dating of paint components: Radiocarbon dating of lead white pigment. Radiocarbon 61 (2), 473–493. https://doi.org/10.1017/RDC.2018.101 (2019).
Strunk, A., Olsen, J., Sanei, H., Rudra, A. & Larsen, N. K. Improving the reliability of bulk sediment radiocarbon dating. Quat. Sci. Rev. 242 , 106442. https://doi.org/10.1016/j.quascirev.2020.106442 (2020).
Article Google Scholar
Calandra, S. et al. Radiocarbon dating of straw fragments in the plasters of ST. Philip Church in archaeological site hierapolis of Phrygia (denizli, Turkey). Radiocarbon 65 (2), 323–334. https://doi.org/10.1017/RDC.2023.20 (2023).
Vasco, G. et al. Mortar characterization and radiocarbon dating as support for the restoration work of the Abbey of Santa Maria di Cerrate (Lecce, South Italy). Heritage 5 (4), 4161–4173. https://doi.org/10.3390/heritage5040215 (2022).
Al-Bashaireh, K. Plaster and mortar radiocarbon dating of Nabatean and Islamic structures, South Jordan. Archaeometry 55 (2), 329–354. https://doi.org/10.1111/j.1475-4754.2012.00677.x (2013).
Goedicke, C. Dating mortar by optically stimulated luminescence: A feasibility study. Geochronometria 38 (1), 42–49. https://doi.org/10.2478/s13386-011-0002-0 (2011).
Panzeri, L., Maspero, F., Galli, A., Sibilia, E. & Martini, M. Luminescence and radiocarbon dating of mortars at Milano-Bicocca laboratories. Radiocarbon 62 (3), 657–666. https://doi.org/10.1017/RDC.2020.6 (2020).
Urbanová, P. et al. The Late Antique suburban complex of Santa Giustina in Padua (North Italy): New datings and new interpretations of some architectural elements. Hortus Artium Medieval. 28 , 185–200. https://doi.org/10.1484/J.HAM.5.134911 (2022).
Folk, R. & Valastro, S. Successful technique for dating of lime mortar by carbon-14. J. Field Archaeol. 3 , 2 (1976).
Google Scholar
Van Strydonck, M., Dupas, M. & Dauchot-Dehon, M. Radiocarbon dating of old mortars. PACT J. 8 , 337–343 (1983).
Heinemeier, J. et al. AMS 14C dating of lime mortar. Nucl. Instrum. Methods Phys. B Beam Interact. Mater. 123 (1–4), 487–495 (1997).
Article ADS CAS Google Scholar
Michalska, D., Czernik, J. & Goslar, T. Methodological aspects of mortars dating (Poznań, Poland, MODIS). Radiocarbon 59 (6), 1891–1906. https://doi.org/10.1017/RDC.2017.128 (2017).
Hajdas, I. et al. Preparation and dating of mortar samples—Mortar Dating Intercomparison Study (MODIS). Radiocarbon 59 (6), 1845–1858. https://doi.org/10.1017/RDC.2017.112 (2017).
Artioli, G. et al. Characterization and selection of mortar samples for radiocarbon dating in the framework of the MODIS2 intercomparison: Two compared procedures. Radiocarbon 1–14 , 2024. https://doi.org/10.1017/RDC.2024.3 (2024).
Lichtenberger, A., Lindroos, A., Raja, R. & Heinemeier, J. Radiocarbon analysis of mortar from Roman and Byzantine water management installations in the Northwest Quarter of Jerash, Jordan. J. Archaeol. Sci. Rep. 2 , 114–127. https://doi.org/10.1016/j.jasrep.2015.01.001 (2015).
Fedi, M. et al. Towards micro-samples radiocarbon dating at INFN-LABEC, Florence. Nucl. Instrum. Methods Phys. Res. B Beams Interact. Mater. At. 465 , 19–23. https://doi.org/10.1016/j.nimb.2019.12.020 (2020).
Cantisani, E. et al. The mortars of Giotto’s Bell Tower (Florence, Italy): Raw materials and technologies. Constr. Build. Mater. 267 , 120801. https://doi.org/10.1016/j.conbuildmat.2020.120801 (2021).
Heinemeier, J., Ringbom, Å., Lindroos, A. & Sveinbjörnsdóttir, Á. E. Successful AMS 14C dating of non-hydraulic lime mortars from the medieval churches of the Åland Islands, Finland. Radiocarbon 52 (1), 171–204. https://doi.org/10.1017/S0033822200045124 (2010).
Ringbom, Å., Lindroos, A., Heinemeier, J. & Sonck-Koota, P. 19 years of mortar dating: Learning from experience. Radiocarbon 56 (2), 619–635. https://doi.org/10.2458/56.17469 (2014).
Gliozzo, E., Pizzo, A. & La Russa, M. F. Mortars, plasters and pigments—research questions and sampling criteria. Archaeol. Anthropol. Sci. 13 (11), 193. https://doi.org/10.1007/s12520-021-01393-2 (2021).
Boaretto, E. Dating materials in good archaeological contexts: The next challenge for radiocarbon analysis. Radiocarbon 51 (1), 275–281. https://doi.org/10.1017/S0033822200033804 (2009).
Pesce, G. L., Ball, R. J., Quarta, G. & Calcagnile, L. Identification, extraction, and preparation of reliable lime samples for 14C dating of plasters and mortars with the “pure lime lumps” technique. Radiocarbon 54 (3–4), 933–942. https://doi.org/10.1017/S0033822200047573 (2012).
Dilaria, S. et al. Phasing the history of ancient buildings through PCA on Mortars’ Mineralogical Profiles: The example of the Sarno Baths (Pompeii). Archaeometry 1–17 , 2020. https://doi.org/10.1111/arcm.12746 (2020).
Miriello, D. et al. Characterisation of archaeological mortars from Pompeii (Campania, Italy) and identification of construction phases by compositional data analysis. J. Archaeol. Sci. 37 (9), 2207–2223. https://doi.org/10.1016/j.jas.2010.03.019 (2010).
Cantisani, E., Fratini, F. & Pecchioni, E. Optical and electronic microscope for minero-petrographic and microchemical studies of lime binders of ancient mortars. Minerals 12 (1), 41. https://doi.org/10.3390/min12010041 (2021).
Artioli, G., Secco, M., & Addis, A. The Vitruvian legacy: Mortars and binders before and after the Roman world. The Contribution of Mineralogy to Cultural Heritage, Gilberto Artioli, Roberta Oberti. https://doi.org/10.1180/EMU-notes.20.4 (2019).
Arizzi, A. & Cultrone, G. Mortars and plasters—how to characterise hydraulic mortars. Archaeol. Anthropol. Sci. 13 (9), 144. https://doi.org/10.1007/s12520-021-01404-2 (2021).
Pesce, G. The need for a new approach to the radiocarbon dating of historic mortars. Radiocarbon 65 (5), 1017–1021. https://doi.org/10.1017/RDC.2023.92 (2023).
Calandra, S., Salvatici, T., Centauro, I., Cantisani, E. & Garzonio, C. A. The mortars of florence riverbanks: Raw materials and technologies of lungarni historical masonry. Appl. Sci. 12 (10), 5200. https://doi.org/10.3390/app12105200 (2022).
Miyata, S. Anion-exchange properties of hydrotalcite-like compounds. Clays Clay Miner. 31 , 305–311. https://doi.org/10.1346/CCMN.1983.0310409 (1983).
Ponce-Antón, G., Ortega, L. A., Zuluaga, M. C., Alonso-Olazabal, A. & Solaun, J. L. Hydrotalcite and hydrocalumite in mortar binders from the medieval castle of portilla (Álava, north Spain): Accurate mineralogical control to achieve more reliable chronological ages. Minerals 8 (8), 326. https://doi.org/10.3390/min8080326 (2018).
Sabbioni, C. et al. Atmospheric deterioration of ancient and modern hydraulic mortars. Atmos. Environ. 35 (3), 539–548. https://doi.org/10.1016/S1352-2310(00)00310-1 (2001).
Boynton, R. S. Chemistry and Technology of Lime and Limestone 2nd edn. (Wiley, 1980).
Bakolas, A. et al. Thermoanalytical research on traditional mortars in Venice. Thermochim. Acta 269 , 817–828. https://doi.org/10.1016/0040-6031(95)02574-X (1995).
Moropoulou, A., Bakolas, A. & Bisbikou, K. Characterization of ancient, Byzantine and later historic mortars by thermal and X-ray diffraction techniques. Thermochim. Acta 269 , 779–795. https://doi.org/10.1016/0040-6031(95)02571-5 (1995).
Riccardi, M. P., Lezzerini, M., Car, F., Franzini, M. & Messiga, B. Microtextural and microchemical studies of hydraulic ancient mortars: Two analytical approaches to understand pre-industrial technology processes. J. Cult. Herit. 8 , 350–360. https://doi.org/10.1016/j.culher.2007.04.005 (2007).
Marshall, J. D. Cathodoluminescence of geological materials by DJ Marshall, Unwin Hyman, 1988. https://doi.org/10.1002/gj.3350260409 (1991).
Ricci, G. et al. Integrated multi-analytical screening approach for reliable radiocarbon dating of ancient mortars. Sci. Rep. 12 (1), 3339. https://doi.org/10.1038/s41598-022-07406-x (2022).
Article ADS CAS PubMed PubMed Central Google Scholar
Toffolo, M. B., Ricci, G., Chapoulie, R., Caneve, L. & Kaplan-Ashiri, I. Cathodoluminescence and laser-induced fluorescence of calcium carbonate: A review of screening methods for radiocarbon dating of ancient lime mortars. Radiocarbon 62 (3), 545–564. https://doi.org/10.1017/RDC.2020.21 (2020).
Lindroos, A., Heinemeier, J., Ringbom, Å., Braskén, M. & Sveinbjörnsdóttir, Á. Mortar dating using AMS 14C and sequential dissolution: Examples from medieval, non-hydraulic lime mortars from the Åland Islands, SW Finland. Radiocarbon 49 (1), 47–67. https://doi.org/10.1017/S0033822200041898 (2007).
Murakami, T., Hodgins, G. & Simon, A. W. Characterization of lime carbonates in plasters from Teotihuacan, Mexico: Preliminary results of cathodoluminescence and carbon isotope analyses. J. Archaeol. Sci. 40 (2), 960–970. https://doi.org/10.1016/j.jas.2012.08.045 (2013).
Chu, V., Regev, L., Weiner, S. & Boaretto, E. Differentiating between anthropogenic calcite in plaster, ash and natural calcite using infrared spectroscopy: Implications in archaeology. J. Archaeol. Sci. 35 (4), 905–911. https://doi.org/10.1016/j.jas.2007.06.024 (2008).
Regev, L., Poduska, K. M., Addadi, L., Weiner, S. & Boaretto, E. Distinguishing between calcites formed by different mechanisms using infrared 236 spectrometry: Archaeological applications. J. Archaeol. Sci. 37 (12), 3022–3029. https://doi.org/10.1016/j.jas.2010.06.027 (2010).
Toffolo, M. B., Regev, L., Dubernet, S., Lefrais, Y. & Boaretto, E. FTIR-based crystallinity assessment of aragonite–calcite mixtures in archaeological lime binders altered by diagenesis. Minerals 9 (2), 121. https://doi.org/10.3390/min9020121 (2019).
Calandra, S. et al. Evaluation of ATR-FTIR spectroscopy for distinguishing anthropogenic and geogenic calcite. J. Phys. Conf. Ser. 2204 (1), 012048. https://doi.org/10.1088/1742-6596/2204/1/012048 (2022).
Surovell, T. A. & Stiner, M. C. Standardizing infrared measures of bone mineral crystallinity: An experimental approach. J. Archaeol. Sci. 28 (6), 633–642. https://doi.org/10.1006/jasc.2000.0633 (2001).
