Have a language expert improve your writing
Run a free plagiarism check in 10 minutes, generate accurate citations for free.
- Knowledge Base
Methodology
- Random vs. Systematic Error | Definition & Examples

Random vs. Systematic Error | Definition & Examples
Published on May 7, 2021 by Pritha Bhandari . Revised on June 22, 2023.
In scientific research, measurement error is the difference between an observed value and the true value of something. It’s also called observation error or experimental error.
There are two main types of measurement error:
Random error is a chance difference between the observed and true values of something (e.g., a researcher misreading a weighing scale records an incorrect measurement).
- Systematic error is a consistent or proportional difference between the observed and true values of something (e.g., a miscalibrated scale consistently registers weights as higher than they actually are).
By recognizing the sources of error, you can reduce their impacts and record accurate and precise measurements. Gone unnoticed, these errors can lead to research biases like omitted variable bias or information bias .
Table of contents
Are random or systematic errors worse, random error, reducing random error, systematic error, reducing systematic error, other interesting articles, frequently asked questions about random and systematic error.
In research, systematic errors are generally a bigger problem than random errors.
Random error isn’t necessarily a mistake, but rather a natural part of measurement. There is always some variability in measurements, even when you measure the same thing repeatedly, because of fluctuations in the environment, the instrument, or your own interpretations.
But variability can be a problem when it affects your ability to draw valid conclusions about relationships between variables . This is more likely to occur as a result of systematic error.
Precision vs accuracy
Random error mainly affects precision , which is how reproducible the same measurement is under equivalent circumstances. In contrast, systematic error affects the accuracy of a measurement, or how close the observed value is to the true value.
Taking measurements is similar to hitting a central target on a dartboard. For accurate measurements, you aim to get your dart (your observations) as close to the target (the true values) as you possibly can. For precise measurements, you aim to get repeated observations as close to each other as possible.
Random error introduces variability between different measurements of the same thing, while systematic error skews your measurement away from the true value in a specific direction.
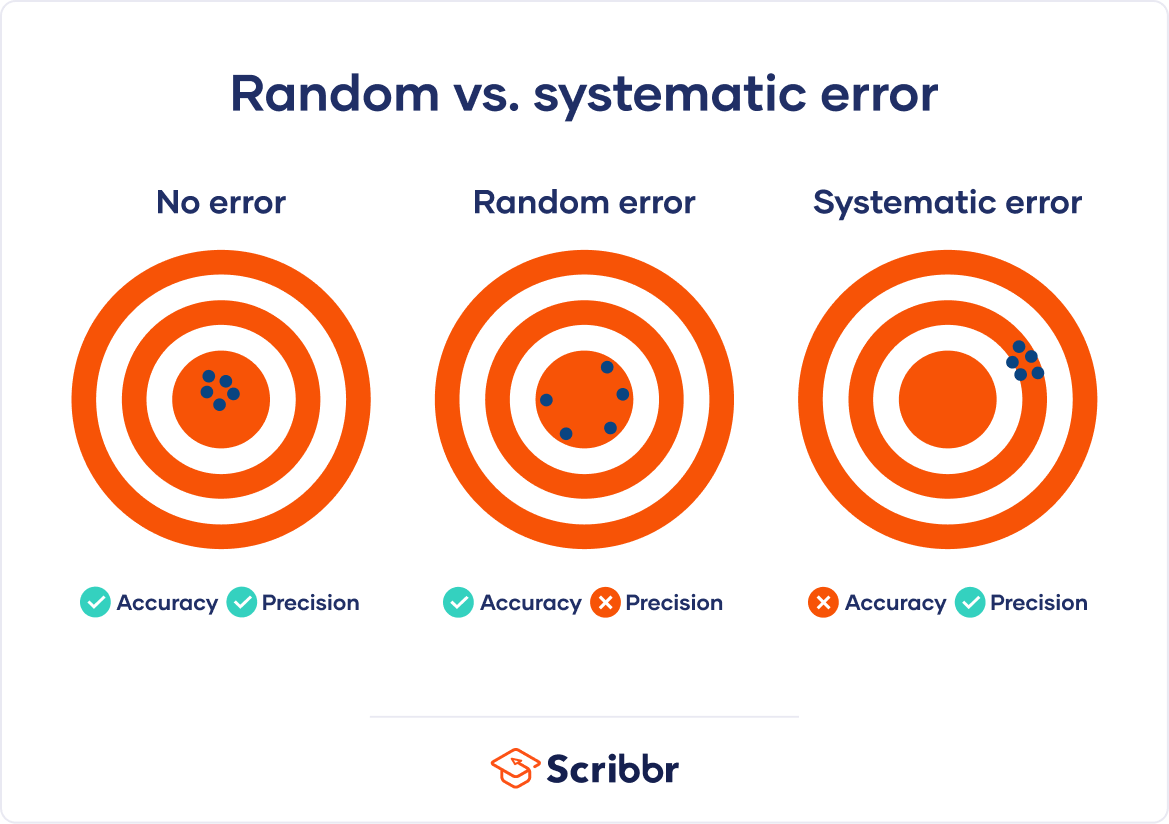
When you only have random error, if you measure the same thing multiple times, your measurements will tend to cluster or vary around the true value. Some values will be higher than the true score, while others will be lower. When you average out these measurements, you’ll get very close to the true score.
