How to Do A/B Testing: 15 Steps for the Perfect Split Test
Planning to run an A/B test? Bookmark this checklist for what to do before, during, and after to get the best results.


THE COMPLETE A/B TESTING KIT
Inside: Intro to A/B Testing, Significance Calculator, and Tracking Template
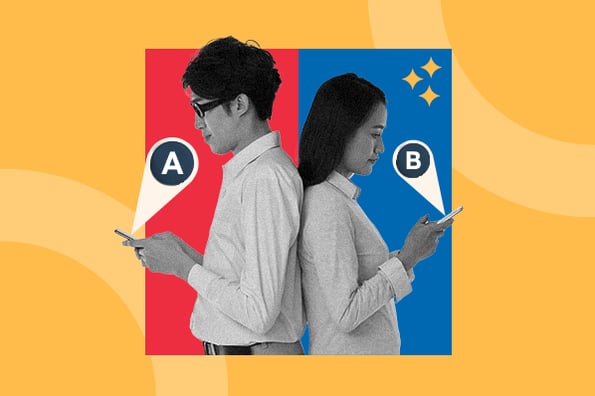
Published: 05/23/24
So, you want to discover what truly works for your audience, and you’ve heard about this mythical form of marketing testing. But you have questions like: “What is A/B testing in marketing, anyway?” and “Why does it matter?”
Don’t worry! You’ll get all the answers to your burning questions. I’ll even tell you the second answer straight away…

When marketers like us create landing pages , write email copy, or design call-to-action buttons, it can be tempting to use our intuition to predict what will make people click and connect.
But as anyone who’s been in marketing for a minute will tell you, always expect the unexpected. So, instead of basing marketing decisions on a “feeling,” you’re much better off running an A/B test to see what the data says.
Keep reading to learn how to conduct the entire A/B testing process before, during, and after data collection so you can make the best decisions based on your results.
.png)
The Complete A/B Testing Kit for Marketers
Start improving your website performance with these free templates.
- Guidelines for effective A/B testing
- Running split tests for email, landing pages, and CTAs
- Free simple significance calculator
- Free A/B test tracking template.
Download Free
All fields are required.
You're all set!
Click this link to access this resource at any time.
Table of Contents
What is A/B testing?
History of a/b testing.
- Why is A/B Testing important?
How does A/B testing work?
A/b testing in marketing, what does a/b testing involve, a/b testing goals, how to design an a/b test, how to conduct a/b testing, how to read a/b testing results, a/b testing examples.
- 10 A/B Testing Tips from Marketing Examples
What Is A/B Testing?
A/B Testing In Marketing
A/B testing, also known as split testing, is a marketing experiment wherein you split your audience to test variations on a campaign and determine which performs better. In other words, you can show version A of a piece of marketing content to one half of your audience and version B to another.
A/B testing is helpful for comparing two versions of a webpage, email newsletter, subject lines, designs, apps, and more or to see which is more successful.
Split testing takes the guesswork out of discerning how your digital marketing materials should look, operate, and be distributed. I'll walk you through everything you need to know about split testing, but I've got you covered if you're a visual learner.
The video below walks you through everything you need to know.
It’s hard to track down the “true” origins of A/B testing. However, in terms of marketing, A/B testing — albeit in its initial and imperfect form — arguably started with American advertiser and author Claude Hopkins.
Hopkins tested his ad campaigns using promotional coupons.
Still, Hopkins’ “Scientific Advertising” process didn’t include the key principles we use in A/B testing today. We have 20th-century biologist Ronald Fisher to thank for those.
Fisher, who defined statistical significance and developed the null hypothesis, helped to make A/B testing more reliable.
That said, the marketing A/B testing we know and love today started in the 1960s and ‘70s. It was also used to test direct response campaign methods. Another key marketing moment came to us in 2000.
At this time, Google engineers ran their first A/B test. (They wanted to know the best number of results to display on the search engine results page.)
Why is A/B testing important?
A/B testing has many benefits to a marketing team, depending on what you decide to test. For example, there is a limitless list of items you can test to determine the overall impact on your bottom line.
But you shouldn’t sleep on using A/B testing to find out exactly what your audience responds best to either. Let’s learn more.
You Can Find Ways To Improve Your Bottom Line
Let’s say you employ a content creator with a $50,000/year salary. This content creator publishes five articles weekly for the company blog, totaling 260 articles per year.
If the average post on the company’s blog generates 10 leads, you could say it costs just over $192 to generate 10 leads for the business ($50,000 salary ÷ 260 articles = $192 per article). That’s a solid chunk of change.
Now, if you ask this content creator to spend two days developing an A/B test on one article, instead of writing two posts in that time, you might burn $192, as you’re publishing fewer articles.
But, if that A/B test finds you can increase conversion rates from 10 to 20 leads, you just spent $192 to potentially double the number of customers your business gets from your blog.
… in a Low Cost, High Reward Way
If the test fails, of course, you lost $192 — but now you can make your next A/B test even more educated. If that second test succeeds, you ultimately spent $384 to double your company’s revenue.
No matter how many times your A/B test fails, its eventual success will almost always outweigh the cost of conducting it.
You can run many types of split tests to make the experiment worth it in the end. Above all, these tests are valuable to a business because they’re low in cost but high in reward.
You Can Find Out What Works for Your Audience
A/B testing can be valuable because different audiences behave, well, differently. Something that works for one company may not necessarily work for another.
Let’s take an unlikely B2B marketing tactic as an example. I was looking through HubSpot’s 2024 Industry Trends Report data for an article last week.
I noticed that 10% of B2B marketers planned to decrease their investment in NFTs as part of their strategy in 2024.
My first thought was, “ Huh, NFTs in B2B? ”
Then it hit me. To have that decrease, B2B marketers must’ve been using NFTs in the first place. Even more surprising than this revelation was that 34% of marketers plan to increase investment in NFTs as part of their B2B strategy.
That’s just one example of why conversion rate optimization (CRO) experts hate the term “best practices.” Because that “best practice”? Well, it may not actually be the best practice for you.
But, this kind of testing can be complex if you’re not careful. So, let’s review how A/B testing works to ensure you don’t make incorrect assumptions about what your audience likes.
To run an A/B test, you need to create two different versions of one piece of content, with changes to a single variable .
Then, you’ll show these two versions to two similarly-sized audiences and analyze which one performed better over a specific period. But remember, the testing period should be long enough to make accurate conclusions about your results.
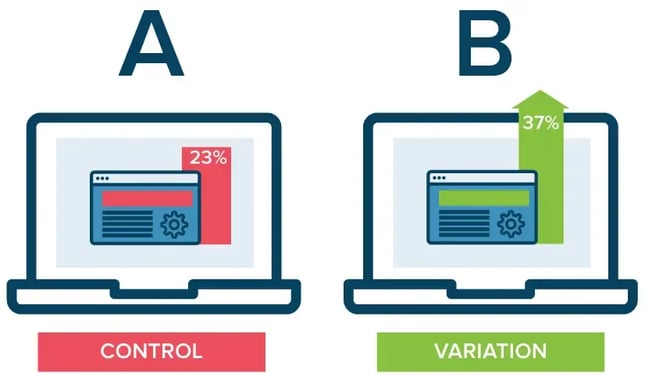
Image Source
A/B testing helps marketers observe how one version of a piece of marketing content performs alongside another. Here are two types of A/B tests you might conduct to increase your website’s conversion rate.
Example 1: User Experience Test
Perhaps you want to see if moving a certain call-to-action (CTA) button to the top of your homepage instead of keeping it in the sidebar will improve its click-through rate.
To A/B test this theory, you’d create another, alternative web page that uses the new CTA placement.
The existing design with the sidebar CTA — or the “ control ” — is version A. Version B with the CTA at the top is the “ challenger .” Then, you’d test these two versions by showing each to a predetermined percentage of site visitors.
Ideally, the percentage of visitors seeing either version is the same.
If you want more information on how to easily perform A/B testing on your website, check out HubSpot’s Marketing Hub or our introductory guide.
Example 2: Design Test
Perhaps you want to find out if changing the color of your CTA button can increase its click-through rate.
To A/B test this theory, you’d design an alternative CTA button with a different button color that leads to the same landing page as the control.
If you usually use a red CTA button in your marketing content, and the green variation receives more clicks after your A/B test, this could merit changing the default color of your CTA buttons to green from now on.
Here are some elements you might decide to test in your marketing campaigns:
- Subject lines.
- Fonts and colors.
- Product images.
- Blog graphics.
- Navigation.
- Opt-in forms.
Of course, this list is not exhaustive. Your options are countless and differ depending on the type of marketing campaign you’re A/B testing. (Blog graphics, for example, typically won’t apply to email campaigns.)
But product images can apply to both email and blog testing.)
But let’s say you wanted to test how different subject lines impacted an email marketing campaign’s conversion rates. What would you need to get started?
Here’s what you’ll need to run a successful A/B test.
- A campaign: You’ll need to pick a marketing campaign (i.e., a newsletter, landing page, or email) that’s already live. We’re going with email.
- What you want to test: You’ll need to pick the element(s) you wish to A/B test. In this case, that would be the subject line used in an email marketing campaign. But you can test all manner of things, even down to font size and CTA button color. Remember, though, if you want accurate measurements, only test one element at a time.
- Your goals: Are you testing for the sake of it? Or do you have well-defined goals? Ideally, your A/B testing should link to your revenue goals. (So, discovering which campaign has a better impact on revenue success.) To track success, you’ll need to select the right metrics. For revenue, you’d track metrics like sales, sign-ups, and clicks.
A/B testing can tell you a lot about how your intended audience behaves and interacts with your marketing campaign.
Not only does A/B testing help determine your audience’s behavior, but the results of the tests can help determine your next marketing goals.
Here are some common goals marketers have for their business when A/B testing.
Increased Website Traffic
You’ll want to use A/B testing to help you find the right wording for your website titles so you can catch your audience’s attention.
Testing different blog or web page titles can change the number of people who click on that hyperlinked title to get to your website. This can increase website traffic.
Providing it’s relevant, an increase in web traffic is a good thing! More traffic usually means more sales.
Higher Conversion Rate
Not only does A/B testing help drive traffic to your website, but it can also help boost conversion rates.
Testing different locations, colors, or even anchor text on your CTAs can change the number of people who click these CTAs to get to a landing page.
This can increase the number of people who fill out forms on your website, submit their contact info to you, and “convert” into a lead.
Lower Bounce Rate
A/B testing can help determine what’s driving traffic away from your website. Maybe the feel of your website doesn’t vibe with your audience. Or perhaps the colors clash, leaving a bad taste in your target audience’s mouth.
If your website visitors leave (or “bounce”) quickly after visiting your website, testing different blog post introductions, fonts, or featured images can retain visitors.
Perfect Product Images
You know you have the perfect product or service to offer your audience. But, how do you know you’ve picked the right product image to convey what you have to offer?
Use A/B testing to determine which product image best catches the attention of your intended audience. Compare the images against each other and pick the one with the highest sales rate.
Lower Cart Abandonment
E-commerce businesses see an average of 70% of customers leave their website with items in their shopping cart. This is known as “shopping cart abandonment” and is, of course, detrimental to any online store.
Testing different product photos, check-out page designs, and even where shipping costs are displayed can lower this abandonment rate.
Now, let’s examine a checklist for setting up, running, and measuring an A/B test.
Designing an A/B test can seem like a complicated task at first. But, trust us — it’s simple.
The key to designing a successful A/B test is to determine which elements of your blog, website, or ad campaign can be compared and contrasted against a new or different version.
Before you jump into testing all the elements of your marketing campaign, check out these A/B testing best practices.
Test appropriate items.
List elements that could influence how your target audience interacts with your ads or website. Specifically, consider which elements of your website or ad campaign influence a sale or conversion.
Be sure the elements you choose are appropriate and can be modified for testing purposes.
For example, you might test which fonts or images best grab your audience’s attention in a Facebook ad campaign. Or, you might pilot two pages to determine which keeps visitors on your website longer.
Pro tip: Choose appropriate test items by listing elements that affect your overall sales or lead conversion, and then prioritize them.
Determine the correct sample size.
The sample size of your A/B test can have a large impact on the results — and sometimes, that is not a good thing. A sample size that is too small will skew the results.
Make sure your sample size is large enough to yield accurate results. Use tools like a sample size calculator to help you figure out the correct number of interactions or visitors to your website or participants in your campaign you need to obtain the best result.
Check your data.
A sound split test will yield statistically significant and reliable results. In other words, your A/B test results are not influenced by randomness or chance. But how can you be sure your results are statistically significant and reliable?
Just like determining sample size, tools are available to help verify your data.
Tools, such as Convertize’s AB Test Significance Calculator , allow users to plug in traffic data and conversion rates of variables and select the desired level of confidence.
The higher the statistical significance achieved, the less you can expect the data to occur by chance.
Pro tip: Ensure your data is statistically significant and reliable by using tools like A/B test significance calculators.

Schedule your tests.
When comparing variables, keeping the rest of your controls the same is important — including when you schedule to run your tests.
If you’re in the ecommerce space, you’ll need to take holiday sales into consideration.
For example, if you run an A/B test on the control during a peak sales time, the traffic to your website and your sales may be higher than the variable you tested in an “off week.”
To ensure the accuracy of your split tests, pick a comparable timeframe for both tested elements. Run your campaigns for the same length of time to get the best, most accurate results.
Pro tip: Choose a timeframe when you can expect similar traffic to both portions of your split test.
Test only one element.
Each variable of your website or ad campaign can significantly impact your intended audience’s behavior. That’s why looking at just one element at a time is important when conducting A/B tests.
Attempting to test multiple elements in the same A/B test will yield unreliable results. With unreliable results, you won’t know which element had the biggest impact on consumer behavior.
Be sure to design your split test for just one element of your ad campaign or website.
Pro tip: Don’t try to test multiple elements at once. A good A/B test will be designed to test only one element at a time.
Analyze the data.
As a marketer, you might have an idea of how your target audience behaves with your campaign and web pages. A/B testing can give you a better indication of how consumers really interact with your sites.
After testing is complete, take some time to thoroughly analyze the data. You might be surprised to find that what you thought was working for your campaigns was less effective than you initially thought.
Pro tip: Accurate and reliable data may tell a different story than you first imagined. Use the data to help plan or change your campaigns.
To get a comprehensive view of your marketing performance, use our robust analytics tool, HubSpot's Marketing Analytics software .
Follow along with our free A/B testing kit , which includes everything you need to run A/B testing, including a test tracking template, a how-to guide for instruction and inspiration, and a statistical significance calculator to determine whether your tests were wins, losses, or inconclusive.
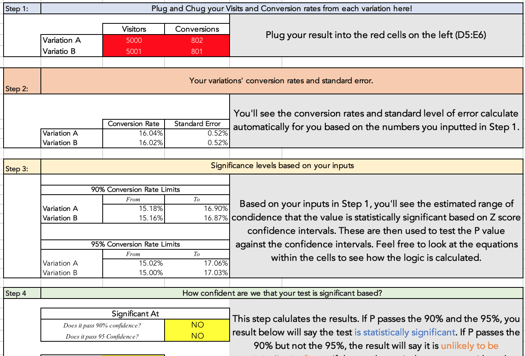
Before the A/B Test
Let’s cover the steps to take before you start your A/B test.
1. Pick one variable to test.
As you optimize your web pages and emails, you’ll find there are many variables you want to test. But to evaluate effectiveness, you’ll want to isolate one independent variable and measure its performance.
Otherwise, you can’t be sure which variable was responsible for changes in performance.
You can test more than one variable for a single web page or email — just be sure you’re testing them one at a time.
To determine your variable, look at the elements in your marketing resources and their possible alternatives for design, wording, and layout. You may also test email subject lines, sender names, and different ways to personalize your emails.
Pro tip: You can use HubSpot’s AI Email Writer to write email copy for different audiences. The software is built into HubSpot’s marketing and sales tools.
Keep in mind that even simple changes, like changing the image in your email or the words on your CTA button , can drive big improvements. In fact, these sorts of changes are usually easier to measure than the bigger ones.
Note: Sometimes, testing multiple variables rather than a single variable makes more sense. This is called multivariate testing.
If you’re wondering whether you should run an A/B test versus a multivariate test, here’s a helpful article from Optimizely that compares the processes.
2. Identify your goal.
Although you’ll measure several metrics during any one test, choose a primary metric to focus on before you run the test. In fact, do it before you even set up the second variation.
This is your dependent variable , which changes based on how you manipulate the independent variable.
Think about where you want this dependent variable to be at the end of the split test. You might even state an official hypothesis and examine your results based on this prediction.
If you wait until afterward to think about which metrics are important to you, what your goals are, and how the changes you’re proposing might affect user behavior, then you may not set up the test in the most effective way.
3. Create a 'control' and a 'challenger.'
You now have your independent variable, your dependent variable, and your desired outcome. Use this information to set up the unaltered version of whatever you’re testing as your control scenario.
If you’re testing a web page, this is the unaltered page as it exists already. If you’re testing a landing page, this would be the landing page design and copy you would normally use.
From there, build a challenger — the altered website, landing page, or email that you’ll test against your control.
For example, if you’re wondering whether adding a testimonial to a landing page would make a difference in conversions, set up your control page with no testimonials. Then, create your challenger with a testimonial.
4. Split your sample groups equally and randomly.
For tests where you have more control over the audience — like with emails — you need to test with two or more equal audiences to have conclusive results.
How you do this will vary depending on the A/B testing tool you use. Suppose you’re a HubSpot Enterprise customer conducting an A/B test on an email , for example.
HubSpot will automatically split traffic to your variations so that each variation gets a random sampling of visitors.
5. Determine your sample size (if applicable).
How you determine your sample size will also vary depending on your A/B testing tool, as well as the type of A/B test you’re running.
If you’re A/B testing an email, you’ll probably want to send an A/B test to a subset of your list large enough to achieve statistically significant results.
Eventually, you’ll pick a winner to send to the rest of the list. (See “The Science of Split Testing” ebook at the end of this article for more.)
If you’re a HubSpot Enterprise customer, you’ll have some help determining the size of your sample group using a slider.
It’ll let you do a 50/50 A/B test of any sample size — although all other sample splits require a list of at least 1,000 recipients.
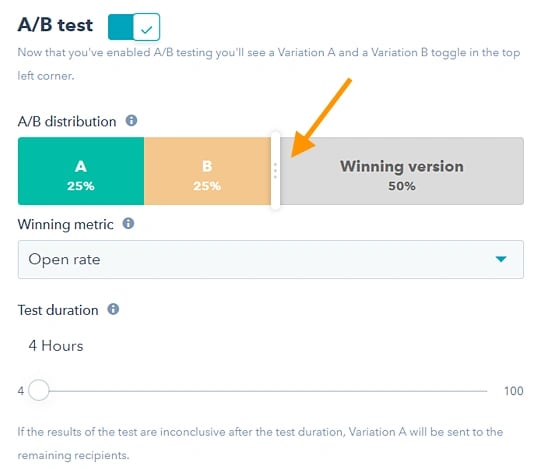
If you’re testing something that doesn’t have a finite audience, like a web page, then how long you keep your test running will directly affect your sample size.
You’ll need to let your test run long enough to obtain a substantial number of views. Otherwise, it will be hard to tell whether there was a statistically significant difference between variations.
6. Decide how significant your results need to be.
Once you’ve picked your goal metric, think about how significant your results need to be to justify choosing one variation over another.
Statistical significance is a super important part of the A/B testing process that’s often misunderstood. If you need a refresher, I recommend reading this blog post on statistical significance from a marketing standpoint.
The higher the percentage of your confidence level, the more sure you can be about your results. In most cases, you’ll want a confidence level of 95% minimum, especially if the experiment was time-intensive.
However, sometimes, it makes sense to use a lower confidence rate if the test doesn’t need to be as stringent.
Matt Rheault , a senior software engineer at HubSpot, thinks of statistical significance like placing a bet.
What odds are you comfortable placing a bet on? Saying, “I’m 80% sure this is the right design, and I’m willing to bet everything on it,” is similar to running an A/B test to 80% significance and then declaring a winner.
Rheault also says you’ll likely want a higher confidence threshold when testing for something that only slightly improves the conversion rate. Why? Because random variance is more likely to play a bigger role.
“An example where we could feel safer lowering our confidence threshold is an experiment that will likely improve conversion rate by 10% or more, such as a redesigned hero section,” he explained.
“The takeaway here is that the more radical the change, the less scientific we need to be process-wise. The more specific the change (button color, microcopy, etc.), the more scientific we should be because the change is less likely to have a large and noticeable impact on conversion rate,” Rheault says.
7. Make sure you're only running one test at a time on any campaign.
Testing more than one thing for a single campaign can complicate results.
For example, if you A/B test an email campaign that directs to a landing page while you’re A/B testing that landing page, how can you know which change increased leads?
During the A/B Test
Let's cover the steps to take during your A/B test.
8. Use an A/B testing tool.
To do an A/B test on your website or in an email, you’ll need to use an A/B testing tool.
If you’re a HubSpot Enterprise customer, the HubSpot software has features that let you A/B test emails ( learn how here ), CTAs ( learn how here ), and landing pages ( learn how here ).
For non-HubSpot Enterprise customers, other options include Google Analytics , which lets you A/B test up to 10 full versions of a single web page and compare their performance using a random sample of users.
9. Test both variations simultaneously.
Timing plays a significant role in your marketing campaign’s results, whether it’s the time of day, day of the week, or month of the year.
If you were to run version A for one month and version B a month later, how would you know whether the performance change was caused by the different design or the different month?
When running A/B tests, you must run the two variations simultaneously. Otherwise, you may be left second-guessing your results.
The only exception is if you’re testing timing, like finding the optimal times for sending emails.
Depending on what your business offers and who your subscribers are, the optimal time for subscriber engagement can vary significantly by industry and target market.
10. Give the A/B test enough time to produce useful data.
Again, you’ll want to make sure that you let your test run long enough to obtain a substantial sample size. Otherwise, it’ll be hard to tell whether the two variations had a statistically significant difference.
How long is long enough? Depending on your company and how you execute the A/B test, getting statistically significant results could happen in hours... or days... or weeks.
A big part of how long it takes to get statistically significant results is how much traffic you get — so if your business doesn’t get a lot of traffic to your website, it’ll take much longer to run an A/B test.
Read this blog post to learn more about sample size and timing .
11. Ask for feedback from real users.
A/B testing has a lot to do with quantitative data... but that won’t necessarily help you understand why people take certain actions over others. While you’re running your A/B test, why not collect qualitative feedback from real users?
A survey or poll is one of the best ways to ask people for their opinions.
You might add an exit survey on your site that asks visitors why they didn’t click on a certain CTA or one on your thank-you pages that asks visitors why they clicked a button or filled out a form.
For example, you might find that many people clicked on a CTA leading them to an ebook, but once they saw the price, they didn’t convert.
That kind of information will give you a lot of insight into why your users behave in certain ways.
After the A/B Test
Finally, let's cover the steps to take after your A/B test.
12. Focus on your goal metric.
Again, although you’ll be measuring multiple metrics, focus on that primary goal metric when you do your analysis.
For example, if you tested two variations of an email and chose leads as your primary metric, don’t get caught up on click-through rates.
You might see a high click-through rate and poor conversions, in which case you might choose the variation that had a lower click-through rate in the end.
13. Measure the significance of your results using our A/B testing calculator.
Now that you’ve determined which variation performs the best, it’s time to determine whether your results are statistically significant. In other words, are they enough to justify a change?
To find out, you’ll need to conduct a test of statistical significance. You could do that manually, or you could just plug in the results from your experiment to our free A/B testing calculator . (The calculator comes as part of our free A/B testing kit.)
You’ll be prompted to input your result into the red cells for each variation you tested. The template results are for either “Visitors” or “Conversions.” However, you can customize these headings for other types of results.
You’ll then see a series of automated calculations based on your inputs. From there, the calculator will determine statistical significance.
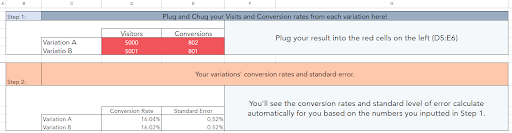
14. Take action based on your results.
If one variation is statistically better than the other, you have a winner. Complete your test by disabling the losing variation in your A/B testing tool.
If neither variation is significant, the variable you tested didn’t impact results, and you’ll have to mark the test as inconclusive. In this case, stick with the original variation or run another test.
You can use failed data to help you figure out a new iteration on your new test.
While A/B tests help you impact results on a case-by-case basis, you can also apply the lessons you learn from each test to future efforts.
For example, suppose you’ve conducted A/B tests in your email marketing and have repeatedly found that using numbers in email subject lines generates better clickthrough rates. In that case, consider using that tactic in more of your emails.
15. Plan your next A/B test.
The A/B test you just finished may have helped you discover a new way to make your marketing content more effective — but don’t stop there. There’s always room for more optimization.
You can even try conducting an A/B test on another feature of the same web page or email you just did a test on.
For example, if you just tested a headline on a landing page, why not do a new test on the body copy? Or a color scheme? Or images? Always keep an eye out for opportunities to increase conversion rates and leads.
You can use HubSpot’s A/B Test Tracking Kit to plan and organize your experiments.
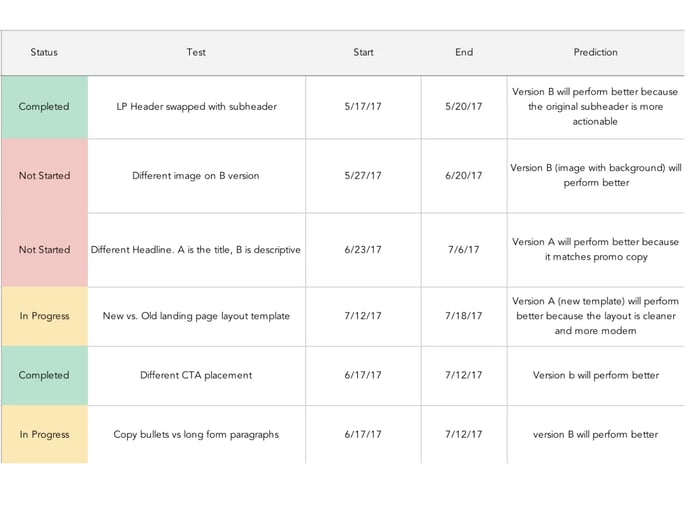
Download This Template Now
As a marketer, you know the value of automation. Given this, you likely use software that handles the A/B test calculations for you — a huge help. But, after the calculations are done, you need to know how to read your results. Let’s go over how.
1. Check your goal metric.
The first step in reading your A/B test results is looking at your goal metric, which is usually conversion rate.
After you’ve plugged your results into your A/B testing calculator, you’ll get two results for each version you’re testing. You’ll also get a significant result for each of your variations.
2. Compare your conversion rates.
By looking at your results, you’ll likely be able to tell if one of your variations performed better than the other. However, the true test of success is whether your results are statistically significant.
For example, variation A had a 16.04% conversion rate. Variation B had a 16.02% conversion rate, and your confidence interval of statistical significance is 95%.
Variation A has a higher conversion rate, but the results are not statistically significant, meaning that variation A won’t significantly improve your overall conversion rate.
3. Segment your audiences for further insights.
Regardless of significance, it’s valuable to break down your results by audience segment to understand how each key area responded to your variations. Common variables for segmenting audiences are:
- Visitor type, or which version performed best for new visitors versus repeat visitors.
- Device type, or which version performed best on mobile versus desktop.
- Traffic source, or which version performed best based on where traffic to your two variations originated.
Let’s go over some examples of A/B experiments you could run for your business.
We’ve discussed how A/B tests are used in marketing and how to conduct one — but how do they actually look in practice?
As you might guess, we run many A/B tests to increase engagement and drive conversions across our platform. Here are five examples of A/B tests to inspire your own experiments.
1. Site Search
Site search bars help users quickly find what they’re after on a particular website. HubSpot found from previous analysis that visitors who interacted with its site search bar were more likely to convert on a blog post.
So, we ran an A/B test to increase engagement with the search bar.
In this test, search bar functionality was the independent variable, and views on the content offer thank you page was the dependent variable. We used one control condition and three challenger conditions in the experiment.
The search bar remained unchanged in the control condition (variant A).
In variant B, the search bar was larger and more visually prominent, and the placeholder text was set to “search by topic.”
Variant C appeared identical to variant B but only searched the HubSpot Blog rather than the entire website.
In variant D, the search bar was larger, but the placeholder text was set to “search the blog.” This variant also searched only the HubSpot Blog.
We found variant D to be the most effective: It increased conversions by 3.4% over the control and increased the percentage of users who used the search bar by 6.5%.
2. Mobile CTAs
HubSpot uses several CTAs for content offers in our blog posts, including ones in the body of the post as well as at the bottom of the page. We test these CTAs extensively to optimize their performance.
We ran an A/B test for our mobile users to see which type of bottom-of-page CTA converted best.
We altered the design of the CTA bar for our independent variable. Specifically, we used one control and three challengers in our test. For our dependent variables, we used pageviews on the CTA thank you page and CTA clicks.
The control condition included our normal placement of CTAs at the bottom of posts. In variant B, the CTA had no close or minimize option.
In variant C, mobile readers could close the CTA by tapping an X icon. Once it was closed out, it wouldn’t reappear.
In variant D, we included an option to minimize the CTA with an up/down caret.
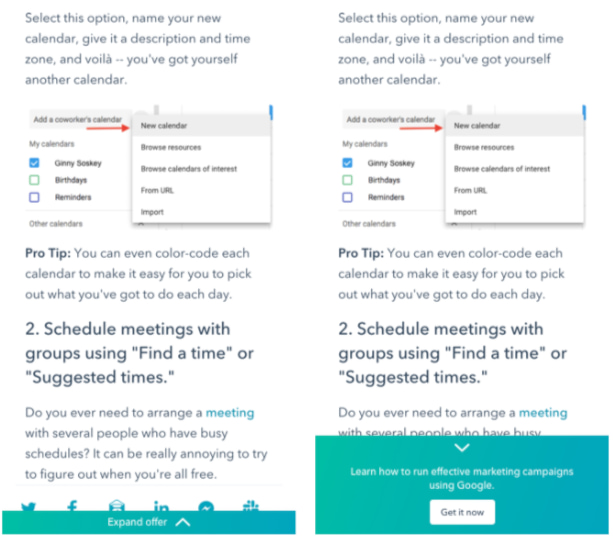
Our tests found all variants to be successful. Variant D was the most successful, with a 14.6% increase in conversions over the control. This was followed by variant C with an 11.4% increase and variant B with a 7.9% increase.
3. Author CTAs
In another CTA experiment, HubSpot tested whether adding the word “free” and other descriptive language to author CTAs at the top of blog posts would increase content leads.
Past research suggested that using “free” in CTA text would drive more conversions and that text specifying the type of content offered would help SEO .
In the test, the independent variable was CTA text, and the main dependent variable was conversion rate on content offer forms.
In the control condition, the author CTA text was unchanged (see the orange button in the image below).
In variant B, the word “free” was added to the CTA text.
In variant C, descriptive wording was added to the CTA text in addition to “free.”
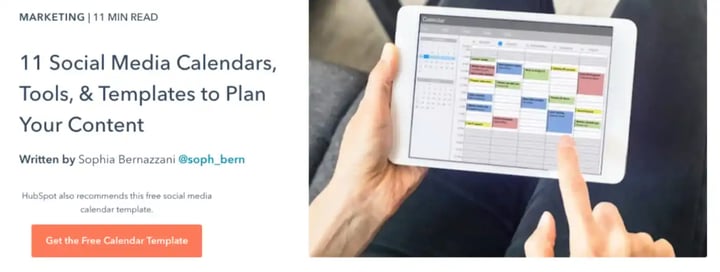
Interestingly, variant B saw a loss in form submissions, down by 14% compared to the control. This was unexpected, as including “free” in content offer text is widely considered a best practice.
Meanwhile, form submissions in variant C outperformed the control by 4%. It was concluded that adding descriptive text to the author CTA helped users understand the offer and thus made them more likely to download.
4. Blog Table of Contents
To help users better navigate the blog, HubSpot tested a new Table of Contents (TOC) module. The goal was to improve user experience by presenting readers with their desired content more quickly.
We also tested whether adding a CTA to this TOC module would increase conversions.
The independent variable of this A/B test was the inclusion and type of TOC module in blog posts. The dependent variables were conversion rate on content offer form submissions and clicks on the CTA inside the TOC module.
The control condition did not include the new TOC module — control posts either had no table of contents or a simple bulleted list of anchor links within the body of the post near the top of the article (pictured below).
In variant B, the new TOC module was added to blog posts. This module was sticky, meaning it remained onscreen as users scrolled down the page. Variant B also included a content offer CTA at the bottom of the module.
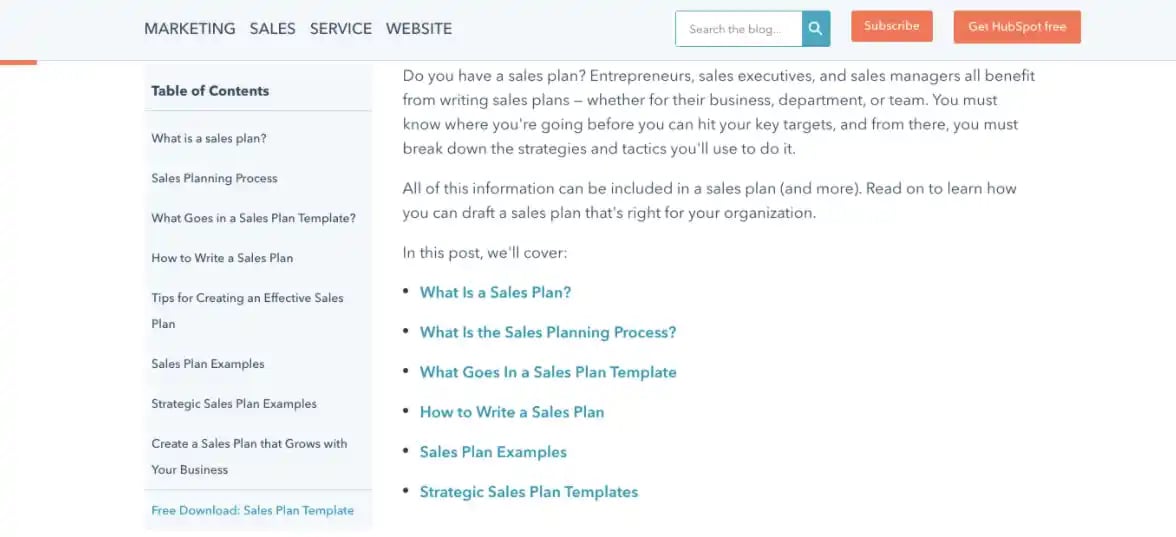
Variant C included an identical module to variant B but with the CTA removed.
Both variants B and C did not increase the conversion rate on blog posts. The control condition outperformed variant B by 7% and performed equally with variant C.
Also, few users interacted with the new TOC module or the CTA inside the module.
5. Review Notifications
To determine the best way of gathering customer reviews, we ran a split test of email notifications versus in-app notifications.
Here, the independent variable was the type of notification, and the dependent variable was the percentage of those who left a review out of all those who opened the notification.
In the control, HubSpot sent a plain text email notification asking users to leave a review. In variant B, HubSpot sent an email with a certificate image including the user’s name.
For variant C, HubSpot sent users an in-app notification.
Ultimately, both emails performed similarly and outperformed the in-app notifications. About 25% of users who opened an email left a review versus the 10.3% who opened in-app notifications.
Users also opened emails more often.
10 A/B Testing Tips From Marketing Experts
I spoke to nine marketing experts from across disciplines to get their tips on A/B testing.
1. Clearly define your goals and metrics first.
“In my experience, the number one tip for A/B testing in marketing is to clearly define your goals and metrics before conducting any tests,” says Noel Griffith, CMO at SupplyGem .
Griffith explains that this means having a solid understanding of what you want to achieve with your test and how you will measure its success. This matters because, without clear goals, it’s easy to get lost in the data and draw incorrect conclusions.
For example, Griffith says, if you’re testing two different email subject lines, your goal could be to increase open rates.
“By clearly defining this goal and setting a specific metric to measure success (e.g., a 10% increase in open rates), you can effectively evaluate the performance of each variant and make data-driven decisions,” says Griffith.
Aside from helping you focus your testing efforts, Noel explains that having clear goals also means you can accurately interpret the results and apply them to improve your marketing strategies.
2. Test only ONE thing during each A/B test.
“This is the most important tip for A/B marketing from my perspective... Always decide on one thing to test for each individual A/B test,” says Hanna Feltges , growth marketing manager at Niceboard .
For example, when A/B testing button placement in emails, Feltges makes sure the only difference between these two emails is the button placement.