Seymour, L. M., Keenan-Jones, D., Zanzi, G. L., Weaver, J. C. & Masic, A. Reactive ceramic aggregates in mortars from ancient water infrastructure serving Rome and Pompeii. Cell Rep. Phys. Sci. 3 , 9. https://doi.org/10.1016/j.xcrp.2022.101024 (2022).
Seymour, L. M. et al. Hot mixing: Mechanistic insights into the durability of ancient Roman concrete. Sci. Adv. 9 (1), eadd1602. https://doi.org/10.1126/sciadv.add1602 (2023).
Article PubMed PubMed Central Google Scholar
Bischoff, W. D., Sharma, S. K. & MacKenzie, F. T. Carbonate ion disorder in synthetic and biogenic magnesian calcites: A Raman spectral study. Am. Min. 70 (5–6), 581–589 (1985).
CAS Google Scholar
Borromeo, L. et al. Raman spectroscopy as a tool for magnesium estimation in Mg- calcite. J. Raman Spectrosc. 48 (7), 983–992. https://doi.org/10.1002/jrs.5156 (2017).
Zolotoyabko, E. et al. Differences between bond lengths in biogenic and geological calcite. Cryst. Growth Des. 10 (3), 1207–1214. https://doi.org/10.1021/cg901195t (2010).
Wehrmeister, U. et al. Amorphous, nanocrystalline and crystalline calcium carbonates in biological materials. J. Raman Spectrosc. 42 (5), 926–935. https://doi.org/10.1002/jrs.2835 (2011).
Calandra, S., Conti, C., Centauro, I. & Cantisani, E. Non-destructive distinction between geogenic and anthropogenic calcite by Raman spectroscopy combined with machine learning workflow. Analyst https://doi.org/10.1039/D3AN00441D (2023).
Article PubMed Google Scholar
Toffolo, M. B. et al. Crystallinity assessment of anthropogenic calcites using Raman micro-spectroscopy. Sci. Rep. 13 , 12971. https://doi.org/10.1038/s41598-023-39842-8 (2023).
Fedi, M. E., Cartocci, A., Manetti, M., Taccetti, F. & Mandò, P. A. The 14C AMS facility at LABEC. Florence. Nucl. Instrum. Methods Phys. Res. B Beam Interact. Mater. At. 259 (1), 18–22. https://doi.org/10.1016/j.nimb.2007.01.140 (2007).
Arrighetti, A. Materials and building techniques in Mugello from the Late Middle Ages to the Early Modern Age; Materiali e tecniche costruttive del Mugello tra basso Medioevo e prima Età Moderna . https://doi.org/10.3989/arq.arqt.2016.001 (2017).
Brogiolo G. P. & Cagnana A. Archeologia dell’architettura. Metodi e interpretazioni (ed. All’insegna del Giglio) (2012).
Vasari, G. Le vite de' più eccellenti architetti, pittori, et scultori italiani, da Cimabue insino a' tempi nostri. Nell'edizione per i tipi di Lorenzo Torrentino, Firenze 1550 (ed. Einaudi) (2015).
Fratini, F., Cantisani, E., Pecchioni, E., Pandeli, E. & Vettori, S. Pietra Alberese: Building material and stone for lime in the Florentine Territory (Tuscany, Italy). Heritage 3 (4), 1520–1538. https://doi.org/10.3390/heritage3040084 (2020).
Pavía, S. Repair mortars for masonry bridges. In Bridge and Infrastructure in Ireland, Proc. 3rdSymp, Dublin (2006).
Pecchioni, E., Fratini, F. & Cantisani, E. Atlas of the Ancient Mortars in Thin Section under Optical Microscop 2nd edn. (Nardini, 2020).
Ellerbrock, R., Stein, M. & Schaller, J. Comparing amorphous silica, short-range-ordered silicates and silicic acid species by FTIR. Sci. Rep. 12 , 11708. https://doi.org/10.1038/s41598-022-15882-4 (2022).
Download references
Acknowledgements
The authors would like to thank Arch. Lucrezia Cuniglio, for the support and the collaboration for the collection of samples. Additionally, appreciation is extended to Laura Chiarantini and Tiziano Catalani for their technical assistance in the SEM-EDS analysis, as well as to Silvia Danise and Elena Pecchioni for facilitating access to OM-CL instrumentation.
Author information
Authors and affiliations.
Department of Earth Sciences, University of Florence, 50121, Florence, Italy
Sara Calandra, Teresa Salvatici & Carlo Alberto Garzonio
Institute of Heritage Science, National Research Council of Italy, 50019, Sesto Fiorentino (Florence), Italy
Emma Cantisani, Barbara Salvadori & Fabio Fratini
Institute of Heritage Science, National Research Council of Italy, 20125, Milan, Italy
Claudia Conti
National Institute for Nuclear Physics, Unit of Florence, 50019, Sesto Fiorentino (Florence), Italy
Serena Barone, Lucia Liccioli & Mariaelena Fedi
Department of Physics and Astronomy, University of Florence, 50019, Sesto Fiorentino (Florence), Italy
Serena Barone
Department of Historical Science and Cultural Heritage, University of Siena, 53100, Siena, Italy
Andrea Arrighetti
You can also search for this author in PubMed Google Scholar
Contributions
S.C., E.C., C.C., M.F. designed the research; E.C., M.F., C.A.G. supervised the research project; F.F., A.A., T.S. archaeological and architectural research; F.F, E.C. collected and prepared the samples; S.C., E.C. OM, XRPD, SEM–EDS characterizations; S.C., C.C. Micro-Raman measurements; S.C., B.S. FTIR measurements; S.B., L.L., S.C. micro-sample preparation; M.F., S.B., L.L. AMS measurements and data analysis; S.C., E.C., M.F., S.B., L.L., A.A., F.F. archaeometric interpretation of results. All authors collaborated to the writing of the manuscript.
Corresponding author
Correspondence to Sara Calandra .
Ethics declarations
Competing interests.
The authors declare no competing interests.
Additional information
Publisher's note.
Springer Nature remains neutral with regard to jurisdictional claims in published maps and institutional affiliations.
Supplementary Information
Supplementary information 1., rights and permissions.
Open Access This article is licensed under a Creative Commons Attribution-NonCommercial-NoDerivatives 4.0 International License, which permits any non-commercial use, sharing, distribution and reproduction in any medium or format, as long as you give appropriate credit to the original author(s) and the source, provide a link to the Creative Commons licence, and indicate if you modified the licensed material. You do not have permission under this licence to share adapted material derived from this article or parts of it. The images or other third party material in this article are included in the article’s Creative Commons licence, unless indicated otherwise in a credit line to the material. If material is not included in the article’s Creative Commons licence and your intended use is not permitted by statutory regulation or exceeds the permitted use, you will need to obtain permission directly from the copyright holder. To view a copy of this licence, visit http://creativecommons.org/licenses/by-nc-nd/4.0/ .
Reprints and permissions
About this article
Cite this article.
Calandra, S., Cantisani, E., Conti, C. et al. A new multi-analytical procedure for radiocarbon dating of historical mortars. Sci Rep 14 , 19979 (2024). https://doi.org/10.1038/s41598-024-70763-2
Download citation
Received : 18 March 2024
Accepted : 21 August 2024
Published : 28 August 2024
DOI : https://doi.org/10.1038/s41598-024-70763-2
Share this article
Anyone you share the following link with will be able to read this content:
Sorry, a shareable link is not currently available for this article.
Provided by the Springer Nature SharedIt content-sharing initiative
- Historical mortars
- Radiocarbon dating
- Geogenic and anthropogenic calcites
- Microsample preparation
By submitting a comment you agree to abide by our Terms and Community Guidelines . If you find something abusive or that does not comply with our terms or guidelines please flag it as inappropriate.
Quick links
- Explore articles by subject
- Guide to authors
- Editorial policies
Sign up for the Nature Briefing newsletter — what matters in science, free to your inbox daily.

- Open access
- Published: 31 August 2024
The links between symptom burden, illness perception, psychological resilience, social support, coping modes, and cancer-related worry in Chinese early-stage lung cancer patients after surgery: a cross-sectional study
- Yingzi Yang ORCID: orcid.org/0000-0003-4242-0444 1 , 2 na1 ,
- Xiaolan Qian 1 na1 ,
- Xuefeng Tang 1 ,
- Chen Shen 2 ,
- Yujing Zhou 3 ,
- Xiaoting Pan 2 &
- Yumei Li 4
BMC Psychology volume 12 , Article number: 463 ( 2024 ) Cite this article
1 Altmetric
Metrics details
This study aims to investigate the links between the clinical, demographic, and psychosocial factors and cancer-related worry in patients with early-stage lung cancer after surgery.
The study utilized a descriptive cross-sectional design. Questionnaires, including assessments of cancer-related worry, symptom burden, illness perception, psychological resilience, coping modes, social support and participant characteristics, were distributed to 302 individuals in early-stage lung cancer patients after surgery. The data collection period spanned from January and October 2023. Analytical procedures encompassed descriptive statistics, independent Wilcoxon Rank Sum test, Kruskal-Wallis- H - test, Spearman correlation analysis, and hierarchical multiple regression.
After surgery, 89.07% had cancer-related worries, with a median (interquartile range, IQR) CRW score of 380.00 (130.00, 720.00). The most frequently cited concern was the cancer itself (80.46%), while sexual issues were the least worrisome (44.37%). Regression analyses controlling for demographic variables showed that higher levels of cancer-related worry (CRW) were associated with increased symptom burden, illness perceptions, and acceptance-rejection coping modes, whereas they had lower levels of psychological resilience, social support and confrontation coping modes, and were more willing to obtain information about the disease from the Internet or applications. Among these factors, the greatest explanatory power in the regression was observed for symptom burden, illness perceptions, social support, and sources of illness information (from the Internet or applications), which collectively explained 52.00% of the variance.
Conclusions
Healthcare providers should be aware that worry is a common issue for early stage lung cancer survivors with a favorable prognosis. During post-operative recovery, physicians should identify patient concerns and address unmet needs to improve patients’ emotional state and quality of life through psychological support and disease education.
Peer Review reports
Introduction
Lung cancer is a common malignant neoplasm that often causes considerable psychological distress to patients and their families [ 1 ]. Due to the increasing public awareness of health screening in recent years and the use and promotion of low-dose computed tomography (CT) screening for early screening and detection of lung cancer, the incidence of early-stage lung cancer has been increasing [ 2 ]. According to the clinical diagnostic criteria for lung cancer, early-stage non-small cell lung cancer refers to a tumor that is confined to the lung and has not metastasized to distant organs or lymph nodes, generally referring to stage I and II [ 3 , 4 ]. For individuals diagnosed with early-stage non-small cell lung cancer, radical surgical resection offers the most beneficial treatment option for extended survival [ 5 , 6 ]. It can be said that after diagnosis and active treatment of early-stage lung cancer patients, the recurrence rate 5 years after surgery is low. Although the survival rate after radical resection has improved, a decline in lung function is inevitable. According to studies [ 7 , 8 ], lung function experienced a steep decline at 1 month after lung resection, partially recovered at 3 months, and stabilized at 6 months after surgery. Patients who have undergone surgery for lung cancer often experience post-treatment symptoms, including pain, dyspnea, and fatigue, which negatively affect their quality of life [ 9 ]. A previous study conducted by our team [ 10 ] found that post-operative lung cancer patients had various unmet needs during their recovery, including physiological, safety, family and social support, and disease information. The psychological distress experienced by patients, including worry, anxiety, and fear, increases due to unmet needs after cancer treatments [ 11 , 12 ].