For this reason, random error isn’t considered a big problem when you’re collecting data from a large sample—the errors in different directions will cancel each other out when you calculate descriptive statistics . But it could affect the precision of your dataset when you have a small sample.
Systematic errors are much more problematic than random errors because they can skew your data to lead you to false conclusions. If you have systematic error, your measurements will be biased away from the true values. Ultimately, you might make a false positive or a false negative conclusion (a Type I or II error ) about the relationship between the variables you’re studying.
Receive feedback on language, structure, and formatting
Professional editors proofread and edit your paper by focusing on:
- Academic style
- Vague sentences
- Style consistency
See an example
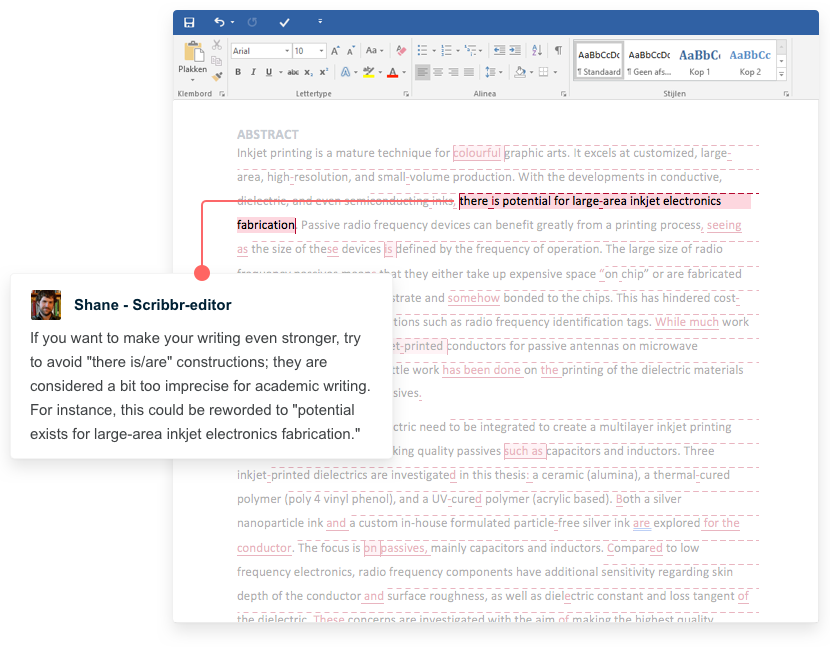
Random error affects your measurements in unpredictable ways: your measurements are equally likely to be higher or lower than the true values.
In the graph below, the black line represents a perfect match between the true scores and observed scores of a scale. In an ideal world, all of your data would fall on exactly that line. The green dots represent the actual observed scores for each measurement with random error added.
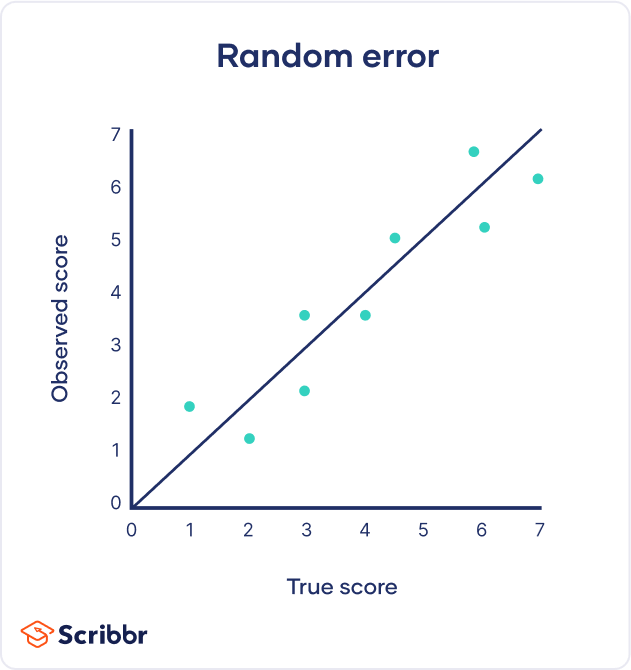
Random error is referred to as “noise”, because it blurs the true value (or the “signal”) of what’s being measured. Keeping random error low helps you collect precise data.
