- Skip to secondary menu
- Skip to main content
- Skip to primary sidebar
Statistics By Jim
Making statistics intuitive

Simple Random Sampling: Definition & Examples
By Jim Frost Leave a Comment
What is Simple Random Sampling?
Simple random sampling (SRS) is a probability sampling method where researchers randomly choose participants from a population . All population members have an equal probability of being selected. This method tends to produce representative, unbiased samples.
For example, if you randomly select 1000 people from a town with a population of 100,000 residents, each person has a 1000/100000 = 0.01 probability. That’s a simple calculation requiring no additional knowledge about the population’s composition. Hence, simple random sampling.
Simple random sampling is a probability sampling method that helps ensure the sample mirrors the population. The process proportionately samples from larger subpopulations more frequently than smaller subpopulations.
Suppose the town contains subpopulation A with 40,000 people and subpopulation B with 10,000. Using SRS with a probability of 0.01, the process will tend to enlist 400 from subpopulation A and 100 from B. Hence, the process tends to produce a proportionate representation in the sample that reflects the entire population. You don’t need to know the details about the subpopulations for this process to work!
Learn more about Types of Sampling Methods in Research .
How to Use Simple Random Sampling
Performing simple random sampling requires that you have a sampling frame that contains a complete list of all population members and the ability to contact and involve them in your study. Learn more about Sampling Frames: Definition, Examples & Uses .

- Define the population.
- Create a list of all population members.
- Assign random numbers to each member.
- Use a random number generator to select participants until you reach your target sample size.
Alternatively, if the population is not too large, you can use a lottery system for drawing the sample. Place all the names in a hat and randomly draw your sample. For large populations, researchers typically use computers to select participants randomly from a database.
Example of Simple Random Sampling
Imagine we are studying the town with 100,000 residents. We want to perform simple random sampling to obtain a sample size of 1000. We first need to define the population. We’ll define it as residents of the town who pay township taxes and are at least 18 years old.
Next, we need to create a complete list of residents who meet those criteria. Perhaps we’ll work with the township tax office to make the list. We’ll add all eligible residents to our list.
Finally, we need to select participants randomly from the list. We can use a computer program to do that. Alternatively, we can print out names on slips of paper and draw them from a basket. We keep drawing from the list until we have 1000 names.
Benefits of Simple Random Sampling
Many statisticians consider simple random sampling to be the gold standard for producing representative samples. Because it is entirely random, it minimizes the potential for researchers biasing the results, even if unintentionally. As you’ll read, there are alternative sampling methods that provide concessions to real-world sampling difficulties. Unfortunately, the alternatives can unwittingly produce a biased sample. Learn more about representative samples .
Procedurally, SRS is the simplest method for obtaining an unbiased sample. While the researchers need a list of the entire population, they don’t need other information about that population, its subpopulations, and its features.
Conversely, other more complex forms of sampling require researchers to understand the population’s characteristics. Then, using that knowledge and a lot of preplanning, they divide the population into strata or clusters and perform other procedures before sampling. With SRS, you just randomly draw from the list until you have enough subjects.
Because simple random sampling tends to produce unbiased samples that mirror the population, it’s excellent for analysts who need to use a sample to infer the properties of a population (i.e., inferential statistics ). In a study, having a representative sample improves both its internal and external validity . After simple random sampling, you can use statistical hypothesis tests to use the sample to draw conclusions about the population.
For more information about inferential statistics, read my articles about Populations, Parameters, and Samples in Inferential Statistics and Descriptive versus Inferential Statistics .
Drawbacks of Simple Random Sampling
Even though there are great benefits to using this method, simple random sampling has some significant drawbacks.
Population List
First and foremost, this method can be quite cumbersome and require ample resources for large populations. You’ll need a list of all population members, which can be a tremendous hurdle by itself. If that list doesn’t exist, you might need to expend considerable resources to create it. An incomplete list can bias your results. Only a complete list allows the researchers to have an equal probability of selecting all population members.
Attempting to perform SRS with an incomplete population list causes undercoverage bias and a nonrepresentative sample.
Learn more about Undercoverage Bias: Definition & Examples .
Then you’ll need to contact and interact with everyone you randomly select. Depending on the nature of your study, that process can be pretty expensive and time-consuming if your participants span a wide geographic range, particularly when you need a large sample size.
Insufficient Representation of Subpopulations
Despite being entirely random, simple random sampling can miss important subpopulations and features in the population. For example, in our town with 100,000 residents, imagine that we’re particularly interested in surveying those who are at least 90 years old. You plan to obtain a sample size of 1000, which is 1 out of 100 residents. However, there are only 50 people in town who are older than 90. Your sample might not include anyone in this vital group! If it does, it’ll be a tiny number that doesn’t provide a clear picture of this subgroup.
Simple random sampling can fail to provide precise data about particular subgroups and differences between subgroups. Other sampling methods can ensure sufficient numbers from small subgroups that produce a clear picture and increase the ability to compare subgroups.
Simple Random Sampling vs. Other Methods
Because you need a list of the entire population, simple random sampling is most feasible when working with a relatively small population that is already defined. For example, if you’re surveying a company and can easily obtain a list of employees from Human Resources, SRS isn’t too difficult. Large populations can require extensive amounts of time and resources just to create the complete list. Simple random sampling is a great option when you don’t know much about your population other than its membership.
However, other sampling methods can be more efficient when creating the population list is difficult, your population is large and dispersed, or you need to guarantee sufficient data for specific subpopulations. Alternative methods can reduce the need for a complete list and reduce the logistical headaches of a geographically extensive study.
For example, a national opinion poll company might consider an alternative method to assess differences between subpopulations, such as gender, race, and age.
These other methods frequently require you to have a greater understanding of your population than SRS requires. Consider the following alternatives to simple random sampling that can also obtain representative samples:
- Systematic sampling : Uses a random starting point but then samples at a fixed interval. Does not require a complete population list.
- Stratified sampling: Divides the population into dissimilar strata. Ensures that the sample includes specific subpopulations and facilitates comparisons between them.
- Cluster sampling : Divides the population into clusters that mirror the entire population. Then you randomly select from a subset of clusters. Reduces the need for a complete list of the population and eases logistics issues.
For a contrast to representative sampling methods, learn about convenience sampling , which tends to produce biased samples.
Learn about the specialized random sampling process that Political Polls use, allowing a relatively small sample to predict an election.
Sampling in Developmental Science: Situations, Shortcomings, Solutions, and Standards (nih.gov)
Share this:

Reader Interactions
Comments and questions cancel reply.
- Bipolar Disorder
- Therapy Center
- When To See a Therapist
- Types of Therapy
- Best Online Therapy
- Best Couples Therapy
- Managing Stress
- Sleep and Dreaming
- Understanding Emotions
- Self-Improvement
- Healthy Relationships
- Student Resources
- Personality Types
- Sweepstakes
- Guided Meditations
- Verywell Mind Insights
- 2024 Verywell Mind 25
- Mental Health in the Classroom
- Editorial Process
- Meet Our Review Board
- Crisis Support
What Is a Random Sample in Psychology?
How Subsets of Subjects Are Used for Research
- Choosing a Random Sample
- Pros and Cons
Random sampling is a technique in which each person is equally likely to be selected. Simply put, a random sample is a subset of individuals randomly selected by researchers to represent an entire group. The goal is to get a sample of people representative of the larger population.
It involves determining the target population, determining the number of participants you need, and then picking participants in a way that gives everyone an equal chance of being selected at random.
At a Glance
Random sampling is important in psychology because it helps ensure that research samples are representative of the entire group. This sampling method can reduce bias, but it can also be costly and time-consuming to perform.
Knowing more about how random sampling works can help you in your own studies and give you a better understanding of how research works and what it might mean about the larger population.
Example of Random Sampling in Psychology
For example, if researchers were interested in learning about alcohol use among college students in the United States, the larger population (in other words, the "group of interest"), would be made up of every kid in every college and university in the country.
It would be virtually impossible to interview each and every one of these people to find out if they drink, what types of alcohol they drink, how often, under what circumstances, how much (a beer or two per week versus enough to get intoxicated every weekend), and so forth.
