- Digital Marketing
- Facebook Marketing
- Instagram Marketing
- Ecommerce Marketing
- Content Marketing
- Data Science Certification
- Machine Learning
- Artificial Intelligence
- Data Analytics
- Graphic Design
- Adobe Illustrator
- Web Designing
- UX UI Design
- Interior Design
- Front End Development
- Back End Development Courses
- Business Analytics
- Entrepreneurship
- Supply Chain
- Financial Modeling
- Corporate Finance
- Project Finance
- Harvard University
- Stanford University
- Yale University
- Princeton University
- Duke University
- UC Berkeley
- Harvard University Executive Programs
- MIT Executive Programs
- Stanford University Executive Programs
- Oxford University Executive Programs
- Cambridge University Executive Programs
- Yale University Executive Programs
- Kellog Executive Programs
- CMU Executive Programs
- 45000+ Free Courses
- Free Certification Courses
- Free DigitalDefynd Certificate
- Free Harvard University Courses
- Free MIT Courses
- Free Excel Courses
- Free Google Courses
- Free Finance Courses
- Free Coding Courses
- Free Digital Marketing Courses

AI in Banking [10 Case Studies] [2024]
In the rapidly altering finance landscape, AI has emerged as a pivotal significance, extending banks’ abilities and reshaping traditional financial patterns. From enhancing customer experiences to mitigating financial risks, AI’s role in banking is pivotal and transformative. This exploration delves into ten distinct case studies where leading banks have successfully implemented AI to address complex challenges in the industry. These examples showcase AI’s innovative applications and highlight its potential to revolutionize banking operations, improve customer service, and bolster financial security. As we navigate through these case studies, we gain insights into the strategic advantages and practical impacts of AI in the banking sector, underscoring its importance in shaping the future of finance.
Related: High-Paying Banking Jobs & Career Paths
Case Study 1: JP Morgan Chase: Streamlining Loan Approvals
The traditional loan approval process is notoriously cumbersome and slow, heavily reliant on manual data handling. This results in prolonged wait times, leading to significant customer dissatisfaction and increasing operational costs due to the extensive need for human oversight and intervention.
To address these inefficiencies, JP Morgan Chase has implemented an advanced AI system that automates key aspects of the loan approval process. This system utilizes machine learning to swiftly and accurately analyze various data points, including applicants’ credit history, recent transaction data, and current financial behaviors. Doing so enhances the speed and accuracy of creditworthiness assessments, reduces reliance on manual processes, and improves overall customer experience by expediting loan approvals.
Overall Impact:
- Increased Speed: Â Loan processing times have dramatically reduced from days to minutes and hours.
- Enhanced Customer Satisfaction: Â Faster loan approvals increase customer satisfaction and loyalty.
- Cost Efficiency: Â Reduced reliance on manual processes decreases operation expenses and improves profitability.
- Scalable Operations: Â The bank can handle more loan applications without significantly increasing staff or resources.
Key Learnings:
- Process Efficiency: Â AI drastically cuts down the time required for loan approvals.
- Operational Cost Reduction: Â Automation reduces the labor-intensive elements of loan processing.
- Enhanced Risk Management: Â AI provides a more accurate and comprehensive loan risk assessment.
- Customer Retention: Â Improved process speeds and accuracy improve customer retention rates.
Future Prospects:
AI algorithms could be enhanced for faster processing, achieving near-instant approval times. Future iterations may further integrate broader economic indicators to refine credit risk assessments, enhancing personalized lending strategies.
Case Study 2: Bank of America: Erica, the AI-Powered Financial Assistant
As digital banking gains traction, customer expectations are also evolving. Users now demand personalized services on-demand and easily accessible through their digital devices. This shift has pushed banks to find innovative solutions to meet these new customer demands without compromising service quality.
Bank of America responded to this digital shift by launching Erica, an AI-driven virtual assistant designed to enhance the mobile banking experience. Accessible via mobile apps, Erica offers a wide range of functionalities that cater to the modern banking customer’s needs. These include handling transaction queries, updating credit reports, and providing proactive financial advice. Erica’s capabilities are powered by sophisticated algorithms that analyze user behavior and large datasets, enabling customized and efficient service that meets the high expectations of today’s bank customers.
- Personalized Customer Interaction: Â Erica offers tailored banking advice, enhancing user engagement.
- Increased Accessibility: Â Round-the-clock availability allows customers to receive instant assistance without waiting for human help.
- Data-Driven Insights: Â Erica provides insights based on a deep analysis of user transactions and behaviors, helping customers manage their finances better.
- Operational Efficiency:  The AI assistant handles regular inquiries, leaving humans to deal with more complex issues.
- Enhanced User Experience: Â AI-driven tools like Erica improve customer experience by providing quick, personalized service.
- Operational Scalability: Â AI can manage increasing volumes of consumer interactions without additional human resources.
- Proactive Service: Â AI enables proactive engagement, offering financial advice and alerts that can prevent issues before they arise.
- Customer Data Utilization: Â Using AI to analyze customer data effectively can lead to more accurate and useful financial advice.
Erica could develop more sophisticated natural language processing capabilities to manage increasingly complex inquiries and transactions. Integration with IoT devices and other platforms may offer holistic financial management solutions, extending personalized services beyond traditional banking.
Case Study 3: HSBC: Enhancing Anti-Money Laundering Efforts
Money laundering remains a formidable challenge for financial institutions worldwide. Traditional systems designed to detect such activities often struggle under modern financial transactions’ heavy volume and complex nature. These systems can be overwhelmed, resulting in undetected fraudulent activities and significant regulatory penalties for banks.
In response, HSBC has integrated an AI-driven system to bolster its anti-money laundering (AML) efforts. This advanced system employs sophisticated machine learning algorithms to analyze many real-time transactions. By detecting unusual patterns and potential illegal activities, the system can far more effectively differentiate between normal and suspicious activities than traditional methods. This AI-enhanced approach allows HSBC to address the complexities of modern financial crime while improving compliance and reducing the risk of oversight.
- Improved Detection Rates: Â The AI system has significantly increased the detection of suspicious transactions, reducing the risk of financial crimes.
- Reduced False Positives: Â Enhanced accuracy in distinguishing legitimate from suspicious activities, minimizing disruptions to innocent customers.
- Compliance Efficiency: Â AI assists in maintaining compliance with evolving regulatory requirements, adapting more quickly to new rules.
- Cost Reduction: Â Automating surveillance reduces the need for extensive manual review teams, lowering operational costs.
- Accuracy in Surveillance: Â AI technologies improve the accuracy and efficiency of financial monitoring systems.
- Adaptive Compliance: Â AI can adapt quickly to new regulatory changes, aiding compliance efforts.
- Resource Optimization: Â Implementing AI reduces the need for large human oversight teams, optimizing resource use.
Future developments may incorporate predictive analytics to detect and predict laundering schemes before they are fully enacted. Integration with international finance monitoring systems could enhance global compliance and tracking capabilities.
Related: Is Banking a stressful job?
Case Study 4: Citibank: Optimizing Customer Service with AI Chatbots
In the fast-paced banking world, high demand for customer service can lead to long wait times and inconsistent service experiences. Such delays and variability often detract from customer satisfaction and can negatively impact customer retention rates. As digital interactions become the norm, banks face the challenge of maintaining high service standards while managing large volumes of customer inquiries efficiently.
Citibank has implemented AI-powered chatbots across its digital platforms to address this challenge. These chatbots are arranged to address a spectrum of consumer inquiries, offer real-time support, and efficiently settle typical issues. By deploying these AI chatbots, Citibank ensures a uniform and agile consumer service experience. The chatbots are equipped to understand and process user queries quickly, offering solutions and guidance instantaneously. This technology reduces the burden on human customer service representatives and enhances overall customer satisfaction by providing timely and reliable support.
- Enhanced Customer Service: Â Immediate response to inquiries improves customer satisfaction.
- 24/7 Availability: Â Customers receive help anytime without needing human agent availability.
- Consistent Experience: Â AI ensures that every customer interaction is handled uniformly, enhancing service reliability.
- Operational Savings: Â The chatbots handle routine inquiries, decreasing the workload on human client service agents and decreasing operational costs.
- Service Accessibility: Â AI tools can provide constant and consistent consumer service.
- Cost Efficiency: Â Automating routine interactions can significantly reduce customer service costs.
- Customer Engagement: Â Real-time interactions facilitated by AI can boost customer engagement and loyalty.
AI chatbots could evolve to handle more sophisticated negotiations and problem-solving tasks, further reducing the need for human intervention. Future versions might seamlessly integrate into omnichannel customer service strategies, providing a unified interface across all banking platforms.
Case Study 5: Santander: Predictive Analytics for Loan Default Prevention
Loan defaults pose a great financial risk to banks, affecting their profits and stability. Traditional risk assessment models often fall short in accurately predicting defaults before they occur, primarily because they may not account for dynamic changes in customers’ financial situations or broader economic trends. This limitation leads to unexpected financial losses and inefficient allocation of resources for risk management.
Santander has adopted a proactive approach to this challenge by integrating predictive analytics models powered by AI into its risk management strategy. These models use a combination of historical data analysis and real-time monitoring of account behaviors to detect early warning signs of potential loan defaults. By identifying at-risk customers before defaults occur, Santander can engage with them to offer tailored financial advice, restructuring options, or other support measures. This early intervention helps mitigate risks associated with loan defaults and improves the bank’s and its customers’ overall financial health.
- Reduced Default Rates: Â Early identification and intervention have led to a decrease in loan defaults.
- Enhanced Customer Support: Â At-risk customers receive tailored advice and restructuring options, improving financial outcomes.
- Operational Efficiency: Â The bank optimizes resource allocation by focusing efforts where they are needed the most.
- Improved Risk Management: Â Better predictive capabilities allow for more accurate risk pricing and reserve allocation.
- Proactive Risk Management: Â Early detection of potential defaults enables more effective mitigation strategies.
- Customer Retention: Â Proactive engagement helps maintain customer relationships and loyalty.
- Financial Health: Â Improved risk assessment contributes to the bank’s overall financial health and stability.
- Resource Allocation: Â AI enables more targeted and efficient use of resources in risk management activities.
Integrating wider socio-economic data could improve predictive models, offering even more precise forecasts of potential defaults. These enhancements allow customized intervention strategies tailored to individual customer profiles and economic conditions.
Case Study 6: Wells Fargo: Fraud Detection Enhancement
Real-time fraud detection in financial transactions presents a major challenge, as traditional methods often lag behind fraudsters’ sophisticated techniques. Wells Fargo faced significant challenges in effectively identifying and preventing fraudulent activities. Their traditional systems struggled to keep up without mistakenly flagging legitimate transactions as fraudulent, leading to customer dissatisfaction and operational inefficiencies.
To address this issue, Wells Fargo implemented an AI-based fraud detection system employing deep learning algorithms to scrutinize real-time transaction patterns. This advanced system is designed to compare each transaction against an extensive database of known fraudulent behaviors, enhancing its ability to make accurate assessments instantly. By doing so, the system significantly improves fraud detection accuracy, minimizing false positives and ensuring that legitimate customer transactions are not disrupted. This method boosts security and enhances the overall customer experience by minimizing delays and errors in transaction processing.
- Improved Fraud Detection: The AI system has a higher accuracy rate in identifying fraudulent transactions, reducing the incidence of fraud.
- Minimized Customer Disruption: Accurate fraud detection means fewer legitimate transactions are flagged incorrectly, ensuring smoother customer experiences.
- Enhanced Security: The system enhances overall transaction security, giving customers greater confidence in using Wells Fargo’s services.
- Cost Efficiency: Decreased fraud incidence reduces financial losses and related costs for the bank.
- Real-Time Processing: AI can process and analyze real-time transactions, offering immediate fraud alerts.
- Data Utilization: Leveraging large datasets enhances the system’s ability to identify and learn from emerging fraud patterns.
- Customer Trust: Improved security measures boost customer trust and satisfaction.
Wells Fargo plans to integrate further enhancements into the AI system, such as adaptive learning capabilities that can evolve with changing fraud tactics. This will allow for even more dynamic and robust fraud prevention mechanisms.
Case Study 7: Barclays: Streamlining Wealth Management
Barclays faced challenges in meeting the high expectations of its high net-worth clients who demand personalized, efficient wealth management services. Traditional methods were slow and often ineffective in providing the customization and rapid service these clients expected, leading to dissatisfaction and operational inefficiencies.
Barclays introduced an AI-driven platform to transform its wealth management services. This platform uses advanced analytics to deeply understand individual client preferences and performance, enabling tailored investment advice and automated portfolio adjustments. This automation enhances service speed and accuracy, improving client satisfaction and streamlining operations.
- Personalized Service: Clients receive highly customized investment advice, improving satisfaction and engagement.
- Increased Efficiency: The AI platform automates routine portfolio management tasks, freeing up advisors to focus on client relationships.
- Better Investment Performance: AI-enhanced analytics provide deeper insights into market trends, aiding better investment decisions.
- Scalability: The platform can efficiently manage many portfolios, scaling as the client base grows.
- Enhanced Customization: AI enables a high degree of personalization in delivering services. This technology tailors interactions to meet individual user needs effectively.
- Advisor Efficiency: Automating routine tasks allows wealth managers to focus more on strategic client interaction.
- Data-Driven Decisions: Utilizing AI for data analysis improves the accuracy and timeliness of investment decisions.
Barclays intends to refine its AI capabilities further, incorporating more comprehensive data sources, including global economic indicators and social trends, to enhance investment strategy recommendations.
Related: Banking Cybersecurity Case Studies
Case Study 8: Deutsche Bank: Optimizing Credit Card Fraud Detection
Credit card fraud poses a major problem for banks, resulting in annual losses amounting to millions and eroding customer trust. This persistent issue challenges financial institutions to enhance their security measures and maintain client confidence. Deutsche Bank faced the challenge of rapidly identifying and mitigating fraudulent credit card activities without affecting genuine transactions.
Deutsche Bank implemented an AI-based solution specifically designed to improve credit card fraud detection. This solution uses advanced machine learning models to monitor and analyze real-time credit card transactions. The system can quickly identify anomalies that suggest fraudulent activity by learning from historical transaction data and continuously adapting to new fraud patterns.
- Increased Detection Accuracy: The AI system significantly enhances the ability to spot fraudulent transactions, reducing financial losses.
- Enhanced Customer Trust: Customers feel more secure using their credit cards, knowing that advanced measures are in place to protect them.
- Operational Efficiency: The automated system allows for faster response times and reduces the workload on manual review teams.
- Reduced False Positives: The system effectively minimizes disruptions to innocent customers by accurately distinguishing between legitimate and fraudulent activities.
- Adaptive Learning: Machine learning models adapting to new data and evolving fraud tactics are more effective than static models.
- Customer Experience: Maintaining a balance between aggressive fraud detection and customer convenience is crucial for customer satisfaction.
- Security as a Priority: Investing in advanced security measures like AI protects the bank’s assets and builds customer loyalty.
Deutsche Bank plans to integrate more granular behavioral analytics to refine the system’s accuracy further. Additionally, collaborating with global financial networks to share fraud intelligence could enhance the system’s predictive capabilities, setting a new standard for fraud prevention in the banking industry.
Case Study 9: Credit Suisse: Enhancing Mortgage Underwriting with AI
Credit Suisse encountered significant challenges in its mortgage underwriting process, which relied heavily on manual input, making it both time-consuming and prone to creating backlogs of applications. This inefficient process delayed loan disbursals and negatively impacted customer satisfaction, as clients experienced lengthy wait times and unpredictable service levels. Streamlining this process was crucial to improving operational efficiency and maintaining customer trust and loyalty.
Credit Suisse adopted an AI-driven approach to transform its mortgage underwriting process. The AI system uses machine learning to assess applicant data such as income, credit score, employment history, market trends, and property evaluations more quickly and accurately than manual methods. This automation allows for faster decision-making and more precise risk assessment.
- Faster Processing Times: The time taken to approve mortgages has been significantly reduced, enhancing customer satisfaction.
- Increased Accuracy: AI provides more accurate assessments of applicant risk profiles, reducing the likelihood of loan defaults.
- Operational Efficiency: Automating routine tasks allows human underwriters to concentrate on handling more complex cases. This shift frees up valuable resources for more critical and detailed work.
- Scalable Underwriting Capacity: The system can handle more applications without additional staff.
- Automation in Risk Assessment: The use of AI for processing and analyzing complex applicant data streamlines risk assessment.
- Improved Customer Experience: Reducing wait times for loan approvals directly impacts customer satisfaction positively.
- Enhanced Decision Making: AI tools provide a deeper insight into potential risks and applicant credibility, aiding better decision-making.
Credit Suisse plans to further enhance the capabilities of its AI system by integrating it with real-time economic indicators and more detailed applicant lifestyle data to predict future financial stability more accurately. This advancement aims to streamline the process and tailor mortgage products more specifically to individual needs, setting a new standard in personalized banking services.
Case Study 10: Standard Chartered: Streamlining Trade Finance Operations
Standard Chartered faced complexities in managing trade finance operations, which involve extensive documentation and verification processes that are traditionally manual and error-prone. These challenges resulted in slow transaction times and higher operational costs, affecting client satisfaction and competitiveness in the global market.
Standard Chartered introduced an AI-driven platform designed to automate and enhance the efficiency of its trade finance operations. Utilizing sophisticated machine learning algorithms, the platform efficiently verifies documents, authenticates data, and streamlines the entire approval process for trade transactions. This integration of advanced technology ensures faster, more accurate handling of the complex documentation and regulatory requirements inherent in trade finance, improving overall transaction speed and reliability. By automating these key steps, the bank has significantly reduced manual errors and sped up the processing of trade finance operations.
- Reduced Processing Time: Transaction times for trade finance operations have been drastically reduced, increasing client satisfaction and transaction volumes.
- Decreased Operational Costs: Automation has minimized the need for extensive manual intervention, significantly cutting operational costs.
- Enhanced Accuracy: The AI system provides a higher level of precision in document verification and data authentication, decreasing the risk of fraud and errors.
- Improved Compliance: The system ensures better adherence to international trade regulations through accurate and automated compliance checks.
- Efficiency through Automation: Automating complex, repetitive tasks can significantly enhance efficiency and accuracy in high-stakes financial operations.
- Client Satisfaction: Quicker processing times and fewer errors directly enhance client relationships and contribute to business expansion.
- Regulatory Compliance: AI tools are vital in ensuring compliance with the continuously changing international trade laws. They help organizations adapt quickly to regulatory updates, maintaining legal integrity across global operations.
Standard Chartered is looking to expand its AI capabilities to include predictive analytics for assessing the potential risks and opportunities in trade finance. Further integration with blockchain technology could enhance security and transparency in international trade transactions, setting new industry standards for efficiency and trust.
Related: Will Banking jobs be Automated?
The integration of AI in banking, as demonstrated through these ten case studies, marks a significant leap toward a more efficient, secure, and customer-centric future in finance. Banks like JP Morgan Chase, Bank of America, HSBC, Citibank, and Santander are at the forefront, harnessing AI to enhance decision-making, streamline operations, and enrich customer interactions. These cases vividly illustrate how AI can effectively address traditional banking challenges, driving significant service delivery and risk management improvements. As the banking industry continues to evolve, the strategic deployment of AI will not only be a competitive advantage but a necessity, paving the way for innovative solutions that meet the complex demands of modern finance.
- What is Sentient AI [Pros & Cons][Deep Analysis] [2024]
- AI in Product Development [5 Case Studies] [2024]
Team DigitalDefynd
We help you find the best courses, certifications, and tutorials online. Hundreds of experts come together to handpick these recommendations based on decades of collective experience. So far we have served 4 Million+ satisfied learners and counting.
10 Ways AI is Being Used in Agriculture [2024]