No difference should be in the subject line, copy, or images, as this could skew the results and make the test invalid.
Feltges applies the same principle to metrics by choosing one metric to evaluate test results
“For emails, I will select a winner based on a predefined metric, such as CTR, open rate, reply rate, etc. In my example of the button placement, I would select CTR as my deciding metric and evaluate the results based on this metric,” Feltges says.
3. Start with a hypothesis to prove or disprove.
Another similarly important tip for A/B testing is to start with a hypothesis. The goal of each A/B test is then to prove the hypothesis right or wrong, Feltges notes.
For example, Feltges poses testing two different subject lines for a cold outreach email. Her hypothesis here is:
“Having a subject line with the prospect’s first name will lead to higher open rates than a subject line without the prospect’s first name,” she says.
Now, she can run multiple tests with the same hypothesis and can then evaluate if the statement is true or not.
Feltges explains that the idea here is that marketers often draw quick conclusions from A/B tests, such as “Having the first name in the subject line performs better.” But that is not 100% true.
A/B tests are all about being precise and specific in the results.
4. Track key test details for accurate planning and analysis.
“I keep a running log of how long my A/B tests for SEO took, and I make sure to track critical metrics like the statistical significance rate that was reached,” says NamePepper Founder Dave VerMeer.
VerMeer explains that the log is organized in a spreadsheet that includes other columns for things like:
- The type of test.
- Details about what was tested.
“If I notice any factors that could have influenced the test, I note those as well,” he adds. Other factors could be a competitor having a special event or something that happened in the news and caused a traffic spike.
“I check the log whenever I’m planning a series of A/B tests. For example, it lets me see trends and forecast how the seasonality may affect the test period lengths. Then I adjust the test schedule accordingly,” VerMeer says.
According to VerMeer, this form of tracking is also helpful for setting realistic expectations and providing clues as to why a test result did or didn’t match up with past performance.
5. Test often…
When I spoke to Gabriel Gan, head of editorial for In Real Life Malaysia , for my guide on running an email marketing audit , he set out two main rules for A/B testing.
For the A/B testing email, Gan recommends setting email A as the incumbent and email B as the contender.
Like Hanna, Gabriel emphasizes changing only one variable at a time. “For example, in email B, when testing open rates, only tweak the subject line and not the preview,” says Gan.
That’s because if you have more than one variable changed from the old email, “it’s almost impossible to determine which new addition you made has contributed to the improvement in OPR/CTR.”
Aside from only changing one variable at a time, Gan recommends testing often until you find out what works and what doesn’t.
“There’s a perception that once you set up your email list and create a template for your emails, you can ‘set it and forget it.’” Gan says. “But now, with the power of A/B testing, with just a few rounds of testing your headlines, visuals, copy, offer, call-to-action, etc., you can find out what your audience loves, do more of it, and improve your conversion rates twofold or threefold.”
6. …But don’t feel like you need to test everything.
“My top tip for A/B testing is only to use it strategically,” says Joe Kevens , director of demand generation at PartnerStack and the founder of B2B SaaS Reviews .
Kevens explains that “strategically” means that only some things warrant an A/B test due to the time and resources it consumes.
“I’ve learned from experience that testing minor elements like CTA button colors can be a waste of time and effort (unless you work at Amazon or some mega-corporation that gets a gazillion page visits, and a minor change can make a meaningful impact),” Kevens says.
Kevens recommends that instead, it’s more beneficial to concentrate on high-impact areas such as homepage layouts, demo or trial pages, and high-profile marketing messages.
That’s because these elements have a better shot to impact conversion rates and overall user experience.
Kevens reminds us that “A/B testing can be powerful, but its effectiveness comes from focusing on changes that can significantly impact your business outcomes.”
7. Use segmentation to micro-identify winning elements.
“When using A/B testing in marketing, don’t limit your target audience to just one set of parameters,” says Brian David Crane , founder and CMO of Spread Great Ideas .
Crane recommends using criteria like demographics, user behavior, past interactions, and buying history to experiment with A/B testing of these different segments. You can then filter the winning strategy for each segment.
“We use core metrics like click-through rates, bounce rates, and customer lifetime value to identify the combination that converts the most,” explains Crane.
.png?width=370&height=370&name=Copy%20of%20Linkedin%20-%201104x736%20-%20Quote%20%2B%20Headshot%20-%20Orange%20(2).png)
8. Leverage micro-conversions for granular insights.
“I know that it’s common to focus on macro-conversions, such as sales or sign-ups, in A/B testing. However, my top tip is to also pay attention to micro-conversions,” says Laia Quintana , head of marketing and sales at TeamUp .
Quintana explains that micro-conversions are smaller actions users take before completing a macro-conversion.
They could be actions like clicking on a product image, spending a certain amount of time on a page, or watching a promotional video.
But why are these micro-conversions important? Quintana states, “They provide granular insights into user behavior and can help identify potential roadblocks in the conversion path.”
For example, if users spend a lot of time on a product page but do not add items to their cart, there might be an issue with the page layout or information clarity.
By A/B testing different elements on the page, you can identify and rectify these issues to improve the overall conversion rate.
“Moreover, tracking micro-conversions allows you to segment your audience more effectively. You can identify which actions are most indicative of a user eventually making a purchase and then tailor your marketing efforts to encourage those actions. This level of detail in your A/B testing can significantly enhance the effectiveness of your marketing strategy,” says Quintana.
9. Running LinkedIn Ads? Start with five different versions and A/B test them.
“A best practice when running LinkedIn Ads is to start a campaign with five different versions of your ad,” says Hristina Stefanova , head of marketing operations at Goose’n’Moose .
Stefanova reminds us that it’s important to tweak just one variable at a time across each version.
For a recent campaign, Stefanova started with five ad variations — four using different hero images and three having the CTA tweaked.
“I let the campaign run with all five variations for a week. At that point, there were two clearly great performing ads, so I paused the other three and continued running the campaign with the two best-performing ones,” says Stefanova.
According to Stefanova, the two ads performed best and had the lowest CPC. The A/B testing exercise helped not only the specific campaign but also helped her to better understand what attracts their target audience.
So what’s next? “Images with people in them are better received, so for upcoming campaigns, I am focusing right away on producing the right imagery. All backed up by real performance data thanks to A/B testing,” Stefanova says.
10. Running SEO A/B tests? Do this with your test and control group URLs.
“Given that the SEO space is constantly evolving, it’s getting increasingly difficult to run any sort of experiments and get reliable and statistically significant results. This is especially true when running SEO A/B tests,” says Ryan Jones , marketing manager at SEOTesting .
Luckily, Jones explains that you can do things to mitigate this and make sure that any SEO A/B tests you run now — and in the future — are reliable. You can then use the tests as a “North Star” when making larger-scale changes to your site.
“My number one tip would be to ensure that your control group and test group of URLs contain as identical URLs as you can make them. For example, if you’re running an A/B test on your PLP pages as an ecommerce site, choose PLPs from the same product type and with the same traffic levels. This way, you can ensure that your test data will be reliable,” says Jones.
Why does this matter? “Perhaps the number one thing that ‘messes’ with A/B test data is control and variant groups that are too dissimilar.
But by ensuring you are testing against statistically similar URLs, you can mitigate this better than anything else,” Jones says.
Start A/B Testing Today
A/B testing allows you to get to the truth of what content and marketing your audience wants to see. With HubSpot’s Campaign Assistant , you’ll be able to generate copy for landing pages, emails, or ads that can be used for A/B testing.
Learn how to best carry out some of the steps above using the free ebook below.
Editor's note: This post was originally published in May 2016 and has been updated for comprehensiveness.
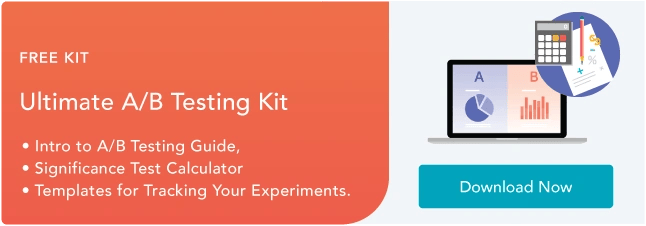
Don't forget to share this post!
Related articles.
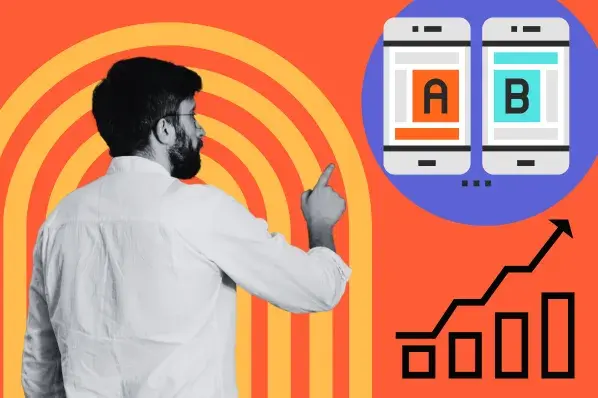
How to Determine Your A/B Testing Sample Size & Time Frame
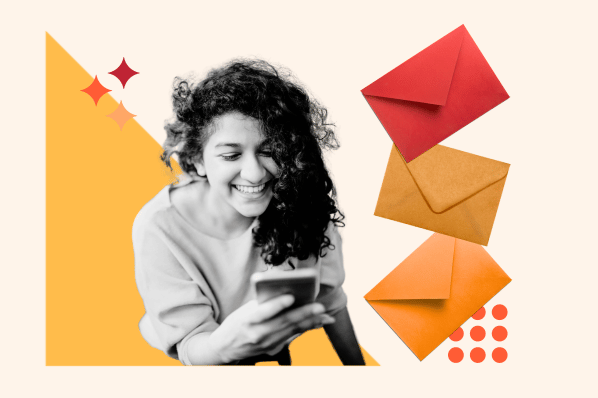
How The Hustle Got 43,876 More Clicks
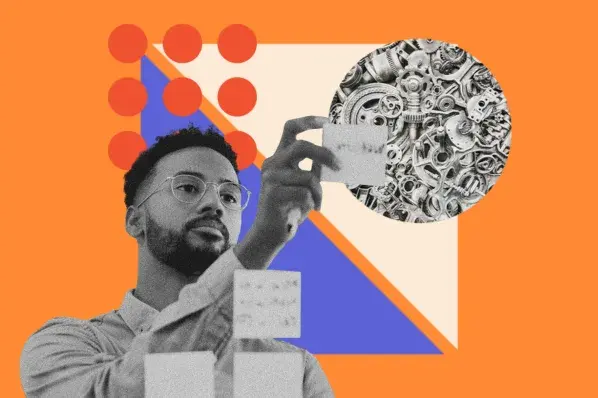
What Most Brands Miss With User Testing (That Costs Them Conversions)
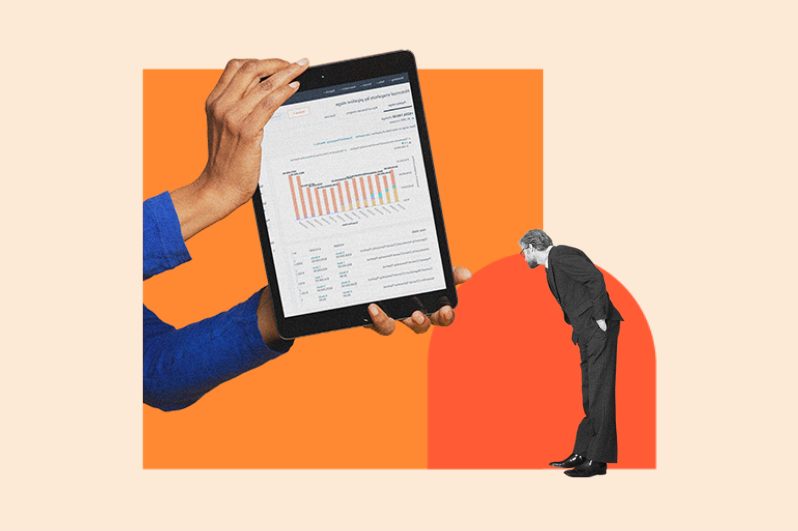
Multivariate Testing: How It Differs From A/B Testing
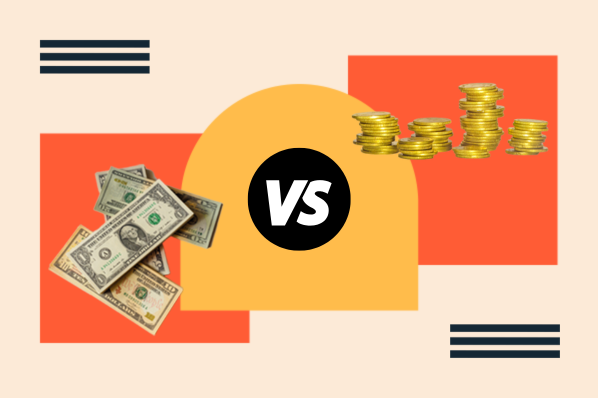
How to A/B Test Your Pricing (And Why It Might Be a Bad Idea)
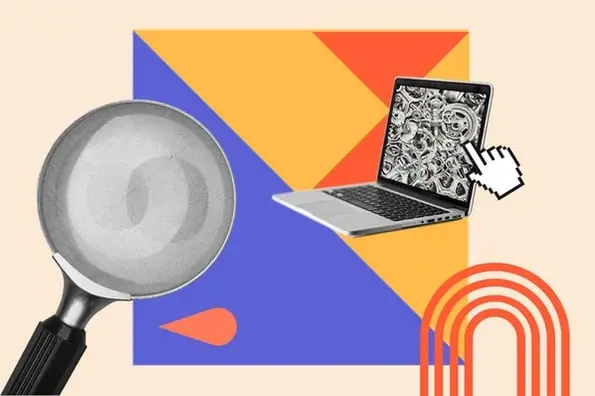
11 A/B Testing Examples From Real Businesses
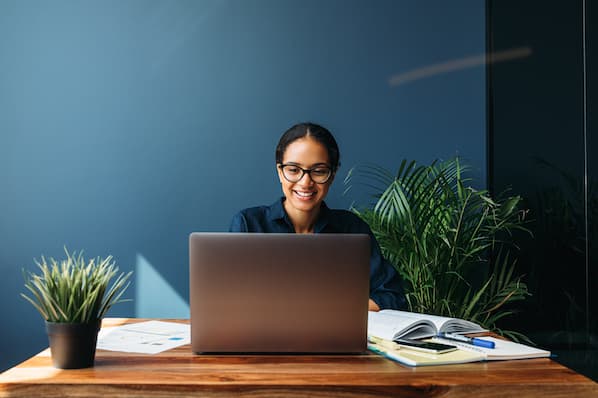
15 of the Best A/B Testing Tools for 2024
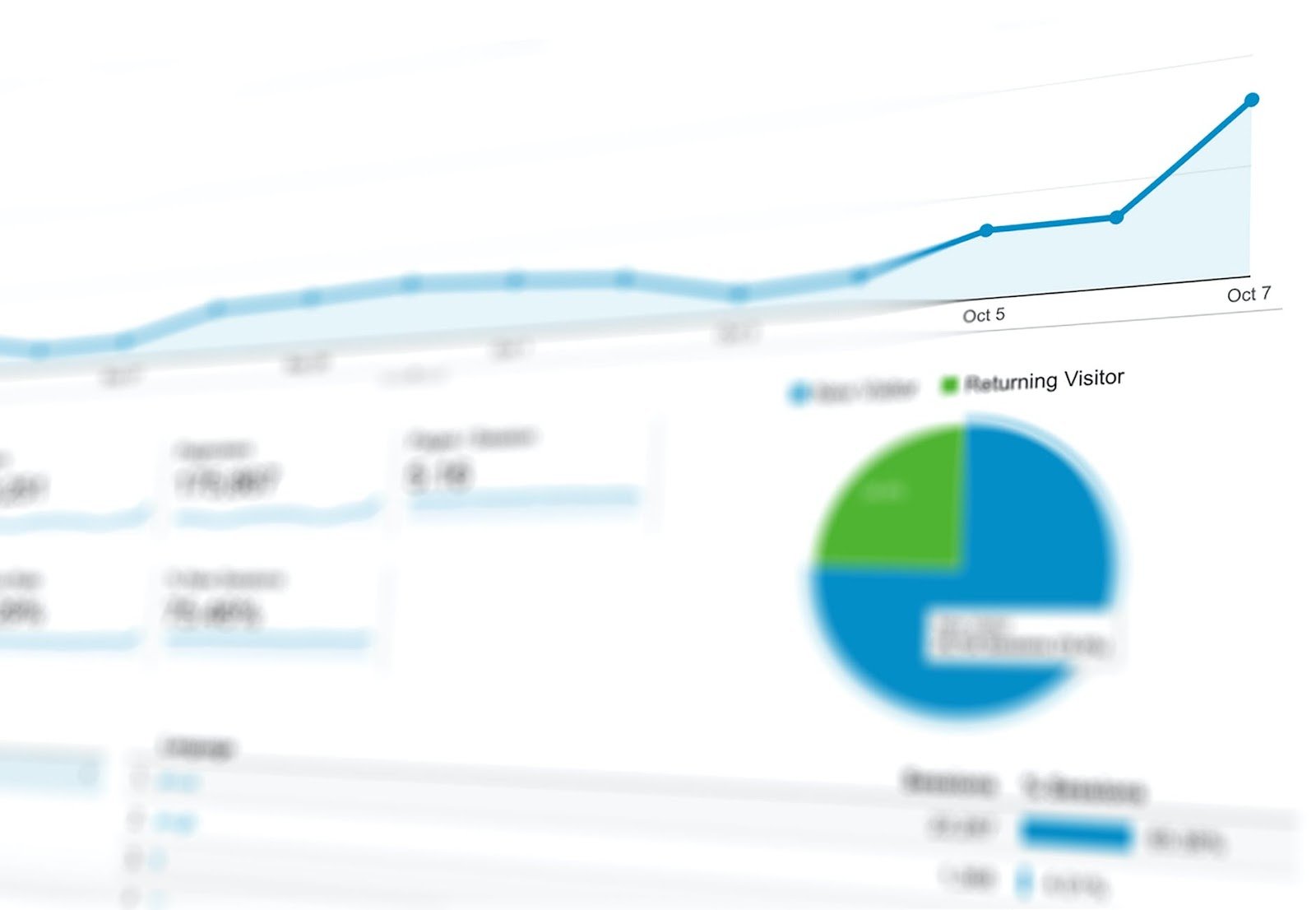
These 20 A/B Testing Variables Measure Successful Marketing Campaigns
![ab test experiment design How to Understand & Calculate Statistical Significance [Example]](https://www.hubspot.com/hubfs/FEATURED%20IMAGE-Nov-22-2022-06-25-18-4060-PM.png)
How to Understand & Calculate Statistical Significance [Example]
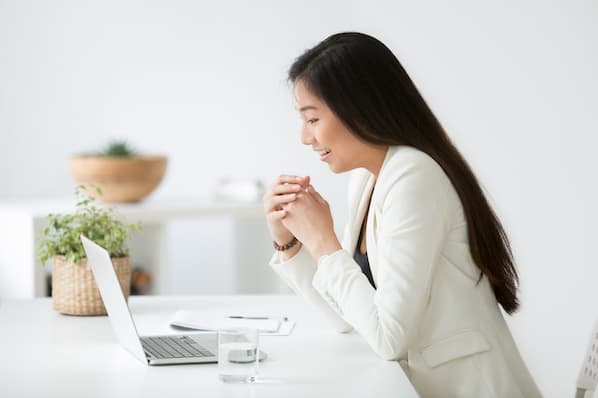
What is an A/A Test & Do You Really Need to Use It?
Learn more about A/B and how to run better tests.
Marketing software that helps you drive revenue, save time and resources, and measure and optimize your investments — all on one easy-to-use platform

- SUGGESTED TOPICS
- The Magazine
- Newsletters
- Managing Yourself
- Managing Teams
- Work-life Balance
- The Big Idea
- Data & Visuals
- Reading Lists
- Case Selections
- HBR Learning
- Topic Feeds
- Account Settings
- Email Preferences
A Refresher on A/B Testing

Spoiler: Many people are doing it wrong.
A/B testing is a way to compare two versions of something to figure out which performs better. While it’s most often associated with websites and apps, the method is almost 100 years old and it’s one of the simplest forms of a randomized controlled experiment. This testing method has risen in popularity over the last couple of decades as companies have realized that the online environment is well-suited to help managers, especially marketers, answer questions like, “What is most likely to make people click? Or buy our product? Or register with our site?”. It’s now used to evaluate everything from website design to online offers to headlines to product descriptions. The test works by showing two sets of users (assigned at random when they visit the site) different versions of a product or site and then determining which influenced your success metric the most. While it’s an often-used method, there are several mistakes that managers make when doing A/B testing: reacting to early data without letting the test run its full course; looking at too many metrics instead of focusing on the ones they most care about; and not doing enough retesting to be sure they didn’t get false positive results.
It’s all about data these days. Leaders don’t want to make decisions unless they have evidence. That’s a good thing, of course, and fortunately there are lots of ways to get information without having to rely on one’s instincts . One of the most common methods, particularly in online settings, is A/B testing.

- Amy Gallo is a contributing editor at Harvard Business Review, cohost of the Women at Work podcast , and the author of two books: Getting Along: How to Work with Anyone (Even Difficult People) and the HBR Guide to Dealing with Conflict . She writes and speaks about workplace dynamics. Watch her TEDx talk on conflict and follow her on LinkedIn . amyegallo
Partner Center
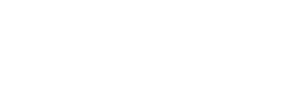
- See Demo Explore Product
The Leanplum Blog
Experiment Design: Your Framework to Successful A/B Testing
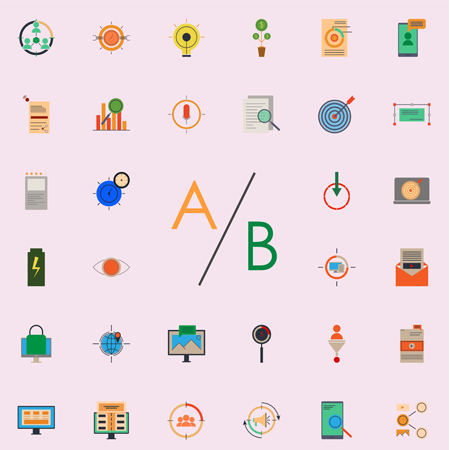
A/B testing — putting two or more versions out in front of users and seeing which impacts your key metrics — is exciting. The ability to make decisions on data that lead to positive business outcomes is what we all want to do.
Though when it comes to A/B testing, there is far more than meets the eye. A/B testing is not as simple as it’s advertised, i.e. “change a button from blue to green and see a lift in your favorite metric”.
The unfortunate reality of A/B testing is that in the beginning, most tests are not going to show positive results. Teams that start testing often won’t find any statistically significant changes in the first several tests they run.
Like picking up any new strategy, you need to learn how to crawl before you can learn how to run. To get positive results from A/B testing, you must understand how to run well-designed experiments. This takes time and knowledge, and a few failed experiments along the way.
In this post, I’ll dive into what it takes to design a successful experiment that actually impacts your metrics.
Setting Yourself Up for Success First up: Beyond having the right technology in place, you also need to understand the data you’re collecting, have the business smarts to see where you can drive impact for your app, the creative mind and process to come up with the right solutions, and the engineering capabilities to act on this.
All of this is crucial for success when it comes to designing and running experiments.
Impact through testing does not happen on a single test. It’s an ongoing process that needs a long-term vision and commitment. There are hardly any quick wins or low-hanging fruit when it comes to A/B testing. You need to set yourself up for success, and that means having all those different roles or stakeholders bought into your A/B testing efforts and a solid process to design successful experiments. So, before you get started with A/B testing, you need to have your Campaign Management strategy in place.
When you have this in place, you’re ready to start. So how do you design a good experiment?
Designing an Experiment The first step: Create the proper framework for experimentation. The goal of experimentation is not simply to find out “which version works better,” but determine the best solution for our users and our business.
In technology, especially in mobile technology, this is an ongoing process. Devices, apps, features, and users change constantly. Therefore, the solutions you’re providing for your users are ever-changing.
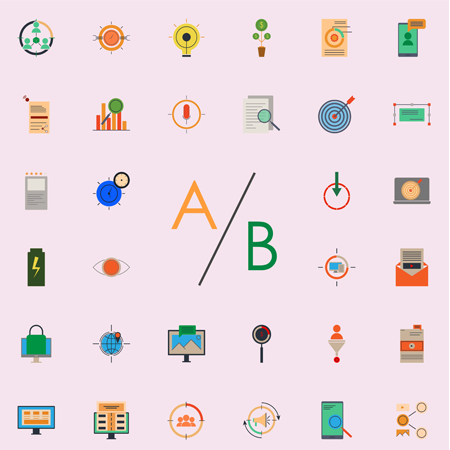
Finding the Problem The basics of experimentation starts — and this may sound cliché — with real problems. It’s hard to fix something that is not broken or is not a significant part of your users’ experience. Problems can be found where you have the opportunity to create value, remove blockers, or create delight.
The starting point of every experiment is a validated pain point. Long before any technical solution, you need to understand the problem you chose to experiment with. Ask yourself:
- What problems do your users face?
- What problems does your business face?
- Why are these problems?
- What proof do have that shows these are problems? Think surveys, gaps or drops in your funnel, business cost, app reviews, support tickets etc. If you do not have any data to show that something is a problem, it’s probably not the right problem to focus on.
Finding Solutions (Yeah, Multiple) Once the problem is validated, you can jump to a solution. I won’t lie, quite often you will already have a solution in mind, even before you’ve properly defined the problem. Solutions are fun and exciting. However, push yourself to first understand the problem, as this is crucial to not just find a solution but finding the right solution.
Inexperienced teams often run their first experiments with the first solution they could think of: “This might work, let’s test it.” they say.
But they don’t have a clear decision-making framework in place. Often, these quick tests don’t yield positive results. Stakeholders in the business lose trust in the process and it becomes harder to convince your colleagues that testing is a valuable practice.
My framework goes as follows.
- Brainstorm a handful of potential solutions. Not just variants — completely different ways to solve the problem for your users within your product.
- Out of this list of eight, grab two-to-three solutions that you’ll mark as “most promising.” These can be based on gut feeling, technically feasible, time/resources, or data.
- Now for these two most likely solutions, find up to four variants for each of these solutions.
This process takes you from the one-set solution you started with to test against the control, to a range of about 10 solutions and variations that can help you bring positive results. In an hour of work, you increase your chances to create a winning experiment significantly.
Now you have your solutions, we’re almost ready to start the experiment. But first…
Defining Success We now have a problem and have a set of solutions with different variants. This means we have an expected outcome. What are we expecting to happen when we run the test and look at the results?
Before you launch your test, you need to define upfront what success will look like. Most successful teams have something that looks like this:
- Primarily decision-making metric: The primary decision-making metric is the goal metric that you want to impact with your test. It’s the single most important user behavior you want to improve.
- Secondary decision-making metrics: These are often two-to-three metrics. They are directly impacted by the experiment, but aren’t the most important metric. The secondary metrics create context for the primary decision-making metric, and help us make the right decisions. Even if the primary metric is positive, but there is too much of a decline in the secondary metrics, this could impact your decision if the experiment was a success or not.
- Monitoring metric: These are extremely important. You don’t use them to make a decision on the success of the outcome of the experiment, but on the health of the environment of the experiment.
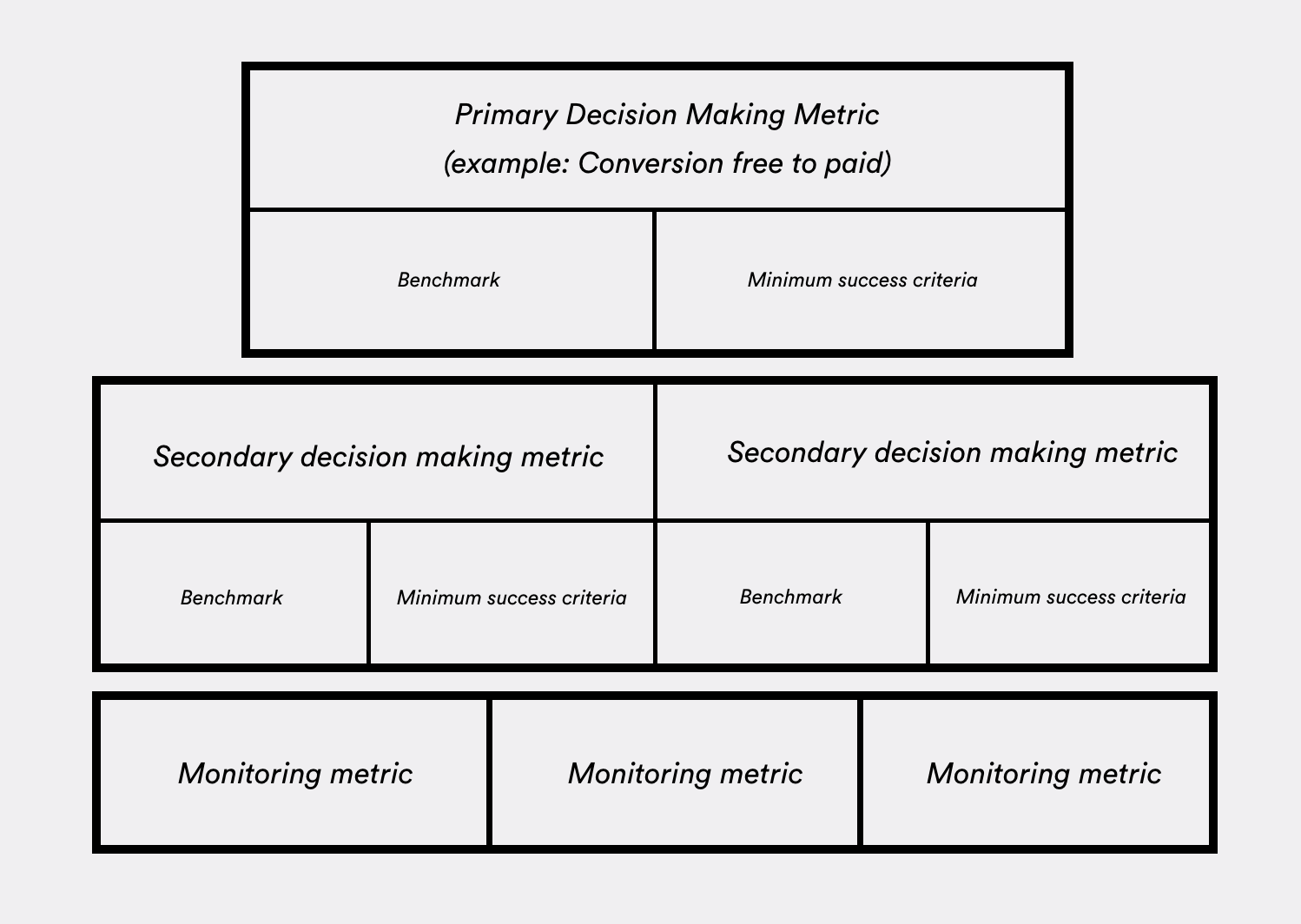
With an A/B test, we want to have a controlled environment where we can decide if the variant we created has a positive outcome. Therefore, we need monitoring metrics to ensure the environment of our experiment is healthy. This could be acquisition data, app crash data, version control, and even external press coverage.
Setting the Minimum Success Criteria Alongside the predefined metrics on which you’ll measure the success of your experiment, you need a clear minimum success criteria. This means setting a defined uplift that you consider successful. Is an increase of 10 percent or 0.5 percent needed to be satisfied about the problem we’re trying to solve?
Since the goal of running an experiment is to make a decision, this criteria is essential to define. As humans, we’re always easily persuaded. If we don’t define upfront what success looks like, we may be too easily satisfied.
For example: If you run a test and see a two percent increase on your primary decision-making metric, is that result good enough? If you did not define a success criteria upfront, you might make the decision that this is okay and roll out the variant to the full audience.
However, as we have many different solutions still on the backlog, we have the opportunity to continue our experimentation and find the best solution for the problem. Success criteria help you to stay honest and ensure you find the best solution for your users and your business.
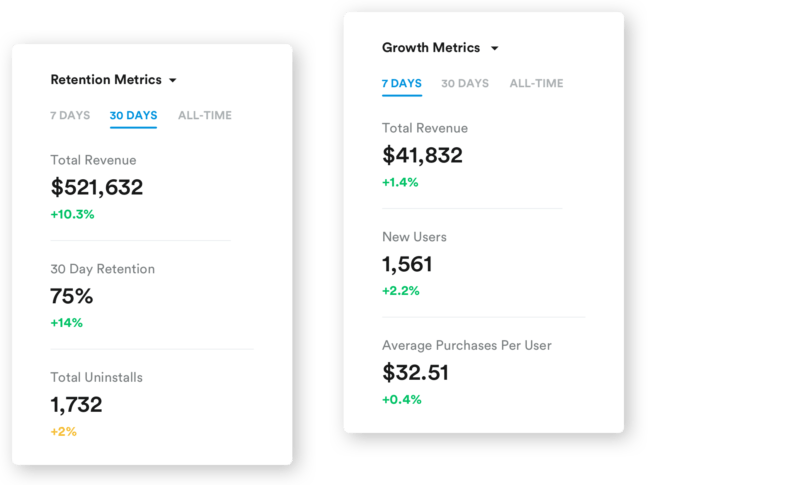
Share Learnings With Your Team Finally, share your learnings. Be mindful here that sometimes learnings come from a combination of experiments where you optimized toward the best solution.
When you share your learnings internally, make sure that you document them well and share with the full context — how you defined and validated your problem, decided on your solution, and chose your metrics.
My advice would be to find a standard template that you can easily fill out and share internally. Personally, I like to keep an experiment tracker . This allows you to document every step and share the positive outcomes and learnings.
Creating a Mobile A/B Testing Framework That Lasts All this is a lot of work — and it’s not always easy. Setting up your framework for experimentation will take trial, error, education, and time! But it’s worth it. If you skip any of the above steps and your experiment fails, you do not know where or why it failed and you are basically guessing again. We all know the notion of “Move fast and break things,” but spending a day extra to set up a proper test that gives the right results and is part of a bigger plan is absolutely worth it.
And don’t worry, you’ll still break plenty of things. Most experiments are failures and that is fine. It’s ok to impact a metric badly with an experiment. Breaking things mean that you’re learning and touching a valuable part of the app. This is the whole reason why you run an experiment, to see if something works better. Sometimes that is not the case… As long as you have well-defined experiment framework, you can justify why this happened and you can set-up a follow-up experiment that will help you find a positive outcome.
Leanplum is a mobile engagement platform that helps forward-looking brands like Grab, IMVU, and Tesco meet the real-time needs of their customers. Schedule your personalized demo here.
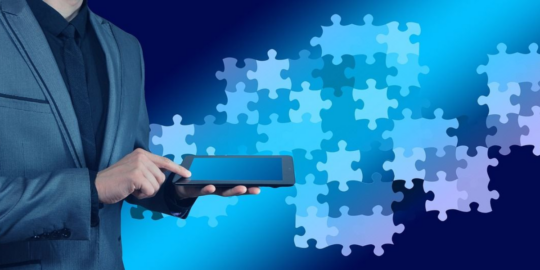
February 21st, 2023
Larry Hsieh on LiveOps: Keep the Game Relevant With Minimal Resources
- Engagement strategies
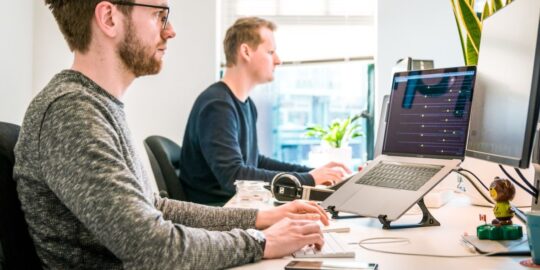
January 31st, 2023
Best Practices: Running LiveOps Events on a Shoestring Budget
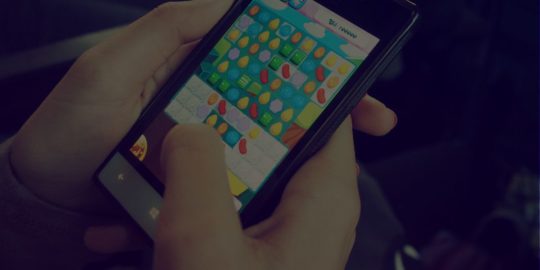
January 10th, 2023
Preparing for Your LiveOps Event
- Reviews / Why join our community?
- For companies
- Frequently asked questions
A/B Testing
What is a/b testing.
A/B testing, or split testing, is a quantitative user research method. In A/B testing, researchers show different users two versions of the same design to identify which one performs better. The A refers to the original design, while the B refers to the variation of the A design.