Instead of undertaking such a monumental task, scientists will pull together a random sample of college students to represent the total population of college students.
Types of Random Sampling in Psychology
Random sampling can occur in a few different ways:
- Simple random sampling involves having a list of each member of the target population. Each person is assigned a number, and then the necessary number of participants is randomly chosen using a lottery method.
- Stratified random sampling involves dividing members of the target population into homogenous groups based on specific characteristics of interest (such as age, gender, diagnosis, etc), and then drawing a random sample from each sub-group.
- Cluster random sampling involves dividing the larger population into smaller clusters that are representative of the larger group. Researchers then draw a random number of clusters to be included in the sample.
- Systematic random sampling involves using a systematic sampling method, like selecting every 15th member of the group to be included in the sample.
How Researchers Create Random Samples
Random sampling can be costly and time-consuming. However, this approach to gathering data for research does provide the best chance of putting together an unbiased sample that is truly representative of an entire group as a whole.
Returning to the imaginary study of alcohol use among college students, here's how random sampling might work:
Determine the Total Population
According to the National Center for Education Statistics ( NCES), approximately 19.7 million students were enrolled in U.S. colleges and universities in 2020, the most recent statistics available. These 20 million individuals represent the total population to be studied.
Determine the Characteristics of the Population
For the purpose of drawing a random sample of this group, all students must have an equal chance of being selected. For example, scientists conducting the study would need to make sure that the sample included the same percentage of men and women as the larger population.
According to the NCES statistics, 11.3 million of the total population of college students are female, and 8.5 million are male. The sample group would need to reflect this same ratio of women to men.
Represent All Characteristics In the Random Sample
Besides gender, researchers would also want to go through the same process for other characteristics—for example, race, cultural background, year in school, socioeconomic status, and so forth, depending on the specific purpose of the study.
For instance, if they wanted to home in on alcohol use among Asian students, they would create a random sample consisting only of Asian students. By the same token, if the study were focused on how much students drink during the week, they would create a questionnaire or other method for finding only kids who drink on weekdays for their research.
Pros and Cons of Random Sampling in Psychology
The most significant benefit of random sampling is that it helps reduce the risk of bias. Researchers also suggest that it can improve statistical analysis and allow them to quantify non-responses.
The biggest downside of random sampling is that it can be difficult to obtain.
This type of sampling tends to be more costly in terms of money, time, and resources. Because of this, researchers sometimes have to utilize other sampling methods.
What This Means For You
When you read a health study based on a random sample, be aware that the findings are based not on every single person in the population that fit certain criteria, but on a subset of subjects chosen to represent them. This should help you put the study in perspective.
Elfil M, Negida A. Sampling methods in clinical research: An educational review . Emerg (Tehran) . 2017;5(1):e52.
Bornstein MH, Jager J, Putnick DL. Sampling in developmental science: Situations, shortcomings, solutions, and standards . Dev Rev . 2013;33(4):357-370. doi:10.1016/j.dr.2013.08.003
National Center for Education Statistics. Fast Facts: Back to school statistics .
Pierce M, McManus S, Jessop C, et al. Says who? The significance of sampling in mental health surveys during COVID-19 . Lancet Psychiatry . 2020;7(7):567-568. doi:10.1016/S2215-0366(20)30237-6
By Kendra Cherry, MSEd Kendra Cherry, MS, is a psychosocial rehabilitation specialist, psychology educator, and author of the "Everything Psychology Book."
Randomization in Statistics and Experimental Design
Design of Experiments > Randomization
What is Randomization?
Randomization in an experiment is where you choose your experimental participants randomly . For example, you might use simple random sampling , where participants names are drawn randomly from a pool where everyone has an even probability of being chosen. You can also assign treatments randomly to participants, by assigning random numbers from a random number table.
If you use randomization in your experiments, you guard against bias . For example, selection bias (where some groups are underrepresented) is eliminated and accidental bias (where chance imbalances happen) is minimized. You can also run a variety of statistical tests on your data (to test your hypotheses) if your sample is random.
Randomization Techniques
The word “random” has a very specific meaning in statistics. Arbitrarily choosing names from a list might seem random, but it actually isn’t. Hidden biases (like a subconscious preference for English names, names that sound like friends, or names that roll off the tongue) means that what you think is a random selection probably isn’t. Because these biases are often hidden, or overlooked, specific randomization techniques have been developed for researchers:
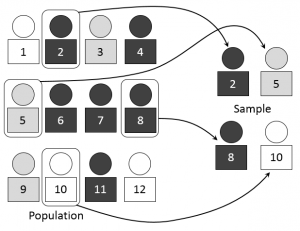
Random Sampling vs. Random Assignment
Random sampling and random assignment are fundamental concepts in the realm of research methods and statistics. However, many students struggle to differentiate between these two concepts, and very often use these terms interchangeably. Here we will explain the distinction between random sampling and random assignment.
Random sampling refers to the method you use to select individuals from the population to participate in your study. In other words, random sampling means that you are randomly selecting individuals from the population to participate in your study. This type of sampling is typically done to help ensure the representativeness of the sample (i.e., external validity). It is worth noting that a sample is only truly random if all individuals in the population have an equal probability of being selected to participate in the study. In practice, very few research studies use “true” random sampling because it is usually not feasible to ensure that all individuals in the population have an equal chance of being selected. For this reason, it is especially important to avoid using the term “random sample” if your study uses a nonprobability sampling method (such as convenience sampling).
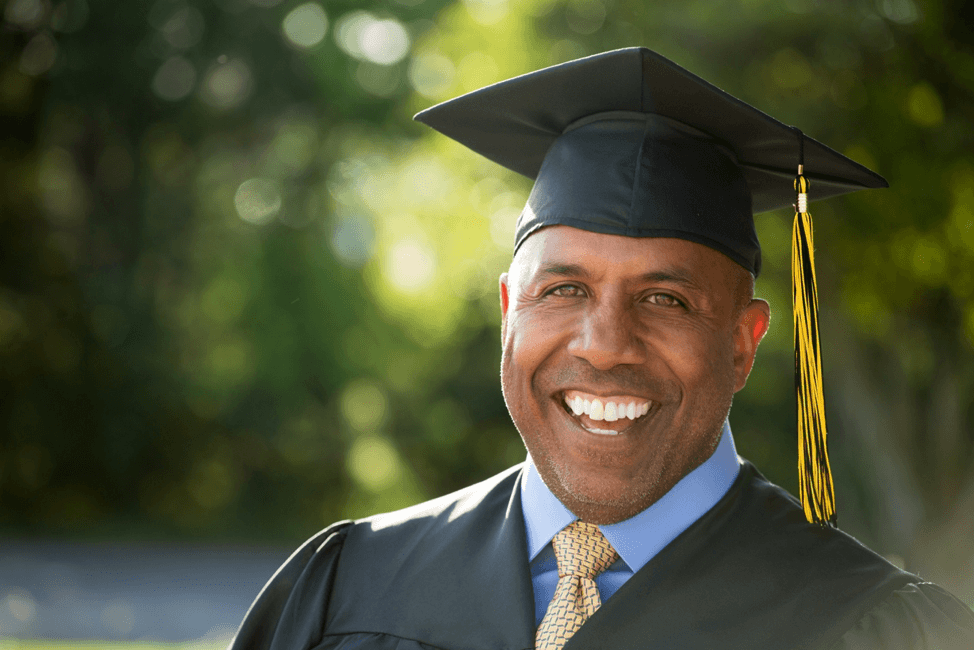
Discover How We Assist to Edit Your Dissertation Chapters
Aligning theoretical framework, gathering articles, synthesizing gaps, articulating a clear methodology and data plan, and writing about the theoretical and practical implications of your research are part of our comprehensive dissertation editing services.
- Bring dissertation editing expertise to chapters 1-5 in timely manner.
- Track all changes, then work with you to bring about scholarly writing.
- Ongoing support to address committee feedback, reducing revisions.