How can AI be used by a Financial Planning Professional? [10 Ways] [2024]

Top 20 AI Marketing Interview Questions & Answers [2024]

AI in Home Automation: 5 Case Studies [2024]

10 Ways AI is Being Used to Help People Stay Fit [2024]

10 Ways AI Is Being Used in Speakers [2024]
Case Study: How a global bank increased cross-selling effectiveness and realized a significant increase on commercial performance
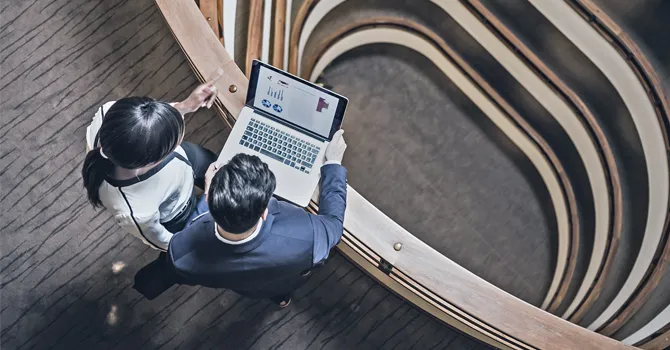
Data analysis, strategic digital tools, and nudges can enhance the commercial effectiveness of bank relationship managers when up-selling and cross-selling high value products and services.
Cross-selling is a critical component of growth in banking. Cross-selling and upselling increases customer lifetime value, generates revenues at low incremental cost, and deepens the customer relationship and loyalty to the institution.
However, the ability to cross-sell effectively and efficiently can be challenging especially with new regulations, tighter controls, and fewer in-person interactions after the COVID-19 pandemic.
A leading global bank was startled to see a steady decline in performance from efforts to cross-sell high-value products like funds, investments, and life insurance to clients after the pandemic.
High-value financial products can be especially hard to sell as they are usually complex, come with a lot of technicalities, and require specialized financial knowledge. Its a buying process that requires effort and focus, and people tend to put it off. Communications of such products on digital channels have to be accessible, and the customer journey simple and barrier-free. Additionally, the role of a knowledgeable and trusted advisor is pivotal to help clients navigate some of these barriers.
How can the bank improve the way it cross-sells and up-sells high-value, complex financial products in an increasingly digital, post-pandemic world?
Leveraging data, analytics and behavioral science, Simon-Kucher introduced a modern, systematic approach to help this bank optimize sales interactions, improve the client experience, and empower its sales and relationship managers.
Identifying buying barriers - There are a number of psychological barriers that are particularly relevant when it comes to derailing a buying decision in financial products and services. These include decision-fatigue, it-can-wait thinking, status-quo bias, and more. As an important first step, Simon-Kucher conducted market tests to validate hypotheses on the most relevant cognitive barriers impacting the high-value financial products offered by the bank.
Sales process audit - To determine if the bank's sales team and sales processes are properly aligned with the buying decisioning process, Simon-Kucher conducted a thorough assessment, audit, and analysis. This methodological process to identify areas for improvement included observing how digital journeys were structured, how information was communicated on digital channels, and how sales were typically conducted paying close attention to the relationship manager's selling speech, what marketing material were available as aids and which ones were used during interactions with clients.
Optimizing sales performance - Another critical element to improve the performance of cross-selling efforts is to leverage optimized digital journeys, selling tools, nudges, and other strategies to enhance sales interactions. For example, working closely with Simon-Kucher, the bank developed pre-defined, interactive scripts to train and guide communications with the client. Communications on digital channels and tools for relationship managers were supported with nudging elements to ensure they help clients progress through the buying journey with recalling mechanisms, anchoring, and default options. There were also mechanisms to ensure the managers followed-up with clients, used approachable language to replace jargon when explaining concepts, and employed examples and simple logics to reduce complexity.
Testing and validation - Testing and validation is an important step in any product development lifecycle to evaluate if the new sales processes are functioning as intended and to ensure quality. Simon-Kucher's rigorous validation testing protocols ensures only the most effective solutions are implemented, and critical business elements are performing.Â
Optimized communication, new behavioral tools and an improved sales process were able to improve the client experience while increasing their commercial effectiveness, to realize a 50 percent increase on conversion rate.
Visit our BE Digital landing page to read more.
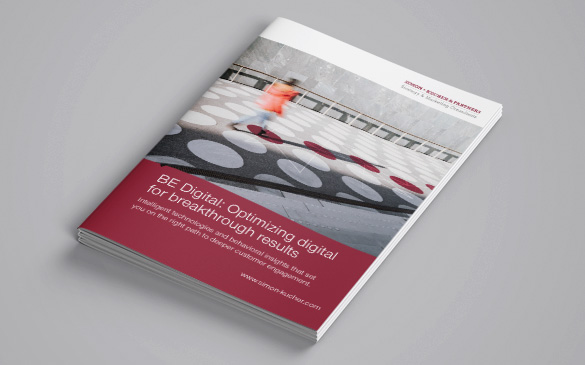
Optimizing digital for breakthrough results.
Related Insights
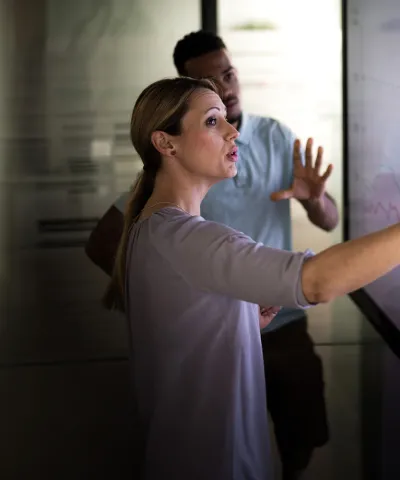
Sales incentives to turbo charge growth for a global fintech
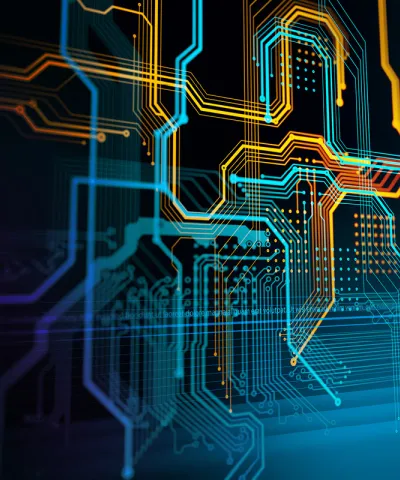
Decoding the Current State of Industrial IoT
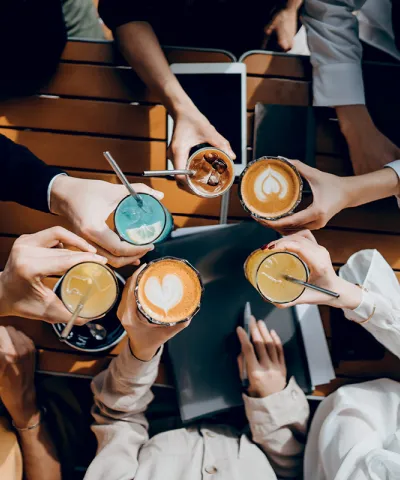
What’s Driving Beverage Trends in 2024?
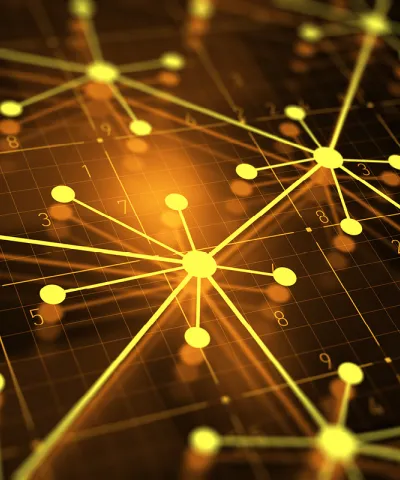
Value Monetization in the Age of AI
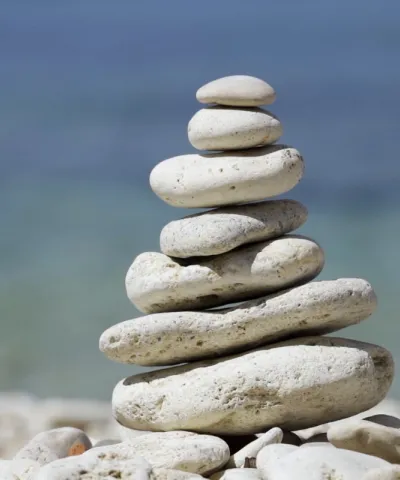
Understanding the Pricing Power of PEOs
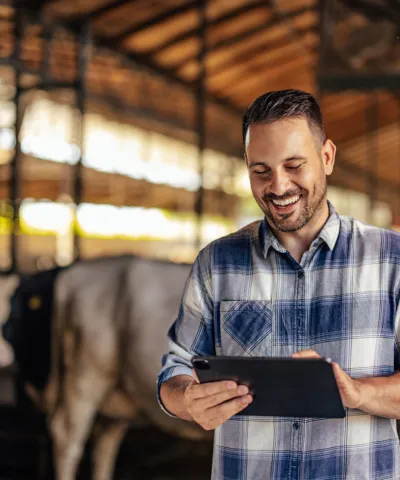
Where tech meets better animal welfare and management
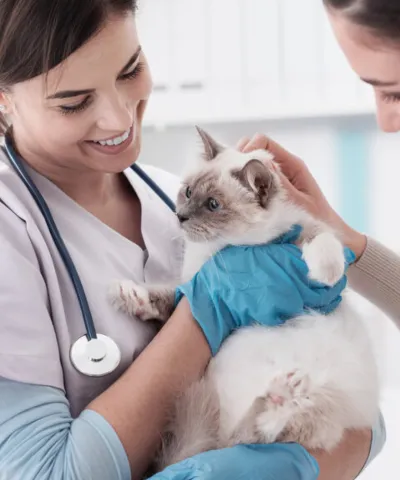
International Key Account Management drives growth for animal health company
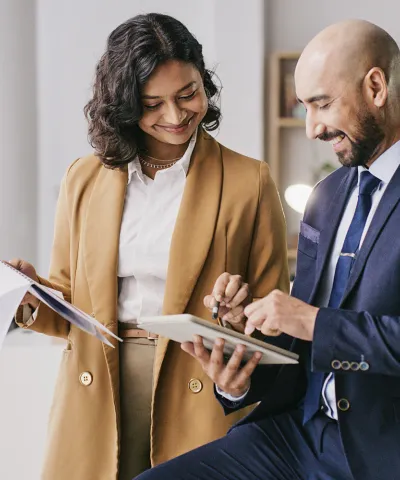
Maximizing up- and cross-sell opportunities for a package tour operator
Our experts are always happy to discuss your issue. Reach out, and we’ll connect you with a member of our team.
- Global | EN
- Global | DE
- Global | FR
- Global | CN
- Global | JA
- Starting Your Business How To Create a Full-Fledged Ecommerce Website: 7 Steps October 25, 2022
- Starting Your Business Omnichannel E-commerce: What You Need to Succeed October 28, 2022
- Starting Your Business The Guide To Opening An Online Retail Store [With 8 Steps To Follow] October 28, 2022
Digital transformation in banking: A complete guide
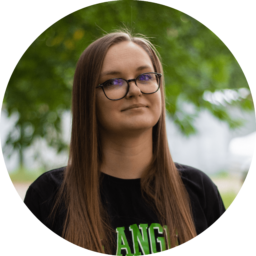
August 02, 2023
Digital transformation is a challenge for the banking industry, but it is necessary to adapt to the modern world where customers expect fast, efficient, and convenient services. Traditional approaches no longer meet the needs of the modern consumer. So, banks that want to remain competitive must abandon conservative methods and fully immerse themselves in the process of digital transformation.
This article discusses what is digital transformation in banking, key factors driving digital transformation , and successful examples of digital transformation in the banking industry.
What is digital transformation in banking?
5 key factors driving digital transformation in banking, technologies that drive digital transformation in banking, successful examples of digital transformation in the banking industry, how can soloway tech help you digitally transform your business.
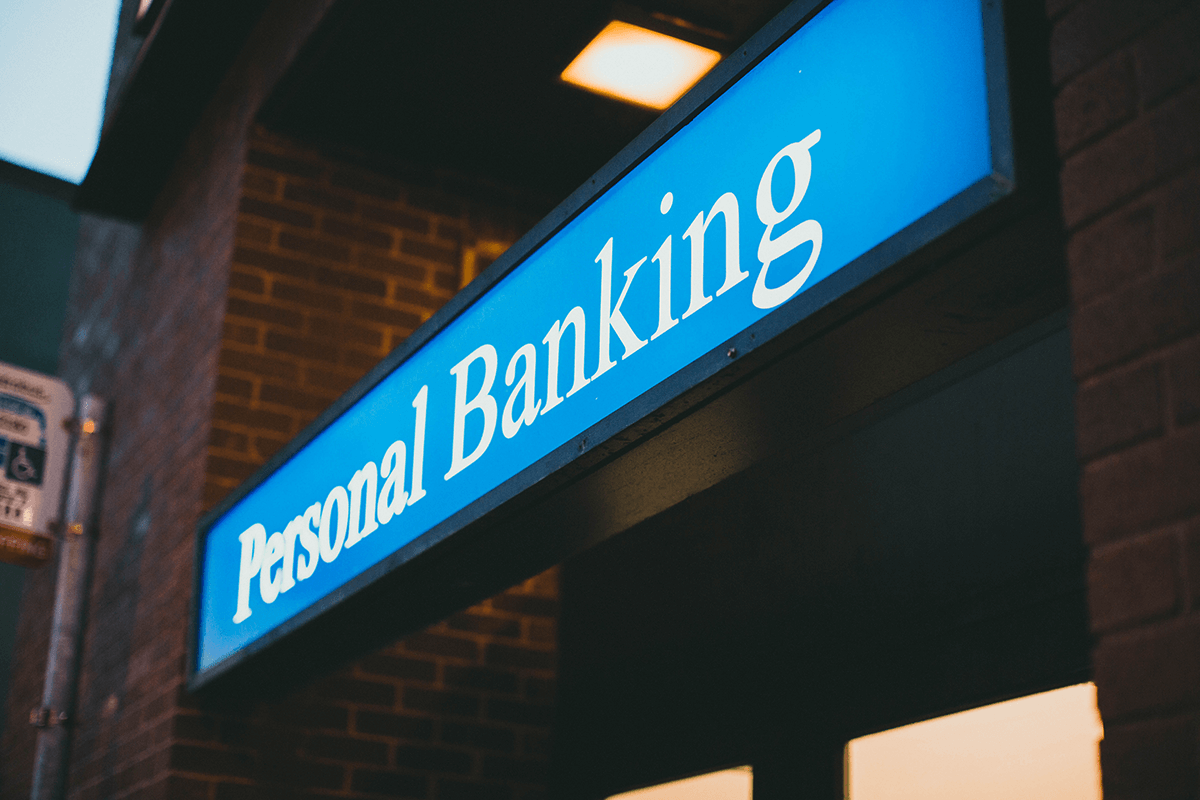
Digital transformation in banking refers to applying new digital technologies and strategies to change and improve banking operations. This includes various changes to increase efficiency, meet customer needs, improve operational effectiveness, and develop new digital products and services.
Mobile applications and personal cabinets on the website are vivid examples of banks’ digital transformation. It is enough to press the buttons on a smartphone or computer to open an account, take out a loan, or order a new plastic card. The services are available not only to individuals but also to legal entities. The accounting departments use client-bank programs to transfer salaries to employees, pay taxes, and receive money from customers.
When you call the hotline of your financial organization, you are answered not by a specialist but by a robot. Virtual assistants have replaced some employees. Moreover, some US banks operate without branches at all. There are employees only in the head office, and customer transactions are conducted exclusively through the Internet.
It is more convenient for people to work with banks remotely, so credit institutions invest a lot of money in digital transformation. It is important that the interface of applications is user-friendly and understandable and transactions are fast. This will attract more customers and, accordingly, increase the profits of the financial company.
Pros and cons of digital transformation in banking
Digital transformation in banking is developing at a rapid pace. It has objective advantages:
- Services of financial organizations are available from anywhere in the world
- The cost of remote operations is cheaper
- There are no queues
- Improved customer service
- Improved operational efficiency
- Big Data and analytics
- Innovation and new opportunities
But there are disadvantages too:
- Dependence on the Internet
- Vulnerability of security systems and regular hacker attacks
- Inaccessibility for some customers
- Threat of job losses
- Dependence on technology
Technology should become a tool that will give banks more flexibility in decision-making and reduce risks.
Fintech companies, which have recently created large-scale services with significantly more interaction points with the client than the classic banking business, are taking the lead. Given that over the last 10 years, the banking industry has experienced a serious tightening of regulatory requirements, fintech is becoming a severe competitor for banks. The solution that banks have found is to change their business model with a focus on digitalization, create their own ecosystems, and develop non-financial services.
Ecosystems are a new global standard for business development and a major stage in the development of the economy. They aggregate data on producers and consumers and help optimize the resources of both. There is no turning back. Creating ecosystems seems to be a common vertical integration strategy for banks when related businesses are pulled up to the core business.
We highlight 5 key factors driving digital transformation in banking:
- Customer experience. Providing convenience and personalization for customers is a crucial factor in digital transformation. Banks should develop and implement innovative digital channels, such as mobile apps, online banking, chatbots, and others, to facilitate access to financial services and improve customer satisfaction.
- Automation and process optimization. The use of automation technologies, such as robo-advisors, machine learning, and artificial intelligence, helps reduce routine operations, lower costs, and improve efficiency. This can include automating lending, foreign exchange, internal audit, and more.
- Evolving regulatory landscape. Regulatory changes and initiatives have pushed banks to adopt digital transformation. Open banking regulations, data protection regulations (such as GDPR), and initiatives promoting competition and innovation have compelled banks to invest in technology to comply with regulations, foster innovation, and enhance transparency.
- Competitive pressure. Fintech startups and tech giants have disrupted the traditional banking landscape. These non-traditional players offer innovative and agile financial services, posing a competitive threat to traditional banks. To remain competitive, banks invest in digital technologies to improve their offerings, provide unique value propositions, and stay ahead of the competition.
- Enhanced customer insights. Digital transformation enables banks to gain deeper insights into customer behavior, preferences, and needs. By analyzing customer data, banks can offer personalized services, targeted marketing campaigns, and customized product recommendations, leading to higher customer satisfaction and loyalty.
These factors interact with each other and require a comprehensive approach for successful digital transformation in the banking industry.
An important point is cybercrime. The emergence of new technologies has left hackers with many loopholes for hacking into networks and devices. At the current growth rate, cyberattack damage will amount to about $10.5 trillion annually by 2025 —a 300% increase from 2015.
However, cyber threats are not slowing down digital transformation. On the contrary, they drive it (this applies to banks and other organizations). The search for vulnerabilities is a never-ending process that contributes to developing security systems.
The main principle of the fight against cybercrime in many banks is that the fight should be at all levels. It means from the protection of external perimeters to specific systems at specific addresses and ports. This includes protection against DoS attacks, firewalls, full control of the bank’s systems, control of viruses to avoid data leakage, etc.
Technologies are evolving at an incredible pace. Artificial Intelligence (AI), Big Data, Blockchain, and other innovations transform how we live, work, and do business.
For example, artificial intelligence allows banks to automate processes and make customer interaction more personalized and efficient. Machine learning can analyze large amounts of data, identify patterns and trends that help make better decisions and predict risks. Machine learning and neural networks also greatly help in document recognition and remote customer verification.
Big Data analysis is becoming a valuable tool in the banking sector, allowing banks to identify patterns, trends, and useful insights hidden in huge amounts of data. It can be used to develop personalized products and services, improve decision-making, detect fraud , and understand and predict customer behavior.
Blockchain is another innovative technology that can tremendously change the banking industry. Most of the current problems in the banking sector are related to the human factor. In particular, they include high commission costs and time spent on money transfers and transactions, internal and external fraud, human error, leakage of personal data, and much more. There are several main areas where blockchain technology can be used in the banking industry:
- Smart contracts
- International payments, settlements for foreign trade transactions, and internal payments
- Transactions with securities
- National digital currency
Other technologies that drive digital transformation in banking include Cloud Computing, Internet of Things (IoT), Robotic Process Automation (RPA), Biometrics, and Open Banking APIs.
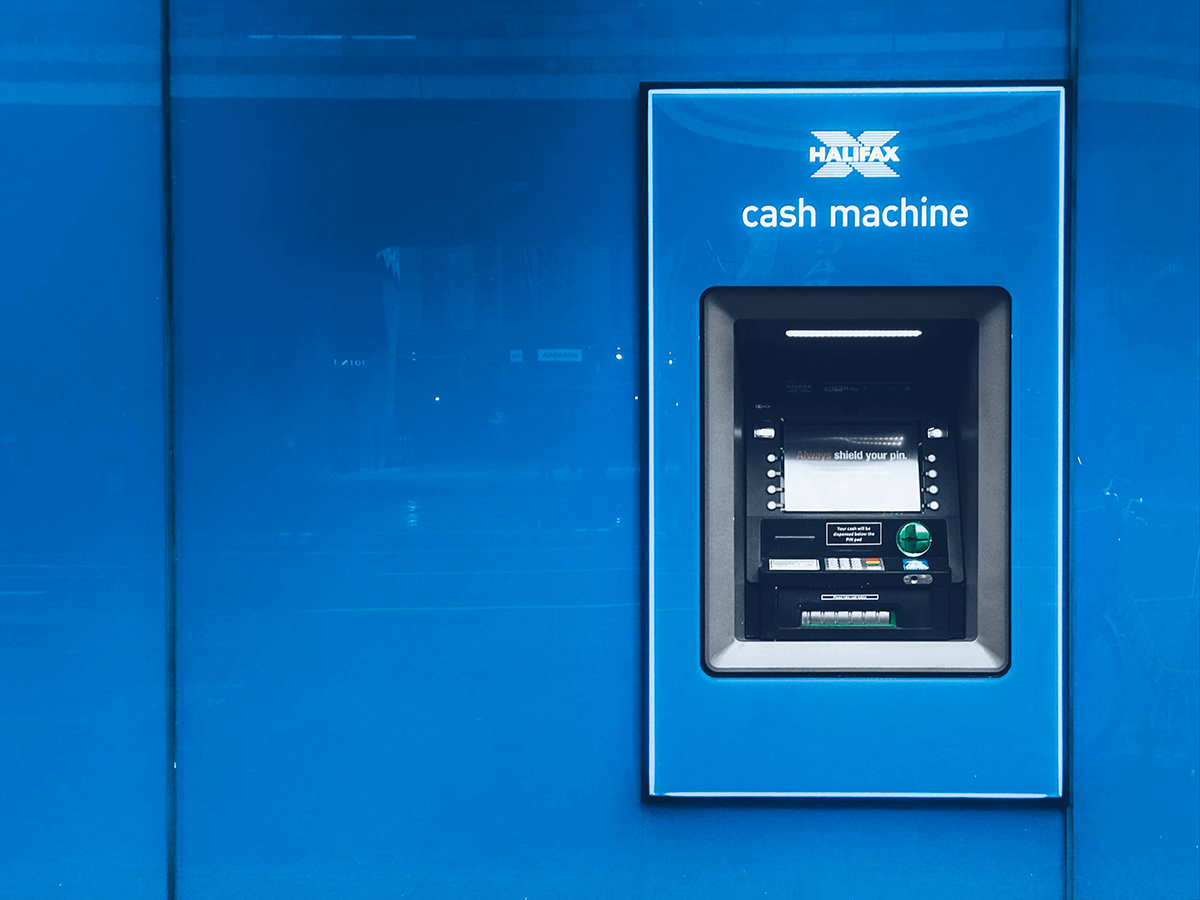
Many success stories of digital transformation in banking demonstrate how digitalization improves customer banking experience and operational efficiency. For example:
- DBS Bank (Singapore). DBS Bank is considered one of the leaders in digital transformation. They have developed a digital platform, DBS Digibank, which provides customers with a wide range of banking services through mobile apps and online banking. They actively use artificial intelligence and analytics to provide personalized recommendations and improve customer experience.
- JPMorgan Chase (USA). JPMorgan Chase has embraced digital transformation to improve operational efficiency and customer service. They have developed their proprietary digital platform, Chase Mobile Banking, which allows customers to perform various banking transactions through mobile devices. They also actively apply machine learning and analytics to better analyze data and deliver services.
- ING Bank (Netherlands). ING Bank has moved from a traditional bank to a digital organization. They provide customers convenient online services and mobile apps and actively use data analytics to provide personalized offers and improve customer experience. They have also implemented digital tools within the bank to streamline processes and improve efficiency.
- BBVA (Spain). BBVA focused on digital transformation and innovation to improve customer experience and banking processes. They developed the BBVA Digital Banking platform, which provides customers with a wide range of services through mobile apps and online banking. They have also implemented blockchain technology to improve the security and efficiency of financial transactions.
- Ally Bank (USA). Ally Bank is an example of a successful digital transformation. They provide a full range of banking services through an online platform, including account opening, lending, investments, and mortgages. Ally Bank actively utilizes digital channels and tools to provide convenience and accessibility to customers.
These examples demonstrate how banks use digital technologies to increase the availability of services, improve customer experience, and optimize their operations.
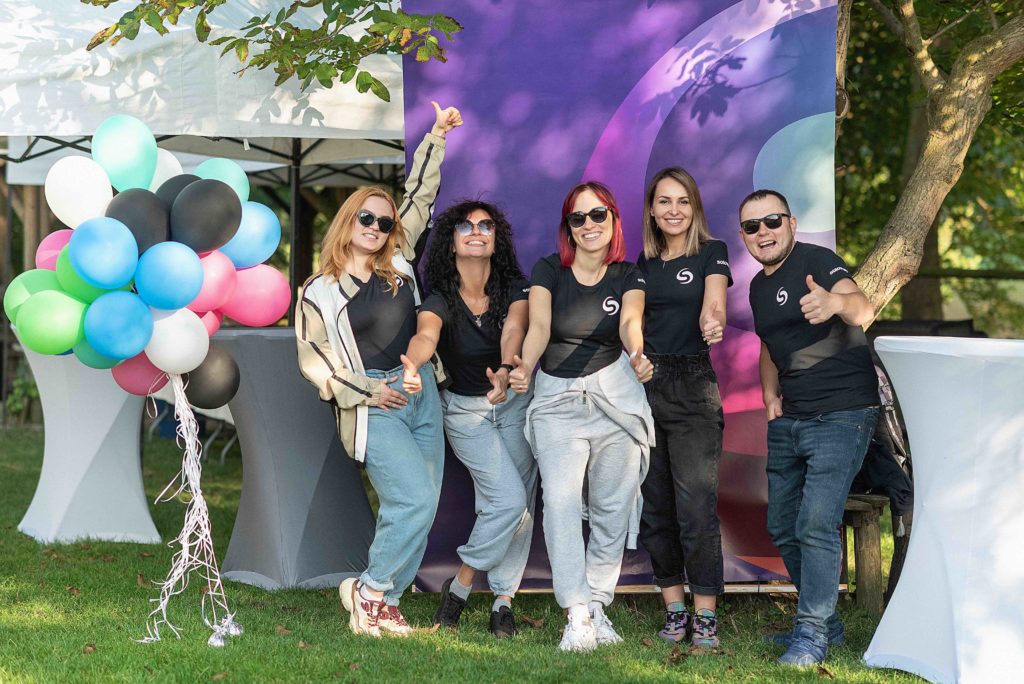
At SoloWay Tech, we specialize in providing comprehensive digital transformation and consulting services to help businesses thrive in the digital age. With our expertise and industry knowledge, we can guide your organization through the complex digital transformation process, enabling you to unlock new opportunities and achieve sustainable growth. We can:
- Consult regarding the digital transformation of your business
- Develop a digital transformation strategy
- Design digital customer experience
- Optimize business processes
- Automate business processes