In recent years, there has been an increasing focus on Cancer-related worry (CRW) as a form of psychological distress experienced by cancer patients. CRW refers to the uncertainty of cancer patients’ future after cancer diagnosis. It encompasses areas of common concern to cancer patients, such as cancer itself, disability, family, work, economic status, loss of independence, physical pain, psychological pain, medical uncertainty, and death. The purpose of CRW is to reflect the unmet needs or concerns of cancer patients [ 13 , 14 , 15 ]. Unlike anxiety, worry primarily reflects the patient’s repetitive thoughts about the uncertainty of the future [ 13 ]. It is also a cognitive manifestation of the uncertainty of disease prognosis [ 16 ].
Currently, measurement scales such as the State Train Anxiety Inventory (STAI) and the Hospital Anxiety and Depression Scale (HADS) are frequently used to evaluate physical symptoms caused by autonomic nervous activity in patients. However, they do not assess patients’ concerns or anxiety content [ 17 ]. Some scholars [ 13 , 17 , 18 ] have developed tools to measure the degree and content of cancer-related worries. The CRW questionnaire is a tool for measuring and evaluating the anxiety status of patients. It can also detect their needs or preferences through convenient means, allowing for the design of personalized care [ 13 ]. These questionnaires were primarily utilized to assess the level and nature of worry among cancer patients diagnosed with breast, prostate, skin, and adolescent cancers [ 19 , 20 , 21 , 22 ], However, it has not been employed in the post-operative population for early-stage lung cancer.
Theory framework
Mishel’s Uncertainty in Illness Theory [ 23 ] defines illness uncertainty as a cognitive state that arises when individuals lack sufficient information to effectively construct or categorize disease-related events. The theory explains how patients interpret the uncertainty of treatment processes and outcomes through a cognitive framework. It consists of three main components (see Fig. 1 ): (a) antecedents of uncertainty, (b) appraisal of uncertainty, and (c) coping with uncertainty. The antecedents of uncertainty include the stimulus frame (such as symptom burden), cognitive capacity (like disease perception), and structure providers (including social support and sources of disease information). Managing uncertainty requires coping modes such as emotional regulation and proactive problem-solving. Studies [ 24 ] have shown that there is a correlation between the way patients manage their emotions and the coping modes they experience when faced with difficulties. Patients with positive emotional coping modes are less likely to worry, while patients with avoidance coping modes are more likely to experience emotional distress. Furthermore, previous research [ 25 ] has shown a strong correlation between psychological resilience and cancer-related worries. Psychological resilience reflected an individual’s ability to adapt and cope with stress or adversity, and was an important indicator of a patient’s psychological traits [ 26 ]. Furthermore, it had a significant impact on their mental state and quality of life. Specifically, higher levels of cancer worries have been linked to lower levels of psychological resilience.
Based on the theoretical framework and literature research presented, it is hypothesized that factors such as psychological resilience, antecedents of disease uncertainty (symptom burden, disease perception, social support and sources of disease information), and coping modes will correlate with the level of cancer-related worries in postoperative patients with early-stage lung cancer. Therefore, the aim of this study was to investigate the potential correlates of cancer-related worry in early-stage lung cancer patients after surgery. This analysis will aid medical professionals in comprehending the worry state and unmet needs of early-stage lung cancer patients after surgery, and in tailoring rehabilitation programs and psychological interventions accordingly.
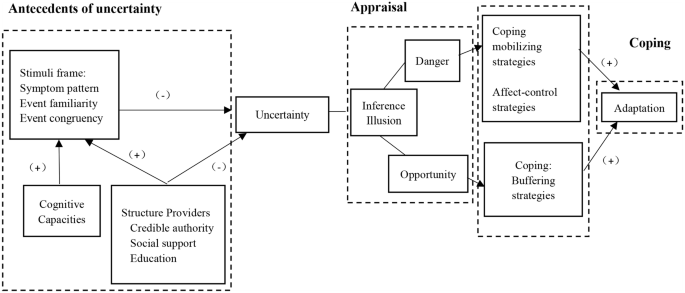
Mishel’s uncertainty in Illness theory framework
Study design
The study was cross-sectional in design. The Stimulating the Reporting of Observational Studies in Epidemiology (STROBE) checklist was completed (see S1 STROBE Checklist).
Setting and participants
This study included patients who underwent surgical treatment for lung cancer at a general hospital in Shanghai between January and October 2023. The study inclusion criteria were: (1) age over 18 years; (2) clinical diagnosis of early-stage primary non-small cell lung cancer (NSCLC), Tumor node metastasis classification (TNM) I to II [ 27 , 28 ], and received video-assisted thoracic surgery; (3) recovery time after surgery was within 1 month. Exclusion criteria were: (1) Postoperative radiotherapy and chemotherapy or second surgery may be required. (2) If other malignant tumors are present, they may also need to be treated. (3) Patients with severe psychological or mental disorders may not be candidates for this survey. (4) Patients with speech communication difficulties or hearing and visual impairments may require additional accommodations.
Sample size was calculated using G*Power software version 3.1.9 [ 29 ]. Calculate power analysis using F-test and linear multiple regression: fixed model, R2 increase as statistical test, and “A priori: Calculate required sample size - given alpha, power, and effect size” as type of power analysis. Cohen’s f2 = 0.15, medium effect size, α = 0.05, power (1-β) = 0.80, number of tested predictors = 8, total number of predictors = 10 as input parameters. The analysis showed that the minimum required sample size was 109 adults. Finally, the required sample size was determined to be over 120 adults with a probability of non-response rate of 10%.
Research team and data collection
The research team consisted of two nursing experts, four nursing researchers (one of whom had extensive experience in nursing psychology research), a group of clinical nurses, and several research assistants. To ensure the scientific quality and rigor of the research, the team was responsible for overseeing the quality of the project design and implementation process. Prior to conducting the formal survey, all research assistants received consistent training and evaluation to ensure consistent interpretation of questionnaire responses.
One month after surgery marks the first stage of the early recovery process for lung cancer patients and is a critical period for psychological adjustment [ 30 ]. Understanding a patient’s psychological state is critical to providing excellent medical care. During this period, patients typically need to visit the outpatient clinic for wound suture removal and dressing changes, further emphasizing the importance of postoperative recovery. Therefore, we chose this time frame for our research to gain a more comprehensive understanding of patients’ psychological well-being.
Prior to the start of the study, the hospital management and department head worked with us to provide comprehensive and standardized training to all participating investigators to ensure the reliability and consistency of the study. Research assistants rigorously screened patients based on predefined inclusion and exclusion criteria, which were designed to ensure a representative sample of lung cancer patients in the early recovery phase.
To obtain informed consent, patients were provided with detailed information and explanations and asked to complete the questionnaire voluntarily. To accommodate the different needs and preferences of patients, we used a combination of electronic and paper questionnaires. The electronic questionnaires were administered via the Questionnaire Star platform ( https://www.wjx.cn/ ), allowing patients to scan a two-dimensional (QR) code to access the survey. For those who preferred paper questionnaires, research assistants provided physical copies and assisted with completion as needed. The questionnaire was designed with consistent instructions to ensure that patients had a full understanding of the questions. Upon completion, each questionnaire was carefully reviewed and checked for accuracy. In addition, to reduce attrition, we obtained patients’ consent to keep their contact information, which allowed us to communicate with them further and collect additional data.
Ethical considerations
The study was approved by the hospital ethics committee. All participants gave informed consent prior to enrollment.
Sociodemographic questionnaire
We developed a sociodemographic survey based on a literature review and expert consultation. The survey includes patient demographic data such as age, sex, residence, lifestyle, education, marital status, childbearing history, religion, insurance, economic status (annual household income), smoking habits, employment status, sources of disease information, and previous psychological counseling. Clinical case information includes physical comorbidity, clinical tumor stage, and cancer type.
Cancer-related worry
The study measured participants’ cancer-related worry using the Brief Cancer-related Worry Inventory (BCWI), which was originally designed by Hirai et al. [ 13 ] to evaluate distinct concerns and anxiety levels among individuals with cancer. For this research, we used the 2019 Chinese edition of the BCWI, as introduced and updated by He et al. [ 31 ] (see Supplementary Table 2 ). The BCWI was comprised of 16 items, which were divided into three domains: (1) future prospects, (2) physical and symptomatic problems, and (3) social and interpersonal problems. Participants were asked to assess their cancer-related worries on a scale ranging from 0 to 100. Worry severity was determined by summing the scores for each item. The higher the total score, the more intense the patient’s cancer-related worry. The BCWI provided a concise evaluation of cancer-related worries in cancer patients. With only 16 items, it was able to differentiate them from symptoms of anxiety, depression, and post-traumatic stress disorder. In this study, the Cronbach’s alpha coefficient for this scale was 0.96.
Symptom burden
The M.D. Anderson Symptom Assessment Scale [ 32 ] was a widely used tool for evaluating symptom burden in cancer patients. The Chinese version of MDASI-C was translated and modified by researchers at the M.D. Anderson Cancer Center. The questionnaire included 13 multidimensional symptom items, such as pain, fatigue, nausea, restless sleep, distress, and shortness of breath, forgetfulness, loss of appetite, lethargy, dry mouth, sadness, vomiting, and numbness. Six additional items were used to evaluate the impact of these symptoms on work, mood, walking, relationships, and daily enjoyment. Patients rated the severity of their symptoms over the past 24 h on a scale of 0 (absent) to 10 (most severe), providing a comprehensive assessment of symptom burden across multiple dimensions. The Cronbach’s alpha coefficient for this study was 0.95.
Illness perception
The Brief Illness Perception Questionnaire (BIPQ) was used to measure participants’ illness perception. The scale, developed by Broadbent [ 33 ], was later revised by Mei [ 34 ] to include a Chinese version. It consisted of eight items divided into cognition, emotion, and comprehension domains as well as one open-ended question (What are the three most important factors in the development of lung cancer, in order of importance? ). The study utilized a 10-point Likert scale to rank items one through eight, with a range of 0–80 points. The ninth item required an open response. A higher total score indicated a greater tendency for individuals to experience negative perceptions and perceive symptoms of illness as more severe. In this study, the Cronbach’s alpha coefficient for this scale of eight scoring items was found to be 0.73.
Psychological resilience
The study measured participants’ psychological resilience using the 10-item Connor Davidson Resilience Scale (CD-RISC-10), originally developed by Connor and Davidson [ 35 ], and later revised by Campbell based on CD-RISC-25. The scale was designed to assess an individual’s level of emotional resilience in a passionate environment. It comprised 10 items, rated on a 5-point Likert scale (0 = never, 1 = rarely, 2 = sometimes, 3 = often, 4 = always). The total score ranged from 0 to 40 points, with higher scores indicating greater resilience. The study utilized the Chinese version of CD-RISC10, which was translated and revised by Ye et al. [ 36 ], to measure psychological resilience. The Cronbach’s alpha coefficient of the scale in this study was 0.96.
Coping modes
The study measured participants’ coping modes using the Medical Coping Modes Questionnaire (MCMQ), a specialized tool for measuring patient coping modes. The MCMQ was first designed by Feifel in 1987 [ 37 ] and was translated and revised into Chinese by Shen S and Jiang Q in 2000 [ 38 ]. It consisted of 20 items and three dimensions: confrontation (eight items), avoidance (seven items), and acceptance-resignation (five items). The study utilized a 4-point scoring system to evaluate coping events, with scores ranging from 1 to 4 based on the strength of each event. Eight items (1, 4, 9, 10, 12, 13, 18, and 19) were negatively scored, resulting in a total score range of 20 to 80 points. A higher score indicated a more frequent use of this coping mode. The three dimensions of this scale can be split into three scales for separate use. The reliability coefficients of the Confrontation Coping Mode Scale, Avoidance Coping Mode Scale, and Acceptance-resignation Coping Mode Scale were 0.69, 0.60, and 0.76.