- Transcript loading…
Researchers and designers use A/B testing to test individual page elements or minor layout variations . They keep everything else on the page the same except the aspect they want to test. This way, they know that any difference in results comes from the variation alone.
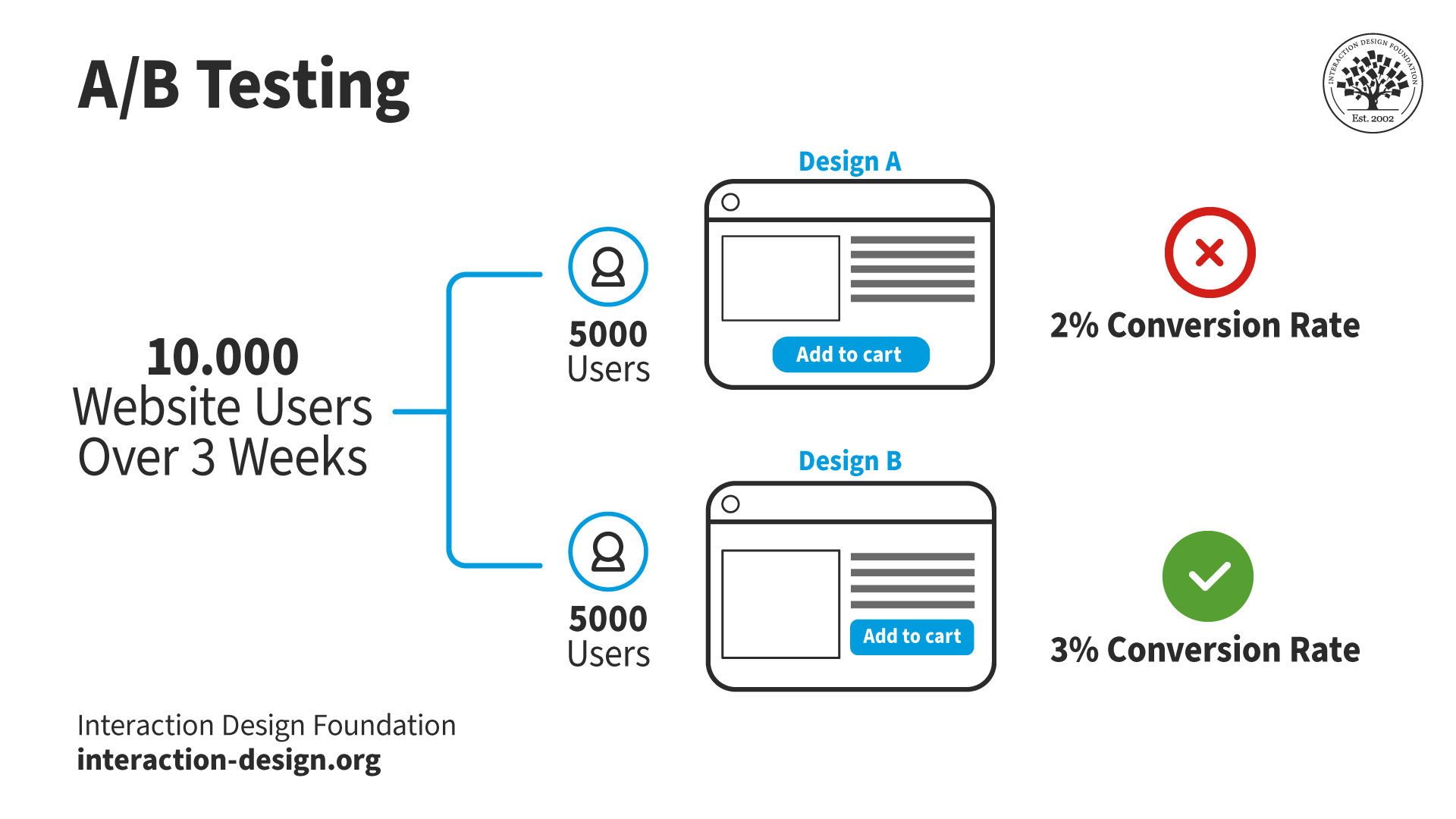
A/B testing is quick, easy, and cheap. Designers and researchers choose this method to test slight differences in their designs.
© Interaction Design Foundation, CC BY-SA 4.0
For example, the online streaming platform, Netflix, used A/B/n testing to find which call to action button resulted in more sign-ups. A/B/n testing extends A/B testing by incorporating more than one design variant.
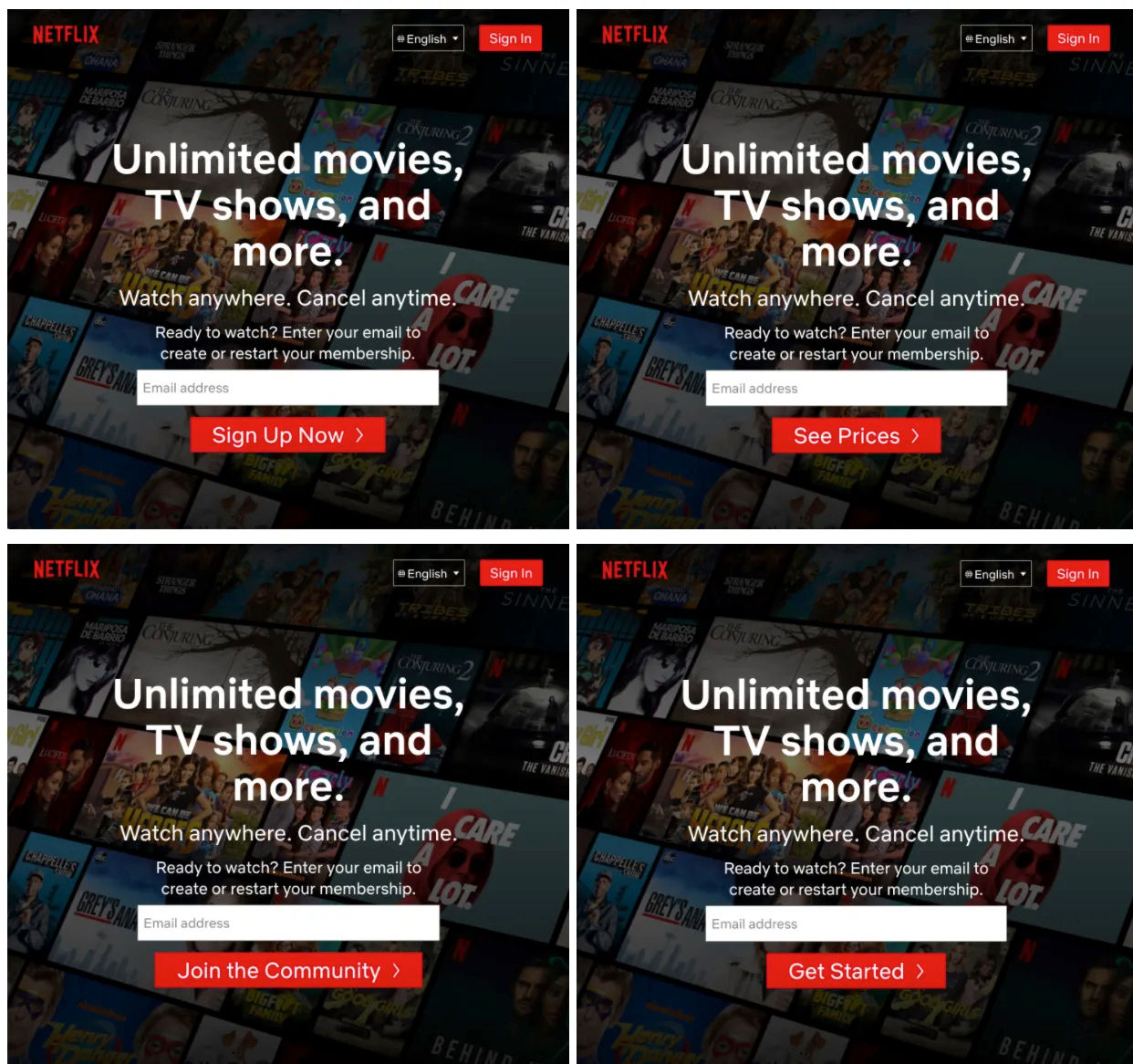
Netflix split site visitors between these four design alternatives. They kept everything the same except the button to ensure their results would be reliable. Once the test was complete, they implemented the phrase “Get Started,” as it resulted in significantly more sign-ups than the other three designs.
© Netflix, Fair use
What Can Designers A/B Test?
A/B testing typically measures the difference in conversion rate between two designs. The conversion rate is the percentage of users who complete a desired action. Some example actions include:
Add item to cart.
Donate money to charity.
Sign up for a newsletter.
Click a specific item in a menu.
Other metrics that A/B testing can measure include:
The time a user spends on a page or site.
The percentage of users who leave a site after viewing only one page (the bounce rate).
A/B testing is limited in what it can measure. However, the variables that researchers can A/B test are almost limitless. Researchers change one variable between design variants and compare the metrics . Here are some examples of variables:
|
|
|
|
| Style (horizontal vs. vertical) | Icons vs. text | Placement (top, bottom, side) |
| Number of columns | Above-the-fold content | Sidebar presence and position |
| Color | Shape and size | Text (“Add to Cart” vs. “Buy Now”) |
| Number of fields | Field types (dropdowns, text input) | Layout and ordering of fields |
| Font styles and sizes | Text color and contrast | Line spacing and text alignment |
| Placement and size | Static vs. carousel | Thumbnails vs. full-size images |
| Overall color theme | Contrast ratios | Button and link colors |
| Placement on the page | Wording and urgency | Design and visibility |
| Headlines and subheadings | Length and style of copy | Use of bullet points vs. paragraphs |
| Alt text for images | Keyboard navigation | Screen reader friendliness |
| Wording and tone | Instructions for resolution | Sound effects |
| Search box placement and design | Search algorithms | Filters and sorting options |
| Timing and frequency | Offer types (newsletter sign-up, discount codes) | Exit-intent vs. timed display |
| Placement and timing | Incentives (discounts, ebooks) | Design elements |
| Timing and frequency | Content and call to action | Sound effects |
| Meta titles and descriptions | Headings structure (H1, H2, H3) | Keyword placement |
| Pricing display ($10 vs. $9.99) | Subscription models vs. one-time purchases | Anchor Pricing (display a higher priced item next to the main product) |
| Types of discounts (percentage off vs. buy one get one) | Placement of sale information | Original price crossed out vs. savings amount highlighted |
Is A/B Testing Worth It?
“Testing leads to failure, and failure leads to understanding.” —Burt Rutan
User researchers and designers use testing to make data-driven design decisions and optimize their products' user experience (UX) . A/B testing is a highly effective user research method that is:
Cost-effective. Researchers can implement A/B testing with live users following deployment. This approach eliminates the need for expensive pre-launch testing environments. For example, a product manager wants to test two landing pages to see which results in more sign-ups. They split the website's traffic between the two versions. The A/B test gives them valuable data without a significant increase in costs.
Efficient. A/B testing provides rapid results, especially for products with substantial user bases. Sometimes, two weeks of testing is enough to collect actionable data.
Straightforward. Analytics tools provide researchers with clear insights into which design variant performs best . Researchers evaluate outcomes based on predefined metrics, like conversion rates. For instance, a researcher tests two call to action buttons. Analytics reveal the variant that leads to higher conversions. These results provide a clear directive for researchers on which element enhances the user experience.
When Should Designers Use A/B Testing?
In this video, William Hudson explains how to fit quantitative research into the project lifecycle:
A/B testing is unsuitable for assessing the qualitative aspects of user experience. Qualitative aspects include:
Satisfaction.
Comprehension.
Emotional response .
Given this, researchers must know what they want to achieve before testing.
For instance, if a researcher relies solely on A/B testing to enhance user satisfaction, it would not provide the insights needed. A/B testing can show users spend more time on a page but cannot explain why users feel more engaged.
When researchers want to understand the 'why' behind user behaviors, they use other research methods. More suitable methods include user interviews , usability testing and surveys . These methods complement the quantitative data from A/B testing.
What Are the Requirements for A/B Testing?
Before a researcher can conduct an A/B test, their website or app must be fully functional. Test results will be unreliable for unfinished products.
For instance, a designer wants to test a product page for a mobile phone case. The page has:
A dropdown menu to choose the case color.
Product photos that change when the user selects a different color.
An “add to basket” button.
The designer creates two designs with different "add to basket" button placements. However, the drop-down list is not functioning correctly. When the user chooses a case color, the product photos change to the wrong color. If users become frustrated, the button's placement will unlikely affect their decision to add to the basket. Any results from the test will be unreliable.
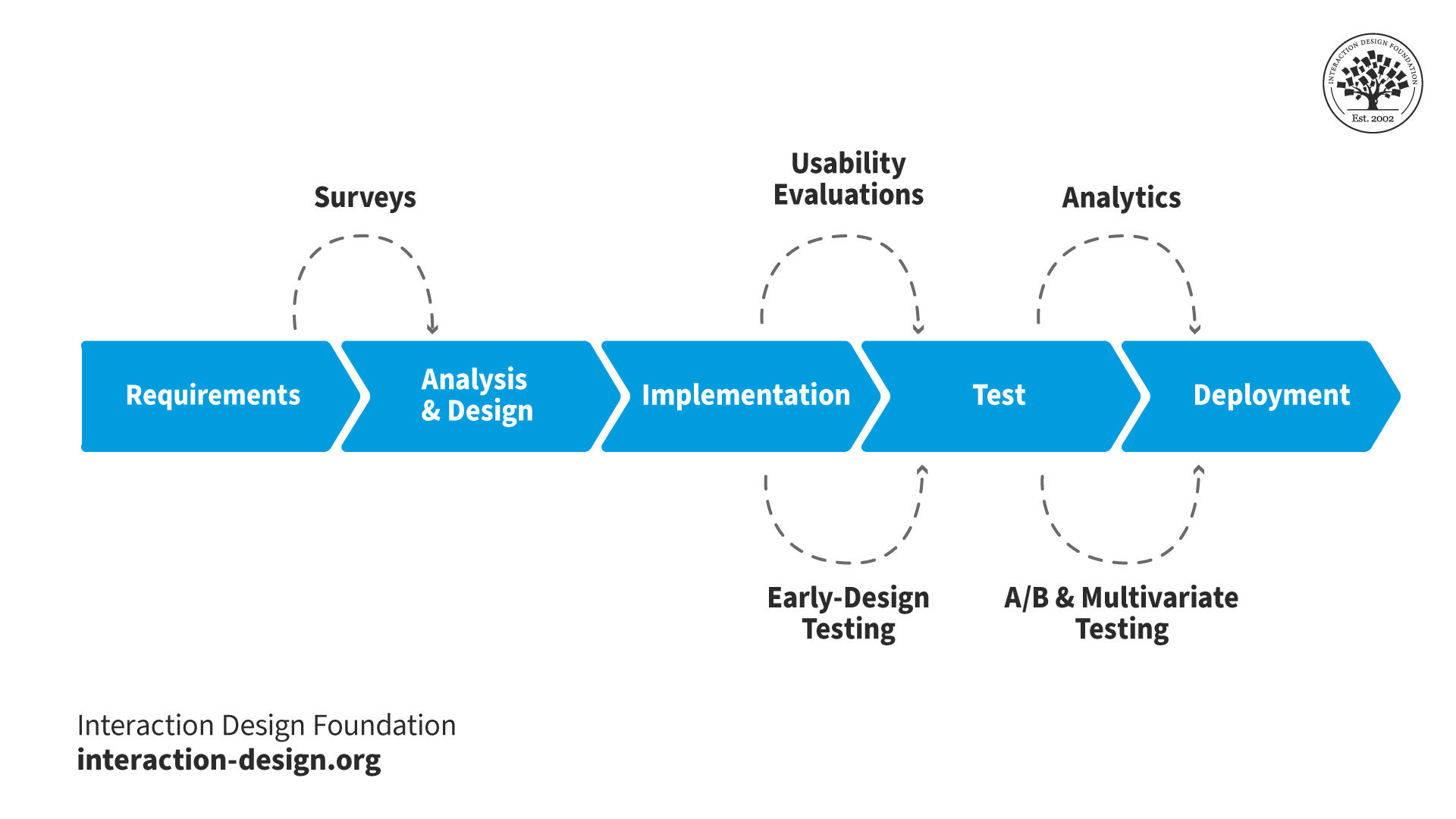
Designers and researchers use A/B testing in the late stages of the development cycle or after deployment. A/B tests need stable, well-designed environments to function correctly.
Also, the number of users tested must be significant enough to see actionable results. Researchers can conduct longer tests for smaller audiences to reach the required sample size. A/B/n testing requires a larger pool of users than A/B testing . More design alternatives mean more participant groups.
A/B sample size calculators help researchers specify a target sample size based on their website’s existing analytics.
Good vs. Bad Research Questions for A/B Testing
Before user researchers conduct testing, they define the questions they want to answer. An example of a bad question is, “Will better product photos reduce the number of customer service queries?” Researchers cannot effectively A/B test this. Many channels to customer service exist, not just product pages.
In this scenario, a good question is, “Will different product photos improve conversions?” Researchers split their users between two different designs, each with different product photos. If significantly more users purchase the product via design B, researchers can be confident:
Users are ordering more.
They are less likely to go to customer service.
Another bad example is, “Will shortening the sign-up process improve user satisfaction?” Satisfaction is challenging to measure with A/B testing, and many ways exist to shorten a sign-up process. The question must be more specific and design-related . For example, “Which design, A or B, leads to more sign-ups?”
How to Run an A/B Test
Once researchers and designers are confident their product is sound and has enough users, they follow a three-part process for A/B testing.
Pre-requisites
Researchers do not need to complete these steps each time they A/B test. However, for first-time A/B testing, these steps are crucial:
Identify key stakeholders . Discover who needs to agree or give resources for the testing. Requirements include getting:
Funding and permission from managers.
Access to existing A/B testing tools and data.
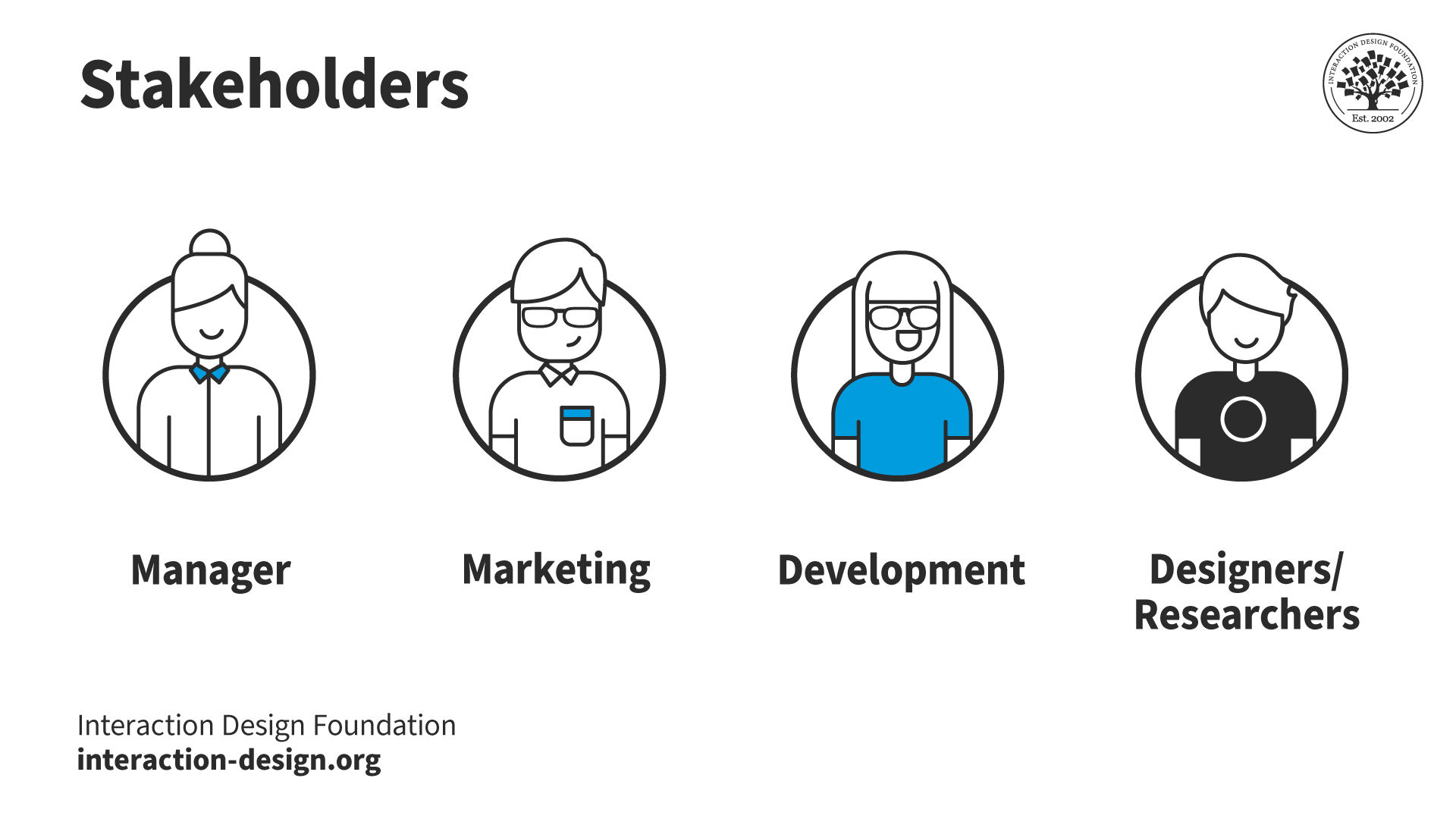
While A/B testing is inexpensive, managers must still approve its use. Marketing or development teams may hold the keys to existing analytics implementations. Finally, design and research colleagues may need to create alternative designs and run the test.
Convince stakeholders of A/B testing's value. It's crucial everyone involved understands why A/B testing is useful. This understanding is critical in scenarios where stakeholders might not be familiar with UX design. Clear examples, like stories of past successes, show stakeholders how A/B testing has helped other projects or companies.
Set up the necessary tools. Choose and set up the software for web analytics and A/B testing. Find the right tools that fit the project's needs and set them up.
Preparation
Once researchers have the required access, permissions and funding, they prepare for the test:
Define research questions. Decide the questions that need answering. For example, “Will changing the button color of a call to action result in more clicks?”
Design the alternatives. Next, create the designs you will test against each other. Make sure these designs are as perfect as possible. For shorter tests, some flaws are acceptable.
Select your user group(s) (optional). Most A/B testing and analytics software allows you to filter results by user group. For this reason, testing specific groups is not always necessary, as you can specify this later. However, if the software doesn’t allow this, you should define this before testing.
Plan your schedule. Finally, decide on a timeline for your test that includes when you'll start, how long it will run and when you'll check on the results. A clear schedule helps manage the test without wasting time or resources.
Results Follow-Up
Once the testing period has finished, researchers view the results and decide their next steps:
Check if the results are reliable. Look at the analytics to see if the differences are significant enough. Minor differences between the performance of designs A and B may be chance. Researchers use methods like chi-square tests to determine whether the results are significant.
If the results are unclear , change the designs and rerun the test, or run the test longer to get more data. These solutions help make sure the next test gives more apparent answers.
If the results are clear , implement the better version.
Keep improving . Researchers don’t only A/B test once; it's an ongoing process. Findings inform and inspire future tests.
Chi-Square Testing for Statistical Significance
Researchers interpret A/B test results to make informed decisions about design choices. A/B testing results are typically straightforward (e.g., which design resulted in more conversions). However, researchers must determine if the results are statistically significant.
Researchers use the chi-square test, a fundamental statistical tool. Chi-square tests play a pivotal role in A/B testing. They reveal whether observed results are statistically significant or chance findings.
Chi-square test results are easy to interpret. If the test indicates a significant difference, researchers can be confident which design is best. For example, a researcher tests two web page versions to increase conversions:
Version A gets 5000 visitors with 100 sign-ups.
Version B gets 5000 visitors with 150 sign-ups.
The researcher analyzes these results using an online chi-square calculator:
They enter each design's successes (sign-ups) and failures (no sign-ups).
They set the significance level at 0.05 (or 5%—the most typical level).
The chi-square test provides a P-value of 0.001362, which is lower than the significance level . Any P-level value under 0.05 is considered statistically significant, while any value over is considered chance.
In this scenario, the researcher is confident their results are statistically significant. They can make design decisions based on these results.
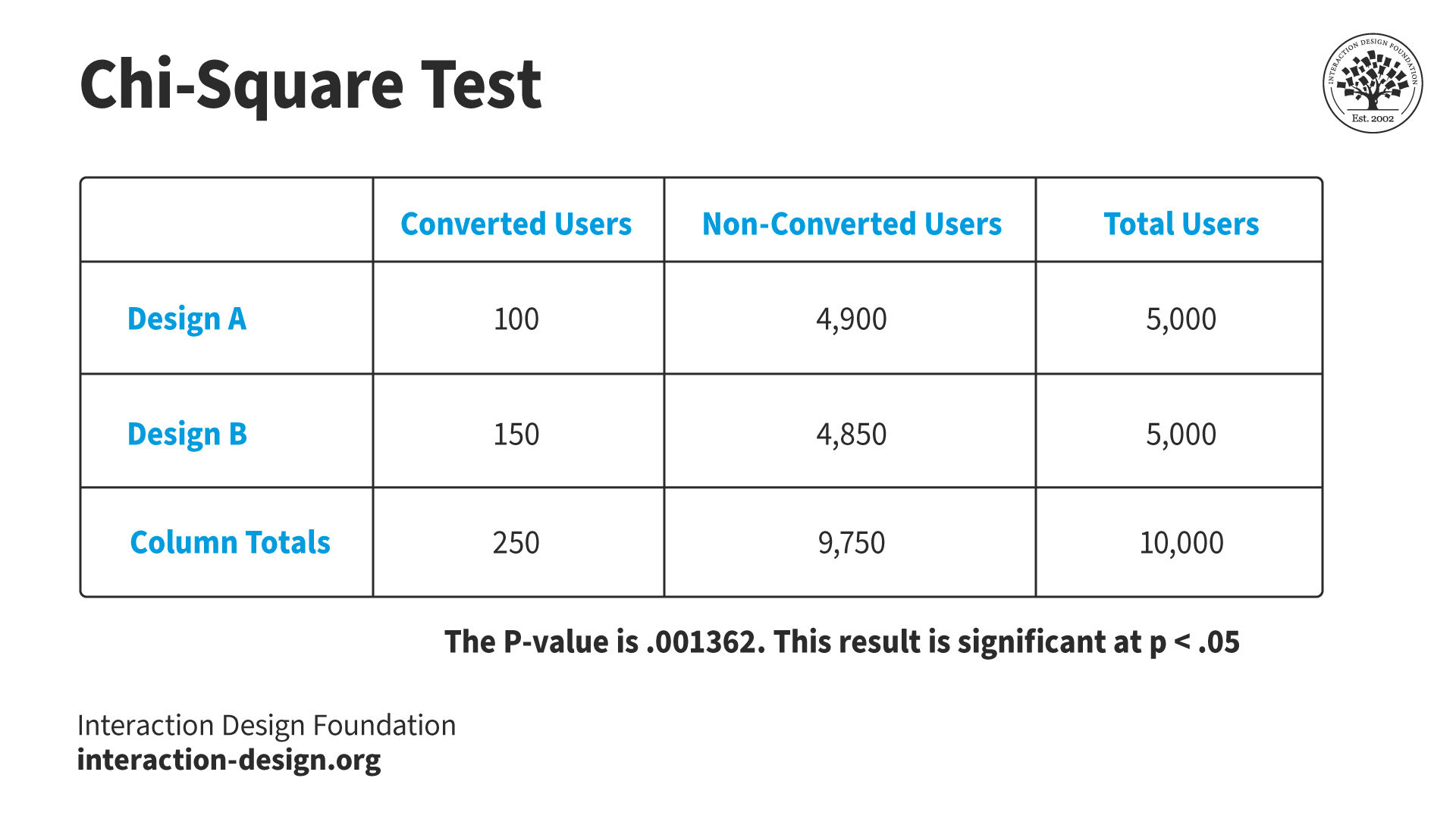
The chi-square test determines if A/B test results are statistically significant. In this example, the difference between conversions may seem small compared to the total users. However, the P-value (the output of the chi-square test) is much lower than the significance level—it is statistically significant. Chi-square tests give researchers and designers the confidence to make data-driven decisions.
Best Practices
Researchers follow these best practices to run A/B tests:
Understand the platform well. Researchers should be familiar with the product before conducting A/B testing. A lack of knowledge leads to unreliable and unuseful results within the context of the platform.
Know the users . Researchers must understand who their users are and what they need from the product. This knowledge is available from existing user research, data and findings.
Choose what to test wisely. Researchers focus on the parts of their site that affect their users the most. For example, an excellent place to start is with user complaints. Other sources, like heat maps and session recordings, provide researchers with test subjects.
Talk to stakeholders. Management and other departments might see problems or have ideas the design team is unaware of.
Set clear goals. Researchers know what they want to achieve with A/B testing. They set measurable goals to guide testing and ensure relevance and focus.
Small changes, big impact. Design changes should be small. Significant changes and overhauls can confuse and upset users. Researchers focus on minor tweaks that make substantial differences.
Use segmentation. Segmentation is helpful after a completed test to review different user groups. Researchers compare demographics and segments like mobile and desktop website visitors.
Limitations and Challenges
A/B testing is typically straightforward and inexpensive. However, researchers must be aware of its limitations and potential stumbling blocks.
Requires a large user base. A/B testing only provides trustworthy results with a sufficient user pool. Without enough people, it might take longer to get results, or the findings might not be reliable.
Outside factors can influence results. External factors like seasonal changes and new trends can negatively affect results. For example, a retailer runs an A/B test on their website during the holiday season to determine the effectiveness of new product photos. However, the increased traffic and buying intent during the holiday season inflates the success of the images. In a regular season, the photos would likely not perform as well.
Focuses on short-term goals. A/B testing typically focuses on immediate results, like how many people click on a button. Long-term goals like customer happiness and brand loyalty are difficult to assess. For instance, a news website runs an A/B test comparing two headline styles to see which generates more clicks. One style leads to a higher click-through rate but relies on clickbait titles that may erode trust over time.
Ethical Concerns. Some tests significantly change what users experience or how products handle their privacy. In these scenarios, researchers must consider ethical practices. For example, an e-commerce site tests an alternative checkout process that adds a last-minute upsell offer. The offer could frustrate users who want to complete their purchases quickly.
A/B vs. Multivariate Testing
Researchers use multivariate testing to test multiple variables between two or more designs. This method is more complex than A/B testing. Researchers may choose multivariate testing over A/B testing for the following reasons:
Complex interactions. It is suitable for examining how multiple variables interact with one another. Multivariate testing can provide insights into more complex user behaviors.
Comprehensive analysis. It allows for a more detailed analysis of how different elements of a page or product work together. This detail can lead to more nuanced improvements.
Optimizes multiple variables simultaneously. It is ideal for optimizing several aspects of a user experience at once. This optimization can lead to significant improvements in performance.
For example, during the 2008 US presidential election, the Obama campaign used multivariate testing to optimize newsletter sign-ups. They tested different combinations of their homepage media (an image or a video) and the call to action button. The team preferred one of the videos. However, testing revealed that an image performed better. This example highlights the importance of user testing and user-centered design.
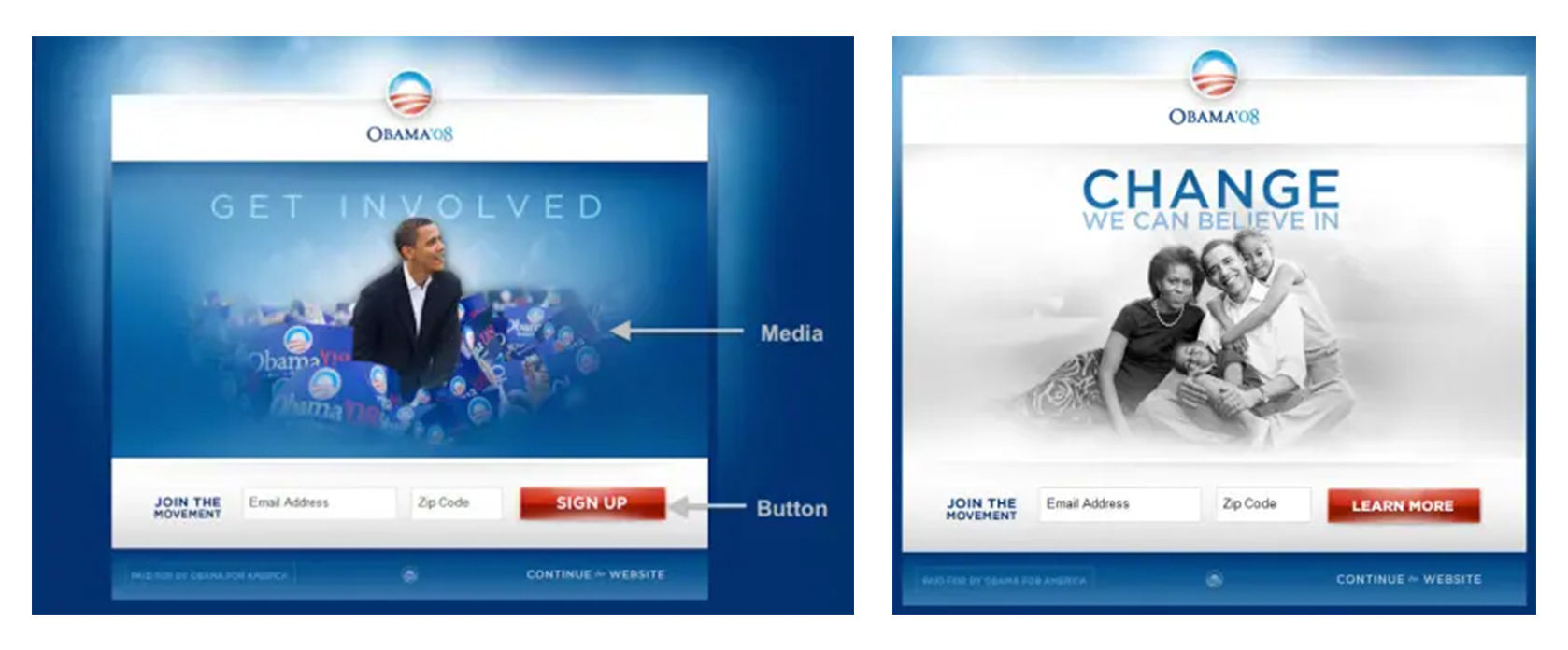
The Obama campaign tested 4 CTA and six media variations (three images and three videos). They found that design option 11 (right image) had 40.6% more signups than the original page (left image). They implemented the more successful design, translating to approximately 2,880,000 additional signups throughout the campaign. These extra signups resulted in an additional USD 60,000,000 in donations.
© Optimizely, Fair use
Researchers may choose A/B testing over multivariate testing for the following reasons:
Simplicity and focus. It is more straightforward to set up and analyze, comparing two versions of a single variable to see which performs better.
Quick to implement. It allows for rapid testing and implementation of changes. This efficiency is ideal for iterative design improvements.
Requires less traffic. It achieves statistically significant results with less traffic. This benefits sites with smaller user bases.
Clear insights. Offers straightforward insights, making it easier to make informed decisions.