- Re-engineer legacy apps
- Develop innovative products and services
- Implement end-to-end ML and AI engines
- Engineer IoT
- Build Big Data infrastructure
- Consult regarding the best implementation of IT infrastructure in your business.
At SoloWay Tech, we understand that each business has unique challenges and requirements. Our collaborative approach, deep industry expertise, and proven methodologies empower us to tailor our services to your specific needs, enabling you to achieve sustainable growth and competitive advantage through digital transformation.
Embark on your digital transformation journey with SoloWay Tech and unlock the full potential of your business in the digital era. Contact us today to learn more about our services and how we can help you drive innovation, efficiency, and success.
Digital transformation has become imperative for the banking industry to adapt to the evolving needs and expectations of customers in the modern world. The shift towards digitalization offers numerous advantages, such as enhanced customer experiences, improved operational efficiency, access to Big Data analytics, and new opportunities for innovation. However, there are also challenges to consider, including cybersecurity risks, potential job losses, and dependence on technology.
To embark on a successful digital transformation journey, businesses may seek the expertise of companies like SoloWay Tech that specialize in assisting organizations in their digitalization efforts. With the right guidance and implementation strategies, banks can harness the power of digital technologies to stay competitive, meet customer expectations, and drive innovation in the ever-evolving banking landscape.
Similar articles
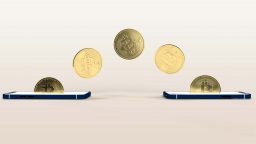
Finance 2025: Digital Transformation in Finance
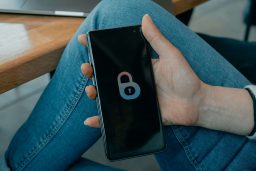
How To Develop A Blockchain Mobile App: Examples And Development Process
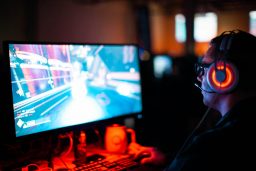
Blockchain Game Development: How to Use Blockchain Technology in Gaming Industry
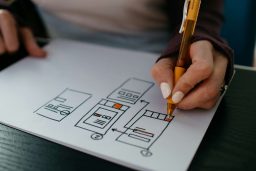
Digitizing Customer Experience: Step-by-Step Guide for Business Success
We use cookies to provide you with a better on website experience, privacy setting.
This website uses cookies to improve your experience while you navigate through the website.
View the Cookie Policy
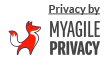
Fill the form below and Do your Solo
Company name, how can we help you.
- Tech & Privacy
- Product Safety
- Press Releases
- Our Experts
Consumer Reports is an independent nonprofit organization that works for a fair, safe and transparent marketplace.
Since we were founded as Consumers Union in 1936, we have advocated for the rights of all consumers. Now, we are united under the Consumer Reports name, bringing together our trusted testing, research, journalism, and advocacy.
We hope you will partner with us and our six million members for a better world.
For 85 years CR has worked for laws and policies that put consumers first. Learn more about CR’s work with policymakers, companies, and consumers to help build a fair and just marketplace at TrustCR.org
Banking apps: The case study for a digital finance standard
Executive Summary
Checking and savings accounts are one of the primary tools that consumers use to manage their financial lives, and the majority of consumers use a mobile banking app. A nationally representative Consumer Reports (CR) survey of 2,097 U.S. adults conducted in February 2023 found that 75% of Americans use one or more banking apps. Such apps allow users to check their balances; monitor their transactions; transfer and receive money; locate physical locations like ATMs, branches, and partner retail stores; pay bills; deposit checks; connect with customer service; and more.
This study builds on CR’s recent evaluations of peer-to-peer (P2P) payment apps and buy now, pay later (BNPL) services by applying CR’s Fair Digital Finance Framework to evaluate banking apps. We evaluated the banking apps across six principles of the Framework: Safety, Privacy, Transparency, User-Centricity, Support for Financial Well-Being, and Inclusivity. This evaluation explores the mobile banking apps, websites, and features related to checking and savings products of five large, traditional banks (Bank of America, Capital One, JPMorgan Chase, U.S. Bank, and Wells Fargo) and five digital banking providers (Albert, Ally, Chime, Current, and Varo).
We identified five key findings with numerical ratings based on those evaluations:
1. Most traditional banks charge maintenance fees; most digital banking providers don’t: Most digital banking providers offer free checking and savings accounts without maintenance fees, while few traditional banks do. Digital banking providers also tend to offer higher interest rates on their savings accounts. All five digital banking providers offer free checking, compared to only one of the five traditional banks. The costs of financial services matter, particularly for low-income consumers. Maintenance fees chip away at the disposable income available to consumers. Low interest rates on savings accounts can inhibit wealth-building opportunities. To avoid banking fees, many consumers choose not to use formal deposit accounts and lose access to important conveniences like electronic payments and remote deposit.
2. Data sharing needs controls and transparency: Most banking service providers tend to share more data than needed to deliver their core service, while only some banking apps offer the ability to opt out of targeted advertising. While these apps may provide conveniences to consumers through customized services and targeted offers, data sharing with third parties and across broader corporate structures leaves consumers vulnerable to predatory practices based on intimate details gathered about their financial lives. This finding represents the biggest opportunity for improvement for the industry; none of the 10 banking apps received high scores.
3. Inconsistent availability of digital tools for financial well-being: Traditional banking apps typically offer more financial well-being tools and features than digital banking providers offer. We reviewed the apps for the following tools: automated savings features, such as setting automatic transfers to savings or round-up savings, where transactions are rounded up to the nearest whole dollar and the change automatically directed to savings; the ability to send a portion of the user’s direct deposit to savings; budgeting tools; goal-setting features; and spending indicators. Although we found that a number of apps do provide a number of these features, only three banking apps we evaluated offer all five of these tools directly in the app.
4. Accessibility features uneven across sector: Traditional banks are more likely than digital banking providers to offer their websites, apps, and policies in Spanish. Banking websites are more likely than banking apps to have built-in accessibility features for people with disabilities. Financial products should be accessible to all users. Language and disability should not be a barrier to using banking apps.
5. Incomplete commitment to fraud protection: Some banking service providers do not explicitly commit to monitoring fraud in real time and to notifying users in the event of suspicious activity. While all banking service providers that we evaluated have fraud education materials on their websites, three do not offer similar materials within their apps. The risks to consumers of banking fraud and scams continue to increase, and banking apps can do more to support users with information and education.
Consumer Reports scored each banking app on the data supporting the five key findings. See the full report for the scores for each banking app. Each company has areas where it excelled and areas for improvement.
- Azure Marketplace
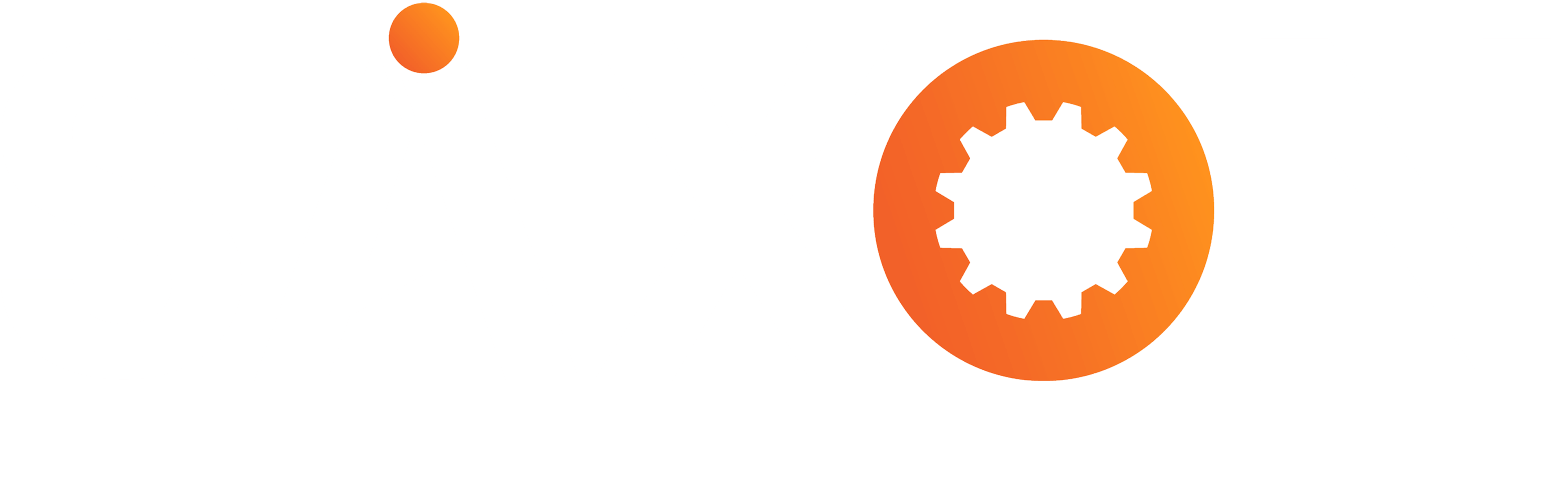
- Search for:
The 6 Definitive Data Analytics Use Cases in Banking and Financial Services
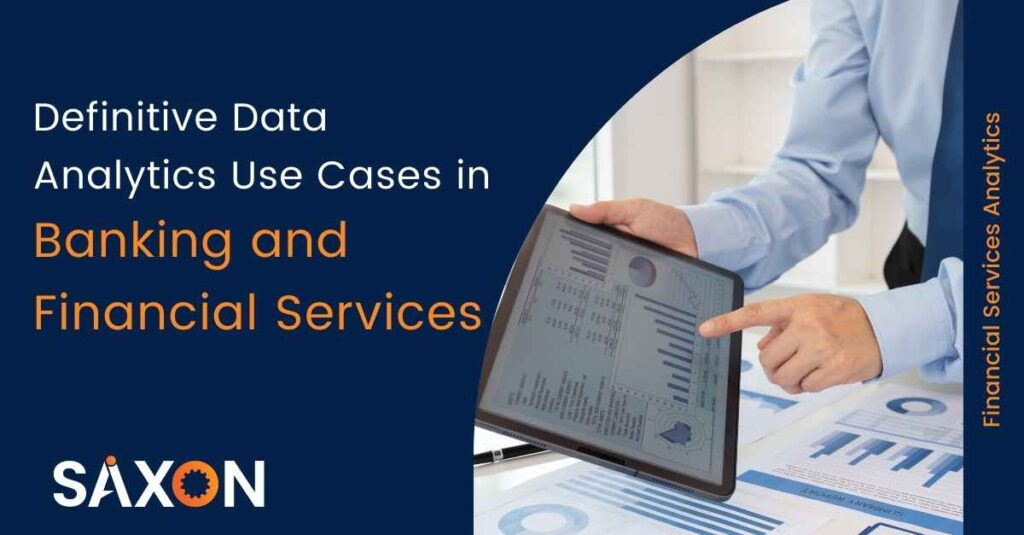
Financial services organizations were traditionally product-centric, but they turned to be customer-centric with the evolving tech landscape. Digital transformation in financial services is more profound than leveraging data and digitization.
Personalization and customer experience now deepened after the pandemic. With the shift towards mobile, almost 89% of customers now prefer mobile banking channels , and digital-only banks are transcending traditional banks. Unlike financial services organizations, fintech start-ups leverage technology and data analytics as per customer preferences.
Intense competition and tech disruption are the game-changer for fintech. Let us talk about the example of loan disbursal. Banks have a lot of KYC and due diligence processes that delay the loan disbursal. But data analytics and AI make it easier for fintech start-ups to decide in minutes. Many leaders were leveraging compelling data analytics use cases in banking and financial services. But they have to be updated regularly with the evolving tech landscape.
Why do banks need to leverage advanced analytics?
Customer experience is the new competitive battleground for banks and financial services – In traditional banking business models, customer service was synonymous with customer experience. But now, ease of access, ease of use, and resolutions in no time seems to be the new face of customer experience. The financial services industry has more challenges with the data flow from these multiple channels with omnichannel presence.
AI is critical in the new CX – The applications are manifold in financial services – chatbots, AI-powered automation, and AI data analytics. Predicting the customer needs, providing services, and resolving queries in no time enhance customer experience, the new norm for financial services.
Data analytics is not about cutting costs but focusing on productivity – Do you know that leveraging advanced data analytics for fraud detection can save costs up to 20%? Earlier it was just automation of a document management system or repetitive processes. But now, it is more about leveraging technology for credit modeling, risk analysis, and fraud to leave humans for more critical projects.
Advanced Analytics in BFSI – Benefits
Updating the data analytics use cases in banking and financial services with the evolving data science methodologies can help organizations sustain stronger customer relationships. Let us look at a few more benefits of advanced analytics.
Customer 360-degree insights – By leveraging advanced analytics, financial services organizations can know more about customer preferences, multichannel touchpoints, and buyer behavior factors. There is a high chance that the sales folks might perceive a different need, but the data speaks another consumer behavior. Understanding the customer in detail is critical for banking and financial services , unlike other industries.
Personalized customer experience – Experts perceive personalization as another critical aspect in BFSI to reduce churn and improve revenues. Offering the right product at the right time while also reaching out with personalized information after understanding every consumer detail is now the norm for sales teams in BFSI. A report from Forrester says that a single point improvement in financial services organizations’ CX score can improve revenues from $5-$123 mn.
Reduction in operational costs – Banks and financial services organizations are under constant pressure to maintain sleek profit margins and improve operations. Financial services firms can leverage predicting analytics, visualization, and AI to automate their workflows. Replacing paper-based forms with digital applications and using NLP technologies where ever necessary also helps in reducing manual efforts and errors.
Risk mitigation – The main challenge for BFSI firms is to analyze risks like credit, claims, and fraud. Though the practice is not new, banks, insurance companies, and investment bankers need to update their risk approach with the evolving technologies and exploding data from multi-channels. Financial services organizations can modernize their risk management practices more efficiently using predictive, behavioral, and advanced analytics .
Competitive advantage – Fintech organizations with technology as their core are already disrupting financial services. Financial services organizations now need to adopt technology faster than before. Processing a loan application can be done in minutes with AI and advanced analytics, thereby providing more scope for customers. Data analytics in banking will enable you to understand the unmet customer needs and help you unfold new consumer-centric business models.
Best Definitive Data Analytics Use Cases in Banking and Financial Services
Most of us know about the data analytics use cases in banking and financial services. Do we need to view them differently after the pandemic? Our experts say that the customer data is changing rapidly, and so are the touchpoints. To successfully implement data analytics in banking, the models should reconsider all the available data from the expanded sources. Let us rethink the advanced analytics use cases with the changing consumer ecosystem.
Credit Modeling – Credit risk modeling is not new in the banking industry. The traditional risk analytics models provided insights based on income sources, loan history, default rates, credit rating, demographics, etc. Many other factors need to be analyzed in conjunction with the standard data. Let us consider the case of consumer loans; different dynamics like social media profiles, utility bills, monthly spending, and savings give more profound insights into the default risk. Unstructured data plays a vital role in credit risk modeling too. AI-based text analysis and consumer persona provide deeper insights into the customers’ financial well-being.
Risk Analysis and Monitoring – Banks and financial services organizations that implement dynamic risk models with advanced analytics seem to be more resilient to significant external changes. Risk models differ between Banks and financial services – credit risk, fraud, and liquidity risk are the major ones for banks; claims risk and fraud for insurance and portfolio risk analysis for investment bankers. The common risk for most financial services firms is fraud detection is continuously evolving. Machine learning, AI, and big data now enable organizations to analyze many transactions, not just based on historical data. Social media profiles, behavioral analytics, predictive analytics , and advanced machine learning models are leveraged collectively for fraud detection.
Customer LifeTime Value – The trickiest one but looks like the most simple one to understand for anyone in the banking perspective. Customer lifetime value provides insights about the future revenue sources from the customer to focus marketing efforts and reduce churn. It is tough to estimate how customer behaviors change with time and the significant factors impacting their decisions. AI-powered advanced models recognize patterns more effectively in the data to provide behavioral insights that humans may not be able to identify.
Product Recommendation Engine – Are we talking about retail? No, product recommendation engines are evolving in banking too. Multiple comparison sites are now available for each financial services product – loan, insurance, mutual funds, credit cards, etc. Consumers can make informed choices, but cross-selling financial products at the right time cater to customer needs and enhances trust. Machine learning models process data in real-time from various content feeds to make the job easier for financial/investment analysts to offer personalized products and services.
Customer segmentation and personalized marketing – Understanding every aspect of the customer is critical for personalization. Customers are now bombarded with different financial products at the same time. How do you know if a customer is looking for an auto loan? Does the customer intend to purchase a home or an automobile? The place and timing of your marketing efforts matter in creating trust and showing intent to act on the marketing messages. You can also reduce awareness marketing efforts if you provide the knowledge at the right stage of the buyer journey.
AI-powered Virtual Assistants – Consider the case of insurance; a loss or damage may not happen multiple times. It is the single touchpoint to show the customers how you care for them and ease the processes. Customers now prefer efficient self-service options to in-person contacts to process their requests. AI-powered virtual assistants add value in answering all the information queries about products, services, and eligibility criteria in financial services. They are also evolving to validate certain criteria based on the rules updated with the machine learning models. It wouldn’t be a surprise to see that an AI-powered assistant does the insurance claims processing in minutes.
Are you interested in more data and analytics use cases in banking and financial services? Get in touch with our experts to get the mind share.