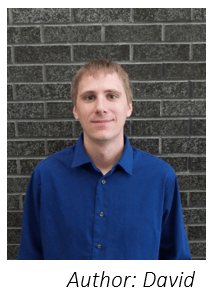
Random assignment refers to the method you use to place participants into groups in an experimental study. For example, say you are conducting a study comparing the blood pressure of patients after taking aspirin or a placebo. You have two groups of patients to compare: patients who will take aspirin (the experimental group) and patients who will take the placebo (the control group). Ideally, you would want to randomly assign the participants to be in the experimental group or the control group, meaning that each participant has an equal probability of being placed in the experimental or control group. This helps ensure that there are no systematic differences between the groups before the treatment (e.g., the aspirin or placebo) is given to the participants. Random assignment is a fundamental part of a “true” experiment because it helps ensure that any differences found between the groups are attributable to the treatment, rather than a confounding variable.
So, to summarize, random sampling refers to how you select individuals from the population to participate in your study. Random assignment refers to how you place those participants into groups (such as experimental vs. control). Knowing this distinction will help you clearly and accurately describe the methods you use to collect your data and conduct your study.
Numbers, Facts and Trends Shaping Your World
Read our research on:
Full Topic List
Regions & Countries
- Publications
- Our Methods
- Short Reads
- Tools & Resources
Read Our Research On:
Methods 101: Random Sampling
The first video in Pew Research Center’s Methods 101 series helps explain random sampling – a concept that lies at the heart of all probability-based survey research – and why it’s important.
Sign up for our weekly newsletter
Fresh data delivered Saturday mornings
901 E St. NW, Suite 300 Washington, DC 20004 USA (+1) 202-419-4300 | Main (+1) 202-857-8562 | Fax (+1) 202-419-4372 | Media Inquiries
Research Topics
- Email Newsletters
ABOUT PEW RESEARCH CENTER Pew Research Center is a nonpartisan, nonadvocacy fact tank that informs the public about the issues, attitudes and trends shaping the world. It does not take policy positions. The Center conducts public opinion polling, demographic research, computational social science research and other data-driven research. Pew Research Center is a subsidiary of The Pew Charitable Trusts , its primary funder.
© 2024 Pew Research Center
Simple Random Sampling
Definition and Different Approaches
Jonathan Kitchen/Getty Images
- Key Concepts
- Major Sociologists
- News & Issues
- Research, Samples, and Statistics
- Recommended Reading
- Archaeology
Simple random sampling is the most basic and common type of sampling method used in quantitative social science research and in scientific research generally . The main benefit of the simple random sample is that each member of the population has an equal chance of being chosen for the study. This means that it guarantees that the sample chosen is representative of the population and that the sample is selected in an unbiased way. In turn, the statistical conclusions drawn from the analysis of the sample will be valid .
There are multiple ways of creating a simple random sample. These include the lottery method, using a random number table, using a computer, and sampling with or without replacement.
Lottery Method of Sampling
The lottery method of creating a simple random sample is exactly what it sounds like. A researcher randomly picks numbers, with each number corresponding to a subject or item, in order to create the sample. To create a sample this way, the researcher must ensure that the numbers are well mixed before selecting the sample population.
Using a Random Number Table
One of the most convenient ways of creating a simple random sample is to use a random number table . These are commonly found at the back of textbooks on the topics of statistics or research methods. Most random number tables will have as many as 10,000 random numbers. These will be composed of integers between zero and nine and arranged in groups of five. These tables are carefully created to ensure that each number is equally probable, so using it is a way to produce a random sample required for valid research outcomes.
To create a simple random sample using a random number table just follow these steps.
- Number each member of the population 1 to N.
- Determine the population size and sample size.
- Select a starting point on the random number table. (The best way to do this is to close your eyes and point randomly onto the page. Whichever number your finger is touching is the number you start with.)
- Choose a direction in which to read (up to down, left to right, or right to left).
- Select the first n numbers (however many numbers are in your sample) whose last X digits are between 0 and N. For instance, if N is a 3 digit number, then X would be 3. Put another way, if your population contained 350 people, you would use numbers from the table whose last 3 digits were between 0 and 350. If the number on the table was 23957, you would not use it because the last 3 digits (957) is greater than 350. You would skip this number and move to the next one. If the number is 84301, you would use it and you would select the person in the population who is assigned the number 301.
- Continue this way through the table until you have selected your entire sample , whatever your n is. The numbers you selected then correspond to the numbers assigned to the members of your population, and those selected become your sample.
Using a Computer
In practice, the lottery method of selecting a random sample can be quite burdensome if done by hand. Typically, the population being studied is large and choosing a random sample by hand would be very time-consuming. Instead, there are several computer programs that can assign numbers and select n random numbers quickly and easily. Many can be found online for free.
Sampling With Replacement
Sampling with replacement is a method of random sampling in which members or items of the population can be chosen more than once for inclusion in the sample. Let’s say we have 100 names each written on a piece of paper. All of those pieces of paper are put into a bowl and mixed up. The researcher picks a name from the bowl, records the information to include that person in the sample, then puts the name back in the bowl, mixes up the names, and selects another piece of paper. The person that was just sampled has the same chance of being selected again. This is known as sampling with replacement.
Sampling Without Replacement
Sampling without replacement is a method of random sampling in which members or items of the population can only be selected one time for inclusion in the sample. Using the same example above, let’s say we put the 100 pieces of paper in a bowl, mix them up, and randomly select one name to include in the sample. This time, however, we record the information to include that person in the sample and then set that piece of paper aside rather than putting it back into the bowl. Here, each element of the population can only be selected one time.
- Understanding Stratified Samples and How to Make Them
- How Systematic Sampling Works
- Cluster Sample in Sociology Research
- Sampling Error
- Understanding Purposive Sampling
- What Is a Quota Sample in Sociology?
- What Is a Snowball Sample in Sociology?
- Chaos Theory
- Convenience Samples for Research
- Visualizing Social Stratification in the U.S.
- The Meaning of "Horticulture"
- Assessing a Situation, in Terms of Sociology
- Definition of a Formal Organization
- How Our Aligning Behavior Shapes Everyday Life
- What Is Game Theory?
- What Is Cultural Capital? Do I Have It?
Simple Random Sampling Method: Definition & Example
Julia Simkus
Editor at Simply Psychology
BA (Hons) Psychology, Princeton University
Julia Simkus is a graduate of Princeton University with a Bachelor of Arts in Psychology. She is currently studying for a Master's Degree in Counseling for Mental Health and Wellness in September 2023. Julia's research has been published in peer reviewed journals.
Learn about our Editorial Process
Saul McLeod, PhD
Editor-in-Chief for Simply Psychology
BSc (Hons) Psychology, MRes, PhD, University of Manchester
Saul McLeod, PhD., is a qualified psychology teacher with over 18 years of experience in further and higher education. He has been published in peer-reviewed journals, including the Journal of Clinical Psychology.
Olivia Guy-Evans, MSc
Associate Editor for Simply Psychology
BSc (Hons) Psychology, MSc Psychology of Education
Olivia Guy-Evans is a writer and associate editor for Simply Psychology. She has previously worked in healthcare and educational sectors.
On This Page:
Simple random sampling is a technique in which each member of a population has an equal chance of being chosen through an unbiased selection method. Each subject in the sample is given a number, and then the sample is chosen randomly.