Social Support
The study used the Perceived Social Support Scale (PSSS) developed by Zimet [ 39 ] to assess the level of self-understanding and perceived social support in postoperative lung cancer patients. The Chinese version of the PSSS, as adapted by Chou [ 40 ], showed satisfactory reliability and validity. The 12-item scale comprised of three dimensions: family, friend, and other support, and was rated using a 7-point Likert scale (1 = strongly disagree, 7 = strongly agree). The scale’s total score ranged from 12 to 84, with a higher score indicating a greater subjective sense of social support received by individuals. The Cronbach’s alpha coefficient for this scale in this study was 0.94.
Statistical analyses
The questionnaire was validated and double-checked before being input into Excel to ensure accuracy. Statistical analysis and processing of the questionnaire data were conducted using Statistical Product and Service Solutions (SPSS) 26.0 software. All statistical tests were two-sided with a significance level of α = 0.05. The quantitative data were presented using means and standard deviations, while the qualitative data were represented by frequency distributions. The data that conforms to normal distribution was analyzed using independent sample t-test for comparison between two groups and one-way ANOVA analysis for comparison between multiple groups. Non-normal distribution data were analyzed using non-parametric Wilcoxon rank sum test for comparison between two groups and Kruskal-Wallis - H -Test for comparison between multiple groups. Additionally, Spearman correlation analysis was used to study the correlations among CRW, MDASI-C, BIPQ, CD-RISC-10, MCMQ, and PSSS. A hierarchical linear regression analysis was performed to identify the multidimensional factors affecting CRW. All variables significantly correlated with the outcome variable ( p < 0.05) were included in the corresponding hierarchical regression analysis. Using Mishel’s Uncertainty in Illness Theory as a framework, a four-step model was adopted to study the factors influencing cancer-related concerns. The model includes individual factors such as sociodemographic and disease-related data, as well as psychological resilience, stimulus frame (symptom burden, illness perception, social support, and sources of disease information), and coping modes.
In this study, 307 questionnaires were distributed (227 electronic, 80 paper). Five paper questionnaires were invalid, resulting in 302 valid questionnaires and a recovery rate of 98.37%. The participants were 302 postoperative lung cancer patients, with 36.75% male and 63.25% female, aged 18–83 years (mean age 52.73, SD 13.07). Most patients (90.07%) were married. Additional demographic and clinical information is in Table 1 .
89.07% of people had cancer-related worries after surgery and median (interquartile range, IQR) score for CRW was 380.00 (130.00, 720.00) with a range of 0-1600. 86.42% reported worry about future prospects, 84.11% worry about physical and symptomatic problems, 79.80% worry about social and interpersonal problems. Among the 16 worry items of BCWI, the highest frequency of patient worries was “About cancer itself” (80.46%), followed by “About whether cancer might get worse in the future” (79.14%); the lowest frequency concern was “about sexual issues” (44.37%). According to the average score of the items in the three dimensions of CRW, it could be seen that patients had the highest standardized score (standardized score = median score/the total score of the dimension*100%) in future prospects (30.00%), the second standardized score in physical and symptomatic problems (20.00%), and the lowest in social and interpersonal problems (15.00%) as it is shown in Table 2 .
On the total score of the CRW scale, patients’ cancer-related worry was significantly correlated with their gender ( p = 0.009) and annual family income ( p = 0.018; see Table 1 ). The results suggested that gender and annual family income were related to the level of concern patients had after developing cancer. Additionally, there was a correlation between patients who received information about their disease from the internet or applications and their level of CRW ( p = 0.024; see Table 1 ).
The correlation analysis between various scales revealed a significant correlation ( p < 0.05; see Table 3 ) between CRW and MDASI-C, BIPQ, CD-RISC-10, and two dimensions of MCMQ (excluding avoidance). It was worth noting that avoidance coping modes did not show a correlation with CRW. In addition, according to the summary of the ninth open-ended question on the BIPQ, patients believed that the main causes of lung cancer were genetics, fatigue, stress from work, family or life, negative emotions (anger, worry) and unhealthy lifestyle (diet, work and rest, smoking), environmental factors (poor air quality, secondhand smoke, cooking fumes), and new coronavirus pneumonia (including vaccination, new coronavirus infection), etc. Further stratified linear regression analysis was conducted to determine the correlation between the dependent and independent variables (Table 4 ).
Table 4 presented the results of the hierarchical linear regression analysis for CRW in early-stage lung cancer patients. First, all scales included in the hierarchical regression analysis were tested for collinearity (variance inflation factor, VIF). The average VIF value was slightly above 1, indicating that the results were acceptable [ 41 ]. Second, the core research variables were divided into four levels according to the theoretical research, and the variables included in each level were analyzed separately. Model 1 included personal characteristics as independent variables and explained 5% of the variance in CRW. The analysis identified only two variables associated with CRW: being female (compared to male) and having low income (compared to high income). In model 2, psychological resilience was identified as an individual psychological characteristic and placed in the second level, resulting in a 9% increase in explanatory power. Model 3 added the antecedents of uncertainty, including symptom burden, illness perception, social support, and source of disease information, at the third level. This significantly increased the explanatory power of the overall regression model by 53%. The addition of coping modes, specifically confrontation coping mode and acceptance-resignation coping mode, to model 4 only increased the explanatory power of the overall regression model by 1%. The overall model demonstrated a total explanatory power of 68%. In Model 4, factors that significantly correlated with CRW included middle income( β = 2.17), psychological resilience( β =-2.42), symptom burden( β = 12.62), illness perception( β = 9.17), social support( β =-3.27), and source of disease( β = 2.01), as well as confrontation coping mode ( β =-1.98) and acceptance-resignation coping mode( β = 2.77), see Table 4 .
This study analyzed data from 302 patients to investigate the clinical, demographic, and psychosocial factors that correlated with cancer-related worry in patients with early-stage lung cancer after surgery. The study extended our understanding of the specific content and relevant factors of psychological distress in post-operative patients with early-stage lung cancer, a relationship that had not been fully investigated.
The study revealed that Chinese patients with early stage lung cancer were primarily concerned about their future prospects related to the disease itself, while sexual life problems caused by cancer were of least concern. This finding was consistent with previous studies [ 42 ], but this study provided more specific information on patients’ cancer-related worries. Lung cancer was widely perceived as a serious illness by the public due to its high cancer-specific mortality rate and low survival rate after diagnosis [ 43 ]. Consequently, patients with lung cancer often experience significant psychological distress after diagnosis. Even if the tumor was successfully removed, patients might still face challenges during recovery [ 44 ]. According to Reese’s research [ 45 ], long-term survivors of lung cancer experienced mild sexual distress. They also noted that sexual distress was significantly associated with physical and emotional symptoms. Although this study found that patients were least concerned about sexual distress, it should be noted that this study was based on the early stages of recovery after cancer surgery. Due to the postoperative repair of their body and emotions, patients were primarily focused on meeting their physiological and safety needs [ 10 ]. Further validation and exploration are required as there are limited studies on sexual distress in post-operative patients with early-stage lung cancer.
This study found that gender and annual family income were associated with the CRW of early-stage lung cancer patients. Among them, women and patients from low-income families had higher CRW scores, which was similar to the results of CRW in other cancer studies [ 14 , 46 ]. The reason might be that women were more conscious about uncomfortable symptoms than men, and women were more concerned about the duration of the disease and subsequent treatment effects than men [ 47 ]. Moreover, lower annual family income might cause patients to face more financial pressure in terms of medical expenses and treatment, thereby increasing their concerns about the consequences of the disease [ 48 ]. In addition, several other studies have found that education level, smoking status, and tumor stage had an impact on cancer-related worry scores [ 14 , 47 ], but this study did not show statistical significance.
After controlling for demographic covariate factors, the study found a significant negative relationship between psychological resilience and CRW. In a study conducted by Chen et al. [ 49 ], lower levels of psychological resilience were observed in post-operative lung cancer patients, which had a direct impact on their emotional state. In the context of treatment and recovery after lung cancer surgery, medical professionals should prioritize enhancing patients’ psychological resilience. This could be achieved through psychological support and appropriate interventions to improve emotional health and quality of life.
The antecedents in the illness uncertainty theoretical framework, such as symptom burden, illness perception, social support, and sources of disease information, were shown to be significantly associated with patients’ cancer worries in this study. The theory of uncertainty in illness [ 50 ] posited that uncertainty was caused by stimulus frames, cognitive abilities, and structural providers. In this study, these antecedents corresponded to symptom burden, illness perception, social support, and sources of disease information, and they correlated with patient worry related to cancer. Patients with a high symptom burden, high illness perception, low social support, and excessive attention to disease information on the Internet were more likely to have high cancer-related concerns. Previous studies [ 40 , 51 ] have verified the relationship between symptom burden, illness perception, and social support with psychological distress in lung cancer patients. However, there have been few studies on this patient group after surgery for early-stage lung cancer, particularly based on the uncertainty theoretical framework. Additionally, when analyzing the antecedents of structured providers, we included the sources from which patients receive information about their disease, particularly statistics from medical staff and online platforms. Our study found that patients who frequently accessed disease information on the internet had higher cancer-related worry scores. This was consistent with previous qualitative studies [ 10 ] which had shown that patients often turn to the internet for disease-related knowledge due to a perceived lack of effective information from medical staff.
This study also explored the association of CRW with coping modes. According to the theoretical framework of uncertainty in illness, coping modes are key for managing uncertainty, as uncertainty influences patients’ coping methods. Previous research [ 51 ] has shown that different coping modes can affect patients’ emotional states. Because this study focused primarily on the factors correlated with CRW, we included coping modes as independent variables in the linear regression analysis. The results indicated that CRW was negatively correlated with the coping mode of confrontation and positively correlated with the coping mode of acceptance-resignation. Acceptance-resignation, a negative coping mode, has been shown [ 52 ] to be associated with patients’ fear of disease progression and negative attitudes toward the disease, which decreases their confidence in treatment. Poręba-Chabros et al. [ 53 ] found that negative coping patterns were significantly associated with depression. When patients adopt an acceptance-resignation coping mode, their compliance behaviors decrease as they succumb to the disease. Conversely, confrontation, a positive coping mode, can enhance patients’ psychosocial adaptability, buffer psychological distress, and improve quality of life [ 52 ]. Interestingly, avoidance coping modes did not show a correlation with CRW in the postoperative population of early stage lung cancer, which is inconsistent with previous studies [ 54 ]. This lack of correlation may be due to several factors. The focus of our study on the first month after surgery may mean that patients are more focused on immediate physical recovery rather than engaging in avoidance behaviors. The nature of avoidance coping may temporarily alleviate worry without addressing underlying concerns, resulting in no measurable effect on CRW. Sample characteristics, measurement limitations, and individual differences in coping modes may also contribute to bias. In addition, strong psychological resilience, robust support systems, positive surgical outcomes, and increased health education may make avoidance modes less relevant or impactful on CRW in this population. Although the two coping modes were found to be associated with cancer-related worry in our study, hierarchical regression analysis showed that their influence on patients’ worry was small. Future research should explore the mechanisms of cancer-related worry and coping modes based on the theoretical framework of illness uncertainty. Given the association between cancer-related worry and coping modes, psychological intervention for patients should be emphasized in clinical practice. Healthcare professionals should conduct comprehensive psychological assessments and provide effective emotional support and education to help cancer patients develop more effective coping modes and reduce worry caused by uncertainty.