Marketing Desk
Explore our services.
- Modern Enterprise Apps
- Intelligent Automation
- Data Analytics and Insights
- Azure Cloud Solutions
- Modern Work
- Digital & App Innovation
Get Started
Related Posts
- Data-driven Supply Chain and Packaging Solutions in the “New Normal”
- Why do you Need Automated Data Pipelines for Accelerated Insights?
- How to gain advantages with Azure Synapse Analytics Integrations?
- AI and Automation
- Business Applications
- Cloud Infrastructure
- Data and analytics
- Latest Buzz
- Microsoft Copilot
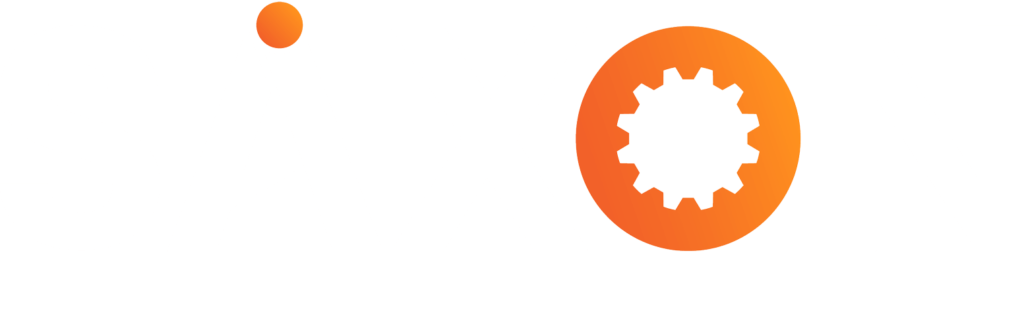
TAKE THE NEXT STEP
- AI & Automation
- Data, Analytics, and Insights
- Azure Cloud Infrastructure
- Digital & App Innovation
- DigitalClerx
- Case Studies
- Infographics
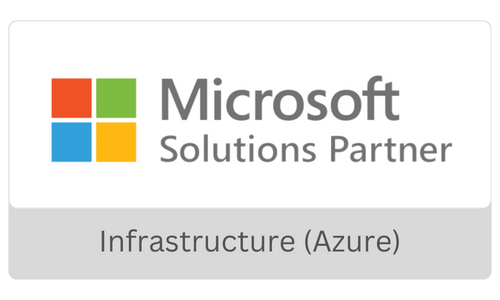
Get the insights that matter
Stay up-to-date with our latest news, updates, and promotions by subscribing to our newsletter.
Copyright © 2008-2023 Saxon. All rights reserved | Privacy Policy
Address: 1320 Greenway Drive Suite # 660, Irving, TX 75038
- Gen AI consulting workshop
- Gen AI for Business
- Microsoft 365 Copilot
- Gen AI for Sales
- Low-Code/No-Code Development
- Microsoft Teams App Development
- Intelligent Business Apps
- Microsoft Power Platform
- App Modernization
- Saxon Vision AI Suite
- Process Intelligence
- Intelligent Document Processing
- Conversational AI
- Robotic Process Automation
- Intelligent Back Office Automation
- Azure AI for Contact Centers
- Data and Analytics
- Microsoft Fabric Services
- Data Engineering and Governance
- Data Migration and Modernization
- Data Visualization & Reporting
- Data Science and Machine Learning
- Azure Consulting Services
- Azure Implementation Services
- Azure Managed Services
- Azure Cost Optimization
- Copilot for Modern Work
- Document Management System
- Intelligent intranet
- Intelligent enterprise search
- Intelligent AI Apps
- DevOps Implementation
- DevOps Consulting Services
- Application Migration
- Azure Integration
- Immersion Day
- 3-Week Pilot Consulting Workshop
- Governance Workshop
- AI Use Case Discovery Workshop
- Cognitive Services POC
- Enterprise Automation Strategy
- Intelligent Sales Nudges
- Azure AI Health Bot
- Yellowbrick
- Automation Anywhere
Banking & Financial Services case studies
Explore case studies, articles, white papers, product info, and more..

- Case Studies
- API Management
- Banking and Financial Services
- B2B Integration
- Financial Accounting Hub
- Healthcare and Life Sciences
- Managed Cloud Services
- Managed File Transfer
- All Case Studies
- Product Info
- Amplify Enterprise Marketplace
- Amplify Integration
- Amplify Platform
- Axway B2B Integration
- Axway Business Network (VAN)
- Axway eInvoicing
- Axway Financial Accounting Hub
- Axway Managed File Transfer
- Axway Validation Authority (VA) Suite
- Specialized Products
- IT Challenges
- Govern APIs
- Secure APIs
- Analyst and research reports
- Manage complexity
- Modernize EDI & B2B
- Move B2B to cloud
- Move MFT to cloud
- Enable Intelligent MFT
- Business Needs
- Accelerate digital projects
- Productize APIs
- Monetize APIs
- Build an API marketplace
- Secure file transfers
- Energy and Utilities
- Government and Public Sector
- Manufacturing, Automotive, and CPG
- Telecommunications
- Transportation and Logistics
- Data sheets
- Infographics
- Reports & survey results
- Solution briefs
- Topical flyers
- White papers
Open banking. The art of the possible in 10 use cases.
- Share this Asset
10 open banking uses cases
How can financial services companies drive real value and service clients better through open banking ? A new report by Altitude Consulting and Axway details a number of use cases to help you visualize the art of the possible in open banking and imagine new ways to grow your organization’s capabilities, gain a competitive edge, and deliver superior customer experiences. Here are 10 of them:
No. 1: Account aggregation
Open banking offers secure APIs for accessing financial account data and benefits all parties: banks, account owners, and fintechs. With open banking, banks have greater control and visibility into third parties that are accessing their clients’ financial data and the purpose for which the data is being used.
No. 2: Consumer spending insights
Financial documents and data from external credit and bank accounts can bridge the gap between banking and buying, uncovering opportunities for banks to proactively engage customers. Customer life stages, psychographics, brand loyalties and other triggers help to create a personalized experience.
No. 3: Buy now, pay later
BNPL (By Now Pay Later) fintechs use the banking systems to verify consumers’ identity, retrieve financial information, verify accounts, conduct KYC checks and make lending decisions. Consent management allows consumers to grant access to data for loan applications and credit approvals which improves conversion rates.
No. 4: Wealth management
Wealth management with open banking capabilities makes serving customers more efficient. Customer permission to retrieve data from external accounts, and conducting a digital KYC, smooths onboarding for new and existing customers looking to take advantage of the bank’s financial planning services.
No. 5: Insurance Sales
Banks can analyze customer data for insurance triggers to provide personalized insurance offers through online and mobile channels. Such triggers are found in personal information updates, purchasing data from external cards aggregated through open banking, and location data from a bank’s mobile app.
No. 6: Personalizing products and experiences
Open banking allows banks to combine data from external sources, including other banks, brokerages, credit issuers and investment managers, and combine it with first party data such as buying history, credit score, income, age and location, to build a deep understanding of each client’s unique needs.
No. 7: Bill payment and management
Improved billing and payment capabilities offer customers more payment options and increase customer engagement. Open banking APIs let billers send bills directly through the bank’s channels and use detailed consumer behavior data to deepen loyalty and offer the right product at the right time.
No. 8: Tax preparation
Open banking provides a number of tax preparation benefits. With a direct connection from bank systems to popular accounting platforms to retrieve tax data, banks will realize operational efficiencies, be able to serve consumer and business clients better, and reduce call volumes during tax season.
No. 9: Payment initiation
Open banking lets consumers and corporations initiate payments directly from their online banking accounts without entering routing data, simplifying the payment experience. Multiple payment options are available within the same payment interface and payments are often processed in minutes or hours.
No. 10: Streamlining account opening
With open banking, digital ID verification and cross- referencing with external accounts reduces manual input by the user, speeding account opening. Funding is done directly from another bank account via ACH transfers. Virtual cards allow new customers to make purchases as soon as the account is funded. * Download this open banking checklist as a pdf
Previous Asset

Take a quick look at the highlights - then get the full story on how Axway improves collaboration and innov...

Amplify API Management Platform helps Commerzbank turn traditional, branch-based services into seamless dig...
Other content in this Stream
Rapidly deploying API-driven products with Axway


The ability for open banking to open up customer data to third party payment vendors will give the banks the ability to provide their customers with better services. In this video, Laurent Van Huffel

Learn about some of the concerns with screen scraping and how open banking can solve common issues while making innovation easier.

Open banking APIs are your financial institution’s product offerings, and your most important channel to market. Here’s why a marketplace helps cater to the business leaders AND product managers.