Sources of random errors
Some common sources of random error include:
- natural variations in real world or experimental contexts.
- imprecise or unreliable measurement instruments.
- individual differences between participants or units.
- poorly controlled experimental procedures.
Random error source | Example |
---|---|
Natural variations in context | In an about memory capacity, your participants are scheduled for memory tests at different times of day. However, some participants tend to perform better in the morning while others perform better later in the day, so your measurements do not reflect the true extent of memory capacity for each individual. |
Imprecise instrument | You measure wrist circumference using a tape measure. But your tape measure is only accurate to the nearest half-centimeter, so you round each measurement up or down when you record data. |
Individual differences | You ask participants to administer a safe electric shock to themselves and rate their pain level on a 7-point rating scale. Because pain is subjective, it’s hard to reliably measure. Some participants overstate their levels of pain, while others understate their levels of pain. |
Random error is almost always present in research, even in highly controlled settings. While you can’t eradicate it completely, you can reduce random error using the following methods.
Take repeated measurements
A simple way to increase precision is by taking repeated measurements and using their average. For example, you might measure the wrist circumference of a participant three times and get slightly different lengths each time. Taking the mean of the three measurements, instead of using just one, brings you much closer to the true value.
Increase your sample size
Large samples have less random error than small samples. That’s because the errors in different directions cancel each other out more efficiently when you have more data points. Collecting data from a large sample increases precision and statistical power .
Control variables
In controlled experiments , you should carefully control any extraneous variables that could impact your measurements. These should be controlled for all participants so that you remove key sources of random error across the board.
Systematic error means that your measurements of the same thing will vary in predictable ways: every measurement will differ from the true measurement in the same direction, and even by the same amount in some cases.
Systematic error is also referred to as bias because your data is skewed in standardized ways that hide the true values. This may lead to inaccurate conclusions.
Types of systematic errors
Offset errors and scale factor errors are two quantifiable types of systematic error.
An offset error occurs when a scale isn’t calibrated to a correct zero point. It’s also called an additive error or a zero-setting error.
A scale factor error is when measurements consistently differ from the true value proportionally (e.g., by 10%). It’s also referred to as a correlational systematic error or a multiplier error.
You can plot offset errors and scale factor errors in graphs to identify their differences. In the graphs below, the black line shows when your observed value is the exact true value, and there is no random error.
The blue line is an offset error: it shifts all of your observed values upwards or downwards by a fixed amount (here, it’s one additional unit).
The purple line is a scale factor error: all of your observed values are multiplied by a factor—all values are shifted in the same direction by the same proportion, but by different absolute amounts.
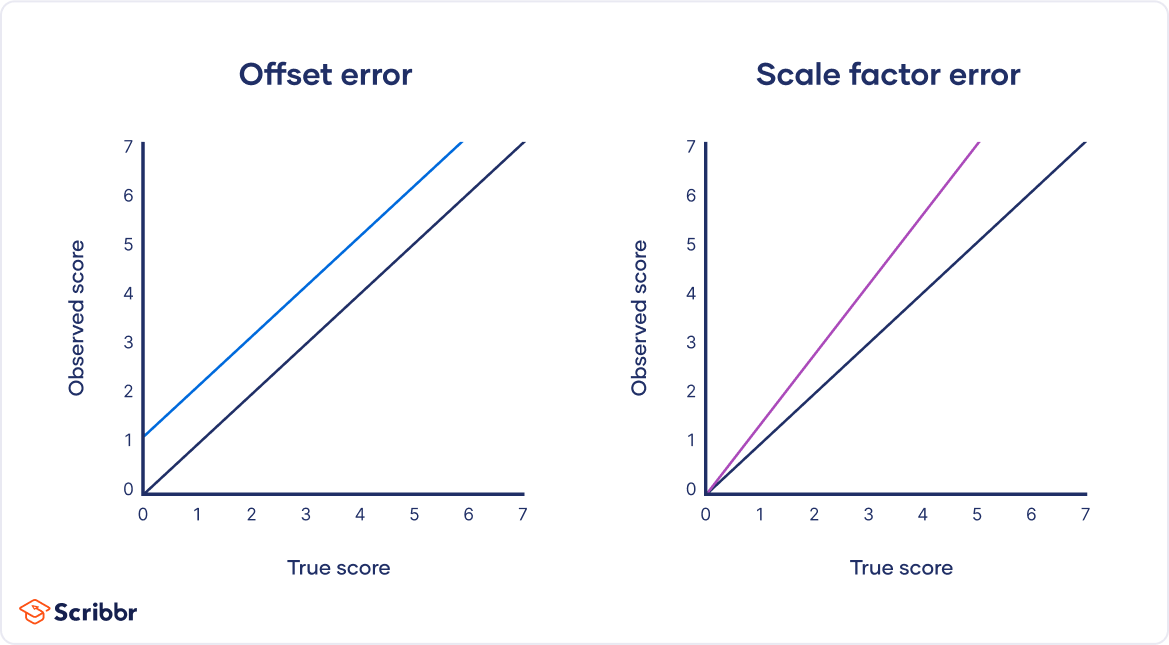
Sources of systematic errors
The sources of systematic error can range from your research materials to your data collection procedures and to your analysis techniques. This isn’t an exhaustive list of systematic error sources, because they can come from all aspects of research.