Alternatives to A/B Testing
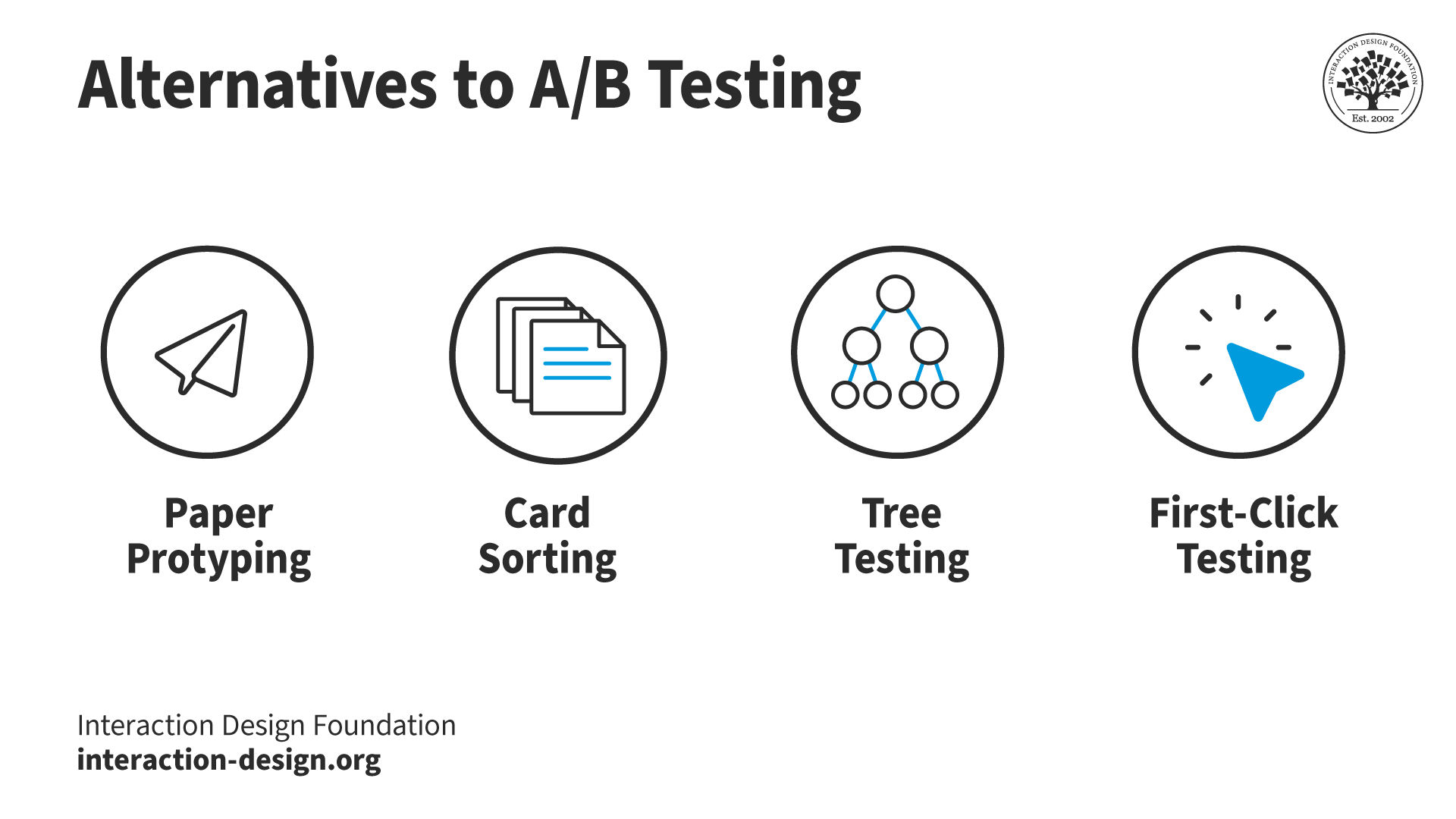
Researchers employ many types of research methods. A/B testing is a powerful tool, but other methods can be more appropriate depending on the situation.
User researchers understand various user research methods. While A/B testing is helpful in many situations, here are four alternatives and why researchers might choose them instead.
Paper prototyping is an early-stage method researchers use for quick, hands-on idea testing. Unlike A/B testing, paper prototyping is about ideation and immediate reactions. Researchers use this method to generate quick feedback on basic design concepts. Paper prototyping happens before the costly development phase. This approach helps researchers quickly identify user preferences and usability hurdles.
Card sorting dives deep into how users mentally organize information. This method offers insights that are sometimes not revealed in A/B testing. Researchers employ card sorting to structure or restructure a product's information architecture. Users group content into categories and reveal patterns that guide information organization. This method ensures the final structure aligns with user expectations.
Tree testing focuses on evaluating the navigational structure of a site. Designers and researchers use this method to refine an existing navigation. Tree testing can also confirm a new structure's usability. This method strips away the visual design elements and focuses on how easily users can find information. Researchers choose this targeted approach over A/B testing to identify navigational issues.
First-click testing assesses a web page layout's immediate clarity and key actions. Researchers use this method to understand if users can quickly determine where to click to complete their goals. A/B testing does not always reveal this information. First-click testing offers precise feedback on the effectiveness of the initial user interaction.
Learn more about A/B Testing
Learn more about A/B testing and other practical quantitative research methods in our course, Data-Driven Design: Quantitative Research for UX .
Jakob Nielsen discusses how A/B testing often puts the focus on short-term improvements .
Find out how and why Netflix implements A/B testing across their platform .
Learn how to Define Stronger A/B Test Variations Through UX Research with the Nielsen Norman Group.
Discover how the 2008 Obama presidential campaign used multivariate testing .
Watch our Master Class with Zoltan Kollin, Design Principal at IBM, for further insights into A/B testing
Questions related to A/B Testing
A large portion of A/B tests do not show a clear improvement. Various factors can contribute to this high failure; for example:
Small sample sizes.
Short testing periods.
Minor changes that don't significantly impact user behavior.
However, these "failures" are invaluable learning opportunities. They provide insights into user preferences and behavior. These insights help researchers refine their hypotheses and approaches for future tests.
To increase the success rate of A/B tests, researchers ensure they have:
A clear hypothesis.
A sufficiently large sample size.
A significant enough variation between the tested versions.
A sufficient test duration to account for variability in user behavior over time.
Don Norman, founding director - Design Lab, University of California, explains how every failure is a learning opportunity:
To conduct A/B testing, researchers can use various tools to set up design alternatives and measure outcomes. Popular tools include:
Google Optimize offers seamless integration with Google Analytics (GA). This integration allows researchers to use their existing GA goals as test objectives. Researchers can easily visualize how their experiments impact user behavior.
Optimizely is a powerful tool that allows extensive experimentation. Researchers can use this platform across websites, mobile apps and connected devices. Optimizely makes it easy for researchers to create and modify experiments without writing code.
VWO (Visual Website Optimizer) provides a suite of tools, including A/B testing, multivariate testing, and split URL testing. VWO’s interface is designed for marketers, making it accessible for those with limited technical skills.
Unbounce is best for testing landing pages. Its drag-and-drop editor enables researchers to create and test landing pages without developer resources.
Adobe Target is part of the Adobe Experience Cloud. This tool suits businesses looking for deep integration with other Adobe products.
These tools allow researchers to make data-driven decisions that enhance user experience. However, success in A/B testing comes from more than just tools. Clear objectives, appropriate metrics and iteration based on findings lead to profitable outcomes.
William Hudson, CEO of Syntagm, UX Expert and Author, explains how researchers and designers use analytics in UX design:
Author: Stewart Cheifet. Appearance time: 0:22 - 0:24. Copyright license and terms: CC / Fair Use. Modified: Yes. Link: https://archive.org/details/CC1218 greatestgames
If both versions in an A/B test perform similarly, it suggests the changes tested did not significantly impact user behavior. This outcome can have several reasons:
Insensitivity to changes. The tested element might not influence user decisions.
Need for more significant changes. Consider testing more noticeable variations.
Well-optimized existing design. The current design effectively meets user needs.
Inconclusive results. The test duration was too short, or the sample size too small.
If A/B tests remain inconclusive, researchers should use different methods to explore more profound insights. Methods include surveys, interviews and usability testing.
Develop a foundational understanding of user research with our course, User Research: Methods and Best Practices .
A/B testing results can mislead due to:
Methodological errors. Unclear questions, biased groups and test groups that are too small.
Incorrect data interpretation. Confusion about significance, not seeing random changes and bias towards expected outcomes.
Overlooking factors. Time of year, market changes and technology updates.
Here's how researchers can mitigate these risks:
Test for statistical significance. Confirm if results are statistically significant or chance findings.
Control external factors. Isolate tests from external factors or account for them.
Run tests for adequate duration. Capture user behavior variations with sufficient test periods.
Avoid multiple changes. Test one design change at a time for clear outcomes.
Focus on user experience. Consider long-term user satisfaction and retention impacts.
Peer review. Ask colleagues to review findings for overlooked errors or biases.
Continuous testing. Refine understanding through ongoing testing and iteration.
This risk mitigation allows researchers and designers to make informed design decisions. Take our course, Data-Driven Design: Quantitative Research for UX , to learn how to run successful A/B and multivariate tests.
User consent is pivotal in A/B testing amidst growing privacy concerns and strict data protection laws like GDPR and CCPA. Here's why user consent matters:
Ethical consideration. Ask for user consent before data collection. This approach honors user privacy and autonomy.
Legal compliance. Explicit consent is often mandatory for data collection and processing. A/B testing data can sometimes personally identify users.
Trust building. Brands that communicate their data practices clearly and respect user choices often gain user trust.
Data quality. Consented participation typically comes from engaged and informed users. This type of user usually provides higher-quality data.
To weave user consent into A/B testing:
Clearly inform users. Clearly explain the A/B test's nature, the data to be collected, its use and the voluntary basis of their participation.
Offer an opt-out. Ensure an accessible opt-out option for users that acknowledges their privacy and choice rights.
Privacy by design. Embed privacy considerations into A/B testing frameworks from the outset. Focus on essential data collection and securing it properly.
Researchers incorporate user consent to align with legal requirements and strengthen user relationships. Learn more about credibility, one of the seven key factors of UX, in this video:
A few key differences exist between A/B testing for B2B (business-to-business) and B2C (business-to-consumer) products:
Decision-making process. B2B tests target multiple stakeholders in longer processes. B2C focuses on emotional triggers and immediate value for individual consumer decisions.
Sales cycle length. B2B's longer sales cycles require extended A/B testing durations. B2C's shorter cycles allow for rapid testing and iterations.
Content and messaging. B2B A/B testing emphasizes information clarity and return-on-investment (ROI) demonstration. B2C testing focuses on emotional appeal, usability, and instant gratification.
Conversion goals. B2B tests often aim at lead generation (e.g., form submissions and whitepaper downloads). B2C targets immediate sales or sign-ups.
User volume and data collection. B2C's more extensive user base facilitates richer data for A/B testing. B2B's niche markets may necessitate more extended tests or multivariate testing for significant data.
User behavior. B2B testing focuses on functionality and efficiency for business needs. B2C prioritizes design, ease of use and personal benefits.
Regulatory considerations. B2B faces stricter regulations affecting test content and data handling. B2C has more flexibility but must respect privacy laws.
Researchers must understand these differences to conduct A/B testing in each domain effectively.
While A/B testing is well known for optimizing website conversion rates and user experience, it is helpful in other areas:
Content strategy. A/B testing can inform what most engages your audience. Refine strategies by testing storytelling methods, article lengths and formats (videos vs. text).
Email design. Test newsletters to enhance open rates and engagement. Experiment with alternative layouts, imagery and interactive features to understand visual preferences.
Voice and tone. Tailor communication to your users effectively. Experiment with voice and tone of content and copy to uncover user preferences.
Error messages and microcopy. Test microcopy variations like error messages to guide users through errors or challenges.
Accessibility. Improve the effectiveness of accessibility features. For example, test the accessibility toolbar placement where users engage with it more.
Torrey Podmajersky, Author, Speaker and UX Writer at Google, explains her process for writing notifications, which includes A/B testing:
King, R., Churchill, E., & Tan, C. (2016). Designing with Data: Improving the User Experience with A/B Testing . O’Reilly.
This book explores the relationship between design practices and data science. King, Churchill and Tan advocate for data-driven A/B testing to refine user experiences. The book details the process for implementing A/B testing in design decisions, from minor tweaks to significant UX changes. It includes real-world examples to illustrate the approach.
Kohavi, R., Tang, D., & Xu, Y. (2022). Trustworthy Online Controlled Experiments: A Practical Guide to A/B Testing . Cambridge University Press.
This book compiles the expertise of leaders from Google, LinkedIn and Microsoft. It covers the design, execution and interpretation of A/B tests. Kohavi, Tang and Xu offer insights into practical applications and real-world examples. Applications include enhancing product features, efficiency and revenue.
Georgiev, G. (2019). Statistical Methods in Online A/B Testing: Statistics for Data-Driven Business Decisions and Risk Management in E-commerce . Independent.
This book focuses on statistical methods for A/B testing. It demystifies complex concepts, making them accessible to professionals with minimal mathematical background. Georgiev covers practical applications in business, risk management and decision-making through online experiments. This book elevates the reader's A/B testing practices in various digital contexts.
Answer a Short Quiz to Earn a Gift
What is the main objective of A/B testing in product design?
- To assess a product's coding structure
- To directly survey user opinions
- To identify the best-performing design variations
How do designers typically split user traffic when A/B testing?
- Users are allowed to choose which version to see.
- Users are directed entirely to the variant(s).
- Users are split evenly between the original and the variant(s).
Which is the best situation for A/B testing?
- When designers conduct qualitative interviews for user feedback.
- When designers optimize minor design elements such as headlines or call-to-action buttons.
- When designers redesign the entire product interface for an improved customer experience.
Which metric is essential when to compare the performance of different versions in an A/B test?
- Code complexity
- Conversion rate
- Number of pages in the design
How does A/B testing contribute to the iterative design process?
- It allows designers to conduct this type of testing only once per project.
- It directly replaces the need for user research.
- It helps refine design variations through continuous testing and data collection.
Better luck next time!
Do you want to improve your UX / UI Design skills? Join us now
Congratulations! You did amazing
You earned your gift with a perfect score! Let us send it to you.
Check Your Inbox
We’ve emailed your gift to [email protected] .
Literature on A/B Testing
Here’s the entire UX literature on A/B Testing by the Interaction Design Foundation, collated in one place:
Take a deep dive into A/B Testing with our course Data-Driven Design: Quantitative Research for UX .
Quantitative research is about understanding user behavior at scale. In most cases the methods we’ll discuss are complementary to the qualitative approaches more commonly employed in user experience. In this course you’ll learn what quantitative methods have to offer and how they can help paint a broader picture of your users’ experience of the solutions you provide—typically websites and apps.
Since quantitative methods are focused on numerical results, we’ll also be covering statistical analysis at a basic level. You don’t need any prior knowledge or experience of statistics, and we won’t be threatening you with mathematical formulas. The approach here is very practical, and we’ll be relying instead on the numerous free tools available for analysis using some of the most common statistical methods.
In the “Build Your Portfolio: Research Data Project” , you’ll find a series of practical exercises that will give you first-hand experience of the methods we’ll cover. If you want to complete these optional exercises, you’ll create a series of case studies for your portfolio which you can show your future employer or freelance customers.
Your instructor is William Hudson . He’s been active in interactive software development for around 50 years and HCI/User Experience for 30. He has been primarily a freelance consultant but also an author, reviewer and instructor in software development and user-centered design.
You earn a verifiable and industry-trusted Course Certificate once you’ve completed the course. You can highlight it on your resume , your LinkedIn profile or your website .
All open-source articles on A/B Testing
What to test.
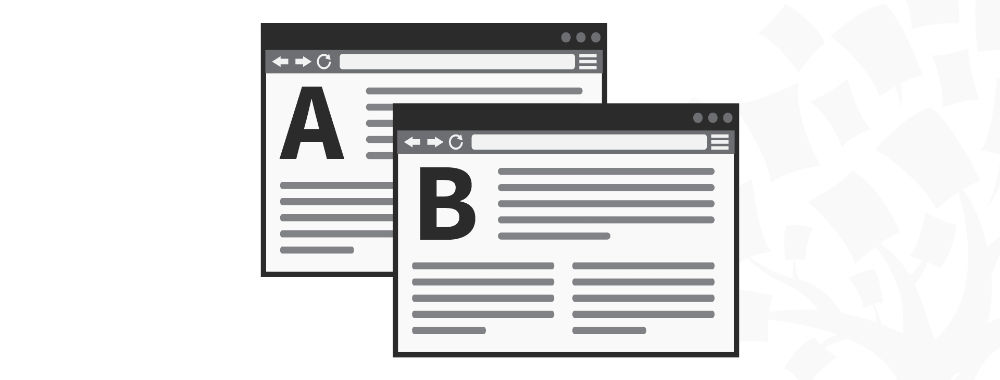
- 3 years ago
Revolutionize UX Design with VR Experiences
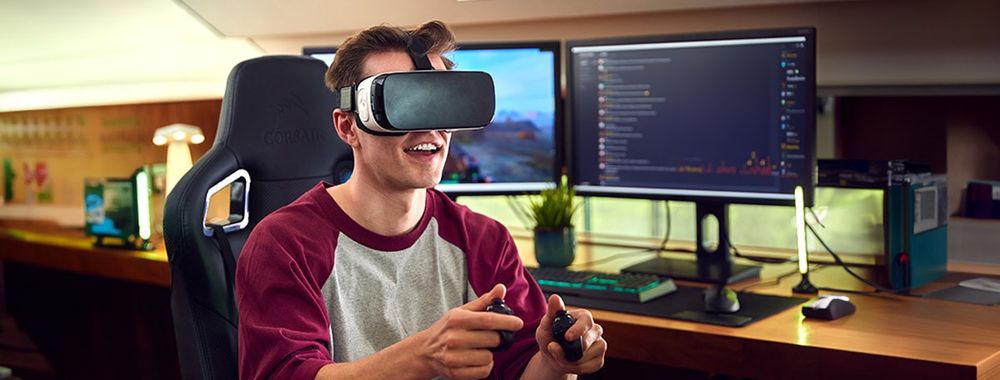
- 3 weeks ago
Open Access—Link to us!
We believe in Open Access and the democratization of knowledge . Unfortunately, world-class educational materials such as this page are normally hidden behind paywalls or in expensive textbooks.
If you want this to change , cite this page , link to us, or join us to help us democratize design knowledge !
Privacy Settings
Our digital services use necessary tracking technologies, including third-party cookies, for security, functionality, and to uphold user rights. Optional cookies offer enhanced features, and analytics.
Experience the full potential of our site that remembers your preferences and supports secure sign-in.
Governs the storage of data necessary for maintaining website security, user authentication, and fraud prevention mechanisms.
Enhanced Functionality
Saves your settings and preferences, like your location, for a more personalized experience.
Referral Program
We use cookies to enable our referral program, giving you and your friends discounts.
Error Reporting
We share user ID with Bugsnag and NewRelic to help us track errors and fix issues.
Optimize your experience by allowing us to monitor site usage. You’ll enjoy a smoother, more personalized journey without compromising your privacy.
Analytics Storage
Collects anonymous data on how you navigate and interact, helping us make informed improvements.
Differentiates real visitors from automated bots, ensuring accurate usage data and improving your website experience.
Lets us tailor your digital ads to match your interests, making them more relevant and useful to you.
Advertising Storage
Stores information for better-targeted advertising, enhancing your online ad experience.
Personalization Storage
Permits storing data to personalize content and ads across Google services based on user behavior, enhancing overall user experience.
Advertising Personalization
Allows for content and ad personalization across Google services based on user behavior. This consent enhances user experiences.
Enables personalizing ads based on user data and interactions, allowing for more relevant advertising experiences across Google services.
Receive more relevant advertisements by sharing your interests and behavior with our trusted advertising partners.
Enables better ad targeting and measurement on Meta platforms, making ads you see more relevant.
Allows for improved ad effectiveness and measurement through Meta’s Conversions API, ensuring privacy-compliant data sharing.
LinkedIn Insights
Tracks conversions, retargeting, and web analytics for LinkedIn ad campaigns, enhancing ad relevance and performance.
LinkedIn CAPI
Enhances LinkedIn advertising through server-side event tracking, offering more accurate measurement and personalization.
Google Ads Tag
Tracks ad performance and user engagement, helping deliver ads that are most useful to you.
Share Knowledge, Get Respect!
or copy link
Cite according to academic standards
Simply copy and paste the text below into your bibliographic reference list, onto your blog, or anywhere else. You can also just hyperlink to this page.
New to UX Design? We’re Giving You a Free ebook!
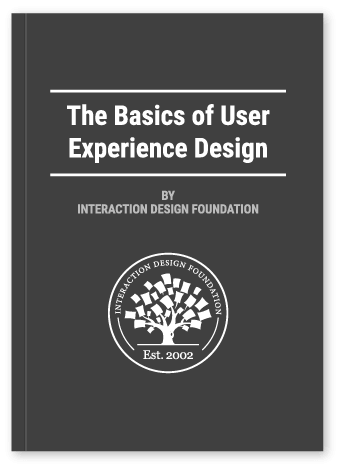
Download our free ebook The Basics of User Experience Design to learn about core concepts of UX design.
In 9 chapters, we’ll cover: conducting user interviews, design thinking, interaction design, mobile UX design, usability, UX research, and many more!
A/B Testing 101
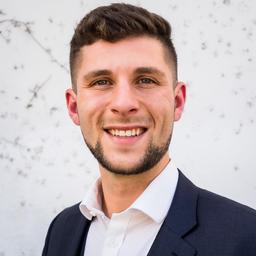
August 30, 2024 2024-08-30
- Email article
- Share on LinkedIn
- Share on Twitter
A/B testing (sometimes also referred to as split testing) is a popular UX research method, with widespread adoption across businesses and industries. To ensure reliable, meaningful, and beneficial results for your organization, follow best practices and avoid common mistakes when planning and setting up an A/B test.
In This Article:
What is a/b testing, why conduct an a/b test, 4 steps for setting up an a/b test, limitations and common mistakes in a/b testing.
A/B testing is a quantitative research method that tests two or more design variations with a live audience to determine which variation performs best according to a predetermined set of business-success metrics.
In an A/B test, you create two or more variations of a design in a live product. Most commonly, you’ll compare the original design A, also called the control version, and one variation B, called the variant. Ideally, the variant should differ from the original design only in one design element alone, such as a button, an image, or a description.
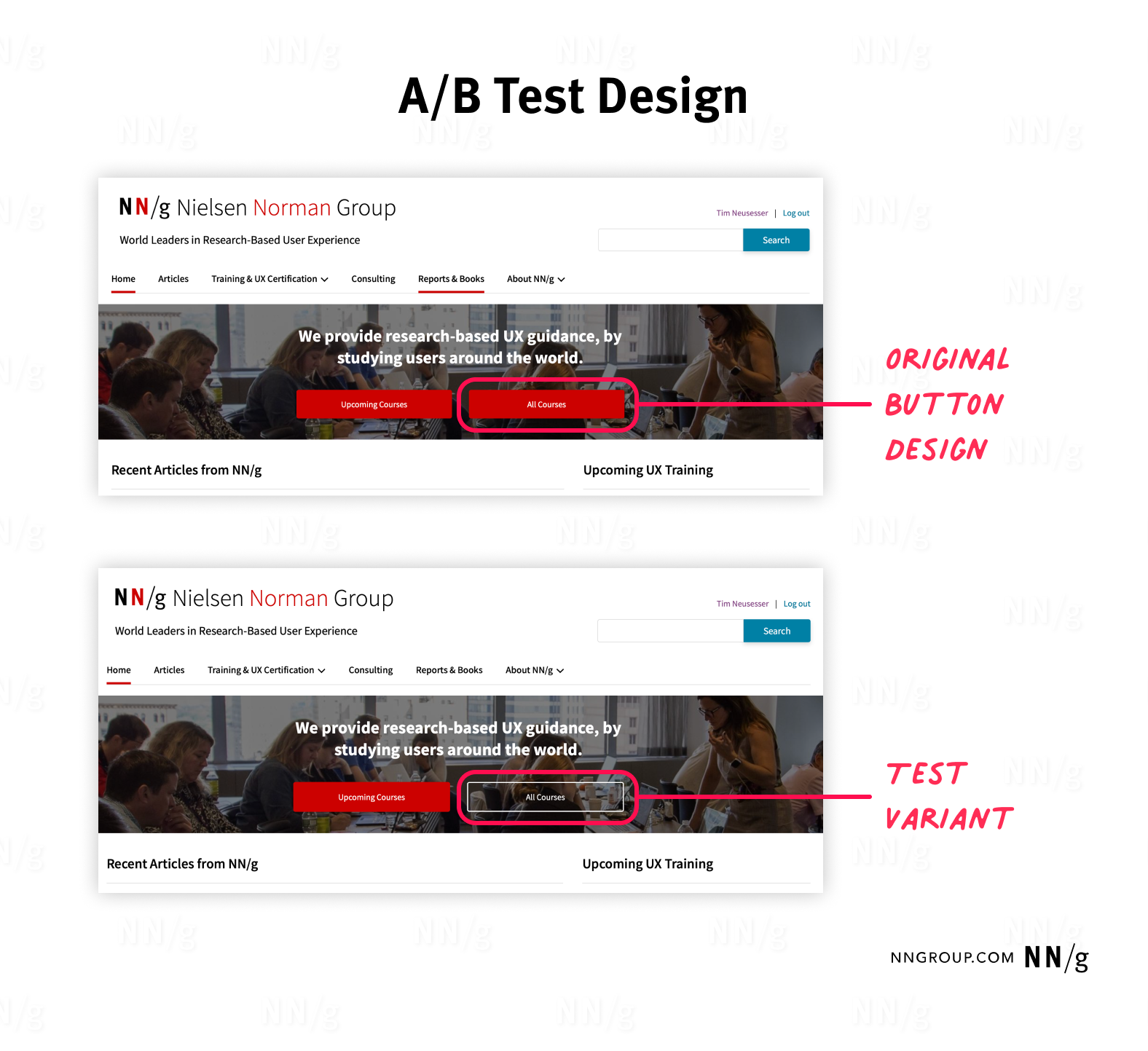
During the A/B test, the incoming traffic of real users to your product is split, so that each visitor will be directed to only one of your design variations. This split of traffic can be such that each variation receives the same share of traffic, or it can be adjusted based on business objectives and risk. (For example, if testing a design variation on half of your site’s traffic would bear too much risk.)
Once the traffic is split to your design variations, you collect a set of metrics to determine which design variation encourages desired user behaviors and thereby better supports your business objectives.
In many cases (but not all), if the variant statistically significantly outperforms the original design, it should be used as the new design of the product. If the test was inconclusive or if your original design outperformed the variation, you should keep the original design. In this case, consider whether testing another design variation might yield better results.
A/B testing can help UX teams determine the improvements in the user experience that are best for their business goals . Additionally, it enables them to make data-driven design decisions, which can result in a high return on investment and tend to be easier to communicate to stakeholders than insights from qualitative studies .
A/B testing is also an efficient method for continuous design improvements, as you can incrementally improve the usability and effectiveness of your product without extensive overhauls .
Common Use Cases
A/B testing requires unambiguous metrics that clearly showcase whether a design variation outperforms the original design. These metrics often focus on monetary aspects, such as revenue or costs. Metrics commonly used in A/B testing include conversion rate, click-through rate, bounce rate, retention rate, and revenue per user.
Industries and products where A/B testing is frequently used and where it can have a significant impact and a high return on investment include:
- Ecommerce (e.g., Amazon)
- Entertainment products (e.g., Netflix, Spotify)
- Social media (e.g., Facebook, Instagram, TikTok)
- Software as a service (e.g., Salesforce, Office365)
- Online publishing (e.g., The New York Times)
- Email marketing
Design elements these industries most commonly test include:
- Call-to-action buttons
- Page layouts
- Website copy
- Checkout pages
- Forms
Following the 4 steps outlined below will increase the likelihood of conducting a test that is reliable, meaningful, and that yields a positive result.
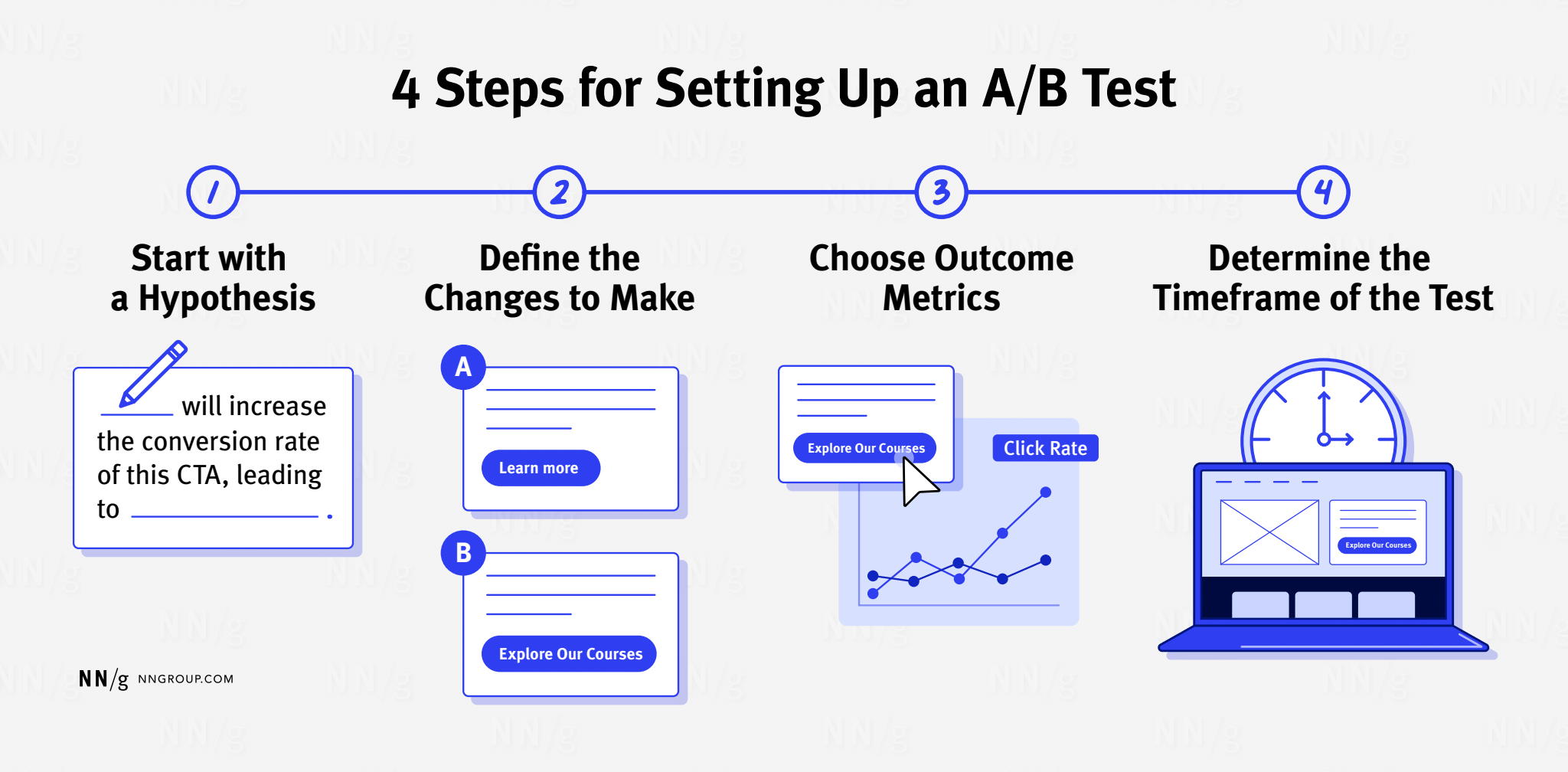
1. Start with a Hypothesis
Before getting started on an A/B test, you should come up with a hypothesis for which changes might have which impact. As stated above, the more this hypothesis is based on user research and business insights, the higher the likelihood that your A/B test will be successful and meaningful. Your hypothesis should be directly connected to a clearly defined goal that you want to accomplish with your A/B test.
Example: You run an ecommerce site. You observed in qualitative usability tests that multiple participants disregarded a call-to-action (CTA) button with the label Purchase . Your hypothesis is that a design change of this page will increase the conversion rate of this CTA, eventually leading to higher sales.
2. Define the Changes to Make
Once you have a strong hypothesis, you must decide which changes to make to which design element to test your hypothesis. These changes should address just one design element and not be an extensive design overhaul. Again, the more this decision is based on insights from user research, the higher the chances that your test will be successful, as these insights will positively impact your ideation process .
Example: Based on your insights from qualitative usability testing, you decide to change the label of the CTA button. During the tests, you observed that participants noticed the button but were unsure about its message. So, you keep the button’s visual design but change the label to Buy Now .
3. Choose Outcome Metrics
Clearly define which metrics you want to track to determine the impact and success of your A/B test. You should define primary metrics , which will tell you if the design variation results in the hoped-for change in behavior. Additionally, you should define and track guardrail metrics , to determine if the change in user behavior truly has a positive impact on the business.
Example: To understand if changing your CTA label to Buy Now results in an increase of sales, you decide to track the CTA’s click rate. Additionally, you will also track the purchase rate and the average sale amount per purchase. These guardrail metrics help you determine whether a higher click rate of your design variation will have a positive business impact.
4. Determine the Timeframe of the Test
Once you have a strong hypothesis and define the changes to make and metrics to track, you must decide for how long to run your A/B test . This parameter depends on the required sample size for your test.
To determine the required sample size for your test , you must define three numbers:
- Baseline outcome-metric value: The outcome metric (e.g., conversion rate, click rate) for your design
- Minimum detectable effect: The minimum relative change in the outcome metric that you want to be able to detect
- Statistical-significance threshold (usually 95% ): The degree of certainty you want to have that your test result is reliable
Once you define these three metrics, you can use a sample-size calculator to determine the required sample size for your A/B test. Even with sufficient traffic, we recommend to run your A/B test for at least 1-2 weeks to account for potential fluctuations in user behavior.
Example: Using your analytics data, you determine that the baseline click rate of your Purchase CTA is 3%. You decide that your minimum detectable effect should be 20% (in other words, you want to be able to detect a change as small as 20% of the 3% baseline click rate, which amounts to a click rate of 3%+/- 0.6% for the variation) and your test should have a statistical significance of 95% (p=0.05) . Using a sample-size calculator, you determine that your required sample size is 13,000 users. With an average of 1,000 daily users on your website, you decide to run your A/B test for 14 days, ensuring a large enough sample size and a long enough timeframe for potential fluctuations in user behavior.
Note that, to choose the minimum detectable effect, you should ask yourself which change in the outcome metric will amount to an effect that is practically significant for your business and worth the cost of the change. In our example, a change of 1% would mean being able to detect whether the click rate is just 0.03% bigger than the current one. To reach statistical significance for such a small difference a much larger sample size would be required, and the 0.03% change may have very little impact and may not be worth pursuing.
Choose Your A/B Testing Tool
If you’ve decided that you want to add A/B testing to your arsenal of research methods, you must choose which tool to use. There is a wide range of offerings, and the ideal tool will be highly contextual, varying based on your unique situation and needs. When selecting the right tool for your A/B testing efforts, consider the following factors:
- Budget: A/B testing tools can range from free to costing multiple thousand dollars per month.
- Complexity of your test(s): Consider how easy or complex the design variations you want to test are. Different tools allow for different levels of complexity, ranging from allowing only simple changes of color or copy to more complex changes to page layouts or allowing multivariate testing and split URL testing.
- Ease of use: Ensure that you and your team are comfortable enough with learning the new tool and that you have sufficient time to allocate to this task.
- Technical requirements: Ensure that the tool integrates seamlessly with your organization's technical infrastructure and consider how much engineering time is required to create the test.
Once you select a tool that seems to fit your needs and requirements you should test the tool before running your first A/B test. The test will help you ensure that the A/B tool is set up correctly and works as intended. One common way to do so is an A/A test, where you create a variant that is exactly the same as your original design.
Testing two identical designs against each other should result in an inconclusive test result. If this is not the case, you can check what might have caused the differences in the test result. This approach helps minimize mistakes and implementation errors and ensures your A/B testing tool is set up correctly before you run the first A/B test.
Just as with any other research method, A/B testing has its limitations. It can provide great value in certain situations and when applied correctly, but it can also be a waste of resources and potentially harmful if applied incorrectly.
Limitations
A/B testing is not suited for:
- Low-traffic pages : To reach a point where an A/B test results in a statistically significant difference between two variations of a design, you often need thousands of users interacting with your product. This makes A/B testing a bad fit for pages with little traffic.
- Testing multiple changes at the same time: A/B testing should not be used to test design variations that change multiple design elements at the same time. While this is technically possible, you will lack an understanding of the impact of each individual change. To test multiple changes at a time, use multivariate testing instead, but be aware that these tests require even more data points to lead to reliable results.
- Understanding why user behavior changed: Similarly to other quantitative research methods, A/B testing is great at providing insights into how user behavior changes but will not provide any insights into why these changes occur. Thus, A/B testing provides the most benefit when it is combined with qualitative research methods. This practice is called triangulation .
Common Mistakes in A/B Testing
Disregarding the limitations of A/B testing and not following best practices can lead to misleading, potentially harmful outcomes. Some of the most common mistakes you should avoid are:
- Missing clearly defined goals: You must have clearly defined goals for the hoped-for outcome of an A/B test. These goals will align your team to understand why the test is conducted, provide guidance in creating design variations, and help build a roadmap for A/B testing based on the expected return on investment of each potential test you could run on your product.
- Stopping the test too early: A/B tests that lack sufficient data points will return unreliable results. Yet, some teams make the mistake of monitoring A/B tests in real time and drawing conclusions too early. To get statistically reliable results, you must wait until the appropriate sample size of a test is reached. Only then you should draw a conclusion and end your A/B test.