What are the necessary components in an open banking solution, and does it make more sense to buy a specialized solution or build it yourself? It depends on your objectives.
Learn who's putting the customer first in the economy of embedded finance
Read this infographic to see 6 things you need to know about accessing and using customer data, including what screen scraping is, what the pitfalls are, and how it's different than open banking.
See how Tribanco digitally transformed to go beyond regulatory compliance and find new open banking opportunities?
Start delivering digital banking services to your members

See why and how credit unions and smaller banks are making digital banking services accessible for everyone, anywhere with universal API management
Here are three digital banking example uses cases to help you imagine new ways to grow your organization’s capabilities, gain a competitive edge, and deliver superior customer experiences.
Putting power in the hands of the business line

To better serve their customers with added-value digital services, Commerzbank shifted from the traditional banking market to the open banking market using Axway’s Amplify Platform.

Powered by Axway’s Amplify API Management Platform, Commerzbank is now able to create new business models enabling innovative fintech partnerships.
Take a quick look at the highlights - then get the full story on how Axway improves collaboration and innovation in financial ecosystems.
Amplify API Management Platform helps Commerzbank turn traditional, branch-based services into seamless digital experiences.

Find out how banks are using their data to gain a competitive edge. This opportunity assessment explores 15 popular fintech use cases.

Transparent Data is a Polish technology company that has created its own business category in data software since 2013. Thanks to a unique combination of high technical competence and experience...

There’s a revolution happening in the financial sector, and in many areas, it’s happening fast. Technology is transforming the way we do just about everything, and financial services are no...

PermataBank is one of Indonesia’s leading banks and a pioneer in mobile banking and mobile cash technology. Its success in harnessing the potential of APIs to drive new revenue and build...
- Share this Hub
Case Study: the Business Banking Transformation Journey at De Volksbank
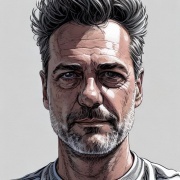
- Website for Roland Flemm
- Contact Roland Flemm
- Twitter for Roland Flemm
- LinkedIn for Roland Flemm
- Facebook for Roland Flemm
- GitHub for Roland Flemm
De Volksbank is the fourth largest bank in the Netherlands. The bank was formed two hundred years ago from regional savings banks. Today, de Volksbank focuses on consumers, independent entrepreneurs, and small and medium-sized enterprises. The bank operates mainly in the areas of payments, savings, and mortgages.
In 2021 a new four-year strategic agenda was introduced focusing on growth by strengthening customer relationships and further increasing the banks' social impact. To realize this ambition, a transformation was initiated that aimed at implementing a uniform agile way of working with independent, integrally responsible customer journey teams. The new strategy should make the bank more customer-centric and enable them to get better, faster, and more effective at delivering value to customers.
The transformation impacted the whole bank. In March 2022, about 3,000 people faced either a change of work, a change of team, and/or a change in the way of working. In the new structure, the bank was split into 14 "Hubs". These are value areas with P&L responsibility. Each hub is responsible for a specific customer journey. A Hub is set up integrally, which implies that each hub contains business, IT, and operations. The entire organization "flipped" to the new model in March 2022.
This case study analyzes the transformation journey using Org Topologies™ Scans and focuses on the Business Banking Hub. It covers three organizational design phases:
Org scan of the pre-agile setup before August 2021.
Org scan of the 2022 dVM pilot .
Org scan of the current layout per February 2023.
We will clarify the stages with OT maps using the following symbols:
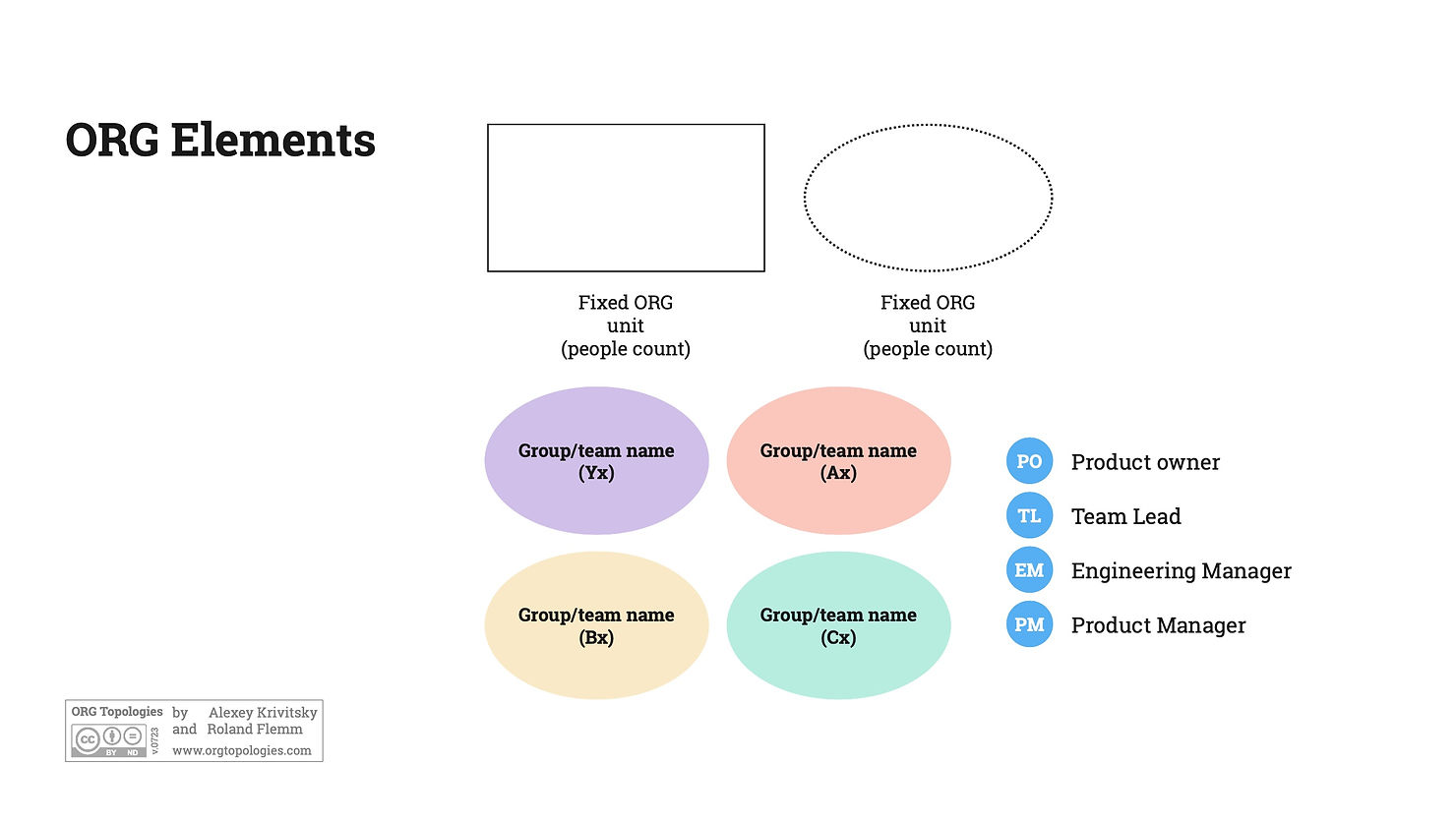
More explanation and background information on Org Topologies™ that help to understand this case study can be found here and here .
1. Pre-transformation setup before August 2021.
The department "Business Banking" was divided into three sub-departments: Operations, Business Lending Sales Support, and Customer Onboarding. These departments were focused on servicing business customers. A single team "monitoren en verbeteren" (monitor and improve) was responsible for product development. This was the department's R&D team. The picture below is a detailed view of the Business Banking department in deVolksbank prior to the transformation.
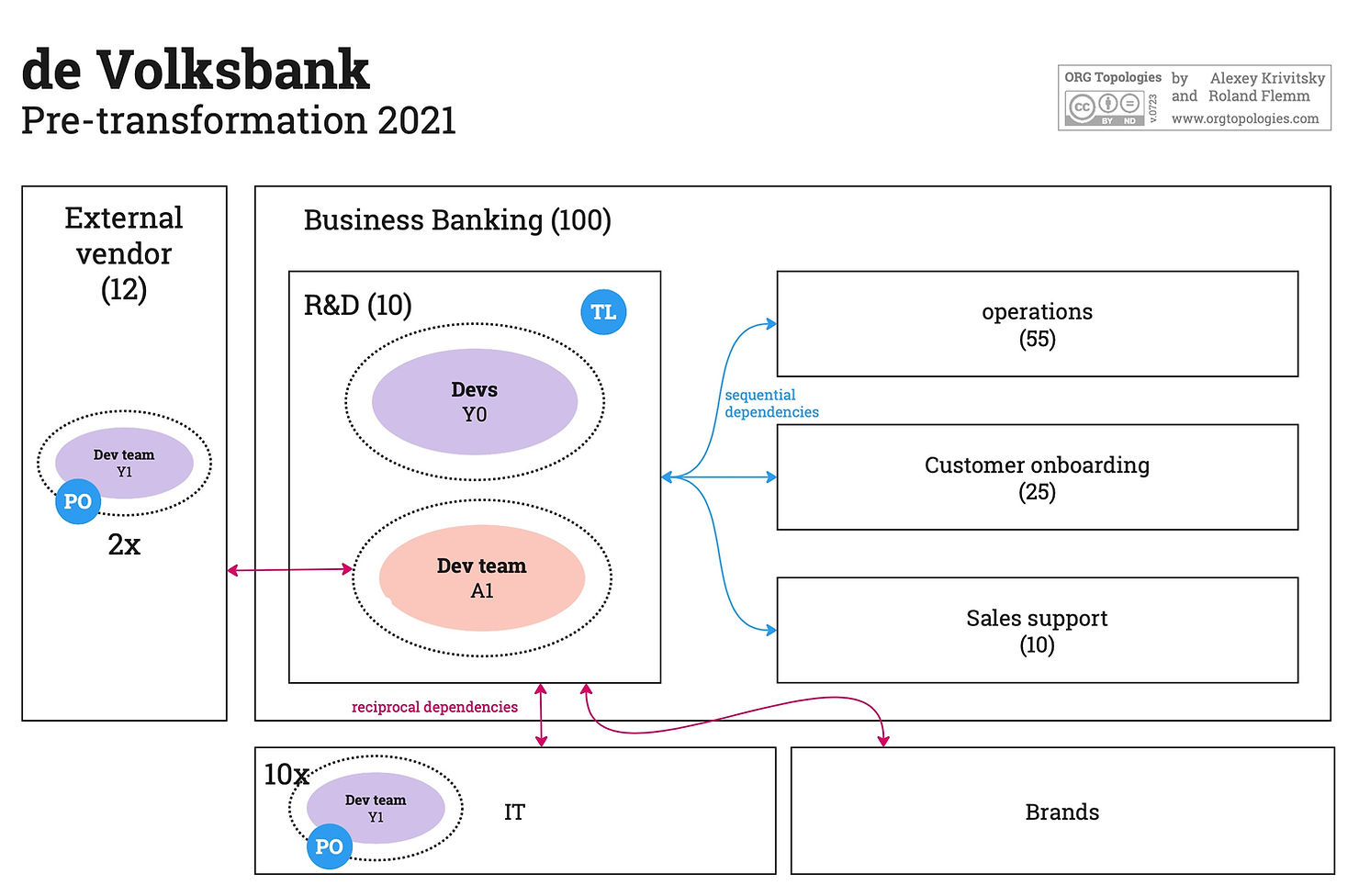
Of the 10 people in "monitoren en verbeteren", 6 were tasked to improve the operational workflow of the three operational teams. They had task scope, no product vision, and no value-based prioritization of work. They were working on improvement requests given to them by the operational teams. These requests did not necessarily have high customer value.
They were working as individuals on unprioritized tasks (three operations groups with different requests). They did not have the skills or authorization to make software and configuration changes in the banking systems.
Their work was restricted to:
specifying changes requested by the operational teams,
assigning them as tasks to technical teams somewhere in the adjacent IT group,
chasing progress.
Working as individuals on unprioritized tasks (three operations groups with all kinds of change requests) makes them a Y0-level group on the OT map .
The queue of work was huge due to the long waiting times for the work in progress. Larger and more complex initiatives were hard to implement and took many months, sometimes years to complete. Tasks belonging to these initiatives got stuck in the worklists of teams in the IT department due to unclear decision processes. The people were used to working in an environment where asynchronous communication via email requests was the norm. They were spending most of their time lobbying in IT to get their requests prioritized and negotiating with a vast landscape of stakeholders. A huge amount of time was wasted waiting.
The other 4 people worked as a Scrum team focusing on New Business Lending products. The subgroup's work was specifying and accepting changes in collaboration with an external software company that developed a New Business Lending product. This team specified customer-centric features for the vendor to develop. The team and their PO were decomposing the customer requirements into tasks to hand them over to the vendor's development teams. This makes them an analyst team for the external developers, archetype A1 in "the speculation department" on the OT map.
The New Business Lending team did not suffer from the bank's IT dependencies but had 100% dependency on their external vendor and they had some dependencies on business stakeholders ("Brands"). They were able to deliver value much faster than the teams with internal IT dependencies.
The developers at the vendor were processing tasks for multiple clients. The vendor did not have dedicated teams per client but had two groups of developers working on specific parts of the product.
2. Pilot with feature teams 2021-2022.
Prior to the bank-wide adoption, a new self-invented model (based on team topologies and Spotify) called "dVM" (de Volksbank samenwerkingsModel) was piloted in the Business Banking department. It is noteworthy to mention that this transformation initiative was driven by the Business side of the bank, not by IT, which is more common.
The people working in the R&D team "monitoren en verbeteren" were placed into four groups, each focusing on a specific customer journey. Each group contained one R&D team and one or more service teams. The R&D teams focused on developing products and the service teams kept their focus on daily operations, serving the customer. There was a tight feedback loop between them. The operational teams were important stakeholders, providing valuable customer feedback. Each R&D team had its team PO, prioritizing work on value.
The following groups were created:
The Customer Onboarding group: everything related to smoothly welcoming new customers.
The New Business Lending Group: building new business lending solutions.
The Business Lending Maintenance Group: Supporting existing business lending solutions.
The Bloxx group: developing an approach allowing business customers to configure their own service and product catalog
Each R&D team was formed with cross-functional business people. Each team was able to address a full range of business problems (features) related to their domain but they had no technical expertise. This makes them A1-level teams (analyst teams, or "the speculation department"). The backlogs contained customer-centric features instead of service-oriented tasks.