Response bias occurs when your research materials (e.g., questionnaires ) prompt participants to answer or act in inauthentic ways through leading questions . For example, social desirability bias can lead participants try to conform to societal norms, even if that’s not how they truly feel.
Your question states: “Experts believe that only systematic actions can reduce the effects of climate change. Do you agree that individual actions are pointless?”
Experimenter drift occurs when observers become fatigued, bored, or less motivated after long periods of data collection or coding, and they slowly depart from using standardized procedures in identifiable ways.
Initially, you code all subtle and obvious behaviors that fit your criteria as cooperative. But after spending days on this task, you only code extremely obviously helpful actions as cooperative.
Sampling bias occurs when some members of a population are more likely to be included in your study than others. It reduces the generalizability of your findings, because your sample isn’t representative of the whole population.
You can reduce systematic errors by implementing these methods in your study.
Triangulation
Triangulation means using multiple techniques to record observations so that you’re not relying on only one instrument or method.
For example, if you’re measuring stress levels, you can use survey responses, physiological recordings, and reaction times as indicators. You can check whether all three of these measurements converge or overlap to make sure that your results don’t depend on the exact instrument used.
Regular calibration
Calibrating an instrument means comparing what the instrument records with the true value of a known, standard quantity. Regularly calibrating your instrument with an accurate reference helps reduce the likelihood of systematic errors affecting your study.
You can also calibrate observers or researchers in terms of how they code or record data. Use standard protocols and routine checks to avoid experimenter drift.
Randomization
Probability sampling methods help ensure that your sample doesn’t systematically differ from the population.
In addition, if you’re doing an experiment, use random assignment to place participants into different treatment conditions. This helps counter bias by balancing participant characteristics across groups.
Wherever possible, you should hide the condition assignment from participants and researchers through masking (blinding) .
Participants’ behaviors or responses can be influenced by experimenter expectancies and demand characteristics in the environment, so controlling these will help you reduce systematic bias.
If you want to know more about statistics , methodology , or research bias , make sure to check out some of our other articles with explanations and examples.
- Normal distribution
- Degrees of freedom
- Null hypothesis
- Discourse analysis
- Control groups
- Mixed methods research
- Non-probability sampling
- Quantitative research
- Ecological validity
Research bias
- Rosenthal effect
- Implicit bias
- Cognitive bias
- Selection bias
- Negativity bias
- Status quo bias
Random and systematic error are two types of measurement error.
Systematic error is a consistent or proportional difference between the observed and true values of something (e.g., a miscalibrated scale consistently records weights as higher than they actually are).
Systematic error is generally a bigger problem in research.
With random error, multiple measurements will tend to cluster around the true value. When you’re collecting data from a large sample , the errors in different directions will cancel each other out.
Systematic errors are much more problematic because they can skew your data away from the true value. This can lead you to false conclusions ( Type I and II errors ) about the relationship between the variables you’re studying.
Random error is almost always present in scientific studies, even in highly controlled settings. While you can’t eradicate it completely, you can reduce random error by taking repeated measurements, using a large sample, and controlling extraneous variables .
You can avoid systematic error through careful design of your sampling , data collection , and analysis procedures. For example, use triangulation to measure your variables using multiple methods; regularly calibrate instruments or procedures; use random sampling and random assignment ; and apply masking (blinding) where possible.