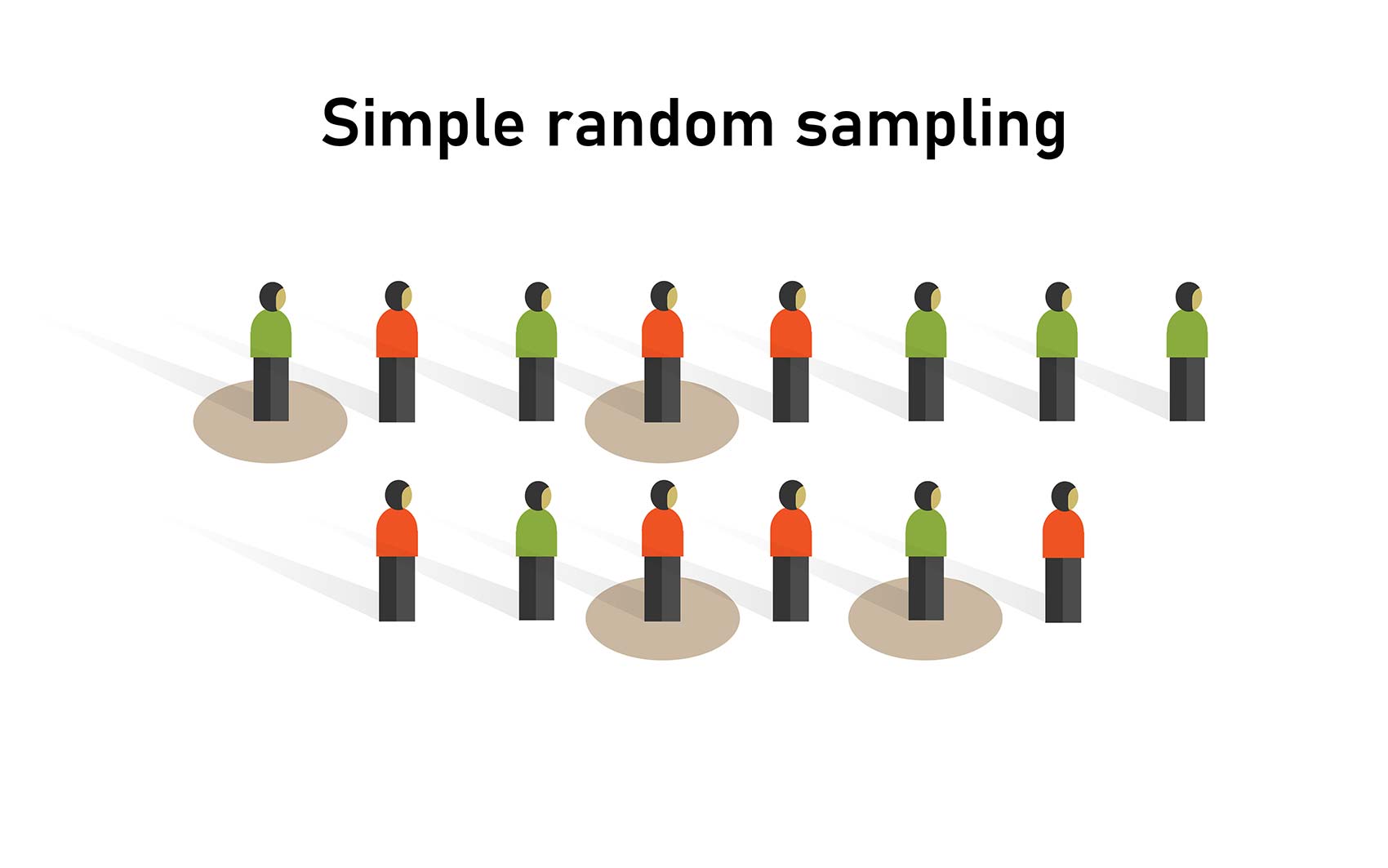
The random sampling method is one of the simplest and most common forms of collecting data, as it provides an unbiased representation of a group. The random subset of selected individuals represents an entire data set.
The goal of simple random sampling is to create a manageable, balanced subset of individuals that is representative of a larger group that would otherwise be too challenging to sample.
For example, if you wanted to conduct a survey about food preferences in a school of 1000 students, and you wanted to sample 100 students.
You could use simple random sampling by assigning each student a number from 1 to 1000, then using a random number generator to pick 100 numbers.
The students assigned those numbers would be the ones you survey.
- First, choose the target population that you wish to study and determine your desired sample size. The smaller the sample size the less likely, it can be generalized to the wider research population and is unlikely to be fully representative.
- The list of the people from which the sample is drawn is called the sampling frame. Examples of sampling frames include the electoral register, schools, drug addicts, etc.).
- Then, assign a sequential number to each subject in the sampling frame.
- Next, individuals are selected using an unbiased selection method. Some examples of simple random sampling techniques include lotteries, random computer number generators, or random draws.
Minimizes Bias
It is the least biased sampling method, as every member of the target population has an equal chance of being chosen. The purpose of simple random sampling is to give each individual an equal chance of being chosen.
This is meant to represent a group that is free from researcher bias. Like any sampling technique, there is room for error, but this method is intended to be an unbiased approach.
Representativeness
Random sampling ensures that every member of the target population has an equal chance of being selected. This helps to ensure that the sample is representative of the population, making it more likely that the findings can be generalized to the entire population.

Limitations
Expensive and time-consuming.
It is a very expensive and time-consuming method; it is difficult to get the name of every member of the target population, especially if it is a very large population, so it is rarely used.
Access to respondents
This is actually quite hard to achieve – especially if the parent population is large. Since the participants do not volunteer to participate, it can be challenging for researchers to gain access to respondents when drawing from a large population.
Sampling error
Sampling errors can occur when the sample does not accurately represent the population as a whole. If this occurs, the researcher would need to restart the sampling process.
Other techniques
There are four types of random sampling techniques (simple, stratified, cluster, and systematic random sampling.
Stratified Random Sampling
- In stratified random sampling , researchers will first divide a population into subgroups, or strata, based on shared characteristics and then randomly select among these groups.
- This method is typically used when a population has distinct differences, such as demographics, level of education, or age, and can easily be broken into subgroups.
Cluster Random Sampling
- Similar to stratified random sampling, cluster random sampling begins by dividing a population into smaller groups.
- However, in cluster sampling, researchers use naturally formed groups to divide a large population up into clusters and then select randomly among the clusters to form the sample.
- Examples of these pre-existing groups could include school districts, city blocks, or households.
Systematic Random Sampling
- Systematic random sampling involves taking random samples at regular periodic intervals.
- For example, if you were conducting a survey in a cafeteria, you could give a survey to every sixth customer that comes into the cafeteria.
- A sample is the participants you select from a target population (the group you are interested in) to make generalizations about. As an entire population tends to be too large to work with, a smaller group of participants must act as a representative sample.
- Representative means the extent to which a sample mirrors a researcher’s target population and reflects its characteristics (e.g., gender, ethnicity, socioeconomic level). In an attempt to select a representative sample and avoid sampling bias (the over-representation of one category of participant in the sample), psychologists utilize various sampling methods.
- Generalisability means the extent to which their findings can be applied to the larger population of which their sample was a part.
Hayes, A. (2021). Simple Random Sample. Investopedia. Retrieved from https://www.investopedia.com/terms/s/simple-random-sample.asp
Simple random sample: Definition and examples. Statistics How To. (n.d.). Retrieved from https://www.statisticshowto.com/probability-and-statistics/statistics-definitions/simple-random-sample/
Simple random sampling: Definition, examples, and how to do it. Qualtrics. (2022). Retrieved from https://www.qualtrics.com/experience-management/research/simple-random-sampling/ nce-management/research/simple-random-sampling/


An official website of the United States government
The .gov means it’s official. Federal government websites often end in .gov or .mil. Before sharing sensitive information, make sure you’re on a federal government site.
The site is secure. The https:// ensures that you are connecting to the official website and that any information you provide is encrypted and transmitted securely.
- Publications
- Account settings
Preview improvements coming to the PMC website in October 2024. Learn More or Try it out now .
- Advanced Search
- Journal List
- Emerg (Tehran)
- v.5(1); 2017

Sampling methods in Clinical Research; an Educational Review
Mohamed elfil.
1 Faculty of Medicine, Alexandria University, Egypt.
Ahmed Negida
2 Faculty of Medicine, Zagazig University, Egypt.
Clinical research usually involves patients with a certain disease or a condition. The generalizability of clinical research findings is based on multiple factors related to the internal and external validity of the research methods. The main methodological issue that influences the generalizability of clinical research findings is the sampling method. In this educational article, we are explaining the different sampling methods in clinical research.
Introduction
In clinical research, we define the population as a group of people who share a common character or a condition, usually the disease. If we are conducting a study on patients with ischemic stroke, it will be difficult to include the whole population of ischemic stroke all over the world. It is difficult to locate the whole population everywhere and to have access to all the population. Therefore, the practical approach in clinical research is to include a part of this population, called “sample population”. The whole population is sometimes called “target population” while the sample population is called “study population. When doing a research study, we should consider the sample to be representative to the target population, as much as possible, with the least possible error and without substitution or incompleteness. The process of selecting a sample population from the target population is called the “sampling method”.