The fact that the R&D teams did not have IT capabilities was a problem because most of the changes they were working on required changes in IT systems. Some teams were able to do no-code changes, but all 'n all, their technical capabilities were limited.
Although still low on the Org Topologies™ map, this setup was experienced by the team members as a huge improvement because now:
they were working full-time in a steady team,
they were working on the specification and delivery of customer features,
they were working on high-value work because work was prioritized on customer value.
At the same time, having limited capabilities combined with end-to-end responsibilities was frustrating. They were wasting time chasing their changes to be delivered. We gradually improved this situation by extending their (technical) capabilities. This desire for autonomy was not appreciated by the surrounding organization. Don't forget this was only a very small pilot in a very big bank, so not surprisingly, the team's appetite for mandate, capabilities, and speed was causing resistance in the remainder of the bank.
The New Business Lending team continued working with the external vendor. In the beginning, this made their life relatively easy since they had limited dependencies on the technical infrastructure and IT teams of the bank. However, the number of teams at the external vendor had grown and now were organized as component teams: Each vendor-component team had a team "PO" managing their team's backlog. Coordinating the work across the other vendor teams was difficult and slowed down the development process. An additional complication was that the external vendor was not solely focused on delivering the bank's requirements but they were also productizing the requirements to market them to other customers. This makes total sense, but it was not helping the bank. In summary, from the bank's point of view, the vendor's business model and organizational design had a negative effect on the quality of service.
The other three teams were depending heavily on the bank's IT teams. The IT departments worked as component teams (Y0 and Y1 archetypes). Due to the high number of technical dependencies and the lack of support for the new feature teams, the Customer Onboarding PO got completely stuck. She had to split customer requirements to feed the IT component teams, she had to fight to get her work prioritized, and she had to bend over backward to find a way to get her changes integrated across multiple release calenders. Not a job you would envy...
The Bloxx team was fortunate because they were the first team to have developers in the team. However, there were some startup problems here too. The developers in the Bloxx team were working under the strict guidance of the bank's PEGA group. This group had a strong architectural drive and was focused on building centralized and reusable solutions for the whole bank. The PEGA developers were telling her how to change the specifications so the solution could fit into the PEGA architecture. It was the world upside down: the Bloxx PO found herself being a lobbyist trying to get the PEGA developers in her team to build what she needed.
One thing all teams had in common was their dependence on "the brands". The bank exposes its services in the Dutch market using four distinctive customer-facing brands. These brands had different and conflicting requirements for the solutions built by the four R&D teams. The brands were powerful: they were deciding on requirements and approved or rejected the changes created by the R&D teams, often on the technical level.
Customer Onboarding tried to get around this problem by adding a brand specialist to their team. This person was working as a proxy on behalf of all brands. In practice, this did not work because the brand specialist's decisions were overruled by other (brand) stakeholders. The dedicated teams were able to deliver in a regular Sprint cadence, which was much faster than before (weeks instead of months). But their mandate was not respected by the unchanged organization. In addition, the contradictory demands of the business stakeholders, and the complex IT environment were frustrating them.
These were the most important learnings from the pilot that helped to improve the dVM org design:
Feature teams with a PO to prioritize work on value and the operational teams' focus on servicing the client resulted in faster delivery of better solutions and better service to the customer.
Reducing the power of the stakeholders (i.e. brands) by clarifying the R&D team's mandates is required.
Reducing the influence of (middle) management by repurposing their role in the service of the teams is needed.
Teams should be bus-dev-ops teams by adding all technical capabilities to the teams required to deliver "Done".
Clear responsibilities and capabilities of the feature teams attract stakeholders who are in need of the capacity to get things done and try to feed their changes into the feature teams. This was unexpected behavior that actually proved this initiative was successful.
Creating feature teams creates much resistance in the organization surrounding these teams. Gradual change is more complex to manage.
3. De Volksbank SamenwerkingsModel (dVM) in Feb 2023.
The pilot setup with R&D teams and service desk teams (operations) close together in groups was implemented in the whole bank. All four Business Banking R&D teams gained autonomy by growing their technical capabilities. Some reached A3 (autonomously working end-to-end on customer journeys) and most were A2 (incomplete teams working on customer journeys, not end-to-end).
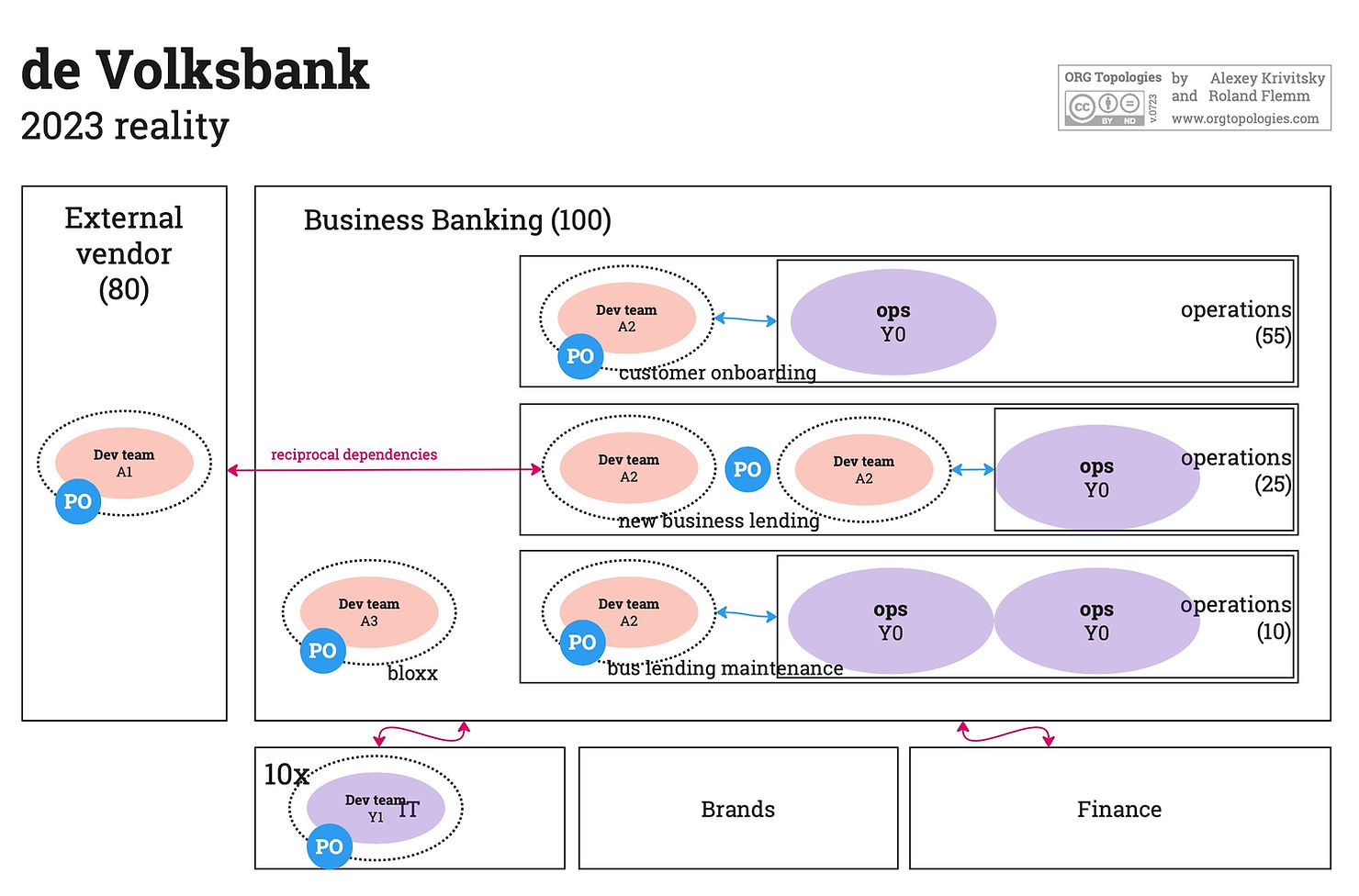
The majority of the dependencies on the brands have been resolved by moving product responsibilities of the brands to the feature teams in the Hubs. The brands now have teams that focus purely on work like marketing and campaigning, not banking product development. The separation between roles and responsibilities is clear: the R&D teams have the mandate and the brand teams are stakeholders to them.
Most of the dependencies on IT have been resolved. Two of the R&D teams now all have the technical capabilities they need to work autonomously. The solution to remove the technical dependencies was achieved by adding developers to the teams. The POs determine what is most valuable, not stakeholders like the architecture group via the (PEGA) developers.
The New Business Lending team's dependencies on the external vendor were not resolved. The vendor has added a "first contact" proxy team to better serve de Volksbank. The proxy team provides lo-code/no-code solutions, is a single point of contact, and handles coordination with the vendor's internal component teams. The bank and the vendor collaborate on further reducing lead times. The proxy team operates at the feature(set) level and is narrowly specialized in analysis (A1 Archetype).
The teams inside a value area (Hub) coordinate using OBEYA . The same practice is used to align with teams in other hubs. The Business Banking Hub Lead says that the implementation of the dVM model was very successful in creating strong autonomous teams. Alignment between all teams inside the hub using OBEYA has proven to be pretty successful too, but there are challenges in resolving cross-hub initiatives. Higher management has a tendency to fall back to old patterns to manage cross-hub problems (instead of trusting the Hubs to self-organize). On one hand, this is due to a lack of experience using OBEYA for that purpose. On the other hand, the Hubs suffer from local thinking. The Hub leadership teams should be more aware of the recurring dependencies between Hubs (and teams) as an indication that the org design can be improved.
Did the new org design make Business Banking more customer-centric and get better, faster, and more effective at delivering value to customers? It definitely did. Further improvements will be achieved by eliminating remaining dependencies, re-evaluating the current org design and improving the OBEYA for better cross-Hub collaboration. We are happy that the Business Banking Hub POs perform experiments with cross-team collaboration (applying practices from the higher B-level).
The dVM design was intended to be an evolutionary model. The OT Scans (TM) can serve as a guide to monitor the progress of the bank's journey to further improve its organizational design. We recommend each department (Hub) plots a current and future state map. This will increase our understanding of why things currently work or do not work, and will enable us to find the root causes and best possible solutions for improving the management of cross-hub problems, resolving remaining impediments, fixing staffing problems, etc.
Acknowledgments
We want to thank the following people for investing their time in creating this case study with us:
D. Karacan (PO New Business Lending)
J. Verhey (Hub Lead Business Banking)
(C) 2022, Alexey Krivitsky and Roland Flemm. Org Topologies™.
What did you think about this post?
Share with your network.
- Share this page via email
- Share this page on Facebook
- Share this page on Twitter
- Share this page on LinkedIn
View the discussion thread.
Scaling gen AI in banking: Choosing the best operating model
Generative AI (gen AI) is revolutionizing the banking industry as financial institutions use the technology to supercharge customer-facing chatbots , prevent fraud, and speed up time-consuming tasks such as developing code, preparing drafts of pitch books, and summarizing regulatory reports.
About the authors
This article is a collaborative effort by Kevin Buehler , Alison Corsi, Mina Jurisic, Larry Lerner , Andrea Siani, and Brian Weintraub , representing views from McKinsey’s Banking Practice and Risk & Resilience Practice.
The McKinsey Global Institute (MGI) estimates that across the global banking sector, gen AI could add between $200 billion and $340 billion in value annually, or 2.8 to 4.7 percent of total industry revenues, largely through increased productivity . 1 “ The economic potential of generative AI: The next productivity frontier ,” McKinsey, June 14, 2023. However, as banks and other financial institutions move to quickly implement the technology, challenges are emerging. Getting gen AI right can potentially unlock tremendous value; getting it wrong can lead to complications . Companies across industries face gen AI risks , including the generation of false or illogical information, intellectual property infringement, limited transparency in how the systems function, issues of bias and fairness, security concerns, and more.
In a previous article, we explored a series of strategies that banks could use to capture the full value of gen AI . Achieving sustained value, beyond initial proofs of concept, requires strong capabilities across seven dimensions:
- strategic road map
- operating model
- risk and controls
- adoption and change management
These dimensions are interconnected and require alignment  across the enterprise. A great operating model on its own, for instance, won’t bring results without the right talent or data in place.
This article takes a closer look at one of these seven dimensions: the operating model, which is essentially a blueprint for how a business puts strategy into action. Subsequent articles will examine some of the other dimensions. In this article, we explain what an operating model is and why it is important, then delve into the operating-model archetypes that have emerged for gen AI in banking—including the one with the best record of success. Finally, we go over important decisions financial institutions need to make as they set up a gen AI operating model.
We have found that across industries, a high degree of centralization works best for gen AI operating models. Without central oversight, pilot use cases can get stuck in silos and scaling becomes much more difficult. Looking at the financial-services industry specifically, we have observed that financial institutions using a centrally led gen AI operating model are reaping the biggest rewards. As the technology matures, the pendulum will likely swing toward a more federated approach, but so far, centralization has brought the best results.
A centrally led gen AI operating model is beneficial for several reasons:
- Given the scarcity of top gen AI talent, centralization allows the enterprise to allocate talent in a way that is more likely to benefit the entire organization. A centrally led operating model can also help the organization build a world-class, cohesive gen AI team that fosters a sense of camaraderie, helping attract and retain talent.
- In a rapidly changing environment where new large language models and gen AI features are regularly being introduced, a central team can stay on top of the evolving gen AI landscape better than several teams dispersed across an organization.
- A centrally led operating model is useful early on in an enterprise’s gen AI push, when it is necessary to make frequent and important decisions on matters such as funding, tech architecture, cloud providers, large language model providers, and partnerships.
- Risk management and keeping up with regulatory developments are easier with a centrally led approach.
Choosing an operating model isn’t a simple binary approach, however. A financial institution can draw insights from the details explored in this article, decide how much to centralize the various components of its gen AI operating model, and tailor its approach to its own structure and culture. An organization, for instance, could use a centralized approach for risk, technology architecture, and partnership choices, while going with a more federated design for strategic decision making and execution.
The importance of the operating model
An operating model is a representation of how a company runs, including its structure (roles and responsibilities, governance, and decision making), processes (performance management, systems, and technology), and people (skills, culture, and informal networks).
About QuantumBlack, AI by McKinsey
QuantumBlack, McKinsey’s AI arm, helps companies transform using the power of technology, technical expertise, and industry experts. With thousands of practitioners at QuantumBlack (data engineers, data scientists, product managers, designers, and software engineers) and McKinsey (industry and domain experts), we are working to solve the world’s most important AI challenges. QuantumBlack Labs is our center of technology development and client innovation, which has been driving cutting-edge advancements and developments in AI through locations across the globe.
Financial institutions that successfully use gen AI have made a concerted push to come up with a fitting, tailored operating model that accounts for the new technology’s nuances and risks, rather than trying to incorporate gen AI into an existing operating model. We have observed that the majority of financial institutions making the most of gen AI are using a more centrally led operating model for the technology, even if other parts of the enterprise are more decentralized. This is likely to evolve as the technology matures.
The right operating model for a financial-services company’s gen AI push should both enable scaling and align with the firm’s organizational structure and culture; there is no one-size-fits-all answer. An effectively designed operating model, which can change as the institution matures, is a necessary foundation for scaling gen AI effectively.
In essence, a suitable operating model enables the financial institution to efficiently carry out three types of activities:
- Strategic steering . Identify clusters, or domains, of gen AI use cases that align with the enterprise’s strategic objectives; sort them by priority into a road map that maximizes value while managing risk; and monitor value creation in order to ensure efficient resource allocation.
- Standard setting . Define common standards (such as those concerning technology architecture choices, data practices, and risk frameworks and controls) to increase efficiency and use insights learned from completed projects on new ones.
- Execution . Design and test use cases’ technical solutions, put the use cases that meet the appropriate performance and safety criteria into production, and scale them if there is a business case for doing so, ensuring that their impact is tracked and delivered.
Operating-model archetypes for gen AI in banking
Banks and other financial institutions can take different approaches to how they set up their gen AI operating models, ranging from the highly centralized to the highly decentralized.
We recently conducted a review of gen AI use by 16 of the largest financial institutions across Europe and the United States, collectively representing nearly $26 trillion in assets. Our review showed that more than 50 percent of the businesses studied have adopted a more centrally led organization for gen AI, even in cases where their usual setup for data and analytics is relatively decentralized. This centralization is likely to be temporary, with the structure becoming more decentralized as use of the new technology matures. Eventually, businesses might find it beneficial to let individual functions prioritize gen AI activities according to their needs.
Among the financial institutions we studied, four organizational archetypes have emerged, each with its own potential benefits and challenges (exhibit).
Highly centralized
Potential benefits. This structure—where a central team is in charge of gen AI solutions, from design to execution, with independence from the rest of the enterprise—can allow for the fastest skill and capability building for the gen AI team.
Potential challenges. The gen AI team can be siloed from the decision-making process. It can also be distant from the business units and other functions, creating a possible barrier to influencing decisions.
Centrally led, business unit executed
Potential benefits. This archetype has more integration between the business units and the gen AI team, reducing friction and easing support for enterprise-wide use of the technology.
Potential challenges. It can slow execution of the gen AI team’s use of the technology because input and sign-off from the business units is required before going ahead.
Business unit led, centrally supported
Potential benefits. With this archetype, it is easy to get buy-in from the business units and functions, as gen AI strategies bubble from the bottom up.
Potential challenges. It can be difficult to implement uses of gen AI across various business units, and different units can have varying levels of functional development on gen AI.
Highly decentralized
Potential benefits. It is easy to get buy-in from the business units and functions, and specialized resources can produce relevant insights quickly, with better integration within the unit or function.
Potential challenges. Business units that do their own thing on gen AI run the risk of lacking the knowledge and best practices that can come from a more centralized approach. They can also have difficulty going deep enough on a single gen AI project to achieve a significant breakthrough.
The operating model with the best results
At this very early stage of the gen AI journey, financial institutions that have centralized their operating models appear to be ahead. About 70 percent of banks and other institutions with highly centralized gen AI operating models have progressed to putting gen AI use cases into production, 2 Live use cases at minimal-viable-product stage or beyond. compared with only about 30 percent of those with a fully decentralized approach. Centralized steering allows enterprises to focus resources on a handful of use cases, rapidly moving through initial experimentation to tackle the harder challenges of putting use cases into production and scaling them. Financial institutions using more dispersed approaches, on the other hand, struggle to move use cases past the pilot stage.
The nascent nature of gen AI has led financial-services companies to rethink their operating models to address the technology’s rapidly evolving capabilities, uncharted risks, and far-reaching organizational implications. More than 90 percent of the institutions represented at a recent McKinsey forum on gen AI in banking reported having set up a centralized gen AI function to some degree, in a bid to effectively allocate resources and manage operational risk.
Our surveys also show that about 20 percent of the financial institutions studied use the highly centralized operating-model archetype, centralizing gen AI strategic steering, standard setting, and execution. About 30 percent use the centrally led, business unit–executed approach, centralizing decision making but delegating execution. Roughly 30 percent use the business unit–led, centrally supported approach, centralizing only standard setting and allowing each unit to set and execute its strategic priorities. The remaining institutions, approximately 20 percent, fall under the highly decentralized archetype. These are mainly large institutions whose business units can muster sufficient resources for an autonomous gen AI approach.
Centralization isn’t friction free. The main obstacles to implementing a centralized operating model have so far stemmed from disagreements over the strategic road map, funding mechanisms, and talent pooling as units fear losing out on crucial resources or having their operational priorities overlooked.
The financial-services companies that have best managed the transition to gen AI already had a high level of organizational agility, allowing them to quickly rework processes and flexibly pool resources, either by locating them in a central hub or by creating ad hoc, centrally coordinated, agile squads to execute use cases. Compared with a traditional AI squad, gen AI teams tend to feature more significant involvement from cloud engineers, business domain experts, and risk and compliance professionals from the beginning of a use case. This is because of two factors: the highly iterative nature of the gen AI development process and the need to consider, even in the early development stage, unforeseen or speculative implications of scaling the applications.
As gen AI technology and organizations’ grasp of its implications mature, the operating model might swing toward a more federated design in both strategic decision making and execution, while standard setting is the likeliest candidate for continued centralization (for example, in risk management, tech architecture, and partnership choices).
A checklist of essential decisions to consider
Choosing and implementing a gen AI operating model requires leaders at financial institutions to make decisions in various areas, including both those directly implicated in the operating model and those that fall into other areas but affect how the model works. Here is a checklist executives can keep in mind as they come up with the best operating model for their organizations:
- Strategy and vision . First, the financial institution needs to decide which leaders will define its gen AI strategy and whether that will be done on an enterprise-wide or business unit level. This should include a vision for the potential value at stake and an assessment of which functions or processes are likely to be affected the most by gen AI.
- Domains and use cases . Next, the institution should ascertain who will determine the enterprise domains, or clusters, of gen AI use cases and the specific use cases within those domains.
- Deployment model . Regarding the implementation of the domains and use cases, the institution should decide whether it will be a “taker” (procuring targeted solutions from vendors), a “shaper” (integrating broader solutions from vendors), or a “maker” (developing in-house solutions that reshape the core business).
- Funding . The institution will need to set out how gen AI use cases will be funded, which will depend on how centralized or decentralized its gen AI approach is. Banks typically fund use cases through a combination of individual business units and a foundation-building central team dedicated to gen AI.
- Talent . The enterprise should define which skills will be needed for gen AI initiatives, then put in place the necessary talent through hiring, upskilling, strategic outsourcing, or a combination of all these strategies. Another step will be to determine the role of “translators” who understand both the business needs and technical requirements of implementing gen AI use cases and domains.
- Risk . The financial institution should determine who defines risk guardrails (such as those related to data privacy and intellectual property infringement) and mitigation strategies. It should also decide to what extent existing frameworks should be adjusted to account for risks specific to gen AI, including whether additional governance is required for particular use cases (such as customer-facing ones).
- Change management . A committee will need to lead the execution of a change management plan to ensure evolutions in mindsets and behaviors as required for the successful adoption of gen AI across the enterprise.
Without the right gen AI operating model in place, it is tough to incorporate enough structure and move quickly enough to generate enterprise-wide impact. To choose the operating model that works best, financial institutions need to address some important points, such as setting expectations for the gen AI team’s role and embedding flexibility into the model so it can adapt over time. That flexibility pertains to not only high-level organizational aspects of the operating model but also specific components such as funding.
The dynamic landscape of gen AI in banking demands a strategic approach to operating models. Banks and other financial institutions should balance speed and innovation with risk, adapting their structures to harness the technology’s full potential. As financial-services companies navigate this journey, the strategies outlined in this article can serve as a guide to aligning their gen AI initiatives with strategic goals for maximum impact. Scaling isn’t easy, and institutions should make a push to bring gen AI solutions to market with the appropriate operating model before they can reap the nascent technology’s full benefits.
Kevin Buehler is a senior partner in McKinsey’s New York office, where Alison Corsi is a consultant, and Brian Weintraub is a partner; Mina Jurisic is a partner in the Paris office, where Andrea Siani is a consultant; and Larry Lerner is a partner in the Washington, DC, office.
The authors wish to thank Antonio Castro for his contributions to this article.
This article was edited by Jana Zabkova, a senior editor in the New York office.
Explore a career with us
Related articles.