Cite this Scribbr article
If you want to cite this source, you can copy and paste the citation or click the “Cite this Scribbr article” button to automatically add the citation to our free Citation Generator.
Bhandari, P. (2023, June 22). Random vs. Systematic Error | Definition & Examples. Scribbr. Retrieved August 12, 2024, from https://www.scribbr.com/methodology/random-vs-systematic-error/
Is this article helpful?
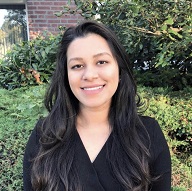
Pritha Bhandari
Other students also liked, reliability vs. validity in research | difference, types and examples, what is a controlled experiment | definitions & examples, extraneous variables | examples, types & controls, what is your plagiarism score.
Learn The Types
Learn About Different Types of Things and Unleash Your Curiosity
Understanding Experimental Errors: Types, Causes, and Solutions
Types of experimental errors.
In scientific experiments, errors can occur that affect the accuracy and reliability of the results. These errors are often classified into three main categories: systematic errors, random errors, and human errors. Here are some common types of experimental errors:
1. Systematic Errors
Systematic errors are consistent and predictable errors that occur throughout an experiment. They can arise from flaws in equipment, calibration issues, or flawed experimental design. Some examples of systematic errors include:
– Instrumental Errors: These errors occur due to inaccuracies or limitations of the measuring instruments used in the experiment. For example, a thermometer may consistently read temperatures slightly higher or lower than the actual value.
– Environmental Errors: Changes in environmental conditions, such as temperature or humidity, can introduce systematic errors. For instance, if an experiment requires precise temperature control, fluctuations in the room temperature can impact the results.
– Procedural Errors: Errors in following the experimental procedure can lead to systematic errors. This can include improper mixing of reagents, incorrect timing, or using the wrong formula or equation.
2. Random Errors
Random errors are unpredictable variations that occur during an experiment. They can arise from factors such as inherent limitations of measurement tools, natural fluctuations in data, or human variability. Random errors can occur independently in each measurement and can cause data points to scatter around the true value. Some examples of random errors include:
– Instrument Noise: Instruments may introduce random noise into the measurements, resulting in small variations in the recorded data.
– Biological Variability: In experiments involving living organisms, natural biological variability can contribute to random errors. For example, in studies involving human subjects, individual differences in response to a treatment can introduce variability.
– Reading Errors: When taking measurements, human observers can introduce random errors due to imprecise readings or misinterpretation of data.
3. Human Errors
Human errors are mistakes or inaccuracies that occur due to human factors, such as lack of attention, improper technique, or inadequate training. These errors can significantly impact the experimental results. Some examples of human errors include:
– Data Entry Errors: Mistakes made when recording data or entering data into a computer can introduce errors. These errors can occur due to typographical mistakes, transposition errors, or misinterpretation of results.
– Calculation Errors: Errors in mathematical calculations can occur during data analysis or when performing calculations required for the experiment. These errors can result from mathematical mistakes, incorrect formulas, or rounding errors.
– Experimental Bias: Personal biases or preconceived notions held by the experimenter can introduce bias into the experiment, leading to inaccurate results.
It is crucial for scientists to be aware of these types of errors and take measures to minimize their impact on experimental outcomes. This includes careful experimental design, proper calibration of instruments, multiple repetitions of measurements, and thorough documentation of procedures and observations.
You Might Also Like:
Patio perfection: choosing the best types of pavers for your outdoor space, a guide to types of pupusas: delicious treats from central america, exploring modern period music: from classical to jazz and beyond.
Types of Error — Overview & Comparison - Expii
- Faculty Resource Center
- Biochemistry
- Bioengineering
- Cancer Research
- Developmental Biology
- Engineering
- Environment
- Immunology and Infection
- Neuroscience
- JoVE Journal
- JoVE Encyclopedia of Experiments
- JoVE Chrome Extension
- Environmental Sciences
- Pharmacology
- JoVE Science Education
- JoVE Lab Manual
- JoVE Business
- Videos Mapped to your Course
- High Schools
- Videos Mapped to Your Course
Chapter 1: Chemical Applications of Statistical Analyses
Back to chapter, types of errors: detection and minimization, previous video 1.2: degrees of freedom, next video 1.5: systematic error: methodological and sampling errors.
Error is the deflection of an obtained result from the expected or true results of an experiment. This happens due to the uncertainty associated with the measurement.
Errors can be expressed in absolute or relative terms.