Strengths and limitations
This study presented evidence for CRW and the influencing factors that postoperative patients with early-stage lung cancer face. Based on the results, healthcare providers could identify the specific unmet needs of these patients more precisely and develop effective intervention strategies to improve their emotional state and quality of life. While the existing literature has extensively discussed psychological symptoms such as anxiety, depression, and fear in patients with mid-to-late stage lung cancer, relatively little research has been conducted on the mental health of postoperative patients with early-stage lung cancer, who are an important group of long-term lung cancer survivors [ 55 ]. As the number of patients diagnosed with early-stage lung cancer increases, so does concern about their mental health and unmet needs [ 56 , 57 ].
Nonetheless, this study also has some limitations. First, the single-center cross-sectional design and relatively small sample size may limit the generalizability of the findings to the broader population of early-stage lung cancer patients.
Second, while the study adopted a theoretical framework, it primarily conducted basic factor analysis without delving into the interaction mechanisms between the identified factors. This limitation restricts our understanding of how these factors interplay to influence cancer-related worry (CRW) in postoperative patients. In addition, while the CRW variable is largely operationalized in a similar way to the BIPQ and psychological resilience, we found no high correlation between these scales, as indicated by the Variance Inflation Factor (VIF). This means that multicollinearity, or the overlap between the variables, is not an issue in our study. However, we recognize that the scales we used may have limitations and might not fully capture the specific experiences of our sample. Therefore, future research should use different methods to measure CRW and related factors to better understand their individual effects on cancer-related worry.
Third, CRW is a dynamic process [ 20 ], and this study only focused on the patients’ situation within one month after surgery. This snapshot approach may not reflect the evolving nature of CRW over time. Longitudinal studies are needed to provide a more comprehensive understanding of how CRW and its influencing factors change throughout the postoperative recovery period.
These limitations suggest that future research should adopt a longitudinal design to analyze and verify the identified factors over an extended period. Additionally, expanding the study to include multiple centers and larger, more diverse sample sizes would enhance the generalizability of the findings. Exploring the interaction mechanisms between factors using advanced analytical methods could provide deeper insights into the complexities of CRW. By addressing these limitations, future research can build on our findings to offer more robust and generalizable evidence on the psychological distress experienced by postoperative early-stage lung cancer.
Implications for practice
The results of the study indicated that early-stage lung cancer patients in China had significant concerns about their future prospects, particularly regarding the disease itself, with less attention paid to the impact of cancer on sexual life. To facilitate postoperative recovery effectively, healthcare providers must promptly identify and address these specific concerns by incorporating routine psychological assessments and developing tailored intervention strategies, such as cognitive-behavioral therapy and resilience training, to improve patients’ mental health and overall well-being.
Moreover, this study identified psychological resilience, symptom burden, illness perception, social support, sources of disease information (from the Internet or applications), and coping modes of confrontation and acceptance-resignation as key predictors of cancer-related worry in postoperative early-stage lung cancer patients. Managing patients’ postoperative emotional states and enhancing their quality of life requires a deep understanding and proactive intervention by healthcare professionals. Specific strategies include providing psychological assessments, developing individualized care plans, facilitating support groups, and utilizing technology for continuous support.
The study provided insight into cancer-related worry among Chinese patients after surgery for early-stage lung cancer. The results showed that patients were most concerned about their future prospects, particularly the disease itself, while relatively little attention was paid to their sexual distress. The study identified several key factors that correlated with cancer-related worry, including psychological resilience, symptom burden, illness perception, social support, and sources of disease information from the internet, as well as coping modes. These findings emphasized the significance of healthcare providers identifying and addressing the individual needs of patients during post-operative recovery. It is important to improve the emotional state and quality of life of patients through psychological support and disease education. This study provides guidance for post-operative care of patients with early stage lung cancer and suggests avenues for future research. Specifically, further exploration of the mechanisms of these relationships and development of effective interventions are needed.
Data availability
The data that supported the findings of this study are available on request from the corresponding author. The data are not publicly available due to privacy or ethical restrictions.
Meng L, Jiang X, Liang J, Pan Y, Pan F, Liu D. Postoperative psychological stress and expression of stress-related factors HSP70 and IFN-γ in patients with early lung cancer. Minerva Med. 2023;114(1):43–8. https://doi.org/10.23736/s0026-4806.20.06658-6 .
Article PubMed Google Scholar
Zhu S, Yang C, Chen S, Kang L, Li T, Li J, Li L. Effectiveness of a perioperative support programme to reduce psychological distress for family caregivers of patients with early-stage lung cancer: study protocol for a randomised controlled trial. BMJ Open. 2022;12(8):e064416. https://doi.org/10.1136/bmjopen-2022-064416 .
Article PubMed PubMed Central Google Scholar
Rimner A, Ruffini E, Cilento V, Goren E, Ahmad U, Appel S, Bille A, Boubia S, Brambilla C, Cangir AK, et al. The International Association for the study of Lung Cancer Thymic Epithelial tumors Staging Project: an overview of the Central Database Informing Revision of the Forthcoming (Ninth) Edition of the TNM classification of malignant tumors. J Thorac Oncol. 2023;18(10):1386–98. https://doi.org/10.1016/j.jtho.2023.07.008 .
Organization WH. (2023). Lung cancer. Retrieved from https://www.who.int/zh/news-room/fact-sheets/detail/lung-cancer
Association OSCM, House CMAP. Chinese Medical Association guideline for clinical diagnosis and treatment of lung cancer (2023 edition). Chin J Oncol. 2023;45(7):539–74. https://doi.org/10.3760/cma.j.cn112152-20230510-00200 .
Article Google Scholar
Postmus PE, Kerr KM, Oudkerk M, Senan S, Waller DA, Vansteenkiste J, Escriu C, Peters S. Early and locally advanced non-small-cell lung cancer (NSCLC): ESMO Clinical Practice guidelines for diagnosis, treatment and follow-up. Ann Oncol. 2017;28(suppl4):iv1. https://doi.org/10.1093/annonc/mdx222 .
Gu Z, Wang H, Mao T, Ji C, Xiang Y, Zhu Y, Xu P, Fang W. Pulmonary function changes after different extent of pulmonary resection under video-assisted thoracic surgery. J Thorac Dis. 2018;10(4):2331–7. https://doi.org/10.21037/jtd.2018.03.163 .
Chen L, Gu Z, Lin B, Wang W, Xu N, Liu Y, Ji C, Fang W. Pulmonary function changes after thoracoscopic lobectomy versus intentional thoracoscopic segmentectomy for early-stage non-small cell lung cancer. Transl Lung Cancer Res. 2021;10(11):4141–51. https://doi.org/10.21037/tlcr-21-661 .
Guo X, Zhu X, Yuan Y. Research Progress on the correlation of Psychological disorders in Lung Cancer patients. Med Philos. 2020;41(2):36–9. https://doi.org/10.12014/j.issn.1002-0772.2020.02.09 .
Yang Y, Chen X, Pan X, Tang X, Fan J, Li Y. The unmet needs of patients in the early rehabilitation stage after lung cancer surgery: a qualitative study based on Maslow’s hierarchy of needs theory. Support Care Cancer. 2023;31(12):677. https://doi.org/10.1007/s00520-023-08129-z .
Pongthavornkamol K, Lekdamrongkul P, Pinsuntorn P, Molassiotis A. Physical symptoms, unmet needs, and Quality of Life in Thai Cancer survivors after the completion of primary treatment. Asia Pac J Oncol Nurs. 2019;6(4):363–71. https://doi.org/10.4103/apjon.apjon_26_19 .
Park J, Jung W, Lee G, Kang D, Shim YM, Kim HK, Jeong A, Cho J, Shin DW. Unmet supportive care needs after Non-small Cell Lung Cancer Resection at a Tertiary Hospital in Seoul, South Korea. Healthc (Basel). 2023;11(14). https://doi.org/10.3390/healthcare11142012 .
Hirai K, Shiozaki M, Motooka H, Arai H, Koyama A, Inui H, Uchitomi Y. Discrimination between worry and anxiety among cancer patients: development of a brief Cancer-related worry inventory. Psychooncology. 2008;17(12):1172–9. https://doi.org/10.1002/pon.1348 .
Papaleontiou M, Reyes-Gastelum D, Gay BL, Ward KC, Hamilton AS, Hawley ST, Haymart MR. Worry in thyroid Cancer survivors with a favorable prognosis. Thyroid. 2019;29(8):1080–8. https://doi.org/10.1089/thy.2019.0163 .
Ware ME, Delaney A, Krull KR, Brinkman TM, Armstrong GT, Wilson CL, Mulrooney DA, Wang Z, Lanctot JQ, Krull MR, et al. Cancer-related worry as a predictor of 5-yr physical activity level in Childhood Cancer survivors. Med Sci Sports Exerc. 2023;55(9):1584–91. https://doi.org/10.1249/mss.0000000000003195 .
Mathews A. Why worry? The cognitive function of anxiety. Behav Res Ther. 1990;28(6):455–68. https://doi.org/10.1016/0005-7967(90)90132-3 .
Gotay CC, Pagano IS. Assessment of survivor concerns (ASC): a newly proposed brief questionnaire. Health Qual Life Outcomes. 2007. https://doi.org/10.1186/1477-7525-5-15 . 5.
Andersen MR, Smith R, Meischke H, Bowen D, Urban N. Breast cancer worry and mammography use by women with and without a family history in a population-based sample. Cancer Epidemiol Biomarkers Prev. 2003;12(4):314–20.
PubMed Google Scholar
Wu SM, Schuler TA, Edwards MC, Yang HC, Brothers BM. Factor analytic and item response theory evaluation of the Penn State worry questionnaire in women with cancer. Qual Life Res. 2013;22(6):1441–9. https://doi.org/10.1007/s11136-012-0253-0 .
Jackson Levin N, Zhang A, Reyes-Gastelum D, Chen DW, Hamilton AS, Zebrack B, Haymart MR. Change in worry over time among hispanic women with thyroid cancer. J Cancer Surviv. 2022;16(4):844–52. https://doi.org/10.1007/s11764-021-01078-8 .
McDonnell GA, Brinkman TM, Wang M, Gibson TM, Heathcote LC, Ehrhardt MJ, Srivastava DK, Robison LL, Hudson MM, Alberts NM. Prevalence and predictors of cancer-related worry and associations with health behaviors in adult survivors of childhood cancer. Cancer. 2021;127(15):2743–51. https://doi.org/10.1002/cncr.33563 .
Jones SM, Ziebell R, Walker R, Nekhlyudov L, Rabin BA, Nutt S, Fujii M, Chubak J. Association of worry about cancer to benefit finding and functioning in long-term cancer survivors. Support Care Cancer. 2017;25(5):1417–22. https://doi.org/10.1007/s00520-016-3537-z .
Mishel MH. Uncertainty in illness. Image J Nurs Sch. 1988;20(4):225–32. https://doi.org/10.1111/j1547-50691988tb00082x .
Brand H, Speiser D, Besch L, Roseman J, Kendel F. Making sense of a health threat: illness representations, coping, and psychological distress among BRCA1/2 mutation carriers. Genes (Basel). 2021;12(5). https://doi.org/10.3390/genes12050741 .
Gordon R, Fawson S, Moss-Morris R, Armes J, Hirsch CR. An experimental study to identify key psychological mechanisms that promote and predict resilience in the aftermath of treatment for breast cancer. Psychooncology. 2022;31(2):198–206. https://doi.org/10.1002/pon.5806 .
Harms CA, Cohen L, Pooley JA, Chambers SK, Galvão DA, Newton RU. Quality of life and psychological distress in cancer survivors: the role of psycho-social resources for resilience. Psychooncology. 2019;28(2):271–7. https://doi.org/10.1002/pon.4934 .