Capturing the full value of generative AI in banking
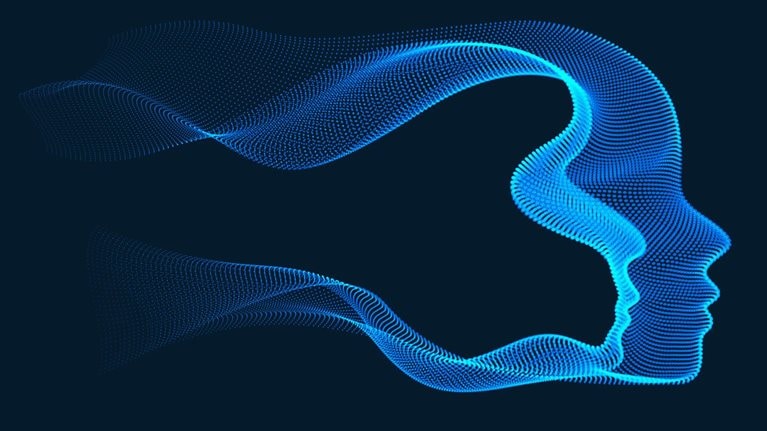
Been there, doing that: How corporate and investment banks are tackling gen AI
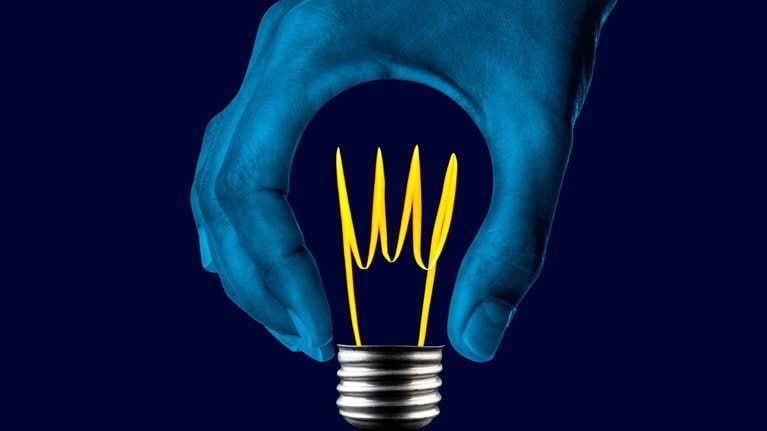
A generative AI reset: Rewiring to turn potential into value in 2024
- Access Points
Managed Switches
- Commercial Access Switches
- Industrial Access Switches
Unlicensed Band Radio
- Point-to-Point
- Point-to-Multipoint
Cloud Networking Platforms
Accessories
- Power Solutions AC /DC PoE Injectors
Passive Optical Network
- Hospitality
- Public Wi-Fi
- Public Hotspots
- Book a Demo
- Banking - SBI
Wireless Technology by HFCL Reshapes India's Largest Bank, Paving the Way to Secure Financial Data
- Case Studies
- Email to Friend
- Download Case Study
Customer Profile
The State Bank of India (SBI) is India's largest and one of the oldest public sector banks and stands as a cornerstone of the country's banking and financial sector. With a legacy of trust and reliability, it is the preferred choice for millions of Indians, offering innovative banking solutions, digital services, and a customer-centric approach. Its strong global presence also positions it as a prominent player in international banking, serving customers across borders. Its Global IT Center (GITC) exemplifies dedication to pushing banking excellence with modern technologies.
- Vertical: Banking and Financial Services
- Location: India
- Type: Public Sector Bank
- Size: One of India's largest banks
Need for high-speed Wi-Fi deployment within the SBI's Global IT Center to support the bank's digital transformation journey and keep up with the growing focus on accelerated digitalization. The existing network was insufficient to handle the comprehensive transition, which involved significant front-end and back-end changes required for the bank's technology-enabled banking landscape.
The Wi-Fi 6 upgrade at the SBI's GITC resulted in an impressive 2x reduction in employee complaints about network slowness, fostering a more efficient and productive work environment. The improved network capabilities and stability empowered the bank's IT team and tech partners to explore innovative applications and services. With Wi-Fi 6 Access Points supporting a vast number of connected devices, the adoption of IoT devices in the banking environment was facilitated. Additionally, the deployed solution enhanced security with advanced features, ensuring robust network and customer data protection against potential cyber threats.
Solution Deployed
The deployed solution comprised both Hardware and Software components, ensuring a ubiquitous, secure, and scalable Wi-Fi Access solution across the GITC Campus.

ion4xi Wi-Fi 6 (2x2)
MU-MIMO high-capacity indoor Access Points

Unified cloud network management for a single window view of the entire network
Get Wired & Wireless Now

Presenting Banking Products
Isabelle, vice-president of customer loyalty and insight at a big bank, has led the development of a package of new products/services for clients, and a five-minute presentation to explain the offering. In a pilot test, where client managers randomly select walk-in customers and offer to go through the presentation, some agree to listen but others don’t have the time. Several months later, when data about client profitability is available, she notices that average profit from clients who listened to the presentation is lower than those who did not. Disappointed by the outcome and at a loss to understand why, she pulls the customer-profile data hoping that data analysis will explain the decrease in profitability.
The overarching objectives are: (i) “Story-telling with data” – Does the presentation work? If so, how can Isabelle tell the story so that it works despite the negative profitability? (ii) Illustrating the role of analytics in the design of experiments and the analyses of results (iii) Understanding how different kinds of analytics (descriptive–predictive–prescriptive) can be used in a business context. The specific analytical tasks required are: (i) To run and interpret a single-variable linear regression model (ii) To run and interpret a multi-variable linear regression model (iii) To build visualizations to explain the differences between the findings of the two models.
- Story-telling with data
- descriptive analytics
- predictive analytics
- design of experiment
- analyses of experiments
- A/B testing
- visualization
- new product development

Anton S. Ovchinnikov

Ilia Tsetlin
Recommended cases.
Capital Markets or Alms?: An Emerging Paradigm Shift in Disaster Funding

Reference 5408
Published 01 Nov 2006
Length 11 page(s)
Topic Economics & Finance
Region Global
Industry Insurance
Royal Bank of Canada: Creating Profitable Relations with Small Business Clients
Reference 5287
Published 31 May 2005
Length 14 page(s)
Region Other Regions
Industry Financial Services
HSBC Holdings PLC - Building a Global Wholesale Banking Capability
Reference 5482
Published 29 Nov 2007
Length 23 page(s)
Industry Investment Banking
Recently Viewed
Board Process Simulation (A)
By Stanislav Shekshnia
Birkenstock: Exit the Family. Enter a Professional CEO
By Morten Bennedsen , Mark Stabile , Brian Henry
Rasurel: Reviving an Ageing Brand
By Amitava Chattopadhyay , Séverine de Wulf

Product details


Enter the URL below into your favorite RSS reader.
A case study of the assistive technology network in Sierra Leone before and after a targeted systems-level investment
- Citation (BibTeX)

Sorry, something went wrong. Please try again.
If this problem reoccurs, please contact Scholastica Support
Error message:
View more stats
Many people with disabilities in low-income settings, such as Sierra Leone, do not have access to the assistive technology (AT) they need, yet research to measure and address this issue remains limited. This paper presents a case study of the Assistive Technology 2030 (AT2030) funded Country Investment project in Sierra Leone. The research explored the nature and strength of the AT stakeholder network in Sierra Leone over the course of one year, presenting a snapshot of the network before and after a targeted systems level investment.
Mixed-method surveys were distributed via the Qualtrics software twice, in December 2021 and September 2022 to n=20 and n=16 participants (respectively). Qualitative data was analyzed thematically, while quantitative data was analyzed with the NodeXL software and MS Excel to generate descriptive statistics, visualizations, and specific metrics related to indegree, betweenness and closeness centrality of organizations grouped by type.
Findings suggest the one-year intervention did stimulate change within the AT network in Sierra Leone, increasing the number of connections within the AT network and strengthening existing relationships within the network. Findings are also consistent with existing data suggesting cost is a key barrier to AT access for both organizations providing AT and people with disabilities to obtain AT.
While this paper is the first to demonstrate that a targeted investment in AT systems and policies at the national level can have a resulting impact on the nature and strength of the AT, it only measures outcomes at one-year after investment. Further longitudinal impact evaluation would be desirable. Nonetheless, the results support the potential for systemic investments which leverage inter-organizational relationships and prioritize financial accessibility of AT, as one means of contributing towards increased access to AT for all, particularly in low-income settings.
Assistive technology (AT) is an umbrella term which broadly encompasses assistive products (AP) and the related services which improves function and enhances the user’s participation in all areas of life. 1 Assistive products are “any external products (including devices, equipment, instruments and software) […] with the primary purpose to maintain or improve an individual’s functioning and independence and/or well-being, or to prevent impairments and secondary health conditions”. 2
Recently, awareness for the urgent need to improve access to Assistive Technology has expanded, as 2022 global population statistics highlights one in three people, or 2.5 billion people, requires at least one assistive product. 1 The demand for AT is projected to increase to 3.5 billion people by 2050, yet 90% of them lack access to the products and services they need. 1 , 3 A systemic approach which adequately measures outcomes and impact is urgently required to stimulate evidence-based policies and systems which support universal access to AT. 1 , 4 , 5 However, a systemic approach first necessitates baseline understandings of the existing system, inclusive of sociopolitical context and the key stakeholders working within that context.
Assistive technology is necessary for people with disabilities to engage in activities of daily living, such as personal care or employment, and social engagement. 6 Moreover, people with disabilities also require AT to enact their basic human rights, as outlined in the United Nations Convention on the Rights of Persons with Disabilities (UNCRPD). 7 Unfortunately, many people do not have access to the AT they require, an inequity which is perpetuated within low-income settings. 8 Despite this growing disparity and a well-documented association between poverty and disability, 9 research gaps remain related to AT within low-income settings in the global South. 10
In Sierra Leone, the national prevalence of disability is estimated to be 1.3%, according to the most recent population and housing census data. 11 , 12 This is unusually low, as compared to the 16% global prevalence (World Health Organization, 2022). National stakeholders within the AT network argue this statistic does not adequately represent the true scope of disability in Sierra Leone. 10 Their stance is supported by survey data from the Rapid Assistive Technology Assessment (rATA) across a subset of the population in Freetown, which indicated a dramatically different picture: a 24.9% prevalence of self-reported disability on the basis of the Washington Group Questions (20.6% reported as having “some difficulty”, while 4.3% rated “a lot of difficulty” or above), predominantly mobility and vision related disabilities. 13 The rATA also highlighted 62.5% of older people surveyed indicated having a disability, while the incidence of disability among females was nearly 2% higher than in males. 13
Despite the 2011 Sierra Leone Disability Act being implemented, access to AT in Sierra Leone remains poor. 13 The rATA suggests only 14.9% of those with disabilities in Freetown have the assistive products they require, an alarming rate which also fails to consider people with disabilities not surveyed in rural Sierra Leone where access to such services is likely lower. 13 Meanwhile, it is estimated over half of the population of Sierra Leone lives in poverty, with 13% in extreme poverty. 14 As affordability ranks as the top barrier for AT access, poverty further perpetuates the challenges of people with disabilities within this subset of the population to access necessary AT. 13 Within the context of low-resource settings it is therefore imperative that those resources which are allocated to provide assistive products are used in the most optimal manner, and that different stakeholders work together to co-construct a systemic approach which can identify and prioritise those most in need.
This paper presents a dataset collected in tandem with an Assistive Technology 2030 (AT2030) funded Country Investment project in Sierra Leone in collaboration with Clinton Health Access Initiative (CHAI). The study aimed to explore the nature and strength of the assistive technology stakeholder network in Sierra Leone over the course of one year through a mixed methods survey methodology. We provide a systemic snapshot of the AT network in Sierra Leone, highlighting what assistive products are available, who provides and receives them, and how. We also present a relational analysis of the existing AT network, inclusive of the organizations working within areas of AT and their degrees of connectivity and collaboration amongst one another. We hope that such data can strengthen the provision of AT in Sierra Leone through identifying assistive product availability, procurement, and provision, as well as the nature of the relationships between (the relationality ) of the AT network. We also sought to provide an overview of any possible changes to the network over the course of a one-year investment by AT2030.
This study used a mixed methods survey approach, facilitated by Qualtrics online survey software. Surveys were collaboratively developed and distributed at the two time periods in December 2021 and September 2022 (herein respectively described as Baseline=T1 and Follow Up= T2).
Intervention
This paper presents the Sierra Leone country project built within a larger, targeted investment in assistive technology systems development in four African countries,by AT2030, a project led by the Global Disability Innovation Hub and funded by UK Aid. The four in-country projects were administered by Clinton Health Access Initiative (CHAI) in partnership with local government ministries and agencies. As part of this investment, CHAI and its partners convened a Technical Working Group which brought together key stakeholders in the assistive technology field. Over the course of one year, the Technical Working Group had an overarching goal to develop and strengthen key assistive technology related policies in each of the four countries. The data in this study on the AT network in Sierra Leone was collected at the outset and following completion of the AT2030 investment, by researchers who were not part of the investment process, thus allowing for third-party evaluation. To maintain objectivity, neither CHAI nor the funder were responsible for the design, data collection, analysis or reporting of results, but this paper has benefited from a programmatic perspective provided by CHIA.
Participants
Participants included members of relevant ministries involved in assistive technology leadership and/or delivery, and staff representing relevant non-profit organizations (both international and local), service providers and organizations for persons with disabilities. Participants were asked to respond on behalf of their organization. All prospective participants were identified by the researchers and local project partners, including those coordinating the investment identified above, and added to a distribution list on Qualtrics, which only contained pertinent identifying information such as name, organization, and email. Over the course of the study, n=20 (T1) and n=16 (T2) participants consented to and completed surveys. While the relatively small sample size may inherently restrict the generalizability of this study, the sample size is reflective of the size of the assistive technology network in Sierra Leone, which we aimed to explore.
Data collection
The survey was emailed to the distribution list at two time points: December 2021 (T1) and September 2022 (T2). Two reminder emails were sent out via Qualtrics at two-week intervals following each time point, to participants who had not yet completed the surveys as a means to stimulate participant retention. The T1 and T2 surveys were identical, however the T2 survey utilized display logic functionalities such as conditional skipping to prevent retained respondents from completing redundant questions such as demographic information. If a participant completed the survey for the first time during the T2 period, they received the survey in its entirety without conditional skipping.
Survey content
Survey questions aimed to capture what AT is available, how it is being provided, who is receiving it and how. Questions also consisted of demographic information and qualitative prompts to identify participants’ roles within the AT network and critical challenges experienced in enacting their roles, as well as the nature and strength of relationships between stakeholders. Additional data was collected on participatory engagement in policy development, knowledge of assistive technology, and capacity for leadership which will be published separately.
Using the methodology reported by Smith and colleagues, 15 the WHO priority assistive products list was provided for respondents to select the products and associated services their organization provides. Additionally, the survey requested respondents to select from a list of organizations, which ones they were aware of as working within AT areas in Sierra Leone, followed by a subsequent 5-point Likert scale (1-5, 1= no relationship, 5= collaboration) to indicate which organizations they had working relationships with and to what extent. In attempts to maximize response rates and maintain participant retention, two reminder emails were sent to participants for T1 and T2; however, challenges encountered were participant drop-out from T1 to T2.
Data analysis
Data was reviewed across the two time periods and descriptive statistics (counts and means) were calculated for all variables using MS Excel software. Qualitative data employed content analysis of the text responses from each open-ended survey question, with a particular emphasis on themes which represented commonalities or a lack of representation across all stakeholders. Network data was analyzed using the NodeXL software and MS Excel to generate visualizations, and specific metrics related to indegree, betweenness and closeness centrality of organizations grouped by organization type. Indegree represents the total number of incoming connections per organization, while weighted indegree represents the sum of weights (strength) of each connection. Closeness centrality represents the relationship of the organization to the centre of the network (lower scores indicate greater centrality). To accommodate for different response rates at baseline and follow up, indegree was calculated as a proportion of incoming connections out of the total respondents (n) for that time point. Weighted indegree was calculated as a proportion of the sum of weights of incoming connections divided by the total possible weighting for the respondents for that time point (i.e. n*5). Statistical comparisons for overall network metrics across T1 and T2 were calculated using a paired t-test in SPSS v.28. While means are also reported by organization type as a subsample of the overall data, no statistical tests were carried out due to small subsample sizes.
The study received ethical approval from Maynooth University and the Sierra Leone Ethics and Scientific Review Committee. Each survey contained a mandatory informed consent section which required completion prior to respondents accessing the survey questions. Respondents were not required to answer any specific questions and were not coerced to participate. All respondents received a unique identification code to preserve anonymity, and any identifying information was removed prior to data analysis.
A total of 27 participants from 24 organizations participated in the surveys across both baseline and follow-up time points (T1 n=20 and T2 n= 16). Nine individuals and 11 organizations were retained across both T1 and T2 surveys. The majority of participants represented International non-governmental organizations (n=9), followed by Organizations of Persons with Disabilities (n=8), Government Ministry (n=4), Service Delivery organisations (n=4) and Academic Institutions (n=2).
Additionally, the respondents were requested to identify multiple areas of AT that their organizations were aligned with. Advocacy ranked as the top selection (24.5%), followed by direct service provision (14.9%), human resources and capacity building (14.9%), policy or systems development (13.8%), product selection and/or procurement (13.8%), data and information systems (11.7%), and financing (6.4%).
Assistive Products in Sierra Leone
Participants were asked to select from the APL which products and/or product services they provide. Manual wheelchairs, crutches, canes, lower limb prosthetics and orthopaedic footwear were the most selected across both time points. Table 1 lists summarises the types of assistive products and services provided in Sierra Leone, and the number of organisations providing each product and/or service across all 50 APL products.
No products or services provided | Alarm signallers, audio players, closed captioning displays, fall detectors, global positioning locators, hearing loops/FM systems, magnifiers (digital hand-held and optical), personal emergency alarm, pill organizers, watches |
1 organization providing product or service | Braille displays/note takers, communication software, gesture to voice technology, incontinence products, keyboard and mouse emulation software, pressure relief mattresses, screen readers, simplified mobile phones, tablets*, upright supportive chair and table for children*, rubber tips*, pencil grips*, adapted cups*, sponges*, weighted spoons*, weighted vests*, rollators**, time management products**, travel aids** |
2-3 organizations providing product or service | Communication boards, deafblind communicators, hearing aids, orthoses (lower limb, spinal and upper limb), personal digital assistant, pressure relief cushions, prostheses (lower limb), recorders, spectacles, therapeutic footwear, video communication devices, walking frames, wheelchairs (power), |
4-5 organizations providing product or service | Braille writing equipment, canes/sticks, clubfoot braces, handrails/grab bars, standing frames, tricycles, white canes, |
6-9 organizations product or service | Chairs for shower/bath/toilet, ramps |
10 or more organizations | Crutches/axillary, wheelchairs (manual) |
*Other assistive product offered but not on the Assistive Product List **Assistive product not provided, only service related to the prescription, servicing and maintenance, and customization of that Assistive product
Respondents indicated that the products they provide were most commonly procured by their organizations through purchase (38.7%), followed by donation (29%), building products themselves (22.6%) or other (9.7%), which was explicated as recycling used products.
Providers of Assistive Products in Sierra Leone
Participants were asked to indicate whether their organization provided assistive products and/or related services. The findings highlighted 38.3% of stakeholders directly provided AT and 40.4% directly provided AT related services to beneficiaries, while only 21.3% indicated they do not provide AT or AT related services at all.
More specifically, respondents who did indicate providing products and/or services indicated they provided the following services: provision of locally made assistive products, repairs and maintenance of assistive products, education and training of users on the utility of assistive products, referrals of people with disabilities to service providers, prosthetic and orthotics, accessibility assessments, and rehabilitation service provision. Participants whom do not directly provide AT or AT related services indicated their work falls within AT advocacy, fundraising, procurement, policy, and research.
When asked about the challenges they experienced procuring and distributing these products to beneficiaries, qualitative data indicated difficulty sourcing materials, challenges obtaining products due to poor infrastructure, poor quality standards and/or customizability of products, and low technical and managerial support as common barriers. High product and material costs and inadequate funds from both the organizations and beneficiaries was the most commonly cited challenge.
Beneficiaries of Assistive Products in Sierra Leone
When probed on the number of clients they served each month, respondents indicated the range of beneficiaries spanned from as little as 10 per month to upwards of 1000, while one respondent noted there was no fixed number as they serve at the national level. Respondents noted that their beneficiaries were predominantly people with mobility related disabilities or functional limitations (21.4%), closely followed by people with vision disabilities (17.9%), communication disabilities (15.4%) and hearing disabilities (13.1%).
Participants emphasized children and adolescents were the highest populations served, with an equal representation among the ages of 5-12 (23.7%) and 13-18 (23.7%). Adults aged 20-50 years (21%) closely followed, while children under 4 (15.8%) and adults over 50 years of age (15.8%) are equally less represented as beneficiaries of assistive products and services in Sierra Leone.
Respondents whose organizations provide assistive products indicated that their beneficiaries most commonly received APs free of cost (63.2%), followed by client payment (26.3%) and a fixed cost structure (10.5%).
Network Analysis
Respondents were asked to indicate which organizations in the AT network they were aware of, and subsequently to rate the strength of their relationship with the organizations they indicated an awareness of. The degree of relationality among these stakeholders involved in the assistive technology network was then analyzed across the two time points and organizational relationships were visualized using the NodeXL software, presented in Figure 1 and Figure 2 . The colored nodes in the figures depict the various sub-types of organizations, while the lines between the nodes represent their relationships, with thicker lines indicating stronger relationships. The red nodes represent government ministries and agencies, the green represent service delivery organizations, blue represents organization of persons with disabilities, black represents NGOs and yellow represents academic institutions.