While absolute error is the difference between the measured and the true value, the relative error is the percentage of absolute error to the actual value.
Errors can be classified based on the source, magnitude, and sign into systematic, random, and gross errors.
Systematic errors are reproducible as they originate from defective equipment, flawed experiment design, and personal bias.
Random errors arise from uncontrollable variables in the measurement, making them irreproducible and randomly scattered around a central value.
Gross errors occur due to human mistakes and are of larger magnitude.
Systematic errors can be detected and minimized by using standard reference materials, independent analysis, blank determinations, and varying the sample size.
Error is the deviation of the obtained result from the true, expected value or the estimated central value. Errors are expressed in absolute or relative terms.
Absolute error in a measurement is the numerical difference from the true or central value. Relative error is the ratio between absolute error and the true or central value, expressed as a percentage.
Errors can be classified by source, magnitude, and sign. There are three types of errors: systematic, random, and gross.
Systematic or determinate errors emerge from known sources and are reproducible during replicate measurements. Defective equipment and experiment design flaws are familiar sources of these errors. These errors can be minimized by employing standard reference materials, independent analysis, or varying the sample size.
Random errors or indeterminate errors are difficult to reproduce by repeating measurements. These errors originate from uncontrolled variables like electronic noise in the circuit of an electrical instrument and irregular changes in the heat loss rate from a solar collector due to changes in the wind.
Gross errors are caused by human mistakes. The magnitude of these errors is often high. The origin of such errors is entirely based on the observer.

- Science Notes Posts
- Contact Science Notes
- Todd Helmenstine Biography
- Anne Helmenstine Biography
- Free Printable Periodic Tables (PDF and PNG)
- Periodic Table Wallpapers
- Interactive Periodic Table
- Periodic Table Posters
- Science Experiments for Kids
- How to Grow Crystals
- Chemistry Projects
- Fire and Flames Projects
- Holiday Science
- Chemistry Problems With Answers
- Physics Problems
- Unit Conversion Example Problems
- Chemistry Worksheets
- Biology Worksheets
- Periodic Table Worksheets
- Physical Science Worksheets
- Science Lab Worksheets
- My Amazon Books
Systematic vs Random Error – Differences and Examples

Systematic and random error are an inevitable part of measurement. Error is not an accident or mistake. It naturally results from the instruments we use, the way we use them, and factors outside our control. Take a look at what systematic and random error are, get examples, and learn how to minimize their effects on measurements.
- Systematic error has the same value or proportion for every measurement, while random error fluctuates unpredictably.
- Systematic error primarily reduces measurement accuracy, while random error reduces measurement precision.
- It’s possible to reduce systematic error, but random error cannot be eliminated.
Systematic vs Random Error
Systematic error is consistent, reproducible error that is not determined by chance. Systematic error introduces inaccuracy into measurements, even though they may be precise. Averaging repeated measurements does not reduce systematic error, but calibrating instruments helps. Systematic error always occurs and has the same value when repeating measurements the same way.
As its name suggests, random error is inconsistent error caused by chance differences that occur when taking repeated measurements. Random error reduces measurement precision, but measurements cluster around the true value. Averaging measurements containing only random error gives an accurate, imprecise value. Random errors cannot be controlled and are not the same from one measurement to the next.
Systematic Error Examples and Causes
Systematic error is consistent or proportional to the measurement, so it primarily affects accuracy. Causes of systematic error include poor instrument calibration, environmental influence, and imperfect measurement technique.
Here are examples of systematic error:
- Reading a meniscus above or below eye level always gives an inaccurate reading. The reading is consistently high or low, depending on the viewing angle.
- A scale gives a mass measurement that is always “off” by a set amount. This is called an offset error . Taring or zeroing a scale counteracts this error.
- Metal rulers consistently give different measurements when they are cold compared to when they are hot due to thermal expansion. Reducing this error means using a ruler at the temperature at which it was calibrated.
- An improperly calibrated thermometer gives accurate readings within a normal temperature range. But, readings become less accurate at higher or lower temperatures.
- An old, stretched cloth measuring tape gives consistent, but different measurements than a new tape. Proportional errors of this type are called scale factor errors .
- Drift occurs when successive measurements become consistently higher or lower as time progresses. Electronic equipment is susceptible to drift. Devices that warm up tend to experience positive drift. In some cases, the solution is to wait until an instrument warms up before using it. In other cases, it’s important to calibrate equipment to account for drift.