- Testing without a strong hypothesis: Only one in every seven A/B tests is a winning test . And this rate will likely be even lower if you’re testing design elements without having a strong, data-based hypothesis. Just as with prototypes or extensive redesigns, the more insights you gain from user research , the higher the chances of a successful project outcome.
- Focusing on a single metric : The goal of an A/B rest is often to increase or decrease a certain metric. However, if you measure only one metric to determine whether your test is successful, you might disregard important information that can tell you if a design change is truly beneficial for your organization. For example, if you use a deceptive pattern in your design variation, you might positively impact one metric, such as a conversion rate, but might inadvertently negatively affect other metrics, such as retention rate. This is why you should track more than one metric, including guardrail metrics, which can give insight into the true impact of your design variation.
- Disregarding qualitative research and business context: Just because an A/B test yields a statistically significant result, it doesn’t mean that you should follow it blindly. After all, the A/B test might return a false positive or false negative , you might introduce a measurement error , or your result might be statistically significant but not practically significant . Thus, you must combine the results from your A/B test with your expert knowledge of your users and your organization to draw the right conclusions.
Related Courses
Analytics and user experience.
Study your users’ real-life behaviors and make data-informed design decisions
Statistics for UX
Calculate, interpret, and report the numbers from your quantitative UX studies
Measuring UX and ROI
Use metrics from quantitative research to demonstrate value
Related Topics
- Analytics & Metrics Analytics & Metrics
Learn More:
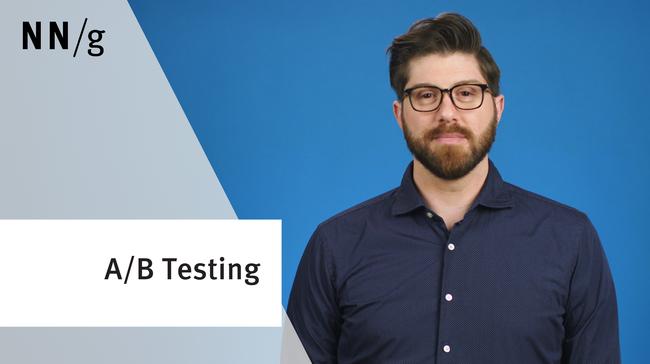
A/B Testing Roadmap
Page Laubheimer · 4 min
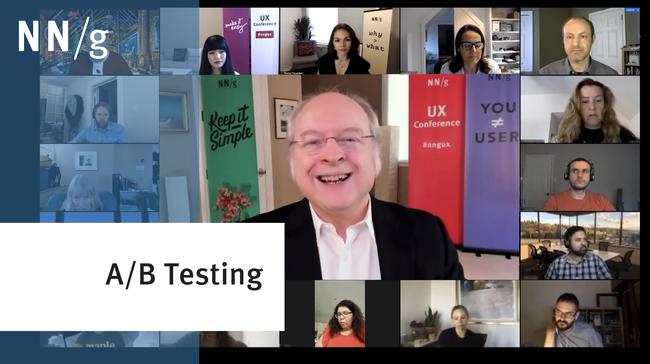
Is A/B Testing Faster than Usability Testing at Getting Results?
Jakob Nielsen · 6 min
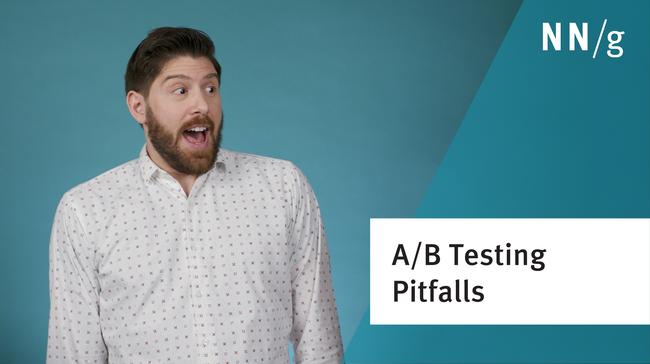
Don't A/B Test Yourself Off a Cliff
Related Articles:
Net Promoter Score: What a Customer-Relations Metric Can Tell You About Your User Experience
Therese Fessenden · 8 min
Multivariate vs. A/B Testing: Incremental vs. Radical Changes
Aurora Harley · 5 min
Define Stronger A/B Test Variations Through UX Research
Jen Cardello · 5 min
Putting A/B Testing in Its Place
Jakob Nielsen · 7 min
Cookie Permissions 101
Samhita Tankala · 9 min
Confounding Variables in Quantitative Studies
Caleb Sponheim · 5 min
Are you an agency specialized in UX, digital marketing, or growth? Join our Partner Program
Learn / Guides / A/B testing guide
Back to guides
The A/B testing handbook: understanding the basics and optimizing results
To build a website your customers love, you need to understand which features give them a thrill—and which put them to sleep. A/B testing takes the guesswork out of engaging with users, providing real insights to help you identify and optimize your website’s best features.
Last updated
Reading time.
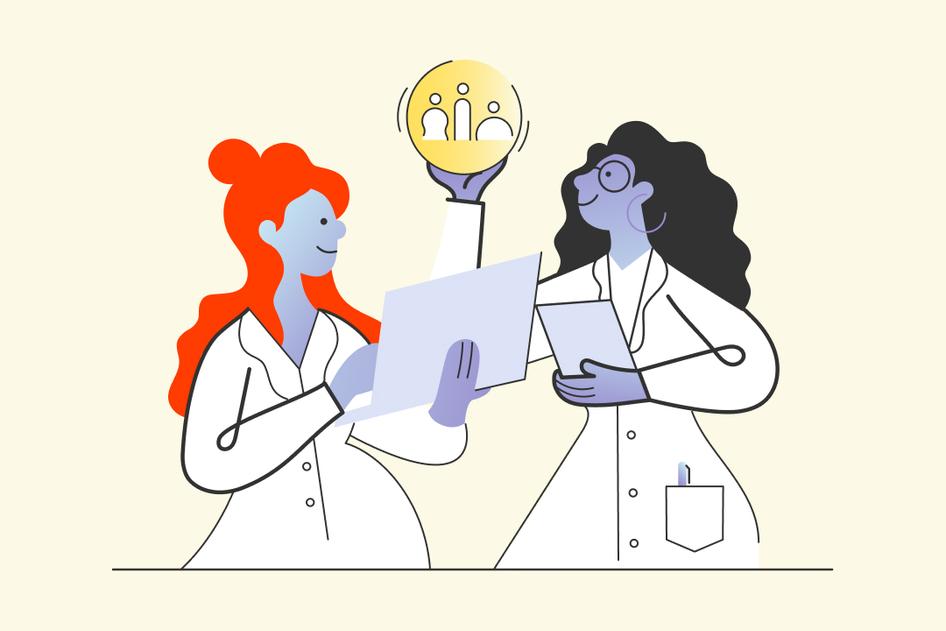
When you run A/B tests regularly, you can measure your team’s theories against real-world data and develop better products for your customers—a win-win situation!
This guide covers the fundamentals of A/B testing on websites and why they're important for you, your business, and the people who matter most: your users. As you go through each chapter, you’ll be able to develop, evaluate, and optimize your A/B tests, and make sure every change produces positive results.
Get the most out of your A/B tests
Hotjar’s digital experience insights help you understand A/B testing results and use them to optimize the user experience.
What is A/B testing?
A/B testing is a research method that runs two versions of a website, app, product, or feature to determine which performs the best. It’s a component of conversion rate optimization (CRO) that you can use to gather both qualitative and quantitative user insights.
An A/B or split test is essentially an experiment where two test variants are shown to users at random, and statistical analysis is used to determine which variation performs better for a given conversion goal.
In its simplest form, an A/B test validates (or invalidates) ideas and hypotheses. Running this type of test lets you ask focused questions about changes to your website, and then collect data about the impact of those changes.
By testing your assumptions with real users, you save a ton of time and energy that would otherwise have been spent on iterations and optimizations.
What A/B testing won’t show you
An A/B test compares the performance of two items or variations against one another. On its own, A/B testing only shows you which variation is winning, not why .
To design better experiments and impactful website changes, you need to understand the deeper motivations behind user behavior and avoid ineffective solutions like guesswork, chance, or stakeholder opinions.
Combining test results with qualitative insights—the kind you get from heatmap, session recording, survey, and feedback tools—is a ‘best of both worlds’ approach that allows you and your team to optimize your website for user experience and business goals.
Why is A/B testing important?
A/B testing is a systematic method used to determine what works (and what doesn’t) for any given asset on your website—a landing page, a logo, a color choice, a feature update, the entire user interface (UI) , or your product messaging.
Whether you're using split tests in marketing campaigns and advertising or A/B testing for product management and development, a structured testing program makes your optimization efforts more effective by pinpointing crucial problem areas that need improvement .
Through this process, your website achieves its intended purpose—to drive maximum engagement or revenue—along with other valuable benefits:
A better user experience : performing A/B tests highlights the features and elements that have the most impact on user experience (UX). This knowledge helps your team develop ideas and make customer-centric, data-informed UX design decisions.
Improved customer satisfaction : gaining insights into what users enjoy helps you deliver a website and product that customers love. Whatever their goal is on your site, A/B testing helps address users' pain points and reduce friction for a more satisfying experience.
Low-risk optimizations : A/B testing lets you target your resources for maximum output with minimal modifications. Launching an A/B test can help you understand whether a new change you’re suggesting will please your target audience, making a positive outcome more certain.
Increased user engagement : A/B testing removes the guesswork from CRO by directly engaging and involving users in the decision process. It lets the users decide—they vote with their actions, clicks, and conversions.
Less reliance on guesswork : A/B tests challenge assumptions and help businesses make decisions based on data rather than on gut feelings—about everything from email subject lines and website information architecture to a complete website redesign
Increased conversion rates : by learning how and why certain elements of the experience impact user behavior, A/B testing lets you maximize your existing traffic and helps you increase conversions
Optimized website performance : A/B testing lets you make minor, incremental changes that significantly impact website performance—like testing APIs, microservices, clusters, and architecture designs to improve reliability
Validated decision-making : use A/B testing to validate the impact of new features, performance improvements, or backend changes before rolling them out to your users
Keep in mind : A/B testing starts with an idea or hypothesis based on user insights .
Hotjar is a digital experience insights platform designed to give you actionable user data so you can spot common areas of friction in the user experience, get ideas for improvements, and find out if your fixes are working (and why or why not).
These four tools help you collect user insights and understand why winning A/B tests succeed, so you can double down on what works:
Heatmaps : see where users click, scroll, and move their mouse on individual pages
Recordings : view how users browse and interact with your pages across an entire session
Surveys : ask users direct questions about their experience
Feedback : let users tag, rate, and comment on any web page element
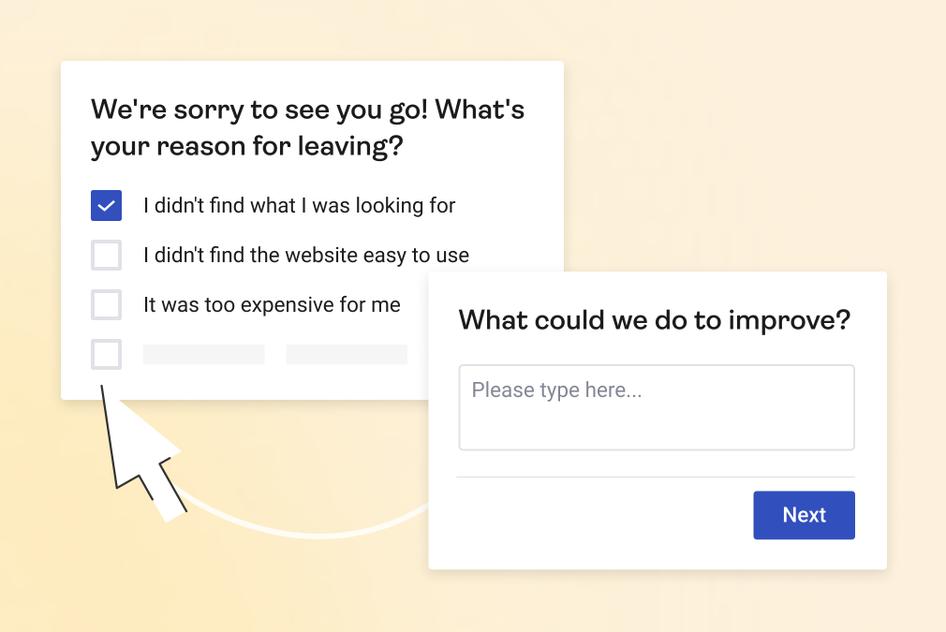
Hotjar Surveys are a way to engage your users in conversation directly
How do you run an A/B test?
Running A/B tests is more than just changing a few colors or moving a few buttons—it’s a process that starts with measuring what’s happening on your website, finding things to improve and build on, testing improvements, and learning what worked for your customers .
Check out our comprehensive guide on how to do A/B testing for your website —everything on developing, evaluating, and optimizing A/B tests. Until then, here’s a quick framework you can use to start running tests:
Developing and setting up A/B tests
From getting a clear understanding of user behavior to connecting problems to opportunities for improvement, the A/B testing process for websites is all about your users .
Researching ideas
A/B testing focuses on experimenting with theories that evolved by studying your market and your customers. Both quantitative and qualitative research help you prepare for the next step in the process: making actionable observations and generating ideas.
Collect data on your website's performance —everything from how many users are coming onto the site and the various conversion goals of different pages to customer satisfaction scores and UX insights .
Use heatmaps to determine where users spend the most time on your pages and analyze their scrolling behavior. Session recording tools also help at this stage by collecting visitor behavior data, which helps identify gaps in the user journey. This can also help you discover problem areas on your website.
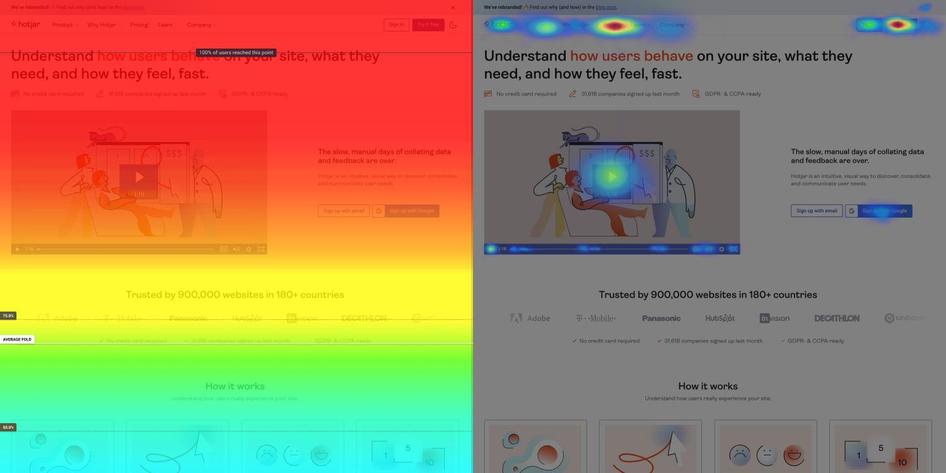
Identifying goals and hypotheses
Before you A/B test anything, you need to identify your conversion goals —the metrics that determine whether or not the variation is more successful than the original version or baseline.
Goals can be anything from clicking a button or link to purchasing a product. Set up your experiment by deciding what variables to compare and how you'll measure their impact.
Once you've identified a goal, you can begin generating evidence-based hypotheses. These identify what aspects of the page or user experience you’d like to optimize and how you think making a change will affect the performance.
Executing the test
Remember, you started the A/B testing process by making a hypothesis about your website users.
Now it’s time to launch your test and gather statistical evidence to accept or reject that claim.
Create a variation based on your hypothesis of what might work from a UX perspective, and A/B test it against the existing version. Use A/B testing software to make the desired changes to an element of your website. This might be changing a CTA button color, swapping the order of elements on the page template, hiding navigation elements, or something entirely custom.
The goal is to gather data showing whether your test hypothesis was correct, incorrect, or inconclusive . It can take a while to achieve a satisfactory result, depending on how big your sample size is.
Important note: ignoring statistical significance is a common A/B testing mistake . Good experiment results will tell you when the results are statistically significant and trustworthy. Otherwise, it would be hard to know if your change truly made an impact.
How to generate A/B testing ideas and hypotheses
Identify where visitors leave your website: use traditional analytics tools to see where people exit your website and complement those insights with Hotjar’s conversion funnels tool
Collect customer feedback : use on-page surveys and feedback widgets to get open-ended feedback about what users really think about your website
Run usability testing : usability testing tools give you insight into how real people use your website and allow you to get their direct feedback about the issues they encounter and the solutions they'd like to see
Study session recordings : observe individual users as they make their way through your website, so you can see the experience from their perspective and notice what they do right before they exit
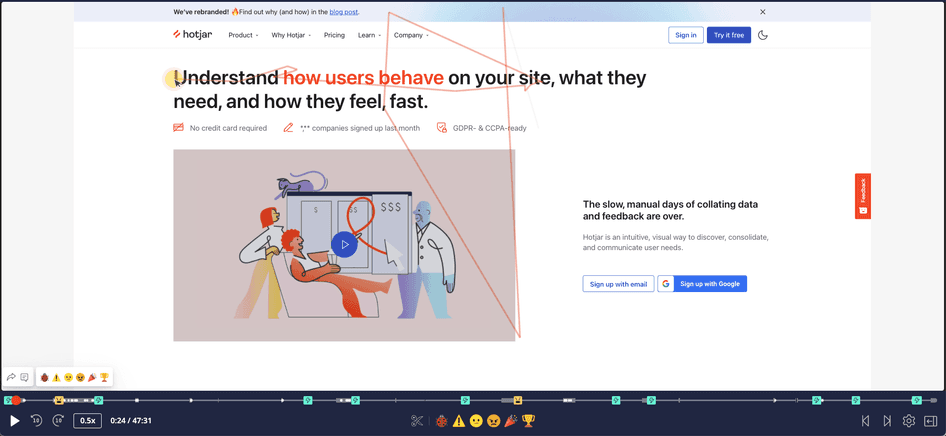
Tracking and evaluating A/B tests
Once your experiment is complete, it’s time to analyze your results. Your A/B testing software will measure data from both versions and present the differences between their performance , indicating how effective the changes were and whether there is a statistically significant improvement.
Analyzing metrics and interpreting results
A/B testing programs live, mature, evolve, and succeed (or fail) through metrics. By measuring the impact of different versions on your metrics, you can ensure that every change to your website produces positive results for your business and your customers.
To measure the impact of your experiments, start by tracking these A/B testing metrics:
Conversion rate: the number of visitors that take a desired action—like completing a purchase or filling out a form
Click-through rate (CTR) : the number of times a user clicks on a link or call to action (CTA) divided by the number of times the element is viewed
Abandonment rate : the percentage of tasks on your website that the customer abandons before being completed—for an ecommerce website, this can mean users purchasing an item in their shopping cart
Retention rate : the percentage of users who return to the same page or website after a certain period
Bounce rate : the percentage of visitors to a website who navigate away from the site after viewing only one page
Scroll depth : the percentage of the page a visitor has seen. For example, if the average scroll depth is 50%, it means that, on average, your website visitors scroll far enough to have seen half of the content on the page.
Time on site : the average amount of time a user spends on your website before leaving
Average order value (AOV) : the average amount of money spent by a customer on a single purchase
Customer satisfaction score (CSAT) : how satisfied customers are with your company's products or services
Note: read our full chapter on A/B testing metrics to make sure you collect the most essential data to analyze your experiment
How to understand the ‘why’ behind your A/B testing results
In A/B testing, quantitative metrics are essential for identifying the best-performing variation of a site or product. But even the best-designed A/B tests can’t pinpoint the exact reasons why one variation succeeds over another. For example, why does one version of a CTA work better than the other? And how can you replicate its success across your website?
Quantitative and qualitative A/B testing metrics should work in harmony: quantitative data answers the what , and qualitative data tells you why .
Tools like Hotjar Heatmaps, Recordings, Surveys, and Feedback integrate with traditional A/B testing tools to help you better understand variant performance.
During a test, you can track user behavior and collect insights to understand how each variation affects the user experience on your website. Then, use these qualitative tools to explore more in-depth ideas, learn about common user pain points, or discover which product features are most interesting to them:
Session Recordings and Heatmaps let you visualize how users react to different variations of a specific page, feature, or iteration. What are visitors drawn to? What confuses them?
Surveys take the guesswork out of understanding user behavior during A/B testing by letting users tell you exactly what they need from or think about each variation
Feedback gives you instant visual insights from real users. They can rate their experience with a variant on a scale, provide context and details, and even screenshot a specific page element before and after you make a change.
Next steps to A/B testing
Like with any CRO process, you’re not only looking for the winning A/B test variation— you need actionable learnings that you can apply to your website to improve the customer experience.
If your variation is a winner, congratulations! 🎉 Deploy it, draw conclusions based on the data, and translate them into practical insights that you can use to improve your website. See if you can apply learnings from the experiment on other pages of your site to continue iterating and enhancing your statistically significant results.
Remember that inconclusive and 'failed' tests give you a clear idea of what doesn't work for your users. There are no losses in A/B testing—only learning.
FAQs about A/B testing
How do i know what to a/b test.
Look for ideas, issues, and opportunities using traditional analytics tools (such as Google Analytics or Mixpanel) to see where visitors leave your website and complement them with product experience insight tools—like Hotjar Heatmaps, Surveys, Recordings, and Feedback—to understand the real user experience and how A/B testing can improve it.
What types of tools and software are there for A/B testing?
A/B testing tools help you formulate ideas and hypotheses, set up and determine statistically significant A/B, split, and multivariate tests, and evaluate the performance of winning variations.
Quantitative A/B testing tools generate numerical data to test ideas and confirm hypotheses about what users need. Qualitative tools integrate with traditional A/B testing tools to give you a deeper understanding of variant performance.
How do I optimize A/B testing?
To keep your A/B testing efforts fruitful in the long run, they should form a cycle that roughly starts and ends with user research.
First, learn how your users interact with your website—including design, specific features, infrastructure changes, CTA buttons, and text—and use a quantitative A/B testing tool to implement testing where you need it most.
Then, fill the gap left by quantitative data with qualitative tools like Hotjar Heatmaps, Surveys, Recordings, and Feedback. This will tell you why your users act the way they do and what you can do about it.
- Business Essentials
- Leadership & Management
- Credential of Leadership, Impact, and Management in Business (CLIMB)
- Entrepreneurship & Innovation
- Digital Transformation
- Finance & Accounting
- Business in Society
- For Organizations
- Support Portal
- Media Coverage
- Founding Donors
- Leadership Team
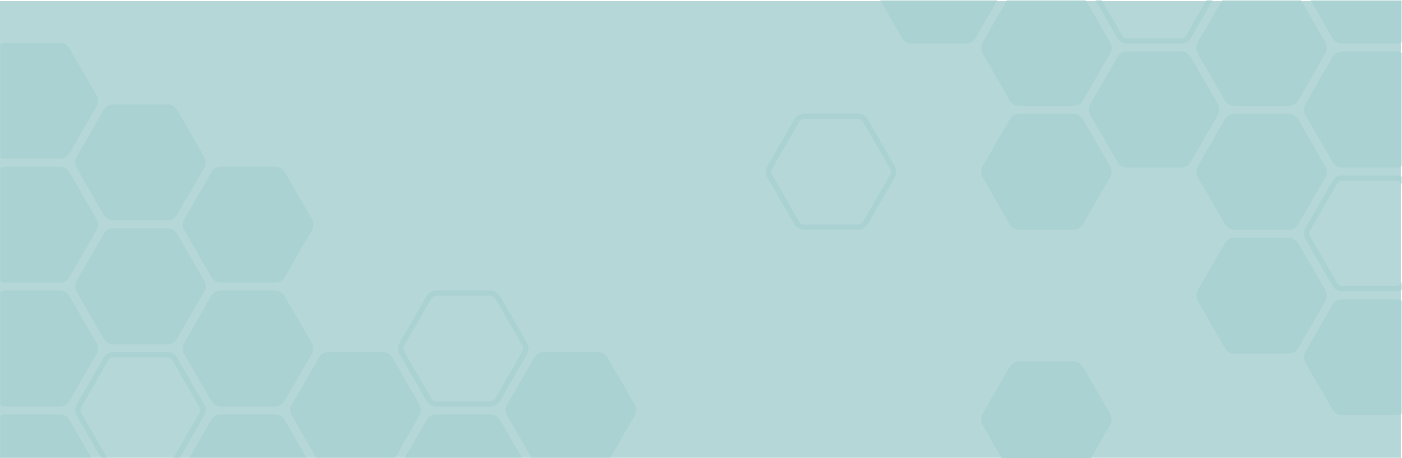
- Harvard Business School →
- HBS Online →
- Business Insights →
Business Insights
Harvard Business School Online's Business Insights Blog provides the career insights you need to achieve your goals and gain confidence in your business skills.
- Career Development
- Communication
- Decision-Making
- Earning Your MBA
- Negotiation
- News & Events
- Productivity
- Staff Spotlight
- Student Profiles
- Work-Life Balance
- AI Essentials for Business
- Alternative Investments
- Business Analytics
- Business Strategy
- Business and Climate Change
- Creating Brand Value
- Design Thinking and Innovation
- Digital Marketing Strategy
- Disruptive Strategy
- Economics for Managers
- Entrepreneurship Essentials
- Financial Accounting
- Global Business
- Launching Tech Ventures
- Leadership Principles
- Leadership, Ethics, and Corporate Accountability
- Leading Change and Organizational Renewal
- Leading with Finance
- Management Essentials
- Negotiation Mastery
- Organizational Leadership
- Power and Influence for Positive Impact
- Strategy Execution
- Sustainable Business Strategy
- Sustainable Investing
- Winning with Digital Platforms
What Is A/B Testing & What Is It Used For?
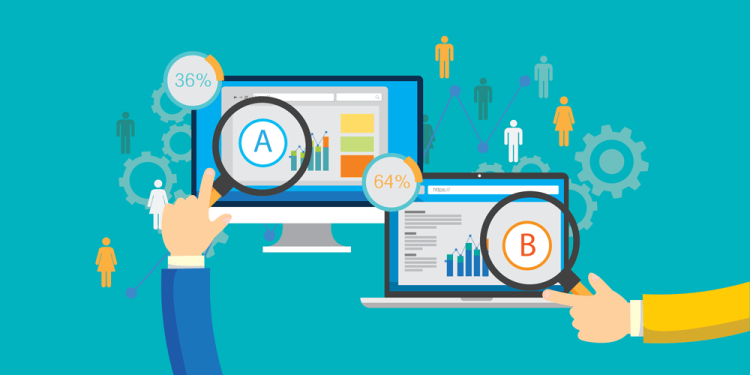
- 15 Dec 2016
You may have experienced meetings where a lot of ideas are circulated about how to improve an existing product or service. In these meetings, differing opinions can quickly turn into a battle of long-winded defenses. Fortunately, the emergence of A/B testing—once thought to be exclusive to tech firms—has become a viable and cost-effective way for all types of businesses to identify and test value-creating ideas.
Related: The Advantages of Data-Driven Decision Making
What Is an A/B test?
In statistical terms, A/B testing is a method of two-sample hypothesis testing. This means comparing the outcomes of two different choices (A and B) by running a controlled mini-experiment. This method is also sometimes referred to as split testing .
What Is A/B Testing Used For?
A/B testing is often discussed in the context of user experience (UX), conversion rate optimization (CRO), and other marketing and technology-focused applications; however, it can be valuable in other situations as well.
Although the concept of A/B testing was galvanized by Silicon Valley giants, the rationale behind A/B testing isn’t new. The practice borrows from traditional randomized control trials to create smaller, more scalable experiments.
For this reason, professionals also perform A/B testing to gather valuable insights and guide important business decisions, such as determining which product features are most important to consumers.
A/B testing is a popular method of experimentation in the fields of digital marketing and web design. For example, a marketer looking to increase e-commerce sales may run an experiment to determine whether the location of the “buy now” button on the product page impacts a particular product’s number of sales.
In this scenario, version A of the product page might feature the button in the top-right corner of the page while version B places the button in the bottom-right corner. With all other variables held constant, randomly selected users interact with the page. Afterward, the marketer can analyze the results to determine which button location resulted in the greatest percentage of sales.

An Example of A/B Testing
As a basic example, let’s say you’re an abstract artist. You’re confident in your technique but aren’t sure how the outside world—and, more importantly, art critics—will respond to your new paintings. Assessing the quality of art is a famously challenging process.
To employ A/B testing for this scenario, start by creating two different paintings that are alike. As you paint both pieces, change one small thing—for instance, add a red square to one painting and not the other. Again, this means that everything about the paintings is alike except for this one modification. Once the change is made, display the two paintings in randomly selected art galleries across the country and wait for your art agent, or another unbiased third party, to gather reactions and report back.
After each painting has been placed in a reasonable amount of art galleries, the feedback may reflect that the painting with the red square received significantly more praise, or maybe it didn’t. The hypothetical outcome doesn’t matter. Rather, what matters is that you can be reasonably confident that your change will or will not make the painting better, and you can go on to create better art as a result.

The randomization aspect of this design is explicitly emphasized because randomization is the gold-standard for eliminating biases. Art is a subjective field and evolves over time. So do the preferences and opinions of customers, clients, or coworkers. A/B testing isn’t a static process, and tests can be repeated or complemented if companies believe that findings may not be valid or applicable anymore.
As a final note, it’s imperative that A/B testing design be rigorous to ensure the validity of results. Furthermore, there may be some decisions where internal opinions are more cost-effective or timely.
Making Data-Driven Business Decisions
Companies like Google, Amazon, and Facebook have all used A/B testing to help create more intuitive web layouts or ad campaigns, and firms in all industries can find value in experimentation. Using data-driven decision-making techniques empowers business leaders to confidently pursue opportunities and address challenges facing their firms. Customers benefit and companies can reap measurable monetary returns by catering to market preferences.
Do you want to learn how to apply fundamental quantitative methods to real business problems? Explore Business Analytics and our other analytics courses to find out how you can use data to inform business decisions.
This post was updated on January 12, 2021. It was originally published on December 15, 2016.

About the Author
AB testing template: how to plan and document experiments
Quarter beginnings usually resonate with growth marketers.
Maybe because that’s when they are preparing their tactical plan, establishing their goals, or just because it's the time for them to think more deeply about their strategies. Regardless, it hasn’t been different to our customers; and that’s exactly the time of the year they usually reach us asking for help to plan their AB tests.
These are the most common questions we get:
How to organize your testing hypotheses
How to prioritize test ideas, which metric to use for analyzing tests performance, how to estimate the test duration beforehand, which method to use to analyze the results, how to document the process for future reviews.
Formulating a roadmap is not always enough for efficient split tests. That’s why we came up with this complete guide to help you test your hypotheses and plan your next marketing strategies.
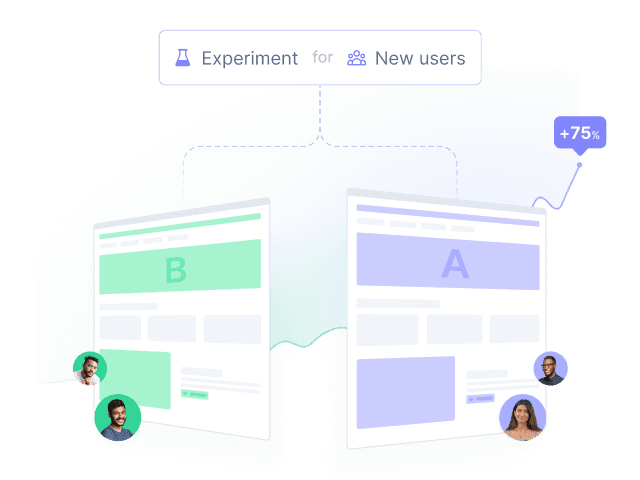
The step-by-step for a good AB testing plan
We've already covered some of those topics in other blog posts. Let's revisit them now.
Listing testing hypothesis requires both data knowledge and creativity, and there's nothing better than brainstorming . Invite all your team members to a 1-hour meeting and let them brood about what should make the conversion rates improve. The more diverse this team is, the better: ask help from designers, copywriters, engineers, data scientists, marketing, and product people.
You should list from 10 to 20 hypotheses to plan your quarter tests.
Example: Donna, a holistic therapist, is trying to get more clients in Singapore, where she lives. These are some ideas she decided to test on her website:
- Discounts for specific services and fidelity campaigns
- First purchase discounts
- A new CTA for limited time to purchase with discounts
- New videos and blog posts for engagement
- New landing pages based on the weather
- Clients testimonials
- Online courses with simple practices users can try at home
- Personalizations based on users’ last sessions
- New heroes with engaging images and copies
- New headlines
If you’re not sure what to begin with, here are some important questions to consider:
- What is the goal of each test?
- What KPIs are you trying to improve?
- What data you have available for the area of improvement?]
- What is the impact of confirming these hypotheses?
- How long will it take to implement each test?
- Who needs to be involved in each task?
If you don’t know how to answer some of these questions, invite your team to collaborate and score the impact, confidence, and ease of the ideas you’ve come up with. You can use the ICE Score method to do that, which we’ll cover in the next section.
Deciding where to start can be one of the most challenging steps. Luckily, a smart method can help you with that: the ICE scoring.
The ICE score model is widely used by growth and product teams to prioritize features and experiments. It helps you evaluate each option by pointing out its impact, your confidence in its potential result, and the ease of implementation. Then, you can rank all options by multiplying these three values to calculate the score.
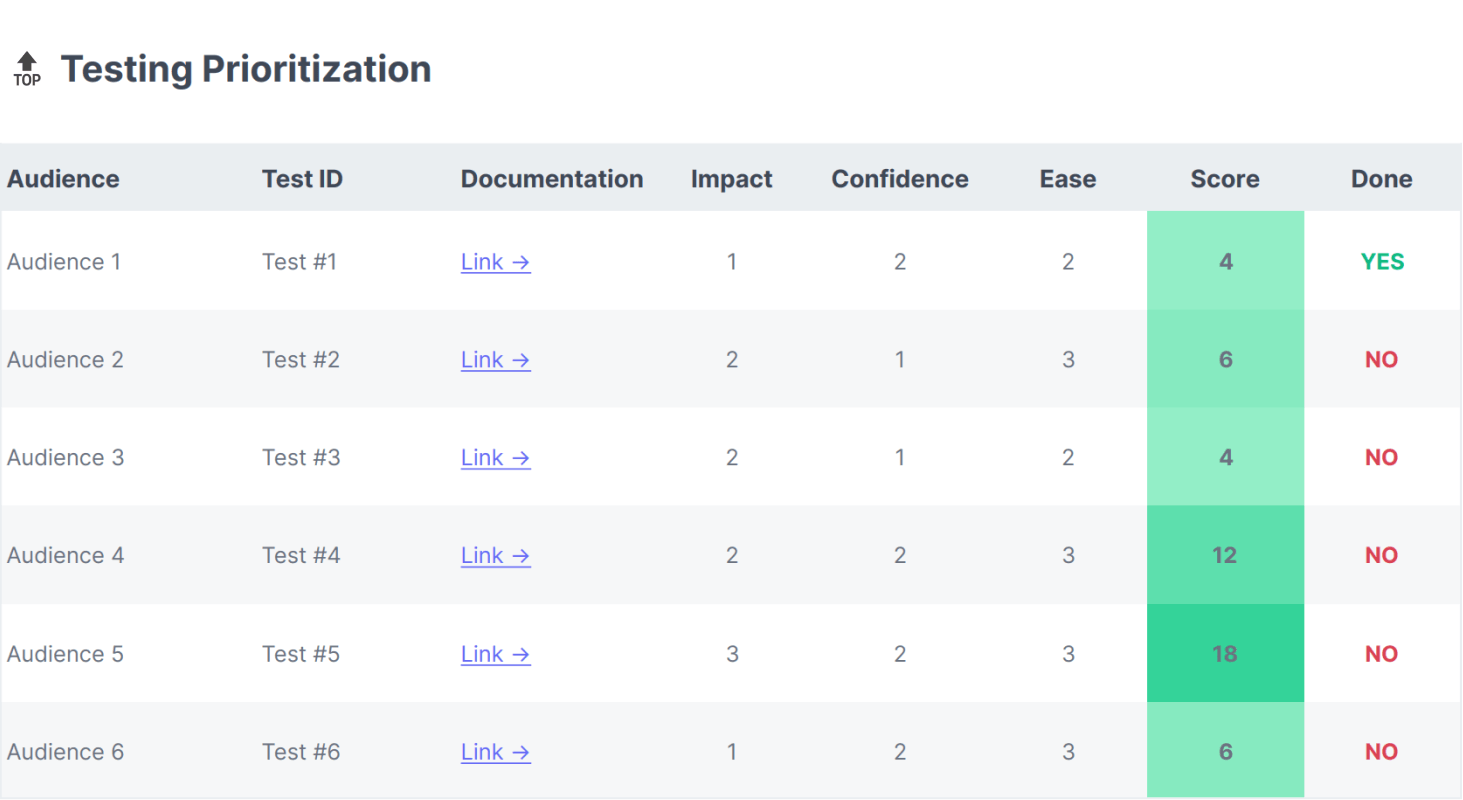
If you wish to know more about how it works, check out this blog post .