National Health Commission, PRC. (2022). Clinical Practice Guideline for Primary Lung Cancer (2022 Version). Medical Journal of Peking Union Medical College Hospital, 13(4), 549–570.Retrieved from https://kns.cnki.net/kcms/detail/11.5882.r.20220629.1511.002.html
Goldstraw P, Chansky K, Crowley J, Rami-Porta R, Asamura H, Eberhardt WE, Nicholson AG, Groome P, Mitchell A, Bolejack V. The IASLC Lung Cancer Staging Project: proposals for revision of the TNM Stage groupings in the Forthcoming (Eighth) Edition of the TNM classification for Lung Cancer. J Thorac Oncol. 2016;11(1):39–51. https://doi.org/10.1016/j.jtho.2015.09.009 .
Faul F, Erdfelder E, Buchner A, Lang AG. Statistical power analyses using G * Power 3.1: tests for correlation and regression analyses. Behav Res Methods. 2009;41(4):1149–60. https://doi.org/10.3758/brm.41.4.1149 .
Nelson DB, Mehran RJ, Mena GE, Hofstetter WL, Vaporciyan AA, Antonoff MB, Rice DC. Enhanced recovery after surgery improves postdischarge recovery after pulmonary lobectomy. J Thorac Cardiovasc Surg. 2023;165(5):1731–40. https://doi.org/10.1016/j.jtcvs.2022.09.064 .
He S, Cui G, Liu W, Tang L, Liu J. Validity and reliability of the brief Cancer-related worry inventory in patients with colorectal cancer after operation. Chin Mental Health J. 2020;34(5):463–8. https://doi.org/10.3969/j.issn.1000-6729.2020.5.013 .
Cleeland CS, Mendoza TR, Wang XS, Chou C, Harle MT, Morrissey M, Engstrom MC. Assessing symptom distress in cancer patients: the M.D. Anderson Symptom Inventory. Cancer. 2000;89(7):1634–46. https://doi.org/10.1002/1097-0142(20001001)89:7%3C1634::aid-cncr29%3E3.0.co;2-v .
Broadbent E, Petrie KJ, Main J, Weinman J. The brief illness perception Questionnaire. J Psychosom Res. 2006;60(6):631–7. https://doi.org/10.1016/j.jpsychores.2005.10.020 .
Mei Y, Li H, Yang Y, Su D, Ma L, Zhang T, Dou W. Reliability and validity of Chinese Version of the brief illness perception questionnaire in patients with breast Cancer. J Nurs 2015(24):11–4 https://doi.org/10.16460/j.issn1008-9969.2015.24.011
Connor KM, Davidson JRT. Development of a new resilience scale: the Connor-Davidson Resilience scale (CD-RISC). Depress Anxiety. 2003;18(2):76–82. https://doi.org/10.1002/da.10113 .
Ye Z, Ruan X, Zeng Z, Xie Q, Cheng M, Peng C, Lu Y, Qiu H. Psychometric properties of 10-item Connor-Davidson Resilience scale among nursing students. J Nurs. 2016;23(21):9–13. https://doi.org/10.16460/j.issn1008-9969.2016.21.009 .
Feifel H, Strack S, Nagy VT, DEGREE OF LIFE-THREAT AND DIFFERENTIAL USE OF COPING MODES. J Psychosom Res. 1987;31(1):91–9. https://doi.org/10.1016/0022-3999(87)90103-6 .
Shen S, Jiang Q. Report on application of Chinese version of MCMQ in 701 patients. Chin J Behav Med Brain Sci. 2000;9(1):18. https://doi.org/10.3760/cma.j.issn.1674-6554.2000.01.008 .
Zimet GD, Dahlem NW, Zimet SG, Farley GK. THE MULTIDIMENSIONAL SCALE OF PERCEIVED SOCIAL SUPPORT. J Pers Assess. 1988;52(1):30–41. https://doi.org/10.1207/s15327752jpa5201_2 .
Chou KL. Assessing Chinese adolescents’ social support: the multidimensional scale of perceived social support. Pers Indiv Differ. 2000;28(2):299–307. https://doi.org/10.1016/s0191-8869(99)00098-7 .
Jia JP, He XQ, Jin YJ. Statistics. 7th ed. Beijing: Renmin University of China; 2018.
Google Scholar
Zhao F, Liu L, Zhang F, Kong Q. Analysis of fear of cancer recurrence in patients with lung cancer after surgery and its influencing factors. J Nurses Train. 2023;38(17):1619–22. https://doi.org/10.16821/j.cnki.hsjx.2023.17.018 .
Wang YH, Li JQ, Shi JF, Que JY, Liu JJ, Lappin JM, Leung J, Ravindran AV, Chen WQ, Qiao YL, et al. Depression and anxiety in relation to cancer incidence and mortality: a systematic review and meta-analysis of cohort studies. Mol Psychiatry. 2020;25(7):1487–99. https://doi.org/10.1038/s41380-019-0595-x .
Morrison EJ, Novotny PJ, Sloan JA, Yang P, Patten CA, Ruddy KJ, Clark MM. Emotional problems, Quality of Life, and Symptom Burden in patients with Lung Cancer. Clin Lung Cancer. 2017;18(5):497–503. https://doi.org/10.1016/j.cllc.2017.02.008 .
Reese JB, Shelby RA, Abernethy AP. Sexual concerns in lung cancer patients: an examination of predictors and moderating effects of age and gender. Support Care Cancer. 2011;19(1):161–5. https://doi.org/10.1007/s00520-010-1000-0 .
Khoshab N, Vaidya TS, Dusza S, Nehal KS, Lee EH. Factors contributing to cancer worry in the skin cancer population. J Am Acad Dermatol. 2020;83(2):626–8. https://doi.org/10.1016/j.jaad.2019.09.068 .
Chen X, He X. Current status of postoperative psychological distress in patients with lung cancer and its influencing factors. Chin J Mod Nurs. 2021;24:3318–22.
Rogers Z, Elliott F, Kasparian NA, Bishop DT, Barrett JH, Newton-Bishop J. Psychosocial, clinical and demographic features related to worry in patients with melanoma. Melanoma Res. 2016;26(5):497–504. https://doi.org/10.1097/cmr.0000000000000266 .
Chen S, Mei R, Tan C, Li X, Zhong C, Ye M. Psychological resilience and related influencing factors in postoperative non-small cell lung cancer patients: a cross-sectional study. Psychooncology. 2020;29(11):1815–22. https://doi.org/10.1002/pon.5485 .
Mishel MH, Braden CJ. Finding meaning: antecedents of uncertainty in illness. Nurs Res. 1988;37(2):98–103.
Tian X, Jin Y, Chen H, Tang L, Jiménez-Herrera MF. Relationships among Social Support, coping style, perceived stress, and psychological distress in Chinese Lung Cancer patients. Asia Pac J Oncol Nurs. 2021;8(2):172–9. https://doi.org/10.4103/apjon.apjon_59_20 .
Prikken S, Luyckx K, Raymaekers K, Raemen L, Verschueren M, Lemiere J, Vercruysse T, Uyttebroeck A. Identity formation in adolescent and emerging adult cancer survivors: a differentiated perspective and associations with psychosocial functioning. Psychol Health. 2023;38(1):55–72. https://doi.org/10.1080/08870446.2021.1955116 .
Poręba-Chabros A, Kolańska-Stronka M, Mamcarz P, Mamcarz I. Cognitive appraisal of the disease and stress level in lung cancer patients. The mediating role of coping styles. Support Care Cancer. 2022;30(6):4797–806. https://doi.org/10.1007/s00520-022-06880-3 .
Park CL, Cho D, Blank TO, Wortmann JH. Cognitive and emotional aspects of fear of recurrence: predictors and relations with adjustment in young to middle-aged cancer survivors. Psychooncology. 2013;22(7):1630–8. https://doi.org/10.1002/pon.3195 .
Jovanoski N, Bowes K, Brown A, Belleli R, Di Maio D, Chadda S, Abogunrin S. Survival and quality-of-life outcomes in early-stage NSCLC patients: a literature review of real-world evidence. Lung Cancer Manag. 2023;12(3). https://doi.org/10.2217/lmt-2023-0003 .
Jonas DE, Reuland DS, Reddy SM, Nagle M, Clark SD, Weber RP, Enyioha C, Malo TL, Brenner AT, Armstrong C, et al. Screening for Lung Cancer with Low-Dose Computed Tomography: updated evidence report and systematic review for the US Preventive Services Task Force. JAMA. 2021;325(10):971–87. https://doi.org/10.1001/jama.2021.0377 .
Ho J, McWilliams A, Emery J, Saunders C, Reid C, Robinson S, Brims F. Integrated care for resected early stage lung cancer: innovations and exploring patient needs. BMJ Open Respir Res. 2017;4(1):e000175. https://doi.org/10.1136/bmjresp-2016-000175 .
Download references
Acknowledgements
The authors would like to thank the nurses (Especially Gu Jingzhi and Liu Jing) and doctors at Shanghai Pulmonary Hospital for facilitating this study and all the patients who kindly participated in the survey.
Scientific clinical research project of Tongji University, JS2210319; Key disciplines of Shanghai’s Three-Year Action Plan to Strengthen Public Health System Construction (2023–2025), GWVI-11.1-28; The National Key Research and Development Plan Project of China, 2022YFC3600903.
Author information
Yingzi Yang and Xiaolan Qian share first authorship.
Authors and Affiliations
Department of Health Care, Shanghai Health and Medical Center, No. 67, Dajishan, Wuxi City, Jiangsu Province, 214063, People’s Republic of China
Yingzi Yang, Xiaolan Qian & Xuefeng Tang
School of Medicine, Tongji University, 1239 Siping Road, Shanghai, 200092, People’s Republic of China
Yingzi Yang, Chen Shen & Xiaoting Pan
Department of Thoracic Surgery, Shanghai Pulmonary Hospital, Tongji University, No.507, Zhengmin Road, Shanghai, 200433, People’s Republic of China
Yujing Zhou
Department of Nursing, Shanghai Pulmonary Hospital, Tongji University, No.507, Zhengmin Road, Shanghai, 200433, People’s Republic of China
You can also search for this author in PubMed Google Scholar
Contributions
Y.Y. and Y. L designed the study. Y.Y., Y.Z., C.S and X.P. ran the study and collected the data. Y.Y., X.Q., X.T. and C.S. analyzed the data; Y.Y., and X. Q. interpreted the results and drafted the paper. Y.Y. wrote the main manuscript text and X.Q. prepared Tables 1 , 2 , 3 and 4 . Y.Y., X.T. and Y.L revised the manuscript. All authors read and approved the final version of the manuscript.
Corresponding authors
Correspondence to Xuefeng Tang or Yumei Li .
Ethics declarations
Ethics approval and consent to participate.
The study was approved by the institutional review board at the Shanghai Pulmonary Hospital (Q23–396). The procedures used in this study adhere to the tenets of the Declaration of Helsinki. Consent for publication Informed consent was obtained from all individual participants included in the study.
Consent for publication
Not applicable.
Competing interests
The authors declare no competing interests.
Additional information
Publisher’s note.
Springer Nature remains neutral with regard to jurisdictional claims in published maps and institutional affiliations.
Electronic supplementary material
Below is the link to the electronic supplementary material.
Supplementary Material 1
Supplementary material 2, rights and permissions.
Open Access This article is licensed under a Creative Commons Attribution-NonCommercial-NoDerivatives 4.0 International License, which permits any non-commercial use, sharing, distribution and reproduction in any medium or format, as long as you give appropriate credit to the original author(s) and the source, provide a link to the Creative Commons licence, and indicate if you modified the licensed material. You do not have permission under this licence to share adapted material derived from this article or parts of it. The images or other third party material in this article are included in the article’s Creative Commons licence, unless indicated otherwise in a credit line to the material. If material is not included in the article’s Creative Commons licence and your intended use is not permitted by statutory regulation or exceeds the permitted use, you will need to obtain permission directly from the copyright holder. To view a copy of this licence, visit http://creativecommons.org/licenses/by-nc-nd/4.0/ .