How to Reduce Systematic Error
Once you recognize systematic error, it’s possible to reduce it. This involves calibrating equipment, warming up instruments because taking readings, comparing values against standards, and using experimental controls. You’ll get less systematic error if you have experience with a measuring instrument and know its limitations. Randomizing sampling methods also helps, particularly when drift is a concern.
Random Error Examples and Causes
Random error causes measurements to cluster around the true value, so it primarily affects precision. Causes of random error include instrument limitations, minor variations in measuring techniques, and environmental factors.
Here are examples of random error:
- Posture changes affect height measurements.
- Reaction speed affects timing measurements.
- Slight variations in viewing angle affect volume measurements.
- Wind velocity and direction measurements naturally vary according to the time at which they are taken. Averaging several measurements gives a more accurate value.
- Readings that fall between the marks on a device must be estimated. To some extent, its possible to minimize this error by choosing an appropriate instrument. For example, volume measurements are more precise using a graduated cylinder instead of a beaker.
- Mass measurements on an analytical balance vary with air currents and tiny mass changes in the sample.
- Weight measurements on a scale vary because it’s impossible to stand on the scale exactly the same way each time. Averaging multiple measurements minimizes the error.
How to Reduce Random Error
It’s not possible to eliminate random error, but there are ways to minimize its effect. Repeat measurements or increase sample size. Be sure to average data to offset the influence of chance.
Which Types of Error Is Worse?
Systematic errors are a bigger problem than random errors. This is because random errors affect precision, but it’s possible to average multiple measurements to get an accurate value. In contrast, systematic errors affect precision. Unless the error is recognized, measurements with systematic errors may be far from true values.
- Bland, J. Martin, and Douglas G. Altman (1996). “Statistics Notes: Measurement Error.” BMJ 313.7059: 744.
- Cochran, W. G. (1968). “Errors of Measurement in Statistics”. Technometrics . Taylor & Francis, Ltd. on behalf of American Statistical Association and American Society for Quality. 10: 637–666. doi: 10.2307/1267450
- Dodge, Y. (2003). The Oxford Dictionary of Statistical Terms . OUP. ISBN 0-19-920613-9.
- Taylor, J. R. (1999). An Introduction to Error Analysis: The Study of Uncertainties in Physical Measurements . University Science Books. ISBN 0-935702-75-X.
Related Posts
- WolframAlpha.com
- WolframCloud.com
- All Sites & Public Resources...
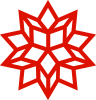
- Wolfram|One
- Mathematica
- Wolfram|Alpha Notebook Edition
- Finance Platform
- System Modeler
- Wolfram Player
- Wolfram Engine
- WolframScript
- Enterprise Private Cloud
- Application Server
- Enterprise Mathematica
- Wolfram|Alpha Appliance
- Corporate Consulting
- Technical Consulting
- Wolfram|Alpha Business Solutions
- Data Repository
- Neural Net Repository
- Function Repository
- Wolfram|Alpha Pro
- Problem Generator
- Products for Education
- Wolfram Cloud App
- Wolfram|Alpha for Mobile
- Wolfram|Alpha-Powered Apps
- Paid Project Support
- Summer Programs
- All Products & Services »
- Wolfram Language Revolutionary knowledge-based programming language. Wolfram Cloud Central infrastructure for Wolfram's cloud products & services. Wolfram Science Technology-enabling science of the computational universe. Wolfram Notebooks The preeminent environment for any technical workflows. Wolfram Engine Software engine implementing the Wolfram Language. Wolfram Natural Language Understanding System Knowledge-based broadly deployed natural language. Wolfram Data Framework Semantic framework for real-world data. Wolfram Universal Deployment System Instant deployment across cloud, desktop, mobile, and more. Wolfram Knowledgebase Curated computable knowledge powering Wolfram|Alpha.
- All Technologies »
- Aerospace & Defense
- Chemical Engineering
- Control Systems
- Electrical Engineering
- Image Processing
- Industrial Engineering
- Mechanical Engineering
- Operations Research
- Actuarial Sciences
- Bioinformatics
- Data Science
- Econometrics
- Financial Risk Management
- All Solutions for Education
- Machine Learning
- Multiparadigm Data Science
- High-Performance Computing
- Quantum Computation Framework
- Software Development
- Authoring & Publishing
- Interface Development
- Web Development
- All Solutions »
- Wolfram Language Documentation
- Fast Introduction for Programmers
- Videos & Screencasts
- Wolfram Language Introductory Book
- Webinars & Training
- Support FAQ
- Wolfram Community
- Contact Support
- All Learning & Support »
- Company Background
- Wolfram Blog
- Careers at Wolfram
- Internships
- Other Wolfram Language Jobs
- Wolfram Foundation
- Computer-Based Math
- A New Kind of Science
- Wolfram Technology for Hackathons
- Student Ambassador Program
- Wolfram for Startups
- Demonstrations Project
- Wolfram Innovator Awards
- Wolfram + Raspberry Pi
- All Company »
IMAGES
COMMENTS
Learn why all science experiments have error, how to calculate it, and the sources and types of errors you should report.