If, just like in the table above, you have different audiences you’d like to test, remember to take a step back to “how to organize your testing hypotheses”. Consider the relevance of your targeted audiences and which pain points you can address by testing new hypotheses.
You should also consider:
- What approaches your competitors already validated
- How your ads are performing
- What keywords bring you most traffic
- What trends there are in your industry right now
- Which personas are interacting the most with your product
Collecting existing data for your experiments and implementing it will have a huge impact on your marketing strategies throughout the next months (or years). Remember that prioritizing the right ideas saves you both time and money.
This should be the easiest step. Usually, your primary metric is very straightforward and highly related to your business goal. However, we strongly suggest you define secondary metrics to help you in the analysis: it is not unusual to run experiments that don't impact the primary conversion rate but change the mid-funnel metrics significantly.
The metrics you choose are generally defined by the goals you expect to achieve with your experiment. However, these are common points to pay attention to:
CTR: which specific elements in your test got the most interactions (a button, an image, a new CTA)? Is this change applicable to other slots throughout your website?
CAC and NPS: has the cost of acquiring new customers decreased? Are customers happy with their current experience?
ROI: did you get an equivalent return on investment of both time invested and costs?
AB tests have specific metrics you should analyze to validate hypotheses. But don’t forget to be creative in your analysis and formulate more hypotheses on why an experiment had more interaction, or how your audience would answer to a minor change. This will allow you to continue creating engaging content that resonates with all variations of your winning test.
From a purely statistical perspective, estimating the test duration is easy after determining the sample size. However, you have to take some things into account:
- What is your current conversion rate?
- What is the minimum improvement you expect to detect in your experiment?
- How many variations will the test have?
All these factors can affect the duration. But it is also important to highlight that you will only know it after your test runs. If the impact of the variant over the baseline is too small, you would probably want to run the test for at least a little while to observe statistical confidence.
You can use the calculator we provide in our free template .
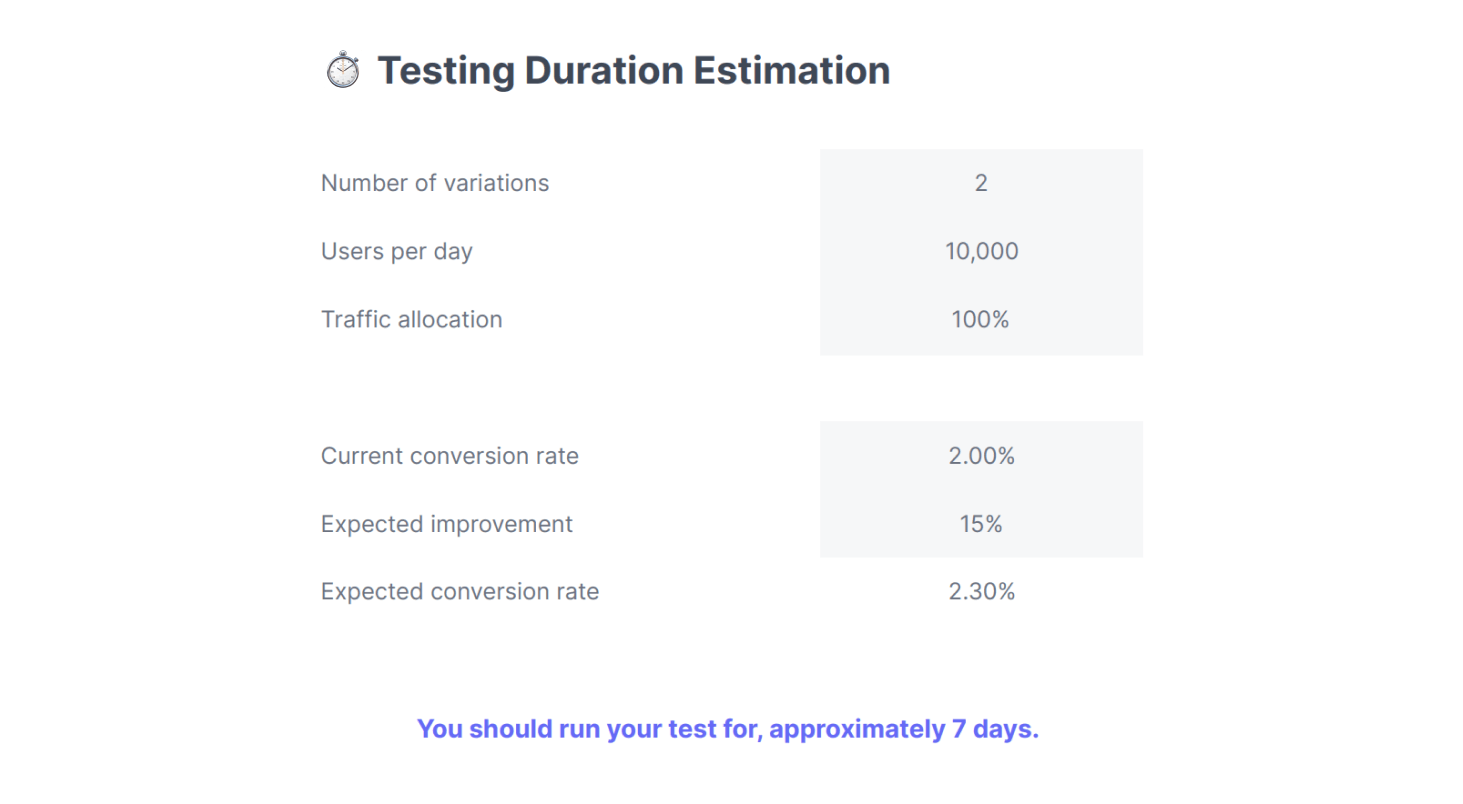
The most used methods are the frequentist and the Bayesian .
The frequentist inference was developed in the 20th century and became the dominant statistical paradigm, widely used in experimental science. It is a statistically sound approach with valid results, but it presents limitations that aren't attractive in AB testing. On the other hand, the Bayesian approach has become the industry standard based on our benchmark, providing richer decision-making information, although the frequentist is still widely used.
Documenting AB tests should be a very straightforward exercise, but many folks dread this aspect of running experiments. It doesn't need to be demanding, so we made a template to help you organize the most critical information. It should guide you on documenting the hypothesis, the target metrics, the results, etc.
A free template guide for you
To help you plan your AB tests, we've designed a free template in a spreadsheet format .
This guide should provide you with:
- A list of ideas to test on your website
- A tool to help you prioritize your experiments using the ICE score
- A calculator to estimate how long you should run your tests
- A template for documenting your experiments
Feel free to download it and share it with your friends if you find it useful!
And if you want to rely on an easy to use platform for creating your tests autonomously and without needing daily help from devs, create your free account and explore Croct.
Learn practical tactics our customers use to grow by 20% or more.
A/B Testing: How to start running perfect experiments and make data-informed decisions
The bigger a company is and the more senior folks are involved, the more people hesitate to experiment. To build a culture of experimentation, you need to rethink your approach. In this article, see more about the science of running perfect experiments and what kind of A/B tests can help you deliver conclusive test results.
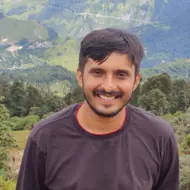
Experimentation is not just about running tests; it's about learning from those tests and driving change. By testing new ideas and approaches, organizations can learn what works and what doesn't, and make data-driven decisions to improve processes, products, and services.
Building a culture of experimentation brings tremendous value to companies. Harvard Business School did a study where they looked at the value testing provided to startups, especially in the ecommerce industry. They found that investors were willing to invest 10 % more dollars into companies that were experimenting than those that weren't.
Similarly, Optimizely’s research found that the media companies using A/B testing reported 9 % more digital revenues. So, we know from the data that when people experiment, they make smarter decisions.
For example, let's say you make 50 product changes by the end of the year, all of which have an effect. Some might be better; some might be worse. If you experiment, you know not to implement the non-performing changes. You're able to roll those back while getting the benefits of changes that worked well.
So, it is helpful to prioritize your tests and play the odds better. However, for folks, it can be a scary prospect because it exposes them to the things that they did wrong. This is where the role of leadership becomes critical.
The Role of Senior Leadership
In some organizations, people may be hesitant to experiment or share their ideas if they feel like they don't have the authority to do so. This can be especially true if senior leadership is not involved in experimentation.
Let's break the myth first. When it comes to experimentation, the highest-paid person's opinion doesn’t always count the most. In fact, giving too much power to the senior leadership can sometimes hinder innovation and creativity, as people may be less willing to take risks or try new things if they feel like they're being micromanaged.
Try creating a culture of experimentation that encourages everyone to participate and contribute their ideas.
So, in pursuit of the greater good for the business and increasing your confidence level, you must be very critical and honest about the things you're doing that are not having an effect. It’ll help your business become more agile and responsive to change, which is critical in today's fast-paced business environment.
The need for well-designed experiments
In school and college, we all saw examples of all these great physicists and classical researchers who ran these beautiful lab experiments. They made one tiny tweak, they measured that with certainty, and even after decades we all benefit from and talk about it in the classrooms.
However, in the business world, people over-analyze the need for experimentation and testing as an idea’s value expires the moment the consumer base shifts and devices become different. If you make a super tiny tweak and it expires in a couple of years, the effect you're having is quite minimal.
For example, someone in your team might think highly about your website’s homepage or landing page. The Call-to-Action (CTA) on the page is trying to drive people to sign up for events or to help them purchase the products, so no point in changing too many things at once.
Because if you change the text and the color and the size of the text and the placement for a test run, it’s hard to know exactly which of these levers made the change.
However, the problem with that type of mentality is it'll work for an organization that's running tens of thousands of experiments and can measure every tweak one by one. But most companies that are running maybe 100 tests a year, need to take big, bold leaps if they want to get somewhere.
Stop holding yourself to this idea that you must be extremely scientifically rigorous, only make very tiny tweaks, and need to be very exact with every test.
Designing a great experiment means exposing yourself to risk, making larger changes, and doing things that move the needle substantially in terms of statistical significance.
The science of a good experiment
Let's say your company decides to add new filters to the product pages as a new feature. An engineer goes out, builds the code to create a filter, and gets ready to implement it on the top of the page. There’s only a single version of that filter. If it fails, we don't know if visitors don't want filters or if the usability of that filter is just poor.
Therefore, great if you want to have a filter, but have different versions of it. You can try it on the top of the page, on the left-hand side, and in other places. You can have it fixed or floating, and even change the order of the filters as well.
The benefit of this experiment is, once you've run this test, let’s say all variants of your filter lose. Now you know conclusively filters are not necessary for your customers. It is time to focus on something else. Or if a version of filters you tried wins, you only implement that quickly. Simply running one filter without any alternatives can lead to misinterpretation of results.
To get the most value from multivariate testing, approach it in a structured and systematic way. It involves:
1. Defining a hypothesis
Before you start experimenting, have a clear idea of what you're testing, your target audience, and what you hope to achieve. Define a hypothesis in your template - a statement that describes what you expect to happen as a result of your experiment.
2. Designing the experiment
Once you have a hypothesis, you need to design an experiment that will test it. It involves identifying the variables you'll be testing, calculating sample size , and determining how to measure the results.
3. Running the experiment
With the experiment designed, it's time to run it. This involves implementing the changes you're testing and collecting quantitative data.
4. Analyzing the results
Once the experiment is complete, it's time to analyze the A/B test results. This involves looking at the data you've collected and determining whether your testing hypothesis was supported or not.

5. Iterating and learning
Use what you've learned from the experiment to iterate and improve your approach. It means using the data to make informed decisions about what to do next and continuing to experiment and learn as you go.
How to start your experimentation journey
Data is critical for measuring the impact of your experiments and making data-driven decisions. It's important to have a clear understanding of the metrics you're using to evaluate success and to measure everything you can to get the most value from your experiments.
Firstly, let’s see what to avoid. When most people start with experimentation, they assume it is about making a simple tweak.
For example, if we the change color from red to blue, this will psychologically trigger the number of visitors to purchase more and increase the conversion rate . And the beauty of a button color test is if it wins, you make money, and if it loses, you lost maybe 15 minutes of your time. It's very easy to run.
But to have a meaningful effect on user behavior, you need to do something very fundamental that's going to affect their experience and deliver a significant result.
For most businesses, experimentation is often very much on the periphery of the decision-making, so it's somebody who's just there to pick the coat of paint on a car that's already been fully designed and assembled. Or it is something driven by senior leadership. VPs and C-level executives are making all the calls, and there's a team on the ground that's merely forced to act out what they're asking for, but then they have the freedom to experiment.
Great experimentation is a marriage of all of these. A place where people have the right to make tweaks. They have the right to be involved in the design of the vehicle itself, and they are a partner to senior leaders in that decision-making process. Senior leaders come with great ideas, and they're allowed to augment them. They're not merely there to execute and measure the ideas of others.
Follow these steps to get going:
1. Start small
Don't try to change everything at once. Instead, start with small experiments that can help you learn and build momentum in real time.
2. Focus on the customer
Experimentation functionality should be focused on delivering value to the customer. Make sure you're testing ideas that will have a real impact on their experience.
3. Measure everything
To get enough data and value from your experiments, it's important to measure everything you can. This means tracking not just the outcomes, but also the process and the baseline metrics you're using to evaluate success.
4. Create a culture of experimentation
Finally, it's important to have an A/B testing tool that uplifts the state of experimentation and innovation. This means giving people the freedom to try new things, rewarding risk-taking, and celebrating successes (and failures) along the way.
Finally...
A testing program is a critical tool for driving digital transformation and Conversion Rate Optimization (CRO). By building a culture of experimentation, organizations can learn what works and what doesn't, and use that knowledge to drive change and deliver a top-notch user experience to their customers.
For a step-by-step guide to digital experimentation, check out the Big Book of Experimentation . It includes best practices for web pages, case studies, and practical tips for success.
About the author
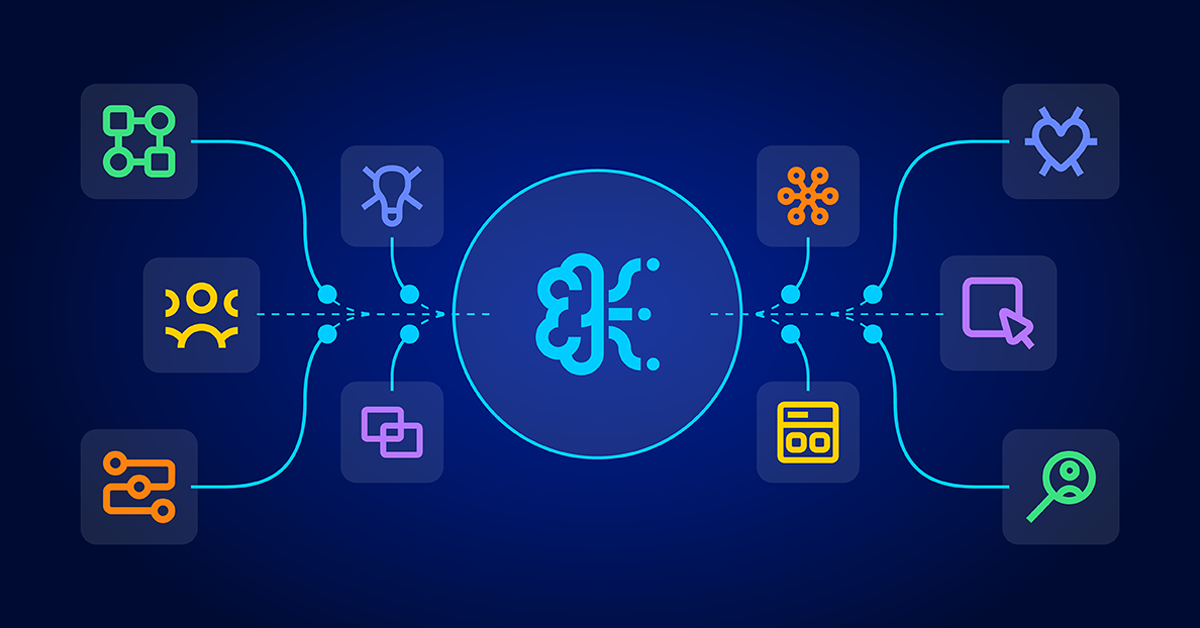
Mastering data-driven decisions: The key to successful product experimentation
- Conversion Optimization
- Growth Marketing
- Digital Analytics
- Brand Marketing
- Digital Marketing
- Digital Psychology
- Ecommerce Marketing
- Product Marketing
- Technical Content Marketing
- Technical Marketing
- Google Analytics 4
- Browse all courses
- CXL Features
- Bottom-of-funnel SEO strategies in tough niches
- Growing AppSumo to 80m with performance marketing
- Account based marketing
- Building a growth process
- Building an innovative product
- Growth mindset: growth vs traditional marketing
- GrowthMaster Training Workshop
- Marketing strategy
- Optimizing Your Growth Process
- Partner Marketing
- Project Management for Marketers
- Retention: the most underrated growth channel
- User-centric marketing
- Data-driven influencer marketing
- Messaging strategy in public relations
- Sales Copywriting & Product Messaging
- Content marketing research
- Content recycling
- Email Marketing: Fundamentals
- Organic Social Media
- Product Marketing Content
- Scaling Content Marketing
- Content strategy and SEO for lead generation
- Growth Focused SEO testing
- On-Page, On-Site & Programmatic SEO
- SEO Link Building
- SEO-Driven Editorial Calendar
- Technical SEO
- Advanced Facebook Ads
- Advanced LinkedIn Ads
- Facebook Ads Creative
- Facebook Ads Experimentation
- Facebook Ads for Beginners
- Google Ads Experiments
- Google Ads for Beginners
- Linkedin Experimentation
- GA4 Intermediate
- Google Analytics 4 for beginners
- Preparing for Your GA4 Implementation
- Special Topics in GTM for GA4
- Attribution
- Data presentation and visualization
- Excel and Sheets for marketers
- Transactional data analysis
- Advanced Google Tag Manager
- Google Tag Manager for Beginners
- The Measurement Matrix
- Advanced Experimentation Masterclass
- CRO Agency masterclass
- Experimentation program management
- Intro to CRO and Experimentation
- Heuristic Evaluation
- Strategic Research for Experimentation
- User research
- Voice of Customer data
- A/B testing foundations
- A/B testing mastery
- CRO for Ecommerce Growth
- Good Practices
- Statistics for A/B testing
- Statistics fundamentals for testing
- Testing Strategies
- Digital psychology & behavioral design
- Intermediate statistics
- Landing Page Optimization
- People & Psychology
- Personalizing for conversion
- Brand strategy
- Positioning
- Radical differentiation
- Integrated Public Relations and SEO
- Storytelling
- Audience building
- Community building
- Community strategy
- Brand tracking 101
- Brand tracking with Momentive
- Customer storytelling and proof
- Segmentation and Persona Research
- Building a marketing agency
- Managing a remote marketing team
- Marketing Management
- Sales and customer success enablement
- Automation with Apps script
- Data collection on the web
- Data extraction
- Mobile Analytics
- Tag managers
- Python for marketers
- R for marketers
- SQL for marketers
- API Applications
- Cloud computing concepts
- Cloud services
- Machine learning applications
- Machine learning fundamentals
- Attention Basics
- Decision Making and Emotions
- Learning and Memory
- Building Habits and Loyalty
- Building Trust
- Cognitive Biases
- Nonconscious Motivation
- Principles of Persuasive Design
- Facebook Ads for ecommerce
- Google Ads for Ecommerce
- Google Shopping
- Selling on Amazon: Perfecting Traffic and Conversions
- Ecommerce Content Marketing
- Ecommerce SEO
- Email and SMS Marketing for Ecommerce
- Customer experience for ecommerce
- Customer journey for ecommerce
- Customer segmentation for ecommerce
- Retention and Customer Lifetime Value
- Ecommerce brand strategy
- Ecommerce merchandising
- Personalization for ecommerce
- Promotional events
- Selling on Marketplaces
- Ecommerce data and metrics
- Ecommerce forecasting
- Ecommerce tech stack
- Unit economics for ecommerce
- Competitive intel & market research
- Introduction to product marketing
- Positioning and company storytelling
- Pricing and packaging
- Product Analytics
- Analyst relations
- Product launches
- Hiring product marketers
- Working with the product team
- What is included in All-access
- First time here? See all resources
- Original research studies
- AB test calculator
- Conversion rate optimization guide
- Conversion optimization guide
- Ecommerce best practices
- Bounce rate guide: The foundations
- Clickthrough rate guide: The foundations
- Follow our B2B strategy podcast
- Sign up now
What is A/B Testing? The Complete Guide: From Beginner to Pro
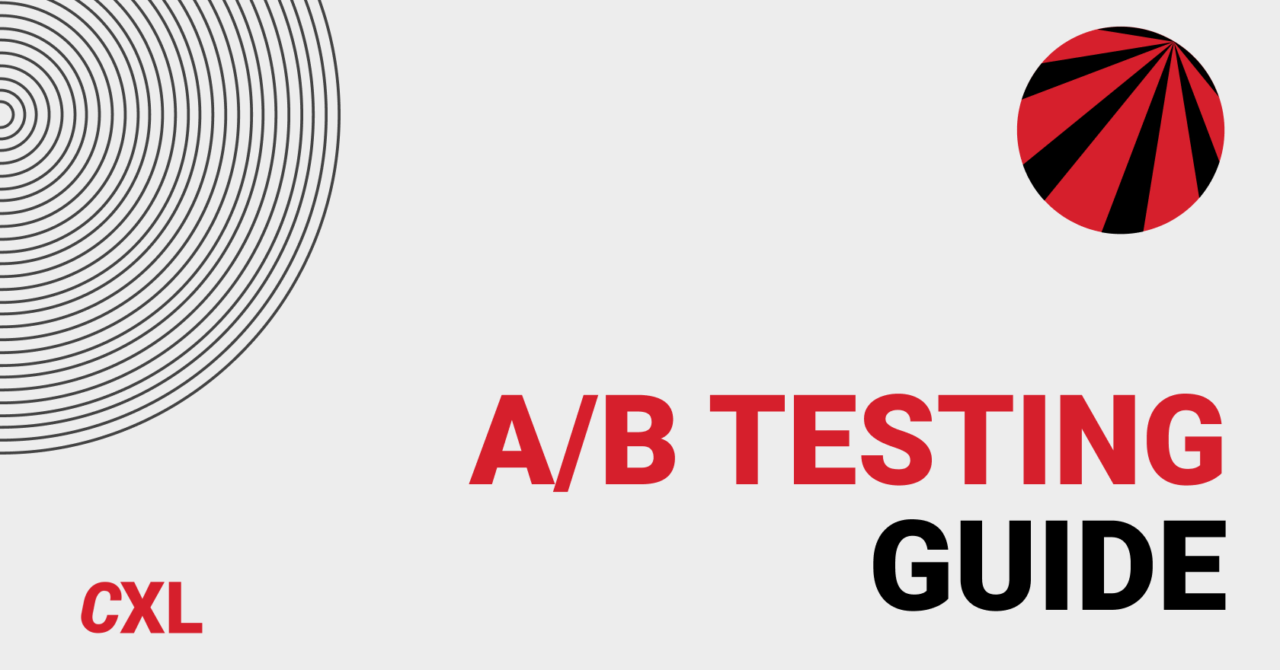
A/B testing splits traffic 50/50 between a control and a variation. A/B split testing is a new term for an old technique — controlled experimentation .
Yet for all the content out there about it, people still test the wrong things and run A/B tests incorrectly .
This guide will help you understand everything you need to get started with A/B testing. You’ll see the best ways to run tests, prioritize hypotheses, analyze results, and the best tools to experiment through A/B testing.
Table of contents
What is a/b testing, how to improve a/b test results, how to prioritize a/b test hypotheses, how long to run a/b tests, how to set up a/b tests, how to analyze a/b test results, how to archive past a/b tests, a/b testing statistics, how to do a/b testing: tools and resources.
A/B testing is a experimentation process where two or more variants (A and B) are compared, in order to determine which variable is more effective.
When researchers test the efficacy of new drugs, they use a “split test.” In fact, most research experiments could be considered a “split test,” complete with a hypothesis , a control, a variation, and a statistically calculated result.
That’s it. For example, if you ran a simple A/B test, it would be a 50/50 traffic split between the original page and a variation:
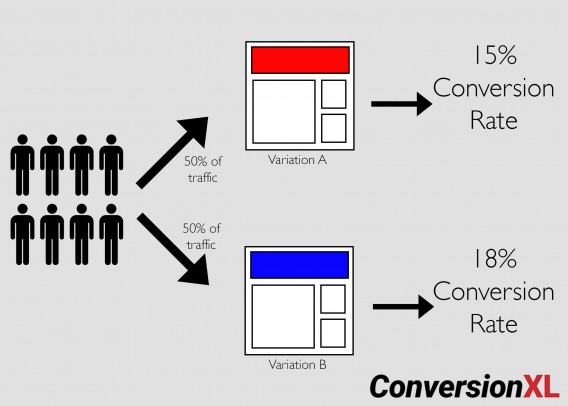
For conversion optimization , the main difference is the variability of Internet traffic. In a lab, it’s easier to control for external variables. Online, you can mitigate them, but it’s difficult to create a purely controlled test.
In addition, testing new drugs requires an almost certain degree of accuracy. Lives are on the line. In technical terms, your period of “exploration” can be much longer, as you want to be damn sure that you don’t commit a Type I error (false positive) .
Online, the process for A/B split-testing considers business goals. It weighs risk vs. reward, exploration vs. exploitation, science vs. business. Therefore, we view results through a different lens and make decisions differently than those running tests in a lab .
You can, of course, create more than two variations. Tests with more than two variations are known as A/B/n tests. If you have enough traffic, you can test as many variations as you like. Here’s an example of an A/B/C/D test, and how much traffic each variation is allocated:
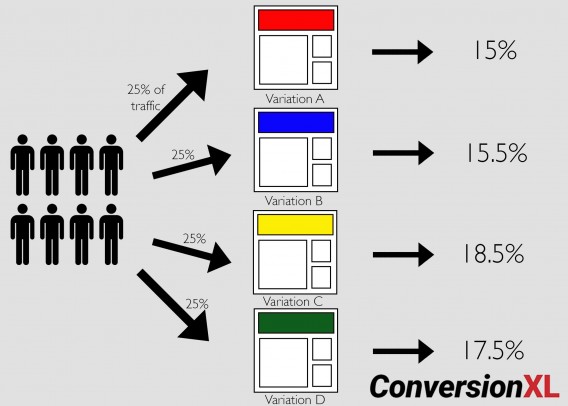
A/B/n tests are great for implementing more variations of the same hypothesis, but they require more traffic because they split it among more pages.
A/B tests, while the most popular , are just one type of online experiment. You can also run multivariate and bandit tests .
A/B Testing, multivariate testing, and bandit algorithms: What’s the Difference?
A/B/n tests are controlled experiments that run one or more variations against the original page. Results compare conversion rates among the variations based on a single change.
Multivariate tests test multiple versions of a page to isolate which attributes cause the largest impact. In other words, multivariate tests are like A/B/n tests in that they test an original against variations, but each variation contains different design elements. For example:
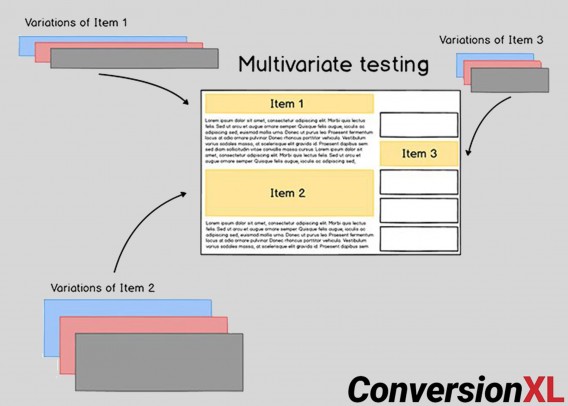
Each element has a specific impact and use case to help you get the most out of your site. Here’s how:
- Use A/B testing to determine the best layouts.
- Use multivariate tests to polish layouts and ensure all elements interact well together.
You need to a ton of traffic to the page you’re testing before even considering multivariate testing. But if you have enough traffic, you should use both types of tests in your optimization program .
Most agencies prioritize A/B testing because you’re usually testing more significant changes (with bigger potential impacts ), and because they’re simpler to run. As Peep once said, “Most top agencies that I’ve talked to about this run ~10 A/B tests for every 1 MVT.”
Bandit algorithms are A/B/n tests that update in real time based on the performance of each variation.
In essence, a bandit algorithm starts by sending traffic to two (or more) pages: the original and the variation(s). Then, to “pull the winning slot machine arm more often,” the algorithm updates based on which variation is “winning.” Eventually, the algorithm fully exploits the best option:
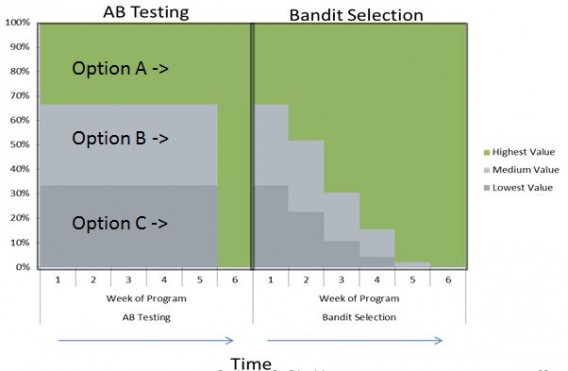
One benefit of bandit testing is that bandits mitigate “regret,” which is the lost conversion opportunity you experience while testing a potentially worse variation. This chart from Google explains that very well:
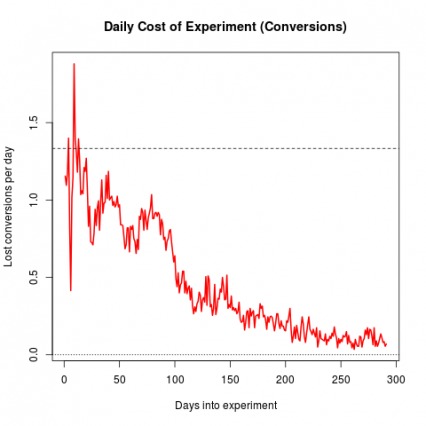
Bandits and A/B/n tests each have a purpose. In general, bandits are great for:
- Headlines and short-term campaigns;
- Automation for scale;
- Blending optimization with attribution .
No matter what type of test you run, it’s important to have a process that improves your chances of success. This means running more tests, winning more tests, and making bigger lifts.
Ignore blog posts that tell you “99 Things You Can A/B Test Right Now.” They’re a waste of time and traffic. A process will make you more money.
Some 74% of optimizers with a structured approach to conversion also claim improved sales. Those without a structured approach stay in what Craig Sullivan calls the “Trough of Disillusionment.” (Unless their results are littered with false positives, which we’ll get into later.)
To simplify a winning process, the structure goes something like this:
- Prioritization;
- Experimentation;
- Analyze, learn, repeat.
Research: Getting data-driven insights
To begin optimization, you need to know what your users are doing and why.
Before you think about optimization and testing, however, solidify your high-level strategy and move down from there. So, think in this order:
- Define your business objectives.
- Define your website goals.
- Define your Key Performance Indicators .
- Define your target metrics.

Once you know where you want to go, you can collect the data necessary to get there. To do this, we recommend the ResearchXL Framework .
Here’s the executive summary of the process we use at CXL:
- Heuristic analysis ;
- Technical analysis;
- Web analytics analysis ;
- Mouse-tracking analysis ;
- Qualitative surveys ;
- User testing and copy testing .
Heuristic analysis is about as close as we get to “best practices.” Even after years of experience, you still can’t tell exactly what will work. But you can identify opportunity areas. As Craig Sullivan puts it :
My experience in observing and fixing things: These patterns do make me a better diagnostician, but they don’t function as truths—they guide and inform my work, but they don’t provide guarantees. Craig Sullivan
Humility is crucial. It also helps to have a framework. When doing heuristic analysis, we assess each page based on the following:
- Distraction.
Technical analysis is an often-overlooked area. Bugs—if they’re around—are a conversion killer. You may think your site works perfectly in terms of user experience and functionality. But does it work equally well with every browser and device? Probably not.
This is a low-hanging—and highly profitable—fruit. So, start by:
- Conducting cross-browser and cross-device testing.
- Doing a speed analysis.
Web analytics analysis is next. First thing’s first: Make sure everything is working. (You’d be surprised by how many analytics setups are broken.)
Google Analytics (and other analytics setups) are a course in themselves, so I’ll leave you with some helpful links:
- Google Analytics 101: How to Set Up Google Analytics ;
- Google Analytics 102: How To Set Up Goals, Segments & Events in Google Analytics .
Next is mouse-tracking analysis , which includes heat maps, scroll maps, click maps , form analytics , and user session replays . Don’t get carried away with pretty visualizations of click maps. Make sure you’re informing your larger goals with this step.
Qualitative research tells you the why that quantitative analysis misses. Many people think that qualitative analysis is “softer” or easier than quantitative, but it should be just as rigorous and can provide insights as important as those from analytics.
For qualitative research , use things like:
- On-site surveys ;
- Customer surveys;
- Customer interviews and focus groups.
Finally there’s user testing . The premise is simple: Observe how actual people use and interact with your website while they narrate their thought process aloud. Pay attention to what they say and what they experience.
With copy testing , you learn how your actual target audience perceives the copy, what clear or unclear, what arguments they care about or not.
After thorough conversion research, you’ll have lots of data. The next step is to prioritize that data for testing.
There are many frameworks to prioritize your A/B tests, and you could even innovate with your own formula. Here’s a way to prioritize work shared by Craig Sullivan .
Once you go through all six steps, you will find issues—some severe, some minor. Allocate every finding into one of five buckets:
- Test. This bucket is where you place stuff for testing.
- Instrument. This can involve fixing, adding, or improving tag/event handling in analytics.
- Hypothesize. This is where you’ve found a page, widget, or process that’s not working well but doesn’t reveal a clear solution.
- Just Do It. Here’s the bucket for no-brainers. Just do it.
- Investigate. If an item is in this bucket, you need to ask questions or dig deeper.
Rank each issue from 1 to 5 stars (1 = minor, 5 = critical). There are two criteria that are more important than others when giving a score:
- Ease of implementation (time/complexity/risk). Sometimes, data tells you to build a feature that will take months to develop. Don’t start there.
- Opportunity. Score issues subjectively based on how big a lift or change they may generate.
Create a spreadsheet with all of your data. You’ll have a prioritized testing roadmap.
We created our own prioritization model to weed out subjectivity (as possible). It’s predicated on the need to bring data to the table. It’s called PXL and looks like this:
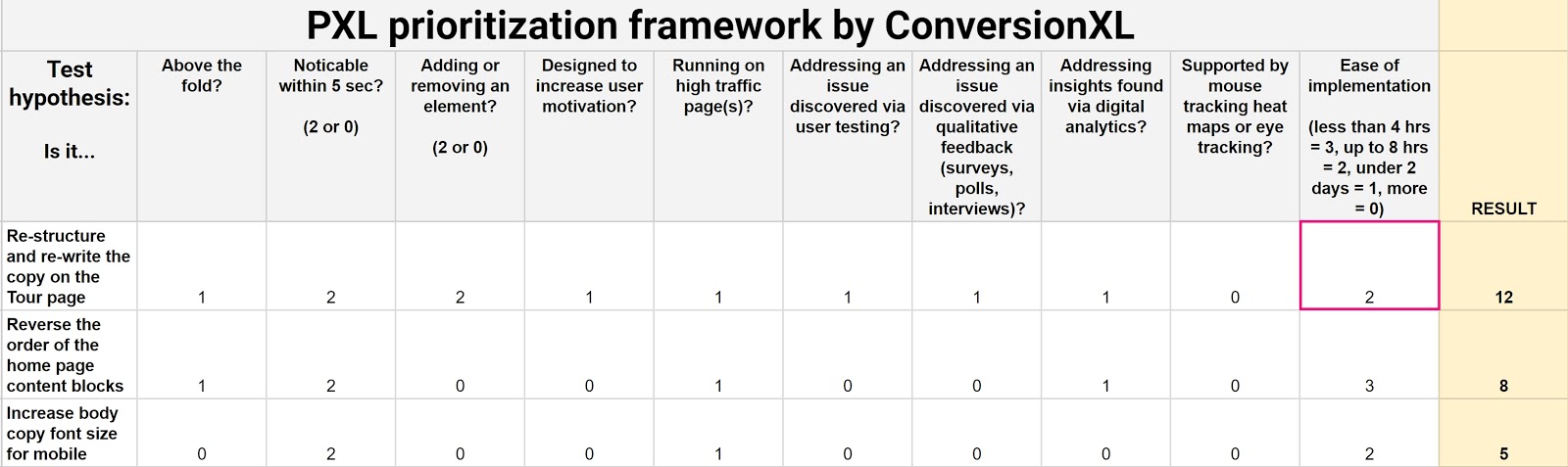
Grab your own copy of this spreadsheet template here . Just click File > Make a Copy to make it your own.