Reprints and permissions
About this article
Cite this article.
Yang, Y., Qian, X., Tang, X. et al. The links between symptom burden, illness perception, psychological resilience, social support, coping modes, and cancer-related worry in Chinese early-stage lung cancer patients after surgery: a cross-sectional study. BMC Psychol 12 , 463 (2024). https://doi.org/10.1186/s40359-024-01946-9
Download citation
Received : 18 May 2024
Accepted : 12 August 2024
Published : 31 August 2024
DOI : https://doi.org/10.1186/s40359-024-01946-9
Share this article
Anyone you share the following link with will be able to read this content:
Sorry, a shareable link is not currently available for this article.
Provided by the Springer Nature SharedIt content-sharing initiative
- Lung cancer
- Psychological distress
- Uncertainty
BMC Psychology
ISSN: 2050-7283
- General enquiries: [email protected]
Have a language expert improve your writing
Run a free plagiarism check in 10 minutes, generate accurate citations for free.
- Knowledge Base
Methodology
- What Is a Research Design | Types, Guide & Examples
What Is a Research Design | Types, Guide & Examples
Published on June 7, 2021 by Shona McCombes . Revised on November 20, 2023 by Pritha Bhandari.
A research design is a strategy for answering your research question using empirical data. Creating a research design means making decisions about:
- Your overall research objectives and approach
- Whether you’ll rely on primary research or secondary research
- Your sampling methods or criteria for selecting subjects
- Your data collection methods
- The procedures you’ll follow to collect data
- Your data analysis methods
A well-planned research design helps ensure that your methods match your research objectives and that you use the right kind of analysis for your data.
Table of contents
Step 1: consider your aims and approach, step 2: choose a type of research design, step 3: identify your population and sampling method, step 4: choose your data collection methods, step 5: plan your data collection procedures, step 6: decide on your data analysis strategies, other interesting articles, frequently asked questions about research design.
- Introduction
Before you can start designing your research, you should already have a clear idea of the research question you want to investigate.
There are many different ways you could go about answering this question. Your research design choices should be driven by your aims and priorities—start by thinking carefully about what you want to achieve.
The first choice you need to make is whether you’ll take a qualitative or quantitative approach.
Qualitative approach | Quantitative approach |
---|---|
and describe frequencies, averages, and correlations about relationships between variables |
Qualitative research designs tend to be more flexible and inductive , allowing you to adjust your approach based on what you find throughout the research process.
Quantitative research designs tend to be more fixed and deductive , with variables and hypotheses clearly defined in advance of data collection.
It’s also possible to use a mixed-methods design that integrates aspects of both approaches. By combining qualitative and quantitative insights, you can gain a more complete picture of the problem you’re studying and strengthen the credibility of your conclusions.
Practical and ethical considerations when designing research
As well as scientific considerations, you need to think practically when designing your research. If your research involves people or animals, you also need to consider research ethics .
- How much time do you have to collect data and write up the research?
- Will you be able to gain access to the data you need (e.g., by travelling to a specific location or contacting specific people)?
- Do you have the necessary research skills (e.g., statistical analysis or interview techniques)?
- Will you need ethical approval ?
At each stage of the research design process, make sure that your choices are practically feasible.
Receive feedback on language, structure, and formatting
Professional editors proofread and edit your paper by focusing on:
- Academic style
- Vague sentences
- Style consistency
See an example
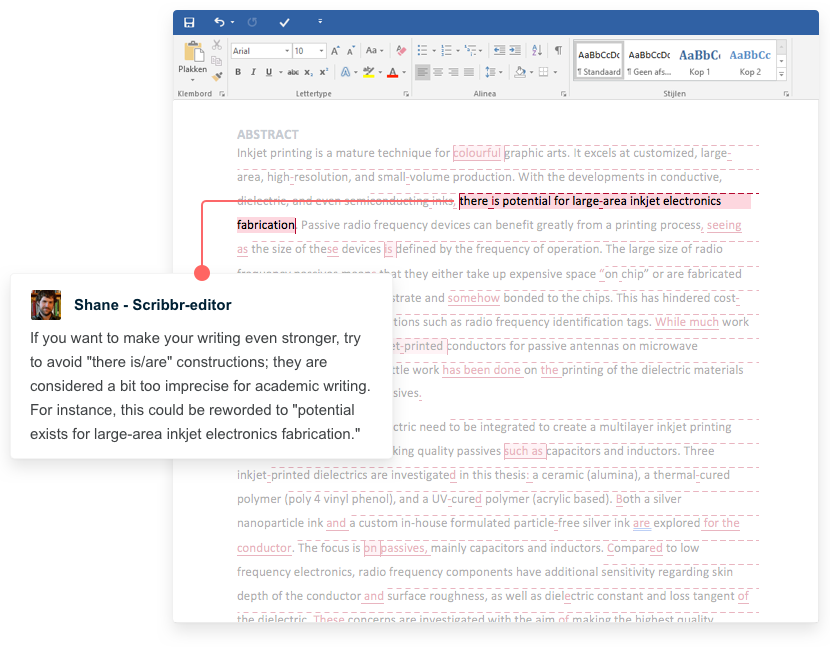
Within both qualitative and quantitative approaches, there are several types of research design to choose from. Each type provides a framework for the overall shape of your research.
Types of quantitative research designs
Quantitative designs can be split into four main types.
- Experimental and quasi-experimental designs allow you to test cause-and-effect relationships
- Descriptive and correlational designs allow you to measure variables and describe relationships between them.
Type of design | Purpose and characteristics |
---|---|
Experimental | relationships effect on a |
Quasi-experimental | ) |
Correlational | |
Descriptive |
With descriptive and correlational designs, you can get a clear picture of characteristics, trends and relationships as they exist in the real world. However, you can’t draw conclusions about cause and effect (because correlation doesn’t imply causation ).
Experiments are the strongest way to test cause-and-effect relationships without the risk of other variables influencing the results. However, their controlled conditions may not always reflect how things work in the real world. They’re often also more difficult and expensive to implement.
Types of qualitative research designs
Qualitative designs are less strictly defined. This approach is about gaining a rich, detailed understanding of a specific context or phenomenon, and you can often be more creative and flexible in designing your research.
The table below shows some common types of qualitative design. They often have similar approaches in terms of data collection, but focus on different aspects when analyzing the data.
Type of design | Purpose and characteristics |
---|---|
Grounded theory | |
Phenomenology |
Your research design should clearly define who or what your research will focus on, and how you’ll go about choosing your participants or subjects.
In research, a population is the entire group that you want to draw conclusions about, while a sample is the smaller group of individuals you’ll actually collect data from.
Defining the population
A population can be made up of anything you want to study—plants, animals, organizations, texts, countries, etc. In the social sciences, it most often refers to a group of people.
For example, will you focus on people from a specific demographic, region or background? Are you interested in people with a certain job or medical condition, or users of a particular product?
The more precisely you define your population, the easier it will be to gather a representative sample.
- Sampling methods
Even with a narrowly defined population, it’s rarely possible to collect data from every individual. Instead, you’ll collect data from a sample.
To select a sample, there are two main approaches: probability sampling and non-probability sampling . The sampling method you use affects how confidently you can generalize your results to the population as a whole.
Probability sampling | Non-probability sampling |
---|---|
Probability sampling is the most statistically valid option, but it’s often difficult to achieve unless you’re dealing with a very small and accessible population.
For practical reasons, many studies use non-probability sampling, but it’s important to be aware of the limitations and carefully consider potential biases. You should always make an effort to gather a sample that’s as representative as possible of the population.
Case selection in qualitative research
In some types of qualitative designs, sampling may not be relevant.
For example, in an ethnography or a case study , your aim is to deeply understand a specific context, not to generalize to a population. Instead of sampling, you may simply aim to collect as much data as possible about the context you are studying.
In these types of design, you still have to carefully consider your choice of case or community. You should have a clear rationale for why this particular case is suitable for answering your research question .
For example, you might choose a case study that reveals an unusual or neglected aspect of your research problem, or you might choose several very similar or very different cases in order to compare them.
Data collection methods are ways of directly measuring variables and gathering information. They allow you to gain first-hand knowledge and original insights into your research problem.
You can choose just one data collection method, or use several methods in the same study.
Survey methods
Surveys allow you to collect data about opinions, behaviors, experiences, and characteristics by asking people directly. There are two main survey methods to choose from: questionnaires and interviews .
Questionnaires | Interviews |
---|---|
) |
Observation methods
Observational studies allow you to collect data unobtrusively, observing characteristics, behaviors or social interactions without relying on self-reporting.
Observations may be conducted in real time, taking notes as you observe, or you might make audiovisual recordings for later analysis. They can be qualitative or quantitative.
Quantitative observation | |
---|---|
Other methods of data collection
There are many other ways you might collect data depending on your field and topic.
Field | Examples of data collection methods |
---|---|
Media & communication | Collecting a sample of texts (e.g., speeches, articles, or social media posts) for data on cultural norms and narratives |
Psychology | Using technologies like neuroimaging, eye-tracking, or computer-based tasks to collect data on things like attention, emotional response, or reaction time |
Education | Using tests or assignments to collect data on knowledge and skills |
Physical sciences | Using scientific instruments to collect data on things like weight, blood pressure, or chemical composition |
If you’re not sure which methods will work best for your research design, try reading some papers in your field to see what kinds of data collection methods they used.
Secondary data
If you don’t have the time or resources to collect data from the population you’re interested in, you can also choose to use secondary data that other researchers already collected—for example, datasets from government surveys or previous studies on your topic.
With this raw data, you can do your own analysis to answer new research questions that weren’t addressed by the original study.
Using secondary data can expand the scope of your research, as you may be able to access much larger and more varied samples than you could collect yourself.
However, it also means you don’t have any control over which variables to measure or how to measure them, so the conclusions you can draw may be limited.
Here's why students love Scribbr's proofreading services
Discover proofreading & editing
As well as deciding on your methods, you need to plan exactly how you’ll use these methods to collect data that’s consistent, accurate, and unbiased.
Planning systematic procedures is especially important in quantitative research, where you need to precisely define your variables and ensure your measurements are high in reliability and validity.
Operationalization
Some variables, like height or age, are easily measured. But often you’ll be dealing with more abstract concepts, like satisfaction, anxiety, or competence. Operationalization means turning these fuzzy ideas into measurable indicators.
If you’re using observations , which events or actions will you count?
If you’re using surveys , which questions will you ask and what range of responses will be offered?
You may also choose to use or adapt existing materials designed to measure the concept you’re interested in—for example, questionnaires or inventories whose reliability and validity has already been established.
Reliability and validity
Reliability means your results can be consistently reproduced, while validity means that you’re actually measuring the concept you’re interested in.
Reliability | Validity |
---|---|
) ) |
For valid and reliable results, your measurement materials should be thoroughly researched and carefully designed. Plan your procedures to make sure you carry out the same steps in the same way for each participant.
If you’re developing a new questionnaire or other instrument to measure a specific concept, running a pilot study allows you to check its validity and reliability in advance.
Sampling procedures
As well as choosing an appropriate sampling method , you need a concrete plan for how you’ll actually contact and recruit your selected sample.
That means making decisions about things like:
- How many participants do you need for an adequate sample size?
- What inclusion and exclusion criteria will you use to identify eligible participants?
- How will you contact your sample—by mail, online, by phone, or in person?
If you’re using a probability sampling method , it’s important that everyone who is randomly selected actually participates in the study. How will you ensure a high response rate?
If you’re using a non-probability method , how will you avoid research bias and ensure a representative sample?