Random and systematic errors are types of measurement error, a difference between the observed and true values of something. FAQ About us . Our editors; Apply as editor; Team; Jobs; Contact ... In a controlled experiment, all variables other than the independent variable are held constant. 41. Extraneous Variables | Examples, Types & Controls ...
possible errors is an important issue in any experimental science. The conclusions we draw from the data, and especially the strength of those conclusions, will depend on
These errors are often classified into three main categories: systematic errors, random errors, and human errors. Here are some common types of experimental errors: 1. Systematic Errors. Systematic errors are consistent and predictable errors that occur throughout an experiment. They can arise from flaws in equipment, calibration issues, or ...
As a member, you'll also get unlimited access to over 88,000 lessons in math, English, science, history, and more. Plus, get practice tests, quizzes, and personalized coaching to help you succeed.
Random Errors: Random errors occur randomly, and sometimes have no source/cause. There are two types of random errors. Observational: When the observer makes consistent observational mistakes (such not reading the scale correctly and writing down values that are constantly too low or too high) Environmental: When unpredictable changes occur in the environment of the experiment (such as ...
Importance of Experimental Errors The scientific approach to understanding the world is based on a number of fundamental assumptions and techniques. Two of the most important are: • Experiments are reproducible. If you say experiment Xproduces result Y, I should be able to do experiment Xmyself and expect to get result Y.
Errors can be classified by source, magnitude, and sign. There are three types of errors: systematic, random, and gross. Systematic or determinate errors emerge from known sources and are reproducible during replicate measurements. Defective equipment and experiment design flaws are familiar sources of these errors.
or. dy − dx. - These errors are much smaller. • In general if different errors are not correlated, are independent, the way to combine them is. dz =. dx2 + dy2. • This is true for random and bias errors. THE CASE OF Z = X - Y. • Suppose Z = X - Y is a number much smaller than X or Y.
An old, stretched cloth measuring tape gives consistent, but different measurements than a new tape. Proportional errors of this type are called scale factor errors. Drift occurs when successive measurements become consistently higher or lower as time progresses. Electronic equipment is susceptible to drift.
As mentioned above, there are two types of errors associated with an experimental result: the "precision" and the "accuracy". One well-known text explains the difference this way: The word "precision" will
experimental errors. Do not list all possible sources of errors there. Your goal is to identify only those significant for that experiment! For example, if the lab table is not perfectly leveled, then for the collision experiments (M6 - Impulse and Momentum) when the track is supposed to be horizontal, results will have a large, significant ...
These include collecting, analyzing, and reporting data. In each of these aspects, errors can and do occur. In this work, we first discuss the importance of focusing on statistical and data errors to continually improve the practice of science. We then describe underlying themes of the types of errors and postulate contributing factors.
These types of errors are also known as "blunders" or "miscalculations" and they happen to everyone. In experimental inquires, these types of errors can occur due to: incorrect reading of instructions (e.g., 50 mL vs. 500 mL, sugar vs. salt, etc.); incorrect measuring (e.g., inches instead of cm, °F instead of °C, voltage instead of ...
Lab ReportTypes of Experimental Errors. Using This Checklist Use this checklist as a preliminary guideline when thinking about and analyzing potential errors in your experiment. The list is a guide but is not comprehensive, so make sure that you check with your instructor about the different types of errors to pay attention to in your lab.
Systematic errors are due to problems with the equipment you used. For example, the balances you used may have been out by 0.1 g for every measurement. For example, the balances you used may have ...
Types of Errors. There are three types of errors that are classified based on the source they arise from; They are: Gross Errors; Random Errors; Systematic Errors; Gross Errors. This category basically takes into account human oversight and other mistakes while reading, recording, and readings.
Learn about valuable skills for doing an experiment, like creating hypotheses, identifying risks, and measuring and recording data accurately.
In an analysis of electronic health records researchers found errors in assessing patients, or errors in ordering and interpreting ... Other projects underway that involve BWH ... but it does tell us that there's more we can be doing to prevent these types of errors from occurring," said Schnipper. Authors of the study include Andrew D ...