Instead of guessing what the impact might be, this framework asks you a set of questions about it:
- Is the change above the fold ? More people notice above-the-fold changes. Thus, those changes are more likely to have an impact.
- Is the change noticeable in under 5 seconds? Show a group of people the control and then the variation(s). Can they tell a difference after 5 seconds? If not, it’s likely to have less of an impact.
- Does it add or remove anything? Bigger changes like removing distractions or adding key information tend to have more of an impact.
- Does the test run on high-traffic pages? An improvement to a high-traffic page generates bigger returns.
Many potential test variables require data to prioritize your hypotheses. Weekly discussions that ask these four questions will help you prioritize testing based on data, not opinions:
- Is it addressing an issue discovered via user testing?
- Is it addressing an issue discovered via qualitative feedback (surveys, polls, interviews)?
- Is the hypothesis supported by mouse tracking, heat maps, or eye tracking ?
- Is it addressing insights found via digital analytics?
We also put bounds on Ease of implementation by bracketing answers according to the estimated time. Ideally, a test developer is part of prioritization discussions.
Grading PXL
We assume a binary scale: You have to choose one or the other. So, for most variables (unless otherwise noted), you choose either a 0 or a 1.
But we also want to weight variables based on importance—how noticeable the change is, if something is added/removed, ease of implementation. For t hese variables, we specifically say how things change. For instance, on the Noticeability of the Change variable, you either mark it a 2 or a 0.
Customizability
We built this model with the belief that you can and should customize variables based on what matters to your business.
For example, maybe you’re working with a branding or user experience team, and hypotheses must conform to brand guidelines. Add it as a variable.
Maybe you’re at a startup whose acquisition engine is fueled by SEO. Maybe your funding depends on that stream of customers. Add a category like, “doesn’t interfere with SEO,” which might alter some headline or copy tests .
All organizations operate under different assumptions. Customizing the template can account for them and optimize your optimization program.
Whichever framework you use, make it systematic and understandable to anyone on the team, as well as stakeholders.
First rule: Don’t stop a test just because it reaches statistical significance. This is probably the most common error committed by beginner optimizers with good intentions.
If you call tests when you hit significance, you’ll find that most lifts don’t translate to increased revenue (that’s the goal, after all). The “lifts” were, in fact, imaginary .
Consider this: When 1,000 A/A tests (two identical pages) were run:
- 771 experiments out of 1,000 reached 90% significance at some point.
- 531 experiments out of 1,000 reached 95% significance at some point.
Stopping tests at significance risks false positives and excludes external validity threats , like seasonality.
Predetermine a sample size and run the test for full weeks, usually at least two business cycles.
How do you predetermine sample size? There are lots of great tools . Here’s how you’d calculate your sample size with Evan Miller’s tool:
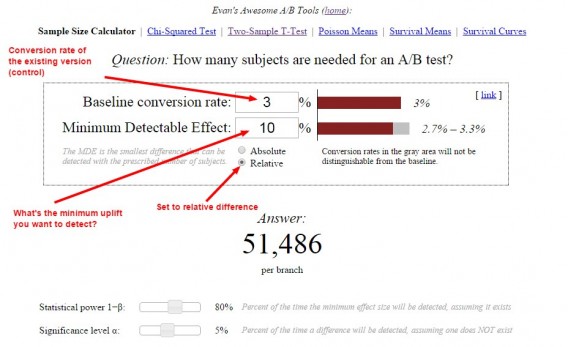
In this example, we told the tool that we have a 3% conversion rate and want to detect at least 10% uplift. The tool tells us that we need 51,486 visitors per variation before we can look at statistical significance levels.
In addition to significance level, there’s something called statistical power. Statistical power attempts to avoid Type II errors (false negatives). In other words, it makes it more likely that you’ll detect an effect if there actually was one.
For practical purposes, know that 80% power is the standard for A/B testing tools . To reach such a level, you need either a large sample size, a large effect size, or a longer duration test.
There are no magic numbers
A lot of blog posts tout magic numbers like “100 conversions” or “1,000 visitors” as stopping points. Math is not magic. Math is math, and what we’re dealing with is slightly more complex than simplistic heuristics like those figures. Andrew Anderson from Malwarebytes put it well:
It is never about how many conversions. It is about having enough data to validate based on representative samples and representative behavior. One hundred conversions is possible in only the most remote cases and with an incredibly high delta in behavior, but only if other requirements like behavior over time, consistency, and normal distribution take place. Even then, it is has a really high chance of a Type I error, false positive. Andrew Anderson
We want a representative sample. How can we get that? Test for two business cycles to mitigate external factors:
- Day of the week. Your daily traffic can vary a lot.
- Traffic sources. Unless you want to personalize the experience for a dedicated source.
- Blog post and newsletter publishing schedule.
- Return visitors. People may visit your site, think about a purchase, then come back 10 days later to buy it.
- External events. A mid-month payday may affect purchasing, for example.
Be careful with small sample sizes. The Internet is full of case studies steeped in shitty math. Most studies (if they ever released full numbers) would reveal that publishers judged test variations on 100 visitors or a lift from 12 to 22 conversions.
Once you’ve set up everything correctly, avoid peeking (or letting your boss peek) at test results before the test finishes. This can result in calling a result early due to “spotting a trend” (impossible). What you’ll find is that many test results regress to the mean.
Regression to the mean
Often, you’ll see results vary wildly in the first few days of the test. Sure enough, they tend to converge as the test continues for the next few weeks. Here’s an example from an ecommerce site:
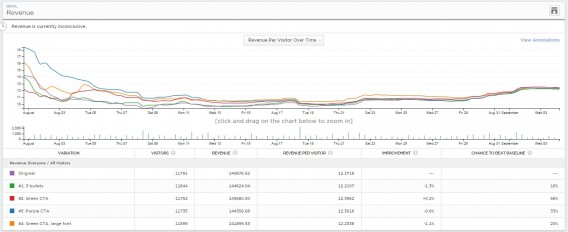
- First couple of days: Blue (variation #3) is winning big—like $16 per visitor vs. $12.50 for Control. Lots of people would (mistakenly) end the test here.
- After 7 days: Blue still winning, and the relative difference is big.
- After 14 days: Orange (#4) is winning!
- After 21 days: Orange still winning!
- End: No difference.
If you’d called the test at less than four weeks, you would have made an erroneous conclusion.
There’s a related issue: the novelty effect. The novelty of your changes (e.g., bigger blue button) brings more attention to the variation. With time, the lift disappears because the change is no longer novel.
It’s one of many complexities related to A/B testing. We have a bunch of blog posts devoted to such topics:
- Stopping A/B Tests: How Many Conversions Do I Need?
- Statistical Significance Does Not Equal Validity (or Why You Get Imaginary Lifts)
Can you run multiple A/B tests simultaneously?
You want to speed up your testing program and run more tests— high-tempo testing. But can you run more than one A/B test at the same time ?Will it increase your growth potential or pollute your data?
Some experts say you shouldn’t do multiple tests simultaneously. Some say it’s fine. In most cases, you will be fine running multiple simultaneous tests; extreme interactions are unlikely.
Unless you’re testing really important stuff (e.g., something that impacts your business model, future of the company), the benefits of testing volume will likely outweigh the noise in your data and occasional false positives.
If there is a high risk of interaction between multiple tests, reduce the number of simultaneous tests and/or let the tests run longer for improved accuracy.
If you want to learn more, read these posts:
- AB Testing: When Tests Collide ;
- Can You Run Multiple A/B Tests at the Same Time?
Once you’ve got a prioritized list of test ideas, it’s time to form a hypothesis and run an experiment. A hypothesis defines why you believe a problem occurs. Furthermore, a good hypothesis:
- Is testable. It is measurable, so it can be tested.
- Solves a conversion problem. Split-testing solves conversion problems.
- Provides market insights. With a well-articulated hypothesis, your split-testing results give you information about your customers, whether the test “wins” or “loses.”
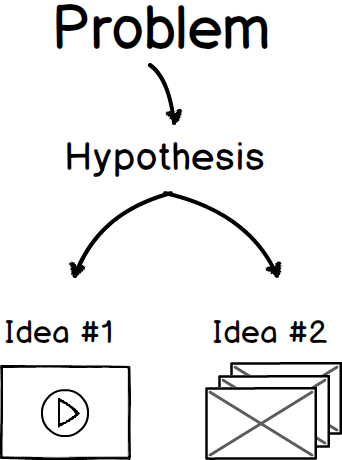
Craig Sullivan has a hypothesis kit to simplify the process:
- Because we saw (data/feedback),
- We expect that (change) will cause (impact).
- We’ll measure this using (data metric).
And the advanced one:
- Because we saw (qualitative and quantitative data),
- We expect that (change) for (population) will cause (impact[s]).
- We expect to see (data metric[s] change) over a period of (X business cycles).
Technical stuff
Here’s the fun part: You can finally think about picking a tool .
While this is the first thing many people think about, it’s not the most important. Strategy and statistical knowledge come first.
That said, there are a few differences to bear in mind. One major categorization in tools is whether they are server-side or client-side testing tools .
Server-side tools render code on the server level. They send a randomized version of the page to the viewer with no modification on the visitor’s browser. Client-side tools send the same page, but JavaScript on the client’s browser manipulates the appearance on the original and the variation.
Client-side testing tools include Optimizely, VWO, and Adobe Target. Conductrics has capabilities for both, and SiteSpect does a proxy server-side method.
What does all this mean for you? If you’d like to save time up front, or if your team is small or lacks development resources, client-side tools can get you up and running faster. Server-side requires development resources but can often be more robust.
While setting up tests is slightly different depending on which tool you use, it’s often as simple as signing up for your favorite tool and following their instructions, like putting a JavaScript snippet on your website.
Beyond that, you need to set up Goals (to know when a conversion has been made). Your testing tool will track when each variation converts visitors into customers.
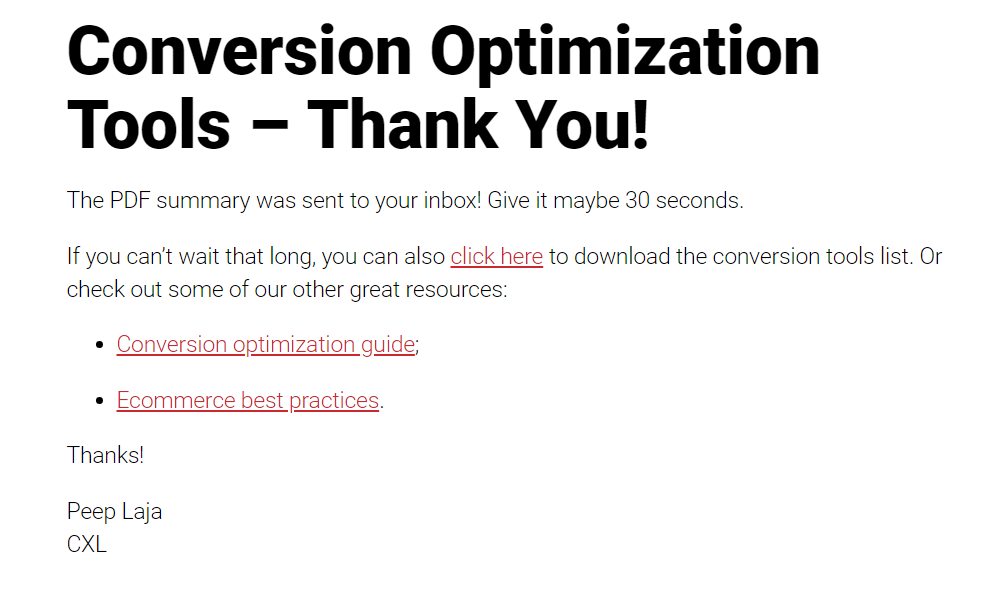
Skills that come in handy when setting up A/B tests are HTML, CSS, and JavaScript/JQuery, as well as design and copywriting skills to craft variations. Some tools allow use of a visual editor, but that limits your flexibility and control .
Alright. You’ve done your research, set up your test correctly, and the test is finally cooked. Now, on to analysis. It’s not as simple as a glimpse at the graph from your testing tool.
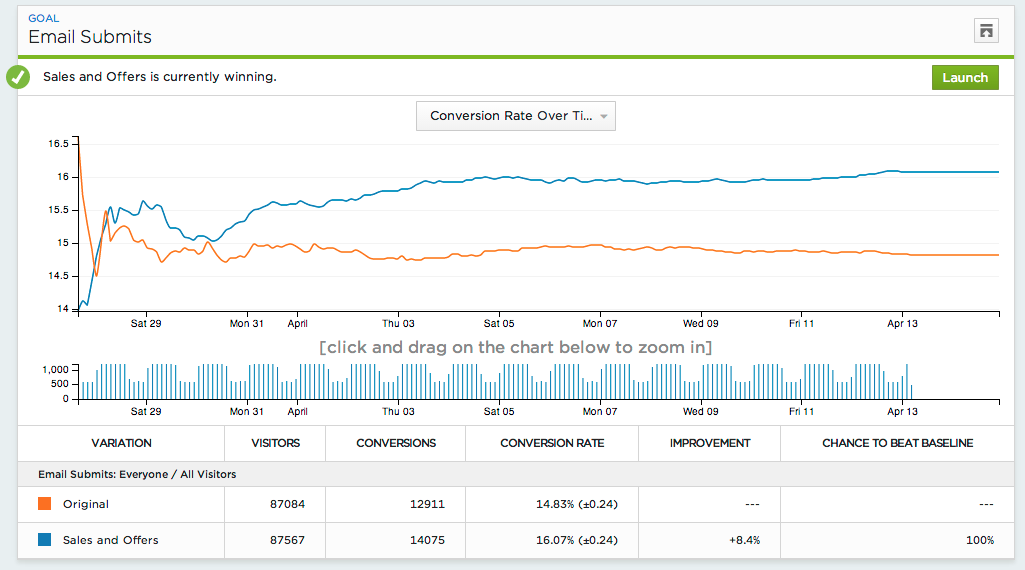
One thing you should always do: Analyze your test results in Google Analytics . It doesn’t just enhance your analysis capabilities; it also allows you to be more confident in your data and decision making.
Your testing tool could be recording data incorrectly. If you have no other source for your test data, you can never be sure whether to trust it. Create multiple sources of data.
What happens if there’s no difference between variations? Don’t move on too quickly. First, realize two things:
1. Your hypothesis might have been right, but implementation was wrong.
Let’s say your qualitative research says that concern about security is an issue. How many ways can you beef up the perception of security ? Unlimited.
The name of the game is iterative testing , so if you were on to something, try a few iterations.
2. Even if there was no difference overall, the variation might beat the control in a segment or two.
If you got a lift for returning visitors and mobile visitors—but a drop for new visitors and desktop users—those segments might cancel each other out, making it seem like there’s “no difference.” Analyze your test across key segments to investigate that possibility.
Data segmentation for A/B tests
The key to learning in A/B testing is segmenting. Even though B might lose to A in the overall results, B might beat A in certain segments (organic, Facebook, mobile, etc).
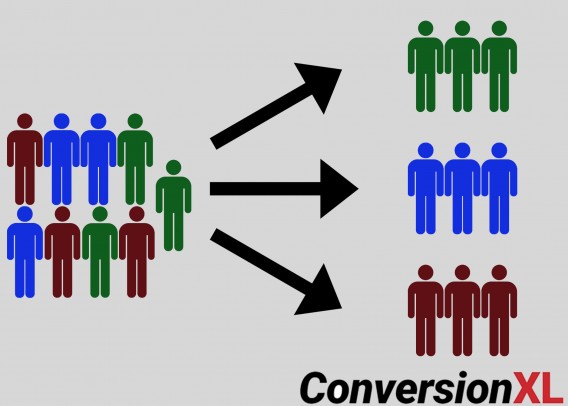
There are a ton of segments you can analyze. Optimizely lists the following possibilities:
- Browser type;
- Source type;
- Mobile vs. desktop, or by device;
- Logged-in vs. logged-out visitors;
- PPC/SEM campaign;
- Geographical regions (city, state/province, country);
- New vs. returning visitors;
- New vs. repeat purchasers;
- Power users vs. casual visitors;
- Men vs. women;
- New vs. already-submitted leads;
- Plan types or loyalty program levels;
- Current, prospective, and former subscribers;
- Roles (if your site has, for instance, both a buyer and seller role).
At the very least—assuming you have an adequate sample size—look at these segments:
- Desktop vs. tablet/mobile;
- New vs. returning;
- Traffic that lands on the page vs. traffic from internal links.
Make sure that you have enough sample size within the segment. Calculate it in advance, and be wary if it’s less than 250–350 conversions per variation within in a given segment.
If your treatment performed well for a specific segment, it’s time to consider a personalized approach for those users.
A/B testing isn’t just about lifts, wins, losses, and testing random shit. As Matt Gershoff said, optimization is about “gathering information to inform decisions,” and the learnings from statistically valid A/B tests contribute to the greater goals of growth and optimization.
Smart organizations archive their test results and plan their approach to testing systematically. A structured approach to optimization yields greater growth and is less-often limited by local maxima .
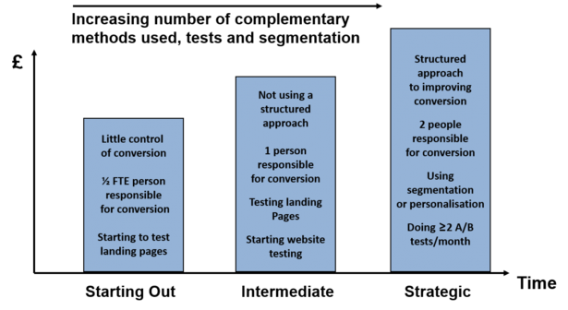
So here’s the tough part: There’s no single best way to structure your knowledge management. Some companies use sophisticated, internally built tools; some use third-party tools; and some use Excel and Trello.
If it helps, here are three tools built specifically for conversion optimization project management:
- Effective Experiments ;
- Growth Hackers’ Projects .
It’s important to communicate across departments and to executives. Often, A/B test results aren’t intuitive to a layperson. Visualization helps.
Annemarie Klaassen and Ton Wesseling wrote an awesome post on visualizing A/B test results . Here’s what they came up with:
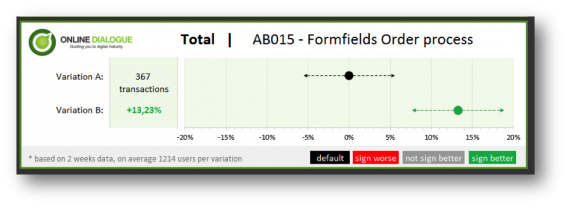
Statistical knowledge is handy when analyzing A/B test results. We went over some of it in the section above, but there’s more to cover.
Why do you need to know statistics? Matt Gershoff likes to quote his college math professor: “How can you make cheese if you don’t know where milk comes from?!”
There are three terms you should know before we dive into the nitty gritty of A/B testing statistics :
- Mean. We’re not measuring all conversion rates, just a sample. The average is representative of the whole.
- Variance. What is the natural variability of a population? That affects our results and how we use them.
- Sampling. We can’t measure the true conversion rate, so we select a sample that is (hopefully) representative.
What is a p-value?
Many use the term “statistical significance” inaccurately. Statistical significance by itself is not a stopping rule, so what is it and why is it important?
To start with, let’s go over p-values , which are also very misunderstood. As FiveThirtyEight recently pointed out, even scientists can’t easily explain p-values .
A p-value is the measure of evidence against the null hypothesis (the control, in A/B testing parlance). A p-value does not tell us the probability that B is better than A.
Similarly, it doesn’t tell us the probability that we will make a mistake in selecting B over A. These are common misconceptions.
The p-value is the probability of seeing the current result or a more extreme one given that the null hypothesis is true. Or, “How surprising is this result?”
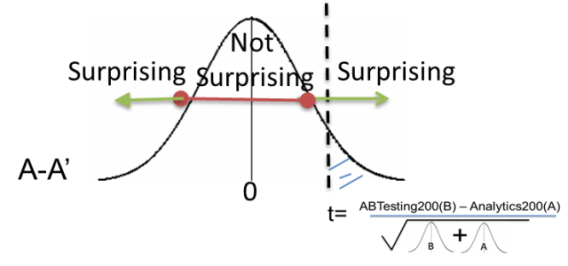
To sum it up, statistical significance (or a statistically significant result) is attained when a p-value is less than the significance level (which is usually set at 0.05).
Significance in regard to statistical hypothesis testing is also where the whole “ one-tail vs. two-tail ” issue comes up.
One-tail vs. two-tail A/B tests
One-tailed tests allow for an effect in one direction. Two-tailed tests look for an effect in two directions—positive or negative.
No need to get very worked up about this. Gershoff from Conductrics summed it up well:
If your testing software only does one type or the other, don’t sweat it. It is super simple to convert one type to the other (but you need to do this BEFORE you run the test) since all of the math is exactly the same in both tests. All that is different is the significance threshold level. If your software uses a one-tail test, just divide the p-value associated with the confidence level you are looking to run the test by two. So, if you want your two-tail test to be at the 95% confidence level, then you would actually input a confidence level of 97.5%, or if at a 99%, then you need to input 99.5%. You can then just read the test as if it was two-tailed. Matt Gershoff
Confidence intervals and margin of error
Your conversion rate doesn’t simply say X%. It says something like X% (+/- Y). That second number is the confidence interval, and it’s of utmost importance to understanding your test results.
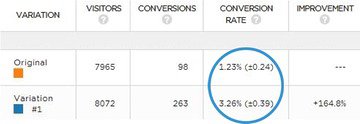
In A/B testing, we use confidence intervals to mitigate the risk of sampling errors . In that sense, we’re managing the risk associated with implementing a new variation.
So if your tool says something like, “We are 95% confident that the conversion rate is X% +/- Y%,” then you need to account for the +/- Y% as the margin of error.
How confident you are in your results depends largely on how large the margin of error is. If the two conversion ranges overlap, you need to keep testing to get a valid result.
Matt Gershoff gave a great illustration of how margin of error works:
Say your buddy is coming to visit you from Round Rock and is taking TX-1 at 5 p.m. She wants to know how long it should take her. You say I have a 95% confidence that it will take you about 60 minutes plus or minus 20 minutes. So your margin of error is 20 minutes, or 33%. If she is coming at 11 a.m. you might say, “It will take you 40 min, plus or minus 10 min,” so the margin of error is 10 minutes, or 25%. So while both are at the 95% confidence level, the margin of error is different. Matt Gershoff
External validity threats
There’s a challenge with running A/B tests: Data isn’t stationary.
A stationary time series is one whose statistical properties (mean, variance, autocorrelation, etc.) are constant over time. For many reasons, website data is non-stationary, which means we can’t make the same assumptions as with stationary data. Here are a few reasons that data might fluctuate:
- Day of the week;
- Positive or negative press mentions;
- Other marketing campaigns;
- Word-of-mouth.
Others include sample pollution , the flicker effect, revenue tracking errors, selection bias, and more. ( Read here. ) These are things to keep in mind when planning and analyzing your A/B tests.
Bayesian or frequentist Stats
Bayesian or Frequentist A/B testing is another hot topic. Many popular tools have rebuilt their stats engines to feature a Bayesian methodology.
Here’s the difference (very much simplified): In the Bayesian view, a probability is assigned to a hypothesis. In the Frequentist view, a hypothesis is tested without being assigned a probability.
Rob Balon , who carries a PhD in statistics and market research, says the debate is mostly esoteric tail wagging from the ivory tower. “In truth,” he says, “most analysts out of the ivory tower don’t care that much, if at all, about Bayesian vs. Frequentist.”
Don’t get me wrong, there are practical business implications to each methodology. But if you’re new to A/B testing, there are much more important things to worry about.
Now, how do you start running A/B tests?
Littered throughout this guide are tons of links to external resources: articles, tools, books and books. We’ve tried to compile all the most valuable knowledge in our A/B Testing course . On top of that, here are some of the best resources (divided by categories).
A/B testing tools
There are a lot of tools for online experimentation. Here’s a list of 53 conversion optimization tools , all reviewed by experts. Some of the most popular A/B testing tools include:
- Optimizely ;
- Adobe Target ;
- Maximyser ;
- Conductrics .
A/B testing calculators
- AB Test Calculator by CXL;
- A/B Split Test Significance Calculator by VWO ;
- A/B Split and Multivariate Test Duration Calculator by VWO ;
- Evan Miller’s Sample Size Calculator .
A/B testing statistics resources
- A/B Testing Statistics: An Easy-to-Understand Guide ;
- Statistical Analysis and A/B Testing ;
- Understanding A/B testing statistics to get REAL Lift in Conversions ;
- One-Tailed vs Two-Tailed Tests (Does It Matter?) ;
- Bayesian vs Frequentist A/B Testing – What’s the Difference? ;
- Sample Pollution ;
- Science Isn’t Broken .
A/B testing/CRO strategy resources
- 4 Frameworks To Help Prioritize & Conduct Your Conversion Testing ;
- What you have to know about conversion optimization ;
- Conversion Optimization Guide .
A/B testing is an invaluable resource to anyone making decisions in an online environment. With a little bit of knowledge and a lot of diligence, you can mitigate many of the risks that most beginning optimizers face.
If you really dig into the information here, you’ll be ahead of 90% of people running tests. If you believe in the power of A/B testing for continued revenue growth, that’s a fantastic place to be.
Knowledge is a limiting factor that only experience and iterative learning can transcend. So get testing!
Working on something related to this? Post a comment in the CXL community !
Related Posts
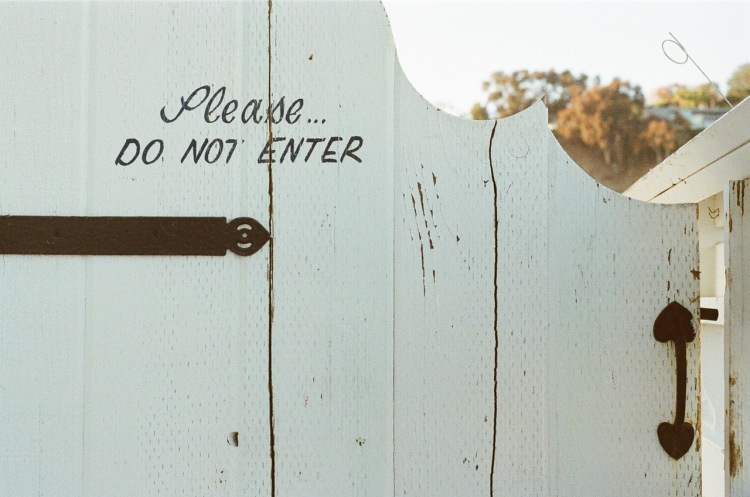
A/B testing is highly useful, no question here. But a lot of businesses should not be…
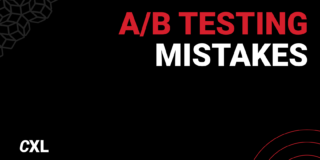
A/B testing is fun. With so many easy-to-use tools, anyone can—and should—do it. However, there's…
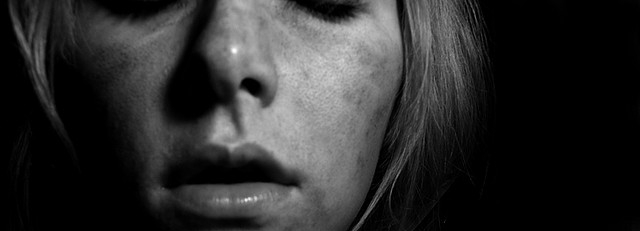
Sometimes A/B testing is made to seem like some magical tool that will fix all…
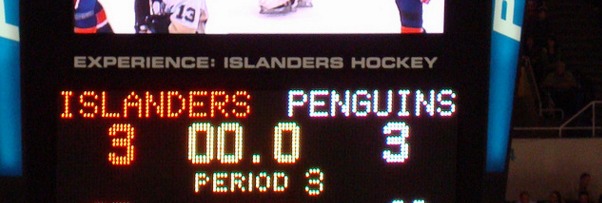
So you ran a test - and you ran it correctly, following A/B testing best…
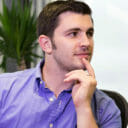
Alex Birkett
Alex Birkett is a former content and growth marketer at CXL. Currently, he is the co-founder at Omniscient Digital and works on user acquisition growth at HubSpot. Follow his writing at alexbirkett.com .
Join the conversation Add your comment
Hi, great article! I have a question about the Evan Miller’s tool. I’m using Monetate as A/B testing tool and some of the KPIs/metrics, such as Revenue Per Session, are measured in dollars. So for example, I can have a campaign that says experiment performs at $4.52 and control at $3.98. What can I consider as a Baseline Conversion Rate?

Hey! You would still use Evan Miller’s tool to calculate how many people you need in the test, but you can’t use the same A/B test calculator for deciding which one is the winner. There’s an excellent answer to this in the CXL Facebook group by Chad Sanderson:
T Test or proportion tests don’t work when measuring Revenue Per Visitor because it violates the underlying assumptions of the test. (For T Test, the assumption is your data is spread evenly around a mean, whereas proportion or binomial tests measure successes or failures only) RPV data is not spread normally around the mean (The vast majority of visitors will purchase nothing) and we’re not looking at a proportion (Because we need to find the average revenue per visitor). So the best way to conduct a test on RPV is to use a Mann-Whitney U or Wilcoxon test, which are both rank based sum tests that is designed exactly for cases like this.
Comments are closed.
Current article:
Search posts.
- Acquisition (182)
- Brand Building (22)
- Business Building (109)
- Copywriting (42)
- CRO & Testing (316)
- Customer Stories (7)
- Digital Analytics (89)
- Marketing Tactics (50)
- Original Research (15)
- Psychology (81)
- Social Media (20)
- User Experience & Persuasive Design (179)
Subscribe to our newsletter.
Join 140,000+ marketers and get a weekly expert-led newsletter focused on helping marketing teams overcome growth challenges, punch above their weight, and crush their competition.
- Your e-mail *
- I agree to receive updates from CXL.
- Comments This field is for validation purposes and should be left unchanged.
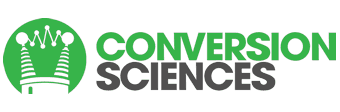
The Ultimate A/B Testing Guide: Everything You Need, All In One Place
Welcome to the ultimate A/B testing guide from the original and most experienced Conversion Optimization Agency on the planet!
In this post, we’re going to cover everything you need to know about A/B testing (also referred to as “split” testing), from start to finish. Here’s what we’ll cover:
Table of Contents
By the end of this guide, you’ll have a thorough understanding of the entire AB testing process and a framework for diving deeper into any topic you wish to further explore.
In addition to this guide, we’ve put together an intuitive 9-part course taking you through the fundamentals of conversion rate optimization. Complete the course, and we’ll review your website for free!
No time to learn it all on your own? Check out our turn-key Conversion Rate Optimization Services and book a consultation to see how we can help you.
1. The Basic Components Of A/B Testing
AB testing, also referred to as “split” or “A/B/n” testing, is the process of testing multiple variations of a web page in order to identifying higher performing variations and improve the page’s conversion rate.
Over the last few years, AB testing has become “kind of a big deal”.
Online marketing tools have become more sophisticated and less expensive, making split testing a more accessible pursuit for small and mid-sized businesses. And with traffic becoming more expensive, the rate at which online businesses are able to convert incoming visitors is becoming more and more important.
The basic A/B testing process looks like this:
- Make a hypothesis about one or two changes you think will improve the page’s conversion rate.
- Create a variation or variations of that page with one change per variation.
- Divide incoming traffic equally between each variation and the original page.
- Run the test as long as it takes to acquire statistically significant findings.
- If a page variation produces a statistically significant increase in page conversions, use it it replace the original page.
Have you ever heard the story of someone changing their button color from red to green and received a $5 million increase in sales that year?
As cool as that sounds, let’s be honest: it is not likely that either you or I will see this kind of a win anytime soon. That said, one button tweak did result in $300 million in new revenue for one business, so it is possible.
AB testing is a scientific way of finding out if your tweak that leads to a boost in conversions is actually significant, or just a random flux.
AB testing (AKA “split testing”) is the process of directing your traffic to two or more variations of a web page.
AB testing is pretty simple to understand:
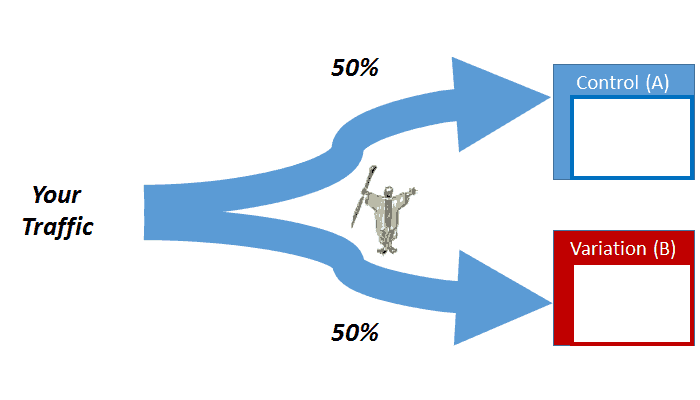
A typical AB test uses AB testing software to divide traffic.
Our testing software is the “Moses” that splits our traffic for us. Additionally, you can choose to experiment with more variations than an AB test. These tests are called A/B/n tests, where “n” represents any number of new variations.
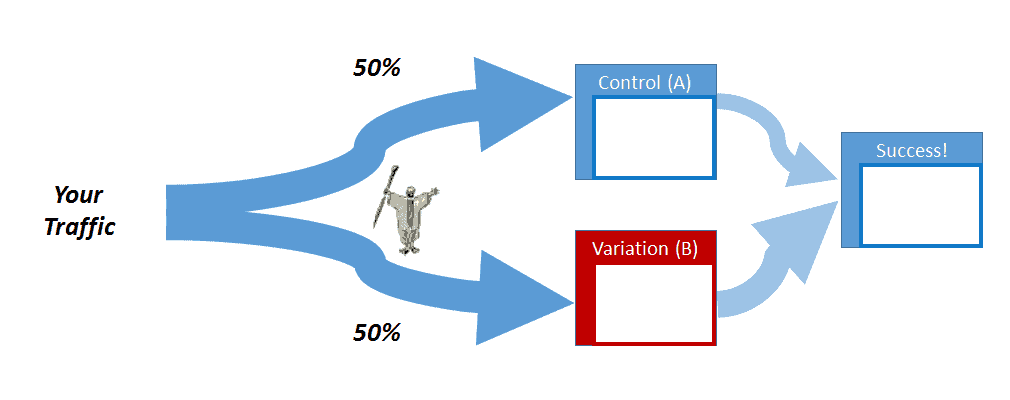
The goal of AB testing is to measure if a variation results in the more conversions.
So that could be an “A/B/C” test, an “A/B/C/D” test, and so on.
Here’s what an A/B/C test would look like:
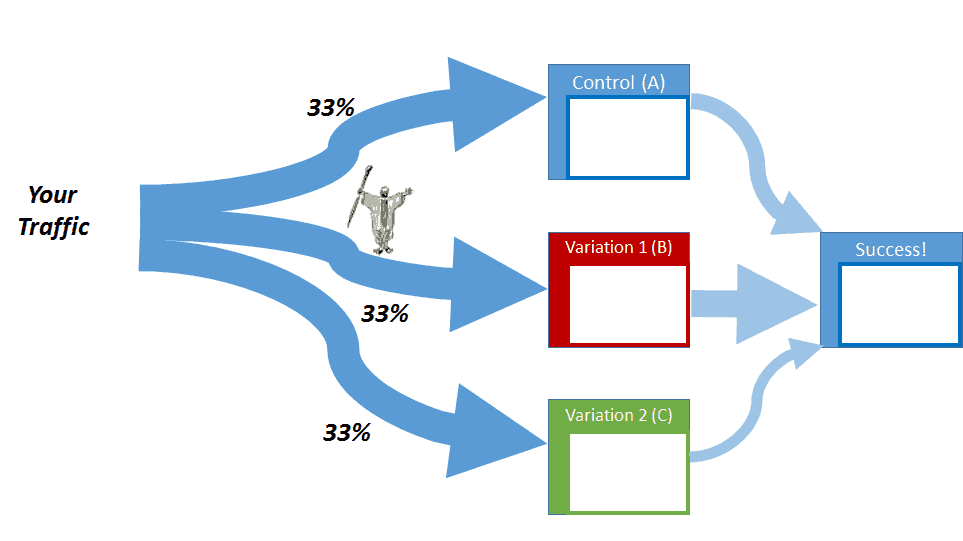
The more variations we have in an AB test, the more we have to divide the traffic.