Sampling types
There are two major categories of sampling methods ( figure 1 ): 1; probability sampling methods where all subjects in the target population have equal chances to be selected in the sample [ 1 , 2 ] and 2; non-probability sampling methods where the sample population is selected in a non-systematic process that does not guarantee equal chances for each subject in the target population [ 2 , 3 ]. Samples which were selected using probability sampling methods are more representatives of the target population.

Sampling methods.
Probability sampling method
Simple random sampling
This method is used when the whole population is accessible and the investigators have a list of all subjects in this target population. The list of all subjects in this population is called the “sampling frame”. From this list, we draw a random sample using lottery method or using a computer generated random list [ 4 ].
Stratified random sampling
This method is a modification of the simple random sampling therefore, it requires the condition of sampling frame being available, as well. However, in this method, the whole population is divided into homogeneous strata or subgroups according a demographic factor (e.g. gender, age, religion, socio-economic level, education, or diagnosis etc.). Then, the researchers select draw a random sample from the different strata [ 3 , 4 ]. The advantages of this method are: (1) it allows researchers to obtain an effect size from each strata separately, as if it was a different study. Therefore, the between group differences become apparent, and (2) it allows obtaining samples from minority/under-represented populations. If the researchers used the simple random sampling, the minority population will remain underrepresented in the sample, as well. Simply, because the simple random method usually represents the whole target population. In such case, investigators can better use the stratified random sample to obtain adequate samples from all strata in the population.
Systematic random sampling (Interval sampling)
In this method, the investigators select subjects to be included in the sample based on a systematic rule, using a fixed interval. For example: If the rule is to include the last patient from every 5 patients. We will include patients with these numbers (5, 10, 15, 20, 25, ...etc.). In some situations, it is not necessary to have the sampling frame if there is a specific hospital or center which the patients are visiting regularly. In this case, the researcher can start randomly and then systemically chooses next patients using a fixed interval [ 4 ].
Cluster sampling (Multistage sampling)
It is used when creating a sampling frame is nearly impossible due to the large size of the population. In this method, the population is divided by geographic location into clusters. A list of all clusters is made and investigators draw a random number of clusters to be included. Then, they list all individuals within these clusters, and run another turn of random selection to get a final random sample exactly as simple random sampling. This method is called multistage because the selection passed with two stages: firstly, the selection of eligible clusters, then, the selection of sample from individuals of these clusters. An example for this, if we are conducting a research project on primary school students from Iran. It will be very difficult to get a list of all primary school students all over the country. In this case, a list of primary schools is made and the researcher randomly picks up a number of schools, then pick a random sample from the eligible schools [ 3 ].
Non-probability sampling method
Convenience sampling
Although it is a non-probability sampling method, it is the most applicable and widely used method in clinical research. In this method, the investigators enroll subjects according to their availability and accessibility. Therefore, this method is quick, inexpensive, and convenient. It is called convenient sampling as the researcher selects the sample elements according to their convenient accessibility and proximity [ 3 , 6 ]. For example: assume that we will perform a cohort study on Egyptian patients with Hepatitis C (HCV) virus. The convenience sample here will be confined to the accessible population for the research team. Accessible population are HCV patients attending in Zagazig University Hospital and Cairo University Hospitals. Therefore, within the study period, all patients attending these two hospitals and meet the eligibility criteria will be included in this study.
Judgmental sampling
In this method, the subjects are selected by the choice of the investigators. The researcher assumes specific characteristics for the sample (e.g. male/female ratio = 2/1) and therefore, they judge the sample to be suitable for representing the population. This method is widely criticized due to the likelihood of bias by investigator judgement [ 5 ].
Snow-ball sampling
This method is used when the population cannot be located in a specific place and therefore, it is different to access this population. In this method, the investigator asks each subject to give him access to his colleagues from the same population. This situation is common in social science research, for example, if we running a survey on street children, there will be no list with the homeless children and it will be difficult to locate this population in one place e.g. a school/hospital. Here, the investigators will deliver the survey to one child then, ask him to take them to his colleagues or deliver the surveys to them.
Conflict of interest:
Have a language expert improve your writing
Run a free plagiarism check in 10 minutes, automatically generate references for free.
- Knowledge Base
- Methodology
- Simple Random Sampling | Definition, Steps & Examples
Simple Random Sampling | Definition, Steps & Examples
Published on 3 May 2022 by Lauren Thomas . Revised on 18 December 2023.
A simple random sample is a randomly selected subset of a population . In this sampling method, each member of the population has an exactly equal chance of being selected, minimising the risk of selection bias .
This method is the most straightforward of all the probability sampling methods , since it only involves a single random selection and requires little advance knowledge about the population. Because it uses randomisation, any research performed on this sample should have high internal and external validity.
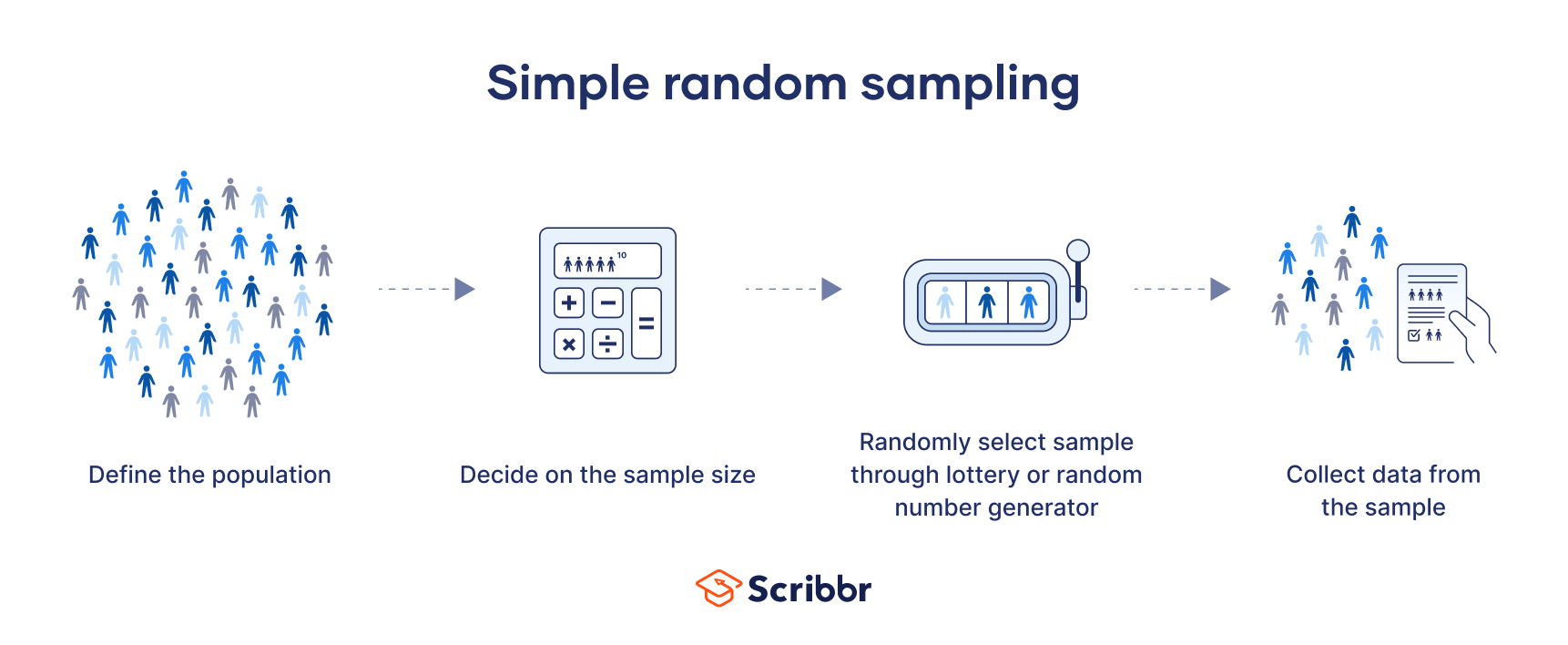
Table of contents
When to use simple random sampling, how to perform simple random sampling, frequently asked questions about simple random sampling.