Data management
It’s also important to create a data management plan for organizing and storing your data.
Will you need to transcribe interviews or perform data entry for observations? You should anonymize and safeguard any sensitive data, and make sure it’s backed up regularly.
Keeping your data well-organized will save time when it comes to analyzing it. It can also help other researchers validate and add to your findings (high replicability ).
On its own, raw data can’t answer your research question. The last step of designing your research is planning how you’ll analyze the data.
Quantitative data analysis
In quantitative research, you’ll most likely use some form of statistical analysis . With statistics, you can summarize your sample data, make estimates, and test hypotheses.
Using descriptive statistics , you can summarize your sample data in terms of:
- The distribution of the data (e.g., the frequency of each score on a test)
- The central tendency of the data (e.g., the mean to describe the average score)
- The variability of the data (e.g., the standard deviation to describe how spread out the scores are)
The specific calculations you can do depend on the level of measurement of your variables.
Using inferential statistics , you can:
- Make estimates about the population based on your sample data.
- Test hypotheses about a relationship between variables.
Regression and correlation tests look for associations between two or more variables, while comparison tests (such as t tests and ANOVAs ) look for differences in the outcomes of different groups.
Your choice of statistical test depends on various aspects of your research design, including the types of variables you’re dealing with and the distribution of your data.
Qualitative data analysis
In qualitative research, your data will usually be very dense with information and ideas. Instead of summing it up in numbers, you’ll need to comb through the data in detail, interpret its meanings, identify patterns, and extract the parts that are most relevant to your research question.
Two of the most common approaches to doing this are thematic analysis and discourse analysis .
Approach | Characteristics |
---|---|
Thematic analysis | |
Discourse analysis |
There are many other ways of analyzing qualitative data depending on the aims of your research. To get a sense of potential approaches, try reading some qualitative research papers in your field.
If you want to know more about the research process , methodology , research bias , or statistics , make sure to check out some of our other articles with explanations and examples.
- Simple random sampling
- Stratified sampling
- Cluster sampling
- Likert scales
- Reproducibility
Statistics
- Null hypothesis
- Statistical power
- Probability distribution
- Effect size
- Poisson distribution
Research bias
- Optimism bias
- Cognitive bias
- Implicit bias
- Hawthorne effect
- Anchoring bias
- Explicit bias
A research design is a strategy for answering your research question . It defines your overall approach and determines how you will collect and analyze data.
A well-planned research design helps ensure that your methods match your research aims, that you collect high-quality data, and that you use the right kind of analysis to answer your questions, utilizing credible sources . This allows you to draw valid , trustworthy conclusions.
Quantitative research designs can be divided into two main categories:
- Correlational and descriptive designs are used to investigate characteristics, averages, trends, and associations between variables.
- Experimental and quasi-experimental designs are used to test causal relationships .
Qualitative research designs tend to be more flexible. Common types of qualitative design include case study , ethnography , and grounded theory designs.
The priorities of a research design can vary depending on the field, but you usually have to specify:
- Your research questions and/or hypotheses
- Your overall approach (e.g., qualitative or quantitative )
- The type of design you’re using (e.g., a survey , experiment , or case study )
- Your data collection methods (e.g., questionnaires , observations)
- Your data collection procedures (e.g., operationalization , timing and data management)
- Your data analysis methods (e.g., statistical tests or thematic analysis )
A sample is a subset of individuals from a larger population . Sampling means selecting the group that you will actually collect data from in your research. For example, if you are researching the opinions of students in your university, you could survey a sample of 100 students.
In statistics, sampling allows you to test a hypothesis about the characteristics of a population.
Operationalization means turning abstract conceptual ideas into measurable observations.
For example, the concept of social anxiety isn’t directly observable, but it can be operationally defined in terms of self-rating scores, behavioral avoidance of crowded places, or physical anxiety symptoms in social situations.
Before collecting data , it’s important to consider how you will operationalize the variables that you want to measure.
A research project is an academic, scientific, or professional undertaking to answer a research question . Research projects can take many forms, such as qualitative or quantitative , descriptive , longitudinal , experimental , or correlational . What kind of research approach you choose will depend on your topic.
Cite this Scribbr article
If you want to cite this source, you can copy and paste the citation or click the “Cite this Scribbr article” button to automatically add the citation to our free Citation Generator.
McCombes, S. (2023, November 20). What Is a Research Design | Types, Guide & Examples. Scribbr. Retrieved September 3, 2024, from https://www.scribbr.com/methodology/research-design/
Is this article helpful?
Shona McCombes
Other students also liked, guide to experimental design | overview, steps, & examples, how to write a research proposal | examples & templates, ethical considerations in research | types & examples, what is your plagiarism score.

COMMENTS
For example, it can look into why the value of the Japanese Yen has decreased. This is so that an analytical study can consider "how" and "why" questions. Another example is that someone might conduct analytical research to identify a study's gap. It presents a fresh perspective on your data.
Learn how descriptive and analytical research differ in their goals, methods, and applications. See examples of research questions on disability and statistics for each type of research.
Table of contents. Step 1: Write your hypotheses and plan your research design. Step 2: Collect data from a sample. Step 3: Summarize your data with descriptive statistics. Step 4: Test hypotheses or make estimates with inferential statistics.
When are analytical study designs used? A study design is a systematic plan, developed so you can carry out your research study effectively and efficiently. Having a design is important because it will determine the right methodologies for your study. Using the right study design makes your results more credible, valid, and coherent.
Revised on June 22, 2023. Quantitative research is the process of collecting and analyzing numerical data. It can be used to find patterns and averages, make predictions, test causal relationships, and generalize results to wider populations. Quantitative research is the opposite of qualitative research, which involves collecting and analyzing ...
Quantitative data analysis is one of those things that often strikes fear in students. It's totally understandable - quantitative analysis is a complex topic, full of daunting lingo, like medians, modes, correlation and regression.Suddenly we're all wishing we'd paid a little more attention in math class…. The good news is that while quantitative data analysis is a mammoth topic ...
Introduction. We search for the determinants of health outcomes, first, by relying on descriptive epidemiology to generate hypotheses about associations between exposures and outcomes. Analytic studies are then undertaken to test specific hypotheses. Samples of subjects are identified and information about exposure status and outcome is collected.
Data Analysis Techniques in Research Examples. ... The selection of a specific method often depends on the nature of the data, the research objectives, and the analytical requirements of the project or organization. Also Read: Quantitative Data Analysis: Types, Analysis & Examples.
Types of Research Designs Compared | Guide & Examples. Published on June 20, 2019 by Shona McCombes.Revised on June 22, 2023. When you start planning a research project, developing research questions and creating a research design, you will have to make various decisions about the type of research you want to do.. There are many ways to categorize different types of research.
Data Analysis. Different statistics and methods used to describe the characteristics of the members of a sample or population, explore the relationships between variables, to test research hypotheses, and to visually represent data are described. Terms relating to the topics covered are defined in the Research Glossary.
What Is Data Analysis? (With Examples) Data analysis is the practice of working with data to glean useful information, which can then be used to make informed decisions. "It is a capital mistake to theorize before one has data. Insensibly one begins to twist facts to suit theories, instead of theories to suit facts," Sherlock Holme's proclaims ...
Descriptive writing focuses on the what, while critical/analytical writing focuses on the so what. Analytical writing should link the discussion back to the research aims, objectives or research questions (the golden thread). Some amount of description will always be needed, but aim to minimise description and maximise analysis to earn higher ...
An analytical study is one in which action will be taken on a cause system to improve the future performance of the system of interest. The aim of an enumerative study is estimation, while an analytical study focuses on prediction. Because of the temporal nature of improvement, the theory and methods for analytical studies are a critical ...
QDA Method #1: Qualitative Content Analysis. Content analysis is possibly the most common and straightforward QDA method. At the simplest level, content analysis is used to evaluate patterns within a piece of content (for example, words, phrases or images) or across multiple pieces of content or sources of communication. For example, a collection of newspaper articles or political speeches.
Analytical approaches range from basic comparisons of measurements to more formal statistical tests (see page on establishing differences from expectations). Incorporating predictions of traits into causal analysis is an area of active research, and so we present a hypothetical example below.
Analytical research is a methodical investigation approach that delves deep into complex subjects through data analysis. It aids in understanding, problem-solving, and informed decision-making in diverse fields. Let's understand the concept of Analytical Research in detail. A retail company is using analytical research to enhance its marketing ...
21. Judgment. Judgment is the ability to make decisions that yield positive and reasonable outcomes. It is an important analytical skill for leaders and management professionals. Judgment skills can help managers make hiring decisions and guide professionals to act in the best interest of their company's goals. 22.
Here are some examples of analytical skills: Critical thinking: The ability to objectively evaluate information and form a reasoned judgment. Data analysis: The process of collecting, organizing, interpreting, and presenting data. Problem-solving: The capacity to identify issues, analyze potential solutions, and implement the most effective ...
When to use thematic analysis. Thematic analysis is a good approach to research where you're trying to find out something about people's views, opinions, knowledge, experiences or values from a set of qualitative data - for example, interview transcripts, social media profiles, or survey responses. Some types of research questions you might use thematic analysis to answer:
Research is vital in any field. It helps in finding out information about various subjects. It is a systematic process of collecting data, documenting critical information, analyzing data, and interpreting it. It employs different methodologies to perform various tasks. Its main task is to collect, compose and analyze data on the subject matter.
Many examples of analytical thinking skills don't involve numbers. You can build your logic and analysis abilities through a variety of capacities, such as: 1. Brainstorming. Using the information in front of you to generate new ideas is a valuable transferable skill that helps you innovate at work.
A subsequent and rigorous inclusion process narrowed the sample down to 19 articles. The combined sample contains 2,776 participants (67% male) and 88 waves of data (average of 4.2). Evidence is mapped according to participants, setting, procedures, outcomes, analytical approach, and key findings.
For example, a marketing analyst might use data analysis methods to determine how successful an advertising campaign recently conducted by the company was. These numbers can also be interpreted so that they provide recommendations for future marketing strategies. Research . Strong research abilities are an essential part of analytical skills ...
Background Many studies have investigated the association between hypothyroidism and frozen shoulder, but their findings have been inconsistent. Furthermore, earlier research has been primarily observational, which may introduce bias and does not establish a cause-and-effect relationship. To ascertain the causal association, we performed a two-sample bidirectional Mendelian randomization (MR ...
Data and sample size. This cross-sectional, descriptive and analytical study was conducted in 2022. The research population was the managers of health sector, both public and private, and international institutions based in Herat province of Afghanistan. The participants were chosen by random sampling.
Non-destructive techniques allow the preservation of the sample mass so that the same sample can be subjected to several analytical procedures and treated for 14 C analysis (Fig. 1, step IV).
Textual analysis is a broad term for various research methods used to describe, interpret and understand texts. All kinds of information can be gleaned from a text - from its literal meaning to the subtext, symbolism, assumptions, and values it reveals. The methods used to conduct textual analysis depend on the field and the aims of the ...
Objectives This study aims to investigate the links between the clinical, demographic, and psychosocial factors and cancer-related worry in patients with early-stage lung cancer after surgery. Methods The study utilized a descriptive cross-sectional design. Questionnaires, including assessments of cancer-related worry, symptom burden, illness perception, psychological resilience, coping modes ...
• Understanding alternate research designs, and methods, including sample selection methods. These methods are unique to the analysis of nutritional factors as either exposures or outcomes, be they in laboratory, clinical or population-based small or large group settings.
A research design is a strategy for answering your research question using empirical data. Creating a research design means making decisions about: Your overall research objectives and approach. Whether you'll rely on primary research or secondary research. Your sampling methods or criteria for selecting subjects. Your data collection methods.