Overall, this representation depicts a relatively centralized network with a higher degree of connections between organizations. Furthermore, ministries and government agencies appear towards the centre of the network, indicating a relatively greater role in connecting organizations to one another, however it is noteworthy that these are not the most central organizations in the network.
Table 2 provides quantitative data which demonstrates the overall number and strength of interconnections among the organizations within the assistive technology network in Sierra Leone. Indegree is the number of identified inward connections, or the number of other organizations who identified a connection with that organization. Indegree data are presented as a mean value per organization type to preserve anonymity. The data visualized in Table 2 significantly increased over one year from baseline to follow up, while the relative centrality of organizations did not change, at least over the one-year time period of this study.
Organization Type | Indegree Mean (SD) | Weighted Indegree Mean (SD) | Closeness Centrality Mean (SD) | |||
Baseline | Follow Up | Baseline | Follow Up | Baseline | Follow Up | |
Ministry or Government Agency | 0.46 (0.11) | 0.50 (0.16) | 9.07 (3.49) | 10.36 (4.34) | 0.53 (0.01) | 0.64 (0.14) |
Organization of Persons with Disabilities | 0.23 (0.07) | 0.38 (0.11) | 3.73 (0.91) | 6.73 (2.19) | 0.54 (0.09) | 0.58 (0.13) |
Service Delivery Organization | 0.34 (0.06) | 0.48 (0.09) | 6.00 (1.52) | 7.84 (2.76) | 0.57 (0.13) | 0.53 (0.01) |
Local NGO | 0.24 (0.07) | 0.40 (0.17) | 4.48 (2.05) | 6.96 (3.87) | 0.52 (0.01) | 0.52 (0.02) |
International NGO | 0.27 (0.07) | 0.40 (0.16) | 4.90 (1.64) | 7.29 (3.77) | 0.55 (0.07) | 0.56 (0.07) |
Overall | 0.29 (0.11) | 0.42* (0.14) | 5.12 (2.34) | 7.51* (3.31) | 0.54 (0.08) | 0.56 (0.10) |
SD – standard deviation, NGO – non-governmental organization *Differs significantly from baseline at p<0.01 (two-tailed)
Overall, there was a statistically significant increase in indegree scores between the two timepoints suggesting a higher level of connection among AT organizations in Sierra Leone following the 1-year investment. This suggests those organizations built more relationships and expanded their reach within the AT network. As relationship strength was measured on a 5-point scale (no awareness, awareness, communication, cooperation, collaboration), we can interpret increases in weighted indegree to suggest greater inter-organizational working between members of the network (please refer to Table 2 ).
These findings suggest the one-year intervention did indeed stimulate change within the AT network in Sierra Leone, increasing the number connections within the AT network, and strengthening existing relationships within the network.
The most common assistive products available in Sierra Leone were indicated to be manual wheelchairs, crutches, canes, lower limb prosthetics and orthopaedic footwear. This aligns with our participants ranking mobility related disabilities or functional limitations as the most common reason for beneficiary referral, as well as the rATA data 13 ). The global report on AT notes “the type, complexity, magnitude and duration of a humanitarian crisis impacts the need for and supply of assistive technology”. 1 When we factor in the sociopolitical context of Sierra Leone and its history of civil war, and the population requiring these products due to political violence, such as lower limb amputations, it is also not surprising that mobility related products are so widely available due to population need. Moreover, as many low-income settings procure their products through donations, often from abroad, these items are probable to be in high circulation in relation to the high global prevalence of mobility related disabilities, likely shaping what products donors perceive as being most relevant. 1
Interestingly, data from the rATA shows the people with disabilities who did have AP, most often obtained their product(s) through purchase, despite cost being the most significant barrier to access. 13 As such, these APs were often purchased through informal and unregulated providers who offer lower costs, such as market vendors. 16 In comparison, our findings demonstrated AT stakeholders providing AP did so predominantly at no-cost. This discrepancy could suggest those who need AT most are not aware of the regulated providers who offer free AP and/or AP services in Sierra Leone, or they simply cannot access them due to infrastructural barriers, or not having AT needed to navigate their environment in the first place. For example, our data highlighted only two organizations offering spectacles, yet the rATA indicated spectacles as being the most common AP obtained by people with disabilities sampled in Sierra Leone. This further supports our interpretation that access to free APs is limited if only a small subset of regulated providers are offering them, leading to an increased reliance on people with disabilities procuring APs from informal and unregulated providers in Sierra Leone. An interconnected and coherent national AT network could offer a way forward, should collaborative relationships among AT stakeholders continue to forge and their collective resources, contacts and beneficiaries were to be cross-pollinated for the advancement of beneficiary access.
As technology and what constitutes as AT continues to advance, juxtaposed with the prevalence of disability increasing, there is a risk that the gap in access to AT will continue to rise. 17 It is therefore paramount that the goal of improving AT related outcomes, such as improved access to AT for all, is first warranted by the measurement of such outcomes. 4 This paper has attempted to provide a systemic snapshot of the AT network in Sierra Leone, highlighting key information such as what assistive products are presently available, who provides them, who receives them (and how), and the relational cohesion of the network itself.
This paper is the first to demonstrate that a targeted investment in assistive technology systems and policies at the national level can have a resulting impact on the nature and strength of the assistive technology ecosystem relationships. It is therefore recommended as an intervention to engage stakeholders within the assistive technology space, in particular policy makers who have power to formulate AP related policy and access. However, this work is limited in scope as it only provides a reassessment of outcomes following the one-year investment, and does provide a more longitudinal evaluation of the impact of that investment in the longer term.
Future research is recommended to replicate the work done to date to evaluate whether there is an improvement in access to assistive technologies over a longer period of time as a result of targeted policy and systems change, as well as larger impacts on policy formulation for AP access. For example, attention to data collection of which types and categories of AP are being manufactured locally can inform policy formation to encourage continuity of local manufacturing, while improving access to AP. Moreover, further studies to investigate factors influencing limited uptake of free AP by persons with disabilities, as explicated above and discovered in this study, are recommended.
CONCLUSIONS
Cohesive AT networks are particularly important in low-income settings such as Sierra Leone, where the intersection of poverty and disability disproportionately reduces people with disabilities’ access to the AT they need. Power and colleagues 18 have proposed the Assistive Technology Embedded Systems Thinking (ATEST) Model as a way of conceptualising the embedded relationships between individual-community- system-country-world influences on assistive technology provision. This paper suggests that even where resources are scarce and systemic relationships are uneven, an internationally-funded investment, which embraces the participation of country-level stakeholders and service providing organisations can result in enhanced inter-organisational working, which in turn has the potential to use existing resources more optimally, allowing greater access to services for individuals most in need.
The findings of this paper demonstrate an increase in organizational collaboration can strengthen assistive technology networks, however key barriers to access remain cost for both organizations providing AT and people with disabilities to obtain AT. Future work should use systemic approaches to leverage organizational relationality and prioritize financial accessibility of AT within systemic approaches to AT policy and practice, to leverage existing resources (particularly no-cost AT) and advance towards the ultimate goal of increased access to AT for all.
Ethics Statement
Ethical approval for the study was granted by Maynooth University and the Sierra Leone Ethics and Scientific Review Committee. The study involved human participants but was not a clinical trial. All participants provided informed consent freely and were aware they could withdraw from the study at any time.
Data Availability
All data generated or analysed during this study are included in this article.
This work was funded by the Assistive Technology 2030 project, funded by the United Kingdom Foreign Commonwealth Development Office (FCDO; UK Aid) and administered by the Global Disability Innovation Hub.
Authorship Contributions
Stephanie Huff led the manuscript preparation and contributed to data analysis. Emma M. Smith led the research design, data collection, analysis and contributed to manuscript preparation. Finally, Malcolm MacLachlan contributed to research design, analysis, manuscript review, and supervision. All authors read and approved the final manuscript.
Disclosure of interest
The authors completed the ICMJE Disclosure of Interest Form (available upon request from the corresponding author) and disclose no relevant interests.
Correspondence to:
Emma M. Smith Maynooth University Maynooth, Co. Kildare Ireland [email protected]
Submitted : February 27, 2024 BST
Accepted : June 26, 2024 BST

IMAGES
COMMENTS
1. Islamic Bank in Qatar: Creating Omni-Channel Experience 👏🏻 3.7K applause. Case study by Atishay Goyal. A team of 8 developers, 1 Business Analyst, and 1 UX designer was setup onsite in Qatar to execute the revamp of CB's digital presence and an Omni-Channel experience to its customers.
Related: FinTech vs Investment Banking . Case Study 20: Lemonade - Disrupting Insurance with AI and Behavioral Economics Background. Founded in 2015, Lemonade represents a new wave of InsureTech companies aiming to revolutionize the traditional insurance model.
In this case, the real issue for customers was how the bank delivered the conversation about loan pricing. Against this deeper understanding of the sources of customer experience, the team was then able to put in place an iterative process of developing and testing rapid prototypes of minimum viable products and refining knowledge with each new ...
In this case study, we look at how Kiwibank, the largest New Zealand-owned bank, is using data and AI to reach new levels of digital transformation in banking. ... by McKinsey is our tech strategy and delivery unit of exceptional technologists building robust software and digital products to meet our clients' needs. Learn more.
Between 2008 and 2014, the Top 4 banks sharply decreased their lending to small business. This paper examines the lasting economic consequences of this contraction, finding that a credit supply shock from a subset of lenders can have surprisingly long-lived effects on real activity. 26 Jun 2017. Working Paper Summaries.
Published July 22, 2022 By Liz Llewellyn-Maxwell. As the world grows more and more digital, customers across every industry are expecting digital-first, timely assistance and support. As shown in this digital transformation in banking case study roundup, all businesses — including banks — have been forced to change and evolve with the times.
Case Study 2: Bank of America: Erica, the AI-Powered Financial Assistant Challenge: ... This advancement aims to streamline the process and tailor mortgage products more specifically to individual needs, setting a new standard in personalized banking services. Case Study 10: Standard Chartered: Streamlining Trade Finance Operations ...
A McKinsey survey of US retail banking customers found that at the banks with the highest degree of reported customer satisfaction, deposits grew 84 percent faster than at the banks with the lowest satisfaction ratings (Exhibit 1). 1. Superior experiences are not only a proven foundation for growth but also a crucial means of countering threats ...
This case study presents insights from customer research with TymeBank clients that bolsters CGAP's hypotheses around how digital banks can support the mission of financial inclusion. As a fully digital South African bank that disproportionately serves low-income rural customers, TymeBank has created a suite of basic products that cater to ...
Case Study: How a global bank increased cross-selling effectiveness and realized a significant increase on commercial performance. Data analysis, strategic digital tools, and nudges can enhance the commercial effectiveness of bank relationship managers when up-selling and cross-selling high value products and services. Cross-selling is a ...
Digital transformation enables banks to gain deeper insights into customer behavior, preferences, and needs. By analyzing customer data, banks can offer personalized services, targeted marketing campaigns, and customized product recommendations, leading to higher customer satisfaction and loyalty.
4. Accessibility features uneven across sector: Traditional banks are more likely than digital banking providers to offer their websites, apps, and policies in Spanish. Banking websites are more likely than banking apps to have built-in accessibility features for people with disabilities. Financial products should be accessible to all users.
ly 2023, or around 10% of the population, according to Openbanking.org.uk2. According to the same study, consumers see open banking for its benefits and associate 60% of use cases with a better customer experience (in terms of speed and. easiness), suggesting an improving understanding of the potential benefits. Another factor that is help.
A bank that curates a platform of third-party products that complement the bank's own banking services (e.g., Banco Inter in Brazil and Starling Bank in the United Kingdom).1 The marketplace approach gives customers from different segments access to a wide range of services. • Banking as a service (BaaS).
Advanced Analytics in BFSI - Benefits. Updating the data analytics use cases in banking and financial services with the evolving data science methodologies can help organizations sustain stronger customer relationships. Let us look at a few more benefits of advanced analytics. Customer 360-degree insights - By leveraging advanced analytics ...
No. 1: Account aggregation. Open banking offers secure APIs for accessing financial account data and benefits all parties: banks, account owners, and fintechs. With open banking, banks have greater control and visibility into third parties that are accessing their clients' financial data and the purpose for which the data is being used.
The entire organization "flipped" to the new model in March 2022. This case study analyzes the transformation journey using Org Topologies™ Scans and focuses on the Business Banking Hub. It covers three organizational design phases: Org scan of the pre-agile setup before August 2021. Org scan of the 2022 dVM pilot.
The McKinsey Global Institute (MGI) estimates that across the global banking sector, gen AI could add between $200 billion and $340 billion in value annually, or 2.8 to 4.7 percent of total industry revenues, largely through increased productivity. 1 However, as banks and other financial institutions move to quickly implement the technology ...
Our analysis indicates that there are hundreds of use cases for generative AI in banking. Productivity is the most obvious benefit. As Figure 2 shows, there is greater potential to boost output in banking than in any other industry. Banking. Insurance. Capital Markets. Software & Platforms. Communications & Media.
The State Bank of India (SBI) is India's largest and one of the oldest public sector banks and stands as a cornerstone of the country's banking and financial sector. With a legacy of trust and reliability, it is the preferred choice for millions of Indians, offering innovative banking solutions, digital services, and a customer-centric approach.
Case study. experienceKotak Mahindra Bank delivers on its customers' expectations, with a frictionless corporate banking experience powered by Finastra. tions."As well as helping us to offer a seamless, consolidated user journey for our corporate customers across almost any device, Finastra Corporate Channels has greatly reduced the time-to ...
Isabelle, vice-president of customer loyalty and insight at a big bank, has led the development of a package of new products/services for clients, and a five-minute presentation to explain the offering. In a pilot test, where client managers randomly select walk-in customers and offer to go through the presentation, some agree to listen but others don't have the time. Several months later ...
Abstract. Isabelle, vice-president of customer loyalty and insight at a big bank, has led the development of a package of new products/services for clients, and a five-minute presentation to explain the offering. In a pilot test, where client managers randomly select walk-in customers and offer to go through the presentation, some agree to ...
Assistive technology (AT) is an umbrella term which broadly encompasses assistive products (AP) and the related services which improves function and enhances the user's participation in all areas of life. 1 Assistive products are "any external products (including devices, equipment, instruments and software) […] with the primary purpose to maintain or improve an individual's ...