Simple random sampling is used to make statistical inferences about a population. It helps ensure high internal validity : randomisation is the best method to reduce the impact of potential confounding variables .
In addition, with a large enough sample size, a simple random sample has high external validity : it represents the characteristics of the larger population.
However, simple random sampling can be challenging to implement in practice. To use this method, there are some prerequisites:
- You have a complete list of every member of the population.
- You can contact or access each member of the population if they are selected.
- You have the time and resources to collect data from the necessary sample size.
Simple random sampling works best if you have a lot of time and resources to conduct your study, or if you are studying a limited population that can easily be sampled.
In some cases, it might be more appropriate to use a different type of probability sampling:
- Systematic sampling involves choosing your sample based on a regular interval, rather than a fully random selection. It can also be used when you don’t have a complete list of the population.
- Stratified sampling is appropriate when you want to ensure that specific characteristics are proportionally represented in the sample. You split your population into strata (for example, divided by gender or race), and then randomly select from each of these subgroups.
- Cluster sampling is appropriate when you are unable to sample from the entire population. You divide the sample into clusters that approximately reflect the whole population, and then choose your sample from a random selection of these clusters.
Prevent plagiarism, run a free check.
There are four key steps to select a simple random sample.
Step 1: Define the population
Start by deciding on the population that you want to study.
It’s important to ensure that you have access to every individual member of the population, so that you can collect data from all those who are selected for the sample.
Step 2: Decide on the sample size
Next, you need to decide how large your sample size will be. Although larger samples provide more statistical certainty, they also cost more and require far more work.
There are several potential ways to decide upon the size of your sample, but one of the simplest involves using a formula with your desired confidence interval and confidence level , estimated size of the population you are working with, and the standard deviation of whatever you want to measure in your population.
The most common confidence interval and levels used are 0.05 and 0.95, respectively. Since you may not know the standard deviation of the population you are studying, you should choose a number high enough to account for a variety of possibilities (such as 0.5).
You can then use a sample size calculator to estimate the necessary sample size.
Step 3: Randomly select your sample
This can be done in one of two ways: the lottery or random number method.
In the lottery method , you choose the sample at random by ‘drawing from a hat’ or by using a computer program that will simulate the same action.
In the random number method , you assign every individual a number. By using a random number generator or random number tables, you then randomly pick a subset of the population. You can also use the random number function (RAND) in Microsoft Excel to generate random numbers.
Step 4: Collect data from your sample
Finally, you should collect data from your sample.
To ensure the validity of your findings, you need to make sure every individual selected actually participates in your study. If some drop out or do not participate for reasons associated with the question that you’re studying, this could bias your findings.
For example, if young participants are systematically less likely to participate in your study, your findings might not be valid due to the underrepresentation of this group.
Probability sampling means that every member of the target population has a known chance of being included in the sample.
Probability sampling methods include simple random sampling , systematic sampling , stratified sampling , and cluster sampling .
Simple random sampling is a type of probability sampling in which the researcher randomly selects a subset of participants from a population . Each member of the population has an equal chance of being selected. Data are then collected from as large a percentage as possible of this random subset.
The American Community Survey is an example of simple random sampling . In order to collect detailed data on the population of the US, the Census Bureau officials randomly select 3.5 million households per year and use a variety of methods to convince them to fill out the survey.
If properly implemented, simple random sampling is usually the best sampling method for ensuring both internal and external validity . However, it can sometimes be impractical and expensive to implement, depending on the size of the population to be studied,
If you have a list of every member of the population and the ability to reach whichever members are selected, you can use simple random sampling.
Samples are used to make inferences about populations . Samples are easier to collect data from because they are practical, cost-effective, convenient, and manageable.
Sampling bias occurs when some members of a population are systematically more likely to be selected in a sample than others.
Cite this Scribbr article
If you want to cite this source, you can copy and paste the citation or click the ‘Cite this Scribbr article’ button to automatically add the citation to our free Reference Generator.
Thomas, L. (2023, December 18). Simple Random Sampling | Definition, Steps & Examples. Scribbr. Retrieved 9 September 2024, from https://www.scribbr.co.uk/research-methods/simple-random-sampling-method/
Is this article helpful?
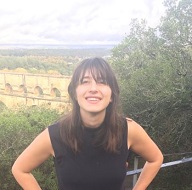
Lauren Thomas
Other students also liked, sampling methods | types, techniques, & examples, a quick guide to experimental design | 5 steps & examples, what is quantitative research | definition & methods.
1.6 Sampling Experiment
Sampling experiment.
- The student will demonstrate the simple random, systematic, stratified, and cluster sampling techniques.
- The student will explain the details of each procedure used.
In this lab, you will be asked to pick several random samples of restaurants. In each case, describe your procedure briefly, including how you might have used the random number generator, and then list the restaurants in the sample you obtained.
The following section contains restaurants stratified by city into columns and grouped horizontally by entree cost (clusters).
Entree Cost | Under $10 | $10 to under $15 | $15 to under $20 | Over $20 |
---|---|---|---|---|
San Jose | El Abuelo Taq, Pasta Mia, Emma’s Express, Bamboo Hut | Emperor’s Guard, Creekside Inn | Agenda, Gervais, Miro’s | Blake’s, Eulipia, Hayes Mansion, Germania |
Palo Alto | Senor Taco, Tuscan Garden, Taxi’s | Ming’s, P.A. Joe’s, Stickney’s | Scott’s Seafood, Poolside Grill, Fish Market | Sundance Mine, Maddalena’s, Sally's |
Los Gatos | Mary’s Patio, Mount Everest, Sweet Pea’s, Andele Taqueria | Lindsey’s, Willow Street | Toll House | Charter House, La Maison Du Cafe |
Mountain View | Maharaja, New Ma’s, Thai-Rific, Garden Fresh | Amber Indian, La Fiesta, Fiesta del Mar, Dawit | Austin’s, Shiva’s, Mazeh | Le Petit Bistro |
Cupertino | Hobees, Hung Fu, Samrat, China Express | Santa Barb. Grill, Mand. Gourmet, Bombay Oven, Kathmandu West | Fontana’s, Blue Pheasant | Hamasushi, Helios |
Sunnyvale | Chekijababi, Taj India, Full Throttle, Tia Juana, Lemon Grass | Pacific Fresh, Charley Brown’s, Cafe Cameroon, Faz, Aruba’s | Lion & Compass, The Palace, Beau Sejour | |
Santa Clara | Rangoli, Armadillo Willy’s, Thai Pepper, Pasand | Arthur’s, Katie’s Cafe, Pedro’s, La Galleria | Birk’s, Truya Sushi, Valley Plaza | Lakeside, Mariani’s |
A Simple Random Sample Pick a simple random sample of 15 restaurants.
- Describe your procedure.
1. __________ | 6. __________ | 11. __________ |
2. __________ | 7. __________ | 12. __________ |
3. __________ | 8. __________ | 13. __________ |
4. __________ | 9. __________ | 14. __________ |
5. __________ | 10. __________ | 15. __________ |
A Systematic Sample Pick a systematic sample of 15 restaurants.
1. __________ | 6. __________ | 11. __________ |
2. __________ | 7. __________ | 12. __________ |
3. __________ | 8. __________ | 13. __________ |
4. __________ | 9. __________ | 14. __________ |
5. __________ | 10. __________ | 15. __________ |
A Stratified Sample Pick a stratified sample , by city, of 20 restaurants. Use 25 percent of the restaurants from each stratum. Round to the nearest whole number.
1. __________ | 6. __________ | 11. __________ | 16. __________ |
2. __________ | 7. __________ | 12. __________ | 17. __________ |
3. __________ | 8. __________ | 13. __________ | 18. __________ |
4. __________ | 9. __________ | 14. __________ | 19. __________ |
5. __________ | 10. __________ | 15. __________ | 20. __________ |
A Stratified Sample Pick a stratified sample , by entree cost, of 21 restaurants. Use 25 percent of the restaurants from each stratum. Round to the nearest whole number.
1. __________ | 6. __________ | 11. __________ | 16. __________ |
2. __________ | 7. __________ | 12. __________ | 17. __________ |
3. __________ | 8. __________ | 13. __________ | 18. __________ |
4. __________ | 9. __________ | 14. __________ | 19. __________ |
5. __________ | 10. __________ | 15. __________ | 20. __________ |
21. __________ |
A Cluster Sample Pick a cluster sample of restaurants from two cities. The number of restaurants will vary.
1. ________ | 6. ________ | 11. ________ | 16. ________ | 21. ________ |
2. ________ | 7. ________ | 12. ________ | 17. ________ | 22. ________ |
3. ________ | 8. ________ | 13. ________ | 18. ________ | 23. ________ |
4. ________ | 9. ________ | 14. ________ | 19. ________ | 24. ________ |
5. ________ | 10. ________ | 15. ________ | 20. ________ | 25. ________ |
This book may not be used in the training of large language models or otherwise be ingested into large language models or generative AI offerings without OpenStax's permission.
Want to cite, share, or modify this book? This book uses the Creative Commons Attribution License and you must attribute Texas Education Agency (TEA). The original material is available at: https://www.texasgateway.org/book/tea-statistics . Changes were made to the original material, including updates to art, structure, and other content updates.
Access for free at https://openstax.org/books/statistics/pages/1-introduction
- Authors: Barbara Illowsky, Susan Dean
- Publisher/website: OpenStax
- Book title: Statistics
- Publication date: Mar 27, 2020
- Location: Houston, Texas
- Book URL: https://openstax.org/books/statistics/pages/1-introduction
- Section URL: https://openstax.org/books/statistics/pages/1-6-sampling-experiment
© Apr 16, 2024 Texas Education Agency (TEA). The OpenStax name, OpenStax logo, OpenStax book covers, OpenStax CNX name, and OpenStax CNX logo are not subject to the Creative Commons license and may not be reproduced without the prior and express written consent of Rice University.
Have a language expert improve your writing
Run a free plagiarism check in 10 minutes, generate accurate citations for free.
- Knowledge Base
Methodology
- Stratified Sampling | Definition, Guide & Examples
Stratified Sampling | Definition, Guide & Examples
Published on September 18, 2020 by Lauren Thomas . Revised on June 22, 2023.
In a stratified sample , researchers divide a population into homogeneous subpopulations called strata (the plural of stratum) based on specific characteristics (e.g., race, gender identity, location, etc.). Every member of the population studied should be in exactly one stratum.
Each stratum is then sampled using another probability sampling method, such as cluster sampling or simple random sampling , allowing researchers to estimate statistical measures for each sub-population.
Researchers rely on stratified sampling when a population’s characteristics are diverse and they want to ensure that every characteristic is properly represented in the sample. This helps with the generalizability and validity of the study, as well as avoiding research biases like undercoverage bias .
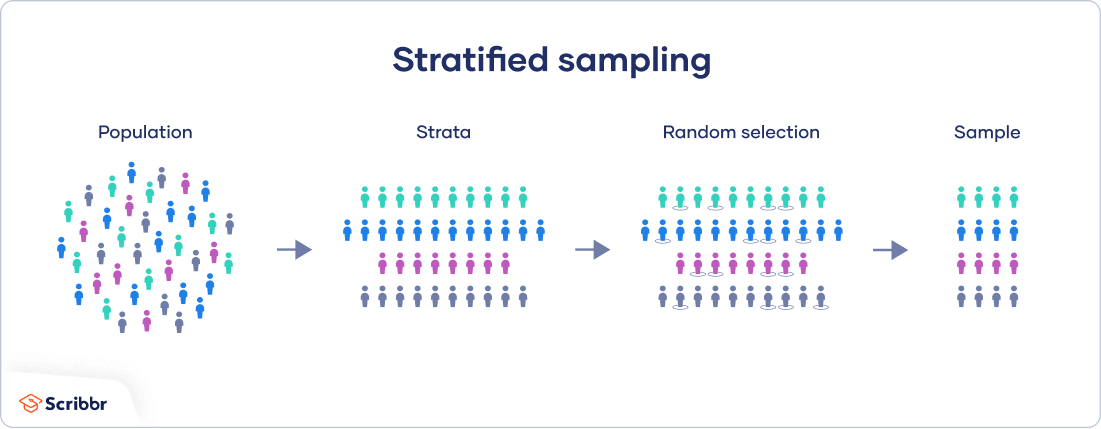
Table of contents
When to use stratified sampling, step 1: define your population and subgroups, step 2: separate the population into strata, step 3: decide on the sample size for each stratum, step 4: randomly sample from each stratum, other interesting articles, frequently asked questions about stratified sampling.
To use stratified sampling, you need to be able to divide your population into mutually exclusive and exhaustive subgroups. That means every member of the population can be clearly classified into exactly one subgroup.
Stratified sampling is the best choice among the probability sampling methods when you believe that subgroups will have different mean values for the variable(s) you’re studying. It has several potential advantages:
Ensuring the diversity of your sample
A stratified sample includes subjects from every subgroup, ensuring that it reflects the diversity of your population. It is theoretically possible (albeit unlikely) that this would not happen when using other sampling methods such as simple random sampling .
Ensuring similar variance
If you want the data collected from each subgroup to have a similar level of variance , you need a similar sample size for each subgroup.
With other methods of sampling, you might end up with a low sample size for certain subgroups because they’re less common in the overall population.
Lowering the overall variance in the population
Although your overall population can be quite heterogeneous, it may be more homogenous within certain subgroups.
For example, if you are studying how a new schooling program affects the test scores of children, both their original scores and any change in scores will most likely be highly correlated with family income. The scores are likely to be grouped by family income category.
In this case, stratified sampling allows for more precise measures of the variables you wish to study, with lower variance within each subgroup and therefore for the population as a whole.
Allowing for a variety of data collection methods
Sometimes you may need to use different methods to collect data from different subgroups.
For example, in order to lower the cost and difficulty of your study, you may want to sample urban subjects by going door-to-door, but rural subjects using mail.
Because only a small proportion of this university’s graduates have obtained a doctoral degree, using a simple random sample would likely give you a sample size too small to properly compare the differences between men, women, and those who do not identify as men or women with a doctoral degree versus those without one.
Here's why students love Scribbr's proofreading services
Discover proofreading & editing
Like other methods of probability sampling , you should begin by clearly defining the population from which your sample will be taken.
Choosing characteristics for stratification
You must also choose the characteristic that you will use to divide your groups. This choice is very important: since each member of the population can only be placed in only one subgroup, the classification of each subject to each subgroup should be clear and obvious.
Stratifying by multiple characteristics
You can choose to stratify by multiple different characteristics at once, so long as you can clearly match every subject to exactly one subgroup. In this case, to get the total number of subgroups, you multiply the numbers of strata for each characteristic.
For instance, if you were stratifying by both race and gender identity, using four groups for the former and three for the latter, you would have 4 x 3 = 12 groups in total.
Next, collect a list of every member of the population, and assign each member to a stratum.
You must ensure that each stratum is mutually exclusive (there is no overlap between them), but that together, they contain the entire population.
Combining these characteristics, you have nine groups in total. Each graduate must be assigned to exactly one group.
Characteristic | Strata | Groups |
---|---|---|
First, you need to decide whether you want your sample to be proportionate or disproportionate.
Proportionate versus disproportionate sampling
In proportionate sampling, the sample size of each stratum is equal to the subgroup’s proportion in the population as a whole.
Subgroups that are less represented in the greater population (for example, rural populations, which make up a lower portion of the population in most countries) will also be less represented in the sample.
In disproportionate sampling, the sample sizes of each strata are disproportionate to their representation in the population as a whole.
You might choose this method if you wish to study a particularly underrepresented subgroup whose sample size would otherwise be too low to allow you to draw any statistical conclusions.
Sample size
Next, you can decide on your total sample size. This should be large enough to ensure you can draw statistical conclusions about each subgroup.
If you know your desired margin of error and confidence level as well as estimated size and standard deviation of the population you are working with, you can use a sample size calculator to estimate the necessary numbers.
Prevent plagiarism. Run a free check.
Finally, you should use another probability sampling method , such as simple random or systematic sampling , to sample from within each stratum.
If properly done, the randomization inherent in such methods will allow you to obtain a sample that is representative of that particular subgroup.
If you want to know more about statistics , methodology , or research bias , make sure to check out some of our other articles with explanations and examples.
- Student’s t -distribution
- Normal distribution
- Null and Alternative Hypotheses
- Chi square tests
- Confidence interval
- Quartiles & Quantiles
- Cluster sampling
- Data cleansing
- Reproducibility vs Replicability
- Peer review
- Prospective cohort study
Research bias
- Implicit bias
- Cognitive bias
- Placebo effect
- Hawthorne effect
- Hindsight bias
- Affect heuristic
- Social desirability bias
Probability sampling means that every member of the target population has a known chance of being included in the sample.
Probability sampling methods include simple random sampling , systematic sampling , stratified sampling , and cluster sampling .
In stratified sampling , researchers divide subjects into subgroups called strata based on characteristics that they share (e.g., race, gender, educational attainment).
Once divided, each subgroup is randomly sampled using another probability sampling method.
You should use stratified sampling when your sample can be divided into mutually exclusive and exhaustive subgroups that you believe will take on different mean values for the variable that you’re studying.
Using stratified sampling will allow you to obtain more precise (with lower variance ) statistical estimates of whatever you are trying to measure.
For example, say you want to investigate how income differs based on educational attainment, but you know that this relationship can vary based on race. Using stratified sampling, you can ensure you obtain a large enough sample from each racial group, allowing you to draw more precise conclusions.
Yes, you can create a stratified sample using multiple characteristics, but you must ensure that every participant in your study belongs to one and only one subgroup. In this case, you multiply the numbers of subgroups for each characteristic to get the total number of groups.
For example, if you were stratifying by location with three subgroups (urban, rural, or suburban) and marital status with five subgroups (single, divorced, widowed, married, or partnered), you would have 3 x 5 = 15 subgroups.
Cite this Scribbr article
If you want to cite this source, you can copy and paste the citation or click the “Cite this Scribbr article” button to automatically add the citation to our free Citation Generator.
Thomas, L. (2023, June 22). Stratified Sampling | Definition, Guide & Examples. Scribbr. Retrieved September 11, 2024, from https://www.scribbr.com/methodology/stratified-sampling/
Is this article helpful?
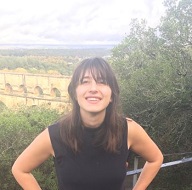
Lauren Thomas
Other students also liked, sampling methods | types, techniques & examples, simple random sampling | definition, steps & examples, cluster sampling | a simple step-by-step guide with examples, what is your plagiarism score.

IMAGES
VIDEO
COMMENTS
When to use simple random sampling. Simple random sampling is used to make statistical inferences about a population. It helps ensure high internal validity: randomization is the best method to reduce the impact of potential confounding variables.. In addition, with a large enough sample size, a simple random sample has high external validity: it represents the characteristics of the larger ...
Random sampling (also called probability sampling or random selection) is a way of selecting members of a population to be included in your study. In contrast, random assignment is a way of sorting the sample participants into control and experimental groups. While random sampling is used in many types of studies, random assignment is only used ...
Simple random sampling (SRS) is a probability sampling method where researchers randomly choose participants from a population. All population members have an equal probability of being selected. This method tends to produce representative, unbiased samples. For example, if you randomly select 1000 people from a town with a population of ...
Probability sampling is a sampling method that involves randomly selecting a sample, or a part of the population that you want to research. It is also sometimes called random sampling. To qualify as being random, each research unit (e.g., person, business, or organization in your population) must have an equal chance of being selected.
Sampling methods in psychology refer to strategies used to select a subset of individuals (a sample) from a larger population, to study and draw inferences about the entire population. Common methods include random sampling, stratified sampling, cluster sampling, and convenience sampling. Proper sampling ensures representative, generalizable, and valid research results.
Random sampling is a technique in which each person is equally likely to be selected. Simply put, a random sample is a subset of individuals randomly selected by researchers to represent an entire group. The goal is to get a sample of people representative of the larger population. It involves determining the target population, determining the ...
Interpret the experiment as a sampling experiment. Answer. The parameter is \( n \), the number of cards dealt. At each stage, we draw a card from a deck, but the deck changes from one draw to the next, so the stages are dependent. The experiment is to select a sample of size \( n \) from the population \(D\), without replacement.
Random selection (also called probability sampling or random sampling) is a way of randomly selecting members of a population to be included in your study. On the other hand, random assignment is a way of sorting the sample participants into control and treatment groups. Random selection ensures that everyone in the population has an equal ...
Sampling Experiment. Class Time: Names: Student Learning Outcomes. The student will demonstrate the simple random, systematic, stratified, and cluster sampling techniques. ... A Simple Random Sample Pick a simple random sample of 15 restaurants. Describe your procedure. Complete the table with your sample. 1. _____ 6. _____
Randomization in an experiment is where you choose your experimental participants randomly. For example, you might use simple random sampling, where participants names are drawn randomly from a pool where everyone has an even probability of being chosen. You can also assign treatments randomly to participants, by assigning random numbers from a ...
Definition 1.2.2 1.2. 2. Stratified sampling is where you break the population into groups called strata, then take a simple random sample from each strata. For example: If you want to look at musical preference, you could divide the individuals into age groups and then conduct simple random samples inside each group.
Random sampling and random assignment are fundamental concepts in the realm of research methods and statistics. However, many students struggle to differentiate between these two concepts, and very often use these terms interchangeably. ... is given to the participants. Random assignment is a fundamental part of a "true" experiment because ...
Methods 101: Random Sampling. The first video in Pew Research Center's Methods 101 series helps explain random sampling - a concept that lies at the heart of all probability-based survey research - and why it's important. Random Sampling. Survey Question Wording. Mode effects.
To create a simple random sample using a random number table just follow these steps. Number each member of the population 1 to N. Determine the population size and sample size. Select a starting point on the random number table. (The best way to do this is to close your eyes and point randomly onto the page.
The sample space of a random experiment is the collection of all possible outcomes. An event associated with a random experiment is a subset of the sample space. The probability of any outcome is a number between \(0\) and \(1\). The probabilities of all the outcomes add up to \(1\).
Limitations. Other techniques. Simple random sampling is a technique in which each member of a population has an equal chance of being chosen through an unbiased selection method. Each subject in the sample is given a number, and then the sample is chosen randomly. This method is considered "simple" because it's straightforward and ...
Sampling types. There are two major categories of sampling methods (figure 1): 1; probability sampling methods where all subjects in the target population have equal chances to be selected in the sample [1, 2] and 2; non-probability sampling methods where the sample population is selected in a non-systematic process that does not guarantee ...
When to use simple random sampling. Simple random sampling is used to make statistical inferences about a population. It helps ensure high internal validity: randomisation is the best method to reduce the impact of potential confounding variables.. In addition, with a large enough sample size, a simple random sample has high external validity: it represents the characteristics of the larger ...
RANDOM SAMPLING AND. RANDOM ASSIGNMENT MADE EASY! Research Randomizer is a free resource for researchers and students in need of a quick way to generate random numbers or assign participants to experimental conditions. This site can be used for a variety of purposes, including psychology experiments, medical trials, and survey research.
Stratified Design. Setup: Number of units, n Number of blocks, J Block size, nj > 2 Number of treated in each block, n1j > 1 Complete randomization within each block, Pr(Tij = 1) = n1j=nj. Analysis: 1 Apply Neyman's analysis to each block. nj. 1 X ^j = TijYij n1j i=1. nj. 1 X (1.
Introduction; 9.1 Null and Alternative Hypotheses; 9.2 Outcomes and the Type I and Type II Errors; 9.3 Distribution Needed for Hypothesis Testing; 9.4 Rare Events, the Sample, and the Decision and Conclusion; 9.5 Additional Information and Full Hypothesis Test Examples; 9.6 Hypothesis Testing of a Single Mean and Single Proportion; Key Terms; Chapter Review; Formula Review
Probability sampling methods help ensure that your sample doesn't systematically differ from the population. In addition, if you're doing an experiment, use random assignment to place participants into different treatment conditions.
Table of contents. When to use stratified sampling. Step 1: Define your population and subgroups. Step 2: Separate the population into strata. Step 3: Decide on the sample size for each stratum. Step 4: Randomly sample from each stratum. Other interesting articles.