Even though the same traffic is sent to the Control and each Variation, a different number of visitors will typically complete their task — buy, signup, subscribe, etc. This is because a many leave your site first.
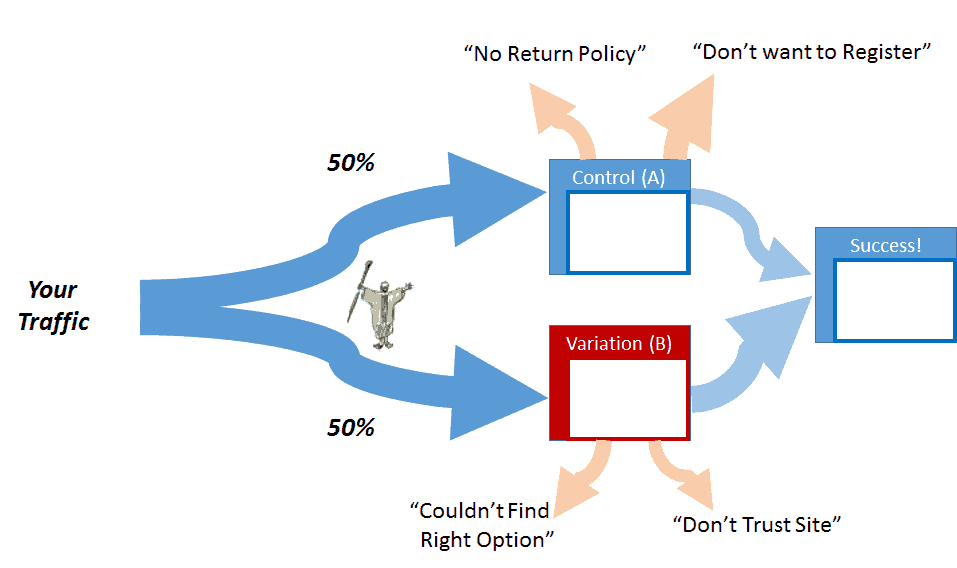
We research our visitors to find out what might be making them leave before converting. These are our test hypotheses.
The primary point of an AB test is to discover what issues cause visitors to leave. The issues above are common to ecommerce websites. In this case we might create additional variations:
- One that adds a return policy to the page.
- One that removes the registration requirement.
- One that adds trust symbols to the site.
By split testing these changes, we see if we can get more of these visitors to finish their purchase, to convert.
How do we know which issues might be causing visitors leave? This is done by researching your visitors, looking at analytics data, and making educated guesses , which we at Conversion Sciences call “hypotheses”.
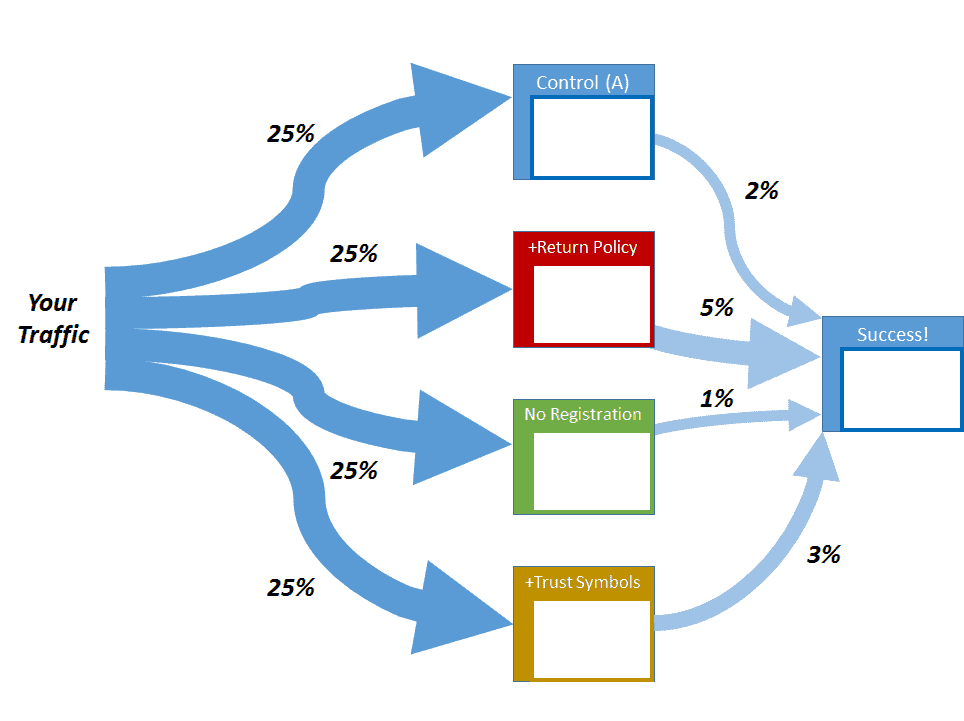
In this example, adding a return policy performed best. Removing the registration requirement performed worse than the Control.
In the image above, the number of visitors that complete a transaction is shown. Based on this data, we would learn that adding a return policy and trust symbols would increase success over the Control or removing registration.
The page that added the return policy is our new Control. Our next test would very likely be to see what happens when we add trust symbols to this new Control. It is not unlikely that combining the two could actually reduce the conversion rate. So we test it.
Likewise, it is possible that removing the registration requirement would work well on the page with the return policy, our new Control. However, we may not test this combination.
With an AB test, we try each change on it’s own variation to isolate the specific issues and decide which combinations to test based on what we learn.
The goal of AB testing is to identify and verify changes that will increase a page’s overall conversion rate, whether those changes are minor or more involved.
I’m fond of saying that AB testing, or split testing, is the “Supreme Court” of data collection. An AB test gives us the most reliable information about a change to our site. It controls for a number of variables that can taint our data.
2. The Proven AB Testing Framework
Now that we have a feel for the tests themselves, we need to understand how these tests fit into the grand scheme of things.
There’s a reason we are able to get consistent results for our clients here at Conversion Sciences. It’s because we have a proven framework in place: a system that allows us to approach any website and methodically derive revenue-boosting insights.
Different businesses and agencies will have their own unique processes within this system, but any CRO agency worth it’s name will follow some variation of the following framework when conducting A/B testing.

For a closer look at each of these nine steps, check out our in-depth breakdown here: The Proven AB Testing Framework Used By CRO Professionals
3. The Critical Statistics Behind Split Testing
You don’t need to be a mathematician to run effective AB tests, but you do need a solid understanding of the statistics behind split testing.
An AB test is an example of statistical hypothesis testing , a process whereby a hypothesis is made about the relationship between two data sets and those data sets are then compared against each other to determine if there is a statistically significant relationship or not.
To put this in more practical terms, a prediction is made that Page Variation #B will perform better than Page Variation #A, and then data sets from both pages are observed and compared to determine if Page Variation #B is a statistically significant improvement over Page Variation #A.
That seems fairly straightforward, so where does it get complicated?
The complexities arrive in all the ways a given “sample” can inaccurately represent the overall “population”, and all the things we have to do to ensure that our sample can accurately represent the population.
Let’s define some terminology real quick.
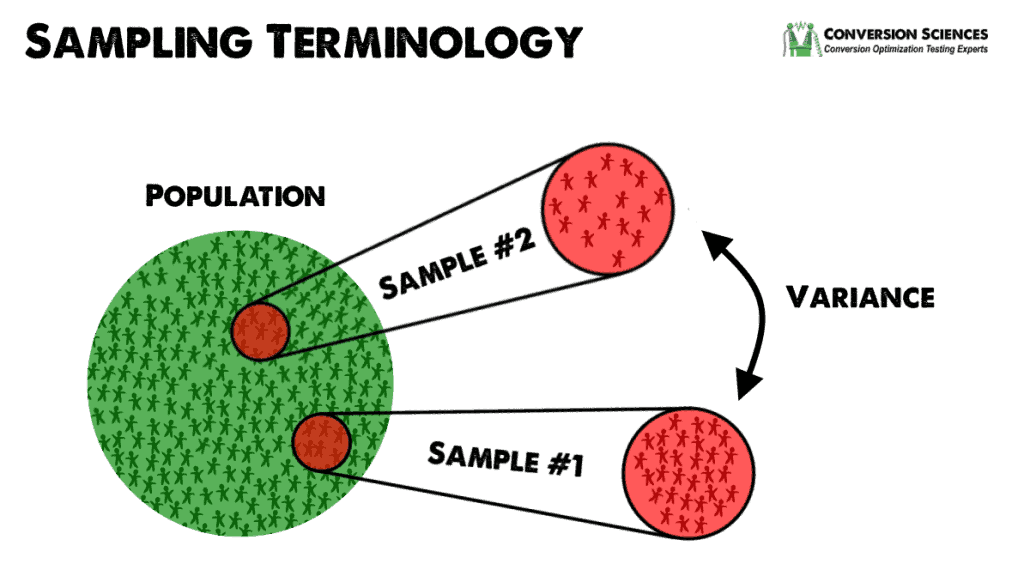
Population and Variance.
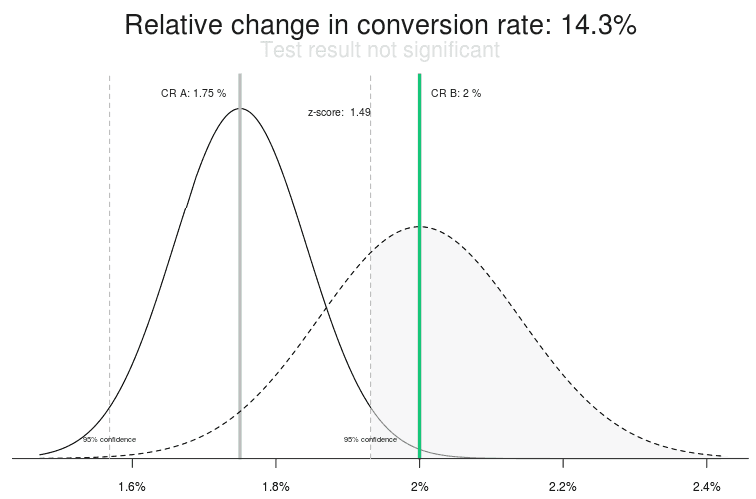
While it appears that one version is doing better than the other, the results overlap too much.
The “ population ” is the group we want information about. It’s the next 100,000 visitors in my previous example. When we’re testing a webpage, the true population is every future individual who will visit that page.
The “ sample ” is a small portion of the larger population. It’s the first 1,000 visitors we observe in my previous example.
In a perfect world, the sample would be 100% representative of the overall population.
For example:
Let’s say 10,000 out of those 100,000 visitors are going to ultimately convert into sales. Our true conversion rate would then be 10%.
In a tester’s perfect world, the mean (average) conversion rate of any sample(s) we select from the population would always be identical to the population’s true conversion rate. In other words, if you selected a sample of 10 visitors, 1 of them (10%) would buy, and if you selected a sample of 100 visitors, then 10 would be buy.
But that’s not how things work in real life.
In real life, you might have only 2 out of the first 100 buy or you might have 20… or even zero. You could have a single purchase from Monday through Friday and then 30 on Saturday.
This variability across samples is expressed as a unit called the “ variance ”, which measures how far a random sample can differ from the true mean (average).
This variance across samples can derail our findings, which is why we have to employ statistically sound hypothesis testing in order get accurate results.
How AB Testing Eliminates Timing Issues
One alternative to AB testing is “serial” testing, or change-something-and-see-what-happens testing. I am a fan of serial testing, and you should make it a point to go and see how changes are affecting your revenue, subscriptions and lead.
There is a problem, however. If you make your change at the same time that a competitor starts an awesome promotion, you may see a drop in your conversion rates. You might blame your change when, in fact, the change in performance was an external market force .
AB testing controls for this.
In an AB test, the first visitor sees the original page, which we call the Control . This is the “A” in the term “AB test”.The next visitor sees a version of the page with the change that’s being tested. We call this a Treatment , or Variation . This is the “B” in the term AB test. We can also have a “C” and a “D” if we have enough traffic.
The next visitor sees the control and the next the treatment. This goes on until we enough people have seen each version to tell us which they like best. We call this statistical significance . Our software tracks these visitors across multiple visits and tells us which version of the page generated the most revenue or leads.
Since visitors come over the same time period, changes in the marketplace — like our competitor’s promotion — won’t affect our results. Both pages are served during the promotion, so there is no before-and-after error in the data.
Another way variance can express itself is in the way different types of traffic behave differently. Fortunately, you can eliminate this type of variance simply by segmenting traffic.
How Visitor Segmentation Controls For Variability
An AB test gathers data from real visitors and customers who are “voting” on our changes using their dollars, their contact information and their commitment to our offerings. If done correctly, the makeup of visitors should be the same for the control and each treatment.
This is important. Visitors that come to the site from an email may be more likely to convert to a customer. Visitors coming from organic search, however, may be early in their research, with not as many ready to buy.
If you sent email traffic to your control and search traffic to the treatment, it may appear that the control is a better implementation. In truth, it was the kind of traffic or traffic segment that resulted in the different performance.
By segmenting types of traffic and testing them separately, you can easily control for this variation and get a much better understanding of visitor behavior.
Why Statistical Significance Is Important
One of the most important concepts to understand when discussing AB testing is statistical significance, which is ultimately all about using large enough sample sizes when testing. There are many places where you can acquire a more technical understanding of this concept, so I’m going to attempt to illustrate it instead in layman’s terms.
Imagine flipping a coin 50 times. While from a probability perspective, we know there is a 50% chance of any given flip landing on heads, that doesn’t mean we will get 25 heads and 25 tails after 50 flips. In reality, we will probably see something like 23 heads and 27 tails or 28 heads and 22 tails.
Our results won’t match the probability because there is an element of chance to any test – an element of randomness that must be accounted for. As we flip more times, we decrease the effect this chance will have on our end results. The point at which we have decreased this element of chance to a satisfactory level is our point of statistical significance.
In the same way, when running an AB tests on a web page, there is an element of chance involved. One variation might happen to receive more primed buyers than the other or perhaps an isolated group of visitors happen to have a negative association with an image used on one page. These chance factors will skew your results if your sample size isn’t large enough.
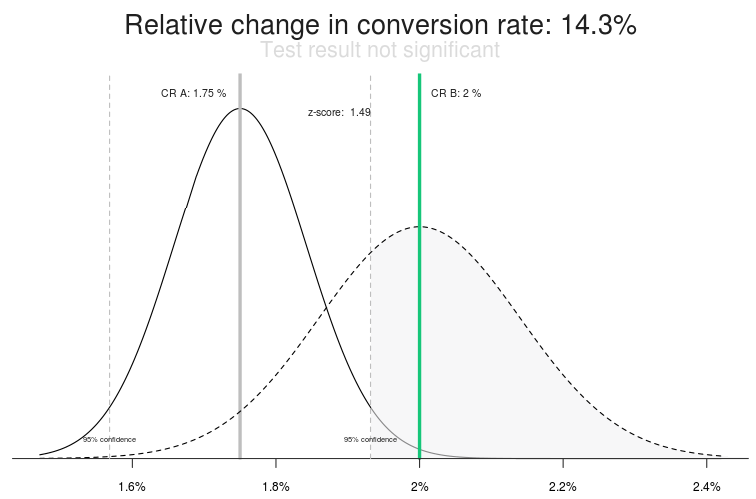
It’s important not to conclude an AB test until you have reach statistically significant results. Here’s a handy tool to check if your sample sizes are large enough.
For a closer look at the statistics behind A/B testing, check out this in-depth post: AB Testing Statistics: An Intuitive Guide For Non-Mathematicians
4. How To Conduct Pre-Test Research
The definition of optimization boils down to understanding your visitors.
In order to succeed at A/B testing, we need to be creating variations that perform better for our visitors. In order to create those types of variations, we need to understand what visitors aren’t liking about our existing site and what they want instead.
Aka we need research.
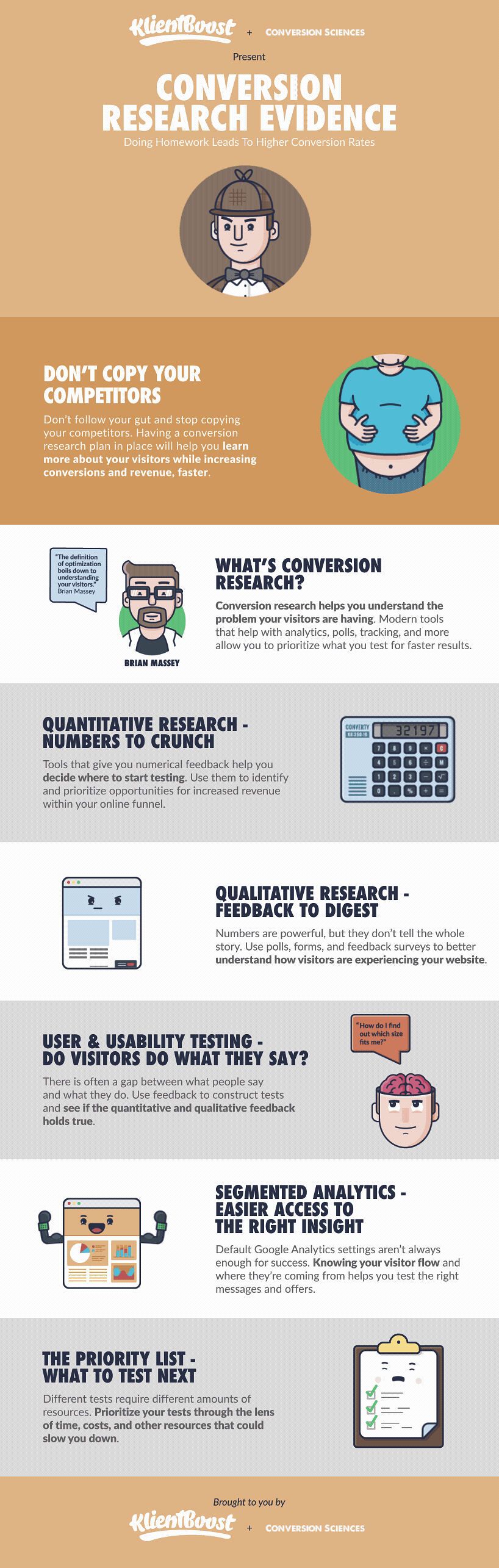
For a close look at each of these sections, check out our full writeup here: AB Testing Research: Do Your Conversion Homework
5. How To Create An A/B Testing Strategy
Once we’ve done our homework and identified both problem areas and opportunities for improvement on our site, it’s time to develop a core testing strategy.
An A/B testing strategy is essentially a lens through which we will approach test creation. It helps us prioritize and focus our efforts in the most productive direction possible.
There are 7 primary testing strategies that we use here at Conversion Sciences.
- Gum Trampoline
- Completion Optimization
- Flow Optimization
- Minesweeper
- Nuclear Option
Since there is little point in summarizing these, click here to read our breakdown of each strategy: The 7 Core Testing Strategies Essential To Optimization
6. “AB” & “Split” Testing Versus “Multivariate” Testing
While most marketers tend to use these terms interchangeably, there are a few differences to be aware of. While AB testing and split testing are the exact same thing, multivariate testing is slightly different.
AB and Split tests refer to tests that measure larger changes on a given page. For example, a company with a long-form landing page might AB test the page against a new short version to see how visitors respond. In another example, a business seeking to find the optimal squeeze page might design two pages around different lead magnets and compare them to see which converts best.
Multivariate testing, on the other hand, focuses on optimizing small, important elements of a webpage, like CTA copy, image placement, or button colors. Often, a multivariate test will test more than two options at a time to quickly identify outlying winners. For example, a company might run a multivariate test cycling 6 different button colors on its most important sales page. With high enough traffic, even a 0.5% increase in conversions can result in a significant revenue boost.
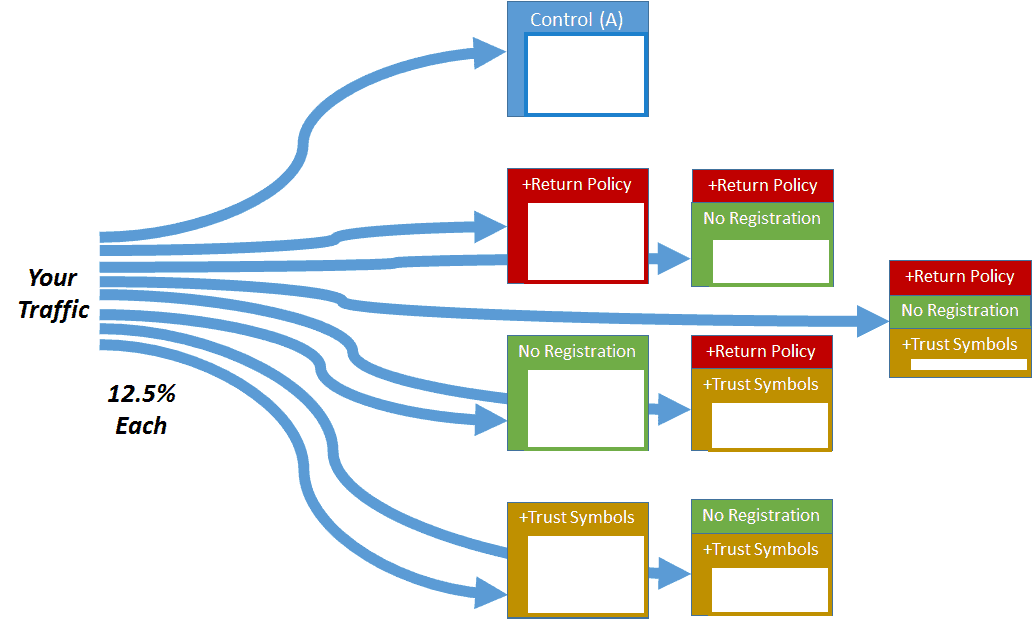
Multivariate testing works through all possible combinations.
While most websites can run meaningful split tests, multivariate tests are typically reserved for bigger sites, as they require a large amount traffic to produce statistically significant results.
For a more in-depth look at multivariate testing, click here: Multivariate Testing: Promises and Pitfalls for High-Traffic Websites
7. How To Analyze Testing Results
After we’ve run our tests, it’s time to collect and analyze the results. My co-founder Joel Harvey explains how Conversion Sciences approaches post-test analysis below:
When you look at the results of an AB testing round, the first thing you need to look at is whether the test was a loser, a winner, or inconclusive. Verify that the winners were indeed winners. Look at all the core criteria: statistical significance, p-value, test length, delta size, etc. If it checks out, then the next step is to show it to 100% of traffic and look for that real-world conversion lift. In a perfect world you could just roll it out for 2 weeks and wait, but usually, you are jumping right into creating new hypotheses and running new tests, so you have to find a balance. Once we’ve identified the winners, it’s important to dive into segments . Mobile versus non-mobile Paid versus unpaid Different browsers and devices Different traffic channels New versus returning visitors (important to setup and integrate this beforehand) This is fairly easy to do with enterprise tools, but might require some more effort with less robust testing tools. It’s important to have a deep understanding of how tested pages performed with each segment. What’s the bounce rate? What’s the exit rate? Did we fundamentally change the way this segment is flowing through the funnel? We want to look at this data in full, but it’s also good to remove outliers falling outside two standard deviations of the mean and re-evaluate the data. It’s also important to pay attention to lead quality. The longer the lead cycle, the more difficult this is. In a perfect world, you can integrate the CRM, but in reality, this often doesn’t work very seamlessly.
For a more in-depth look at post test analysis, including insights from the CRO industry’s foremost experts, click here: 10 CRO Experts Explain How To Profitably Analyze AB Test Results
8. How AB Testing Tools Work
The tools that make AB testing possible provide an incredible amount of power. If we wanted, we could use these tools to make your website different for every visitor to your website. The reason we can do this is that these tools change your site in the visitors’ browsers.
When these tools are installed on your website, they send some code, called JavaScript along with the HTML that defines a page. As the page is rendered, this JavaScript changes it. It can do almost anything:
- Change the headlines and text on the page.
- Hide images or copy.
- Move elements above the fold.
- Change the site navigation.
Primary Functions of AB Testing Tools
AB testing software has the following primary functions.
Serve Different Webpages to Visitors
The first job of AB testing tools is to show different webpages to certain visitors. The person that designed your test will determine what gets showed.
An AB test will have a “control”, or the current page, and at least one “treatment”, or the page with some change. The design and development team will work together to create a different treatment. The JavaScript must be written to transform the control into the treatment.
It is important that the JavaScript work in on all devices and in all browsers used by the visitors to a site. This requires a committed QA effort.
Conversion Sciences maintains a library of devices of varying ages that allows us to test our JavaScript for all visitors.
Split Traffic Evenly
Once we have JavaScript to display one or more treatements, our AB testing software must determine which visitors see the control and which see the treatments.
Typically, every other user will get a different page. The first will see the control, the next will see the first treatment, the next will see the second treatment and the fourth will see the control. Around it goes until enough visitors have been tested to achieve statistical significance.
It is important that the number of visitors seeing each version is about the same size. The software tries to enforce this.
Measure Results
The AB testing software tracks results by monitoring goals. Goals can be any of a number of measurable things:
- Products bought by each visitor and the amount paid
- Subscriptions and signups completed by visitors
- Forms completed by visitors
- Documents downloaded by visitors
Almost anything can be measured, but the most important are business-building metrics such as purchases, subscriptions and leads generated.
The software remembers which test page was seen. It calculates the amount of revenue generated by those who saw the control, by those who saw treatment one, and so on.
At the end of the test, we can answer one very important question: which page generated the most revenue, subscriptions or leads? If one of the treatments wins, it becomes the new control.
And the process starts over.
Do Statistical Analysis
The tools are always calculating the confidence that a result will predict the future. We don’t trust any test that doesn’t have at least a 95% confidence level. This means that we are 95% confident that a new change will generate more revenue, subscriptions or leads.
Sometimes it’s hard to wait for statistical significance, but it’s important lest we make the wrong decision and start reducing the website’s conversion rate.
Report Results
Finally, the software communicates results to us. These come as graphs and statistics.
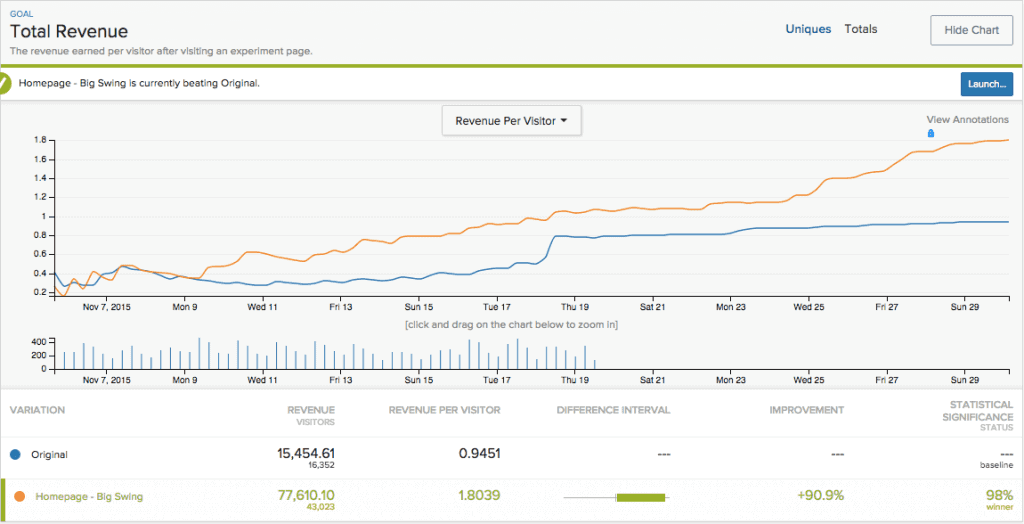
AB Testing Tools deliver data in the form of graphs and statistics.
It’s easy to see that the treatment won this test, giving us an estimated 90.9% lift in revenue per visitor with a 98% confidence.
This is a rather large win for this client.
Selecting The Right Tools
Of course, there are a lot of A/B testing tools out there, with new versions hitting the market every year. While there are certainly some industry favorites, the tools you select should come down to what your specific businesses requires.
In order to help make the selection process easier, we reached out to our network of CRO specialists and put together a list of the top-rated tools in the industry. We rely on these tools to perform for multi-million dollar clients and campaigns, and we are confident they will perform fo you as well.
Check out the full list of tools here: The 20 Most Recommended AB Testing Tools By Leading CRO Experts
9. How To Build An A/B Testing Team
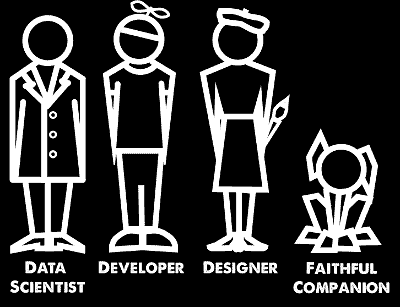
The members of a CRO team.
Conversion Sciences offers a complete turnkey team for testing. Every team that will use these tools must have competent people in the following roles, and we recommend you follow suit in building your own teams.
Data Analyst
The data analyst looks at the data being collected by analytics tools, user experience tools, and information collected by the website owners. From this she begins developing ideas, or hypotheses, for why a site doesn’t have a higher conversion rate.
The data analyst is responsible for designing tests that prove or disprove a hypothesis. Once the test is designed, she hands it off to the designer and developer for implementation.
The designer is responsible for designing new components for the site. These may be as simple as creating a button with a different call to action, to completely redesigning a landing page for conversion.
The designer must be experienced enough to carefully design the changes we are testing. We want to change the element we are testing and nothing else.
Our developers are very good at creating JavaScript that manipulates a page without breaking anything. They are experienced enough to write JavaScript that will run successfully on a variety of devices, operating systems and browsers.
The last thing we want to do is break a commercial website. This can result in lost revenue and invalidate our tests. A good quality assurance person checks the JavaScript and design work to ensure it works on all relevant devices, operating systems and browsers.
Getting Started on AB Testing
Conversion Sciences invites all businesses to work AB testing into their marketing mix. You can start by working with us and then move the effort in-house.
Get started with our 180-day Conversion Catalyst program, a process designed to get you started AND pay for itself with newly discovered revenue.
- Recent Posts
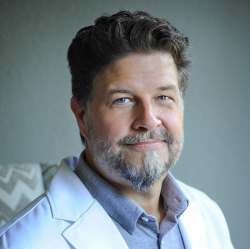
- Confirmation Bias: What It Is and How It’s Hurting Your Website Conversions - August 20, 2024
- The Conversion Optimization Process for High Converting Websites - August 20, 2024
- Two Guys on Your Website: The Surprising Link Between CRO and SEO - June 27, 2024
You might also like
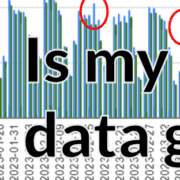
I landed on your blog through Growthhackers.com and found out that you guys are doing great. I really loved this A/B test guide. Keep on generating such great content.
Thanks for the kind words!
The portion regarding calculating sample size is incomplete.
You suggest to navigate to this link to calculate if results are significant: https://vwo.com/tools/ab-test-siginficance-calculator/
This calculator is fine to test if the desired confidence interval is met; however, it doesn’t consider whether the correct sample size was evaluated to determine if the results are statistically significant.
For example, let’s use the following inputs:
Number of visitors – Control = 1000, Variant = 1000 Number of conversions – Control = 10, Variant = 25
If plug these numbers into the calculator, we’ll that it met the confidence interval of 95%. The issue is that the sample size needed to detect a lift 1.5% with base conversions of 1% (10 conversions / 1000 visitors) would be nearly 70,000.
Just because a confidence interval was met does NOT mean that sample size is large enough to be statistically significant. This is a huge misunderstanding in the A/B community and needs to be called out.
Colton, you are CORRECT. We would never rely on an AB test with only 35 conversions.
In this case, we can look at the POWER of the test. Here’s another good tool that calculates the power for you.
The example you present shows a 150% increase, a change so significant that it has a power of 97%.
So, as a business owner, can I accept the risk that this is a false positive (<3%) or will I let the test run 700% longer to hit pure statistical significance?
Trackbacks & Pingbacks
[…] most promising ideas that don’t fall into the “fix it” category get an AB test. This will tell you which conversion rate hacks will improve the site and by how much. It is the […]
[…] This is why we test. […]
[…] is my bread and butter. To be transparent: I’m the CEO of Convert.com. We make A/B testing software and have been investing pretty heavily in GDPR […]
[…] Master the ins and outs OF ONE TRAFFIC SOURCE. Focus on promoting and tweaking your campaigns via A/B testing and make sure you use a good tracking solution so you can analyze the data and focus on what works […]
[…] big and put them where the visitor expects them to be. If you’re looking for good ideas for testing button design, consider the game of […]
[…] didn’t stop with this input. We removed the confusing features from the feature list, and designed an AB test to collect some observational […]
[…] the results or B: use an A/B test. For those of you that don’t know what A/B testing is there an awesome guide over at Conversion […]
[…] experience is one of the more complicated optimization puzzles you’ll tackle, which is why sound A/B testing is such an instrumental part of the […]
[…] testing is distinct from A/B testing in that it involves the simultaneous observation and analysis of more than one outcome […]
[…] AB testing certainly isn’t a new topic here at Conversion Sciences. It’s also not a new topic at Etsy, where the team has been fostering a culture of continuous split testing. […]
[…] Experiment with ordering your pricing plans from most expensive to least expensive. This one is pretty simple, and there’s not much to say except that there is data to suggest this is worth a split test. […]
[…] you know good headings, subheads, and headlines are crucial to improving conversion rates (and A/B testing them is simple), but the actual process of creating the right ones can get […]
[…] At Conversion Sciences, we have a checklist that our team goes through when evaluating a new client website, and today, we’re going to share that checklist with you. This checklist includes virtually everything you’ll want to consider optimizing while putting together your own A/B testing campaign. […]
[…] The Ultimate Guide To A/B Testing […]
[…] If you aren’t A/B testing, you are leaving a ton of money on the table. […]
[…] We recently partnered with NinjaTropic to produce a new video introducing readers to A/B testing as part of our ongoing A/B testing guide. […]
[…] copywriting, but if you are going to break away from best practices, you absolutely need to begin A/B testing your […]
[…] with a copywriter is a great opportunity for AB testing. Instead of simply throwing up something new and hoping it works… test, test, […]
[…] The Ultimate A/B Testing Guide: Everything You Need, All In One Place December 15, 2016 […]
[…] Unexpected Website Forumulas of The Conversion Scientist. Conversion Sciences specializes in A/B Testing of websites. Follow Brian on Twitter @bmassey By SEO Guru| 2016-11-07T17:14:33+00:00 November […]
[…] Pour aller plus loin vous pouvez aussi consulter sur ConversionScience le son guide « Comment le A/B testing améliore les sites Internet« . […]
[…] Unexpected Website Forumulas of The Conversion Scientist. Conversion Sciences specializes in A/B Testing of websites. Follow Brian on Twitter […]
[…] point of AB testing is to make changes and be able to say with confidence that what you did caused conversion rates […]
[…] test. The image is one variable and the headline is a second variable. Technically, this is an AB test with two variables changing. If Variation (B) generated more leads, we wouldn’t know if the image […]
[…] test. The image is one variable and the headline is a second variable. Technically, this is an AB test with two variables changing. If Variation (B) generated more leads, we wouldn’t know if the […]
[…] is a great lesson in how statistical significance and sample size can build or devastate our […]
[…] every site should benefit from it. We take every chance to teach businesses about it. The AB testing process is an important part of conversion optimization and is within reach of almost any business […]
[…] This process only works if you know how to do A/B testing. […]
Leave a Reply
Leave a reply cancel reply.
Your email address will not be published. Required fields are marked *
I agree to the terms and conditions laid out in the Privacy Policy
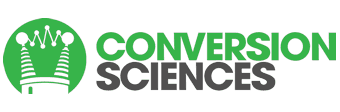
- Optimization Services
- Guaranteed Redesign
- Fully-managed CRO
- AI Optimization
- CRO Training & Coaching
- Conversion Solutions
- eCommerce Optimization
- Lead Generation Solutions
- CRO for Website Redesign
- CRO for Mobile Lead Gen
- CRO for Advertising
- About Conversion Sciences
- Success Stories
- CRO Resources
- Your Customer Creation Equation Book
- Free CRO Course
- CRO Calculator
- Press & Speaking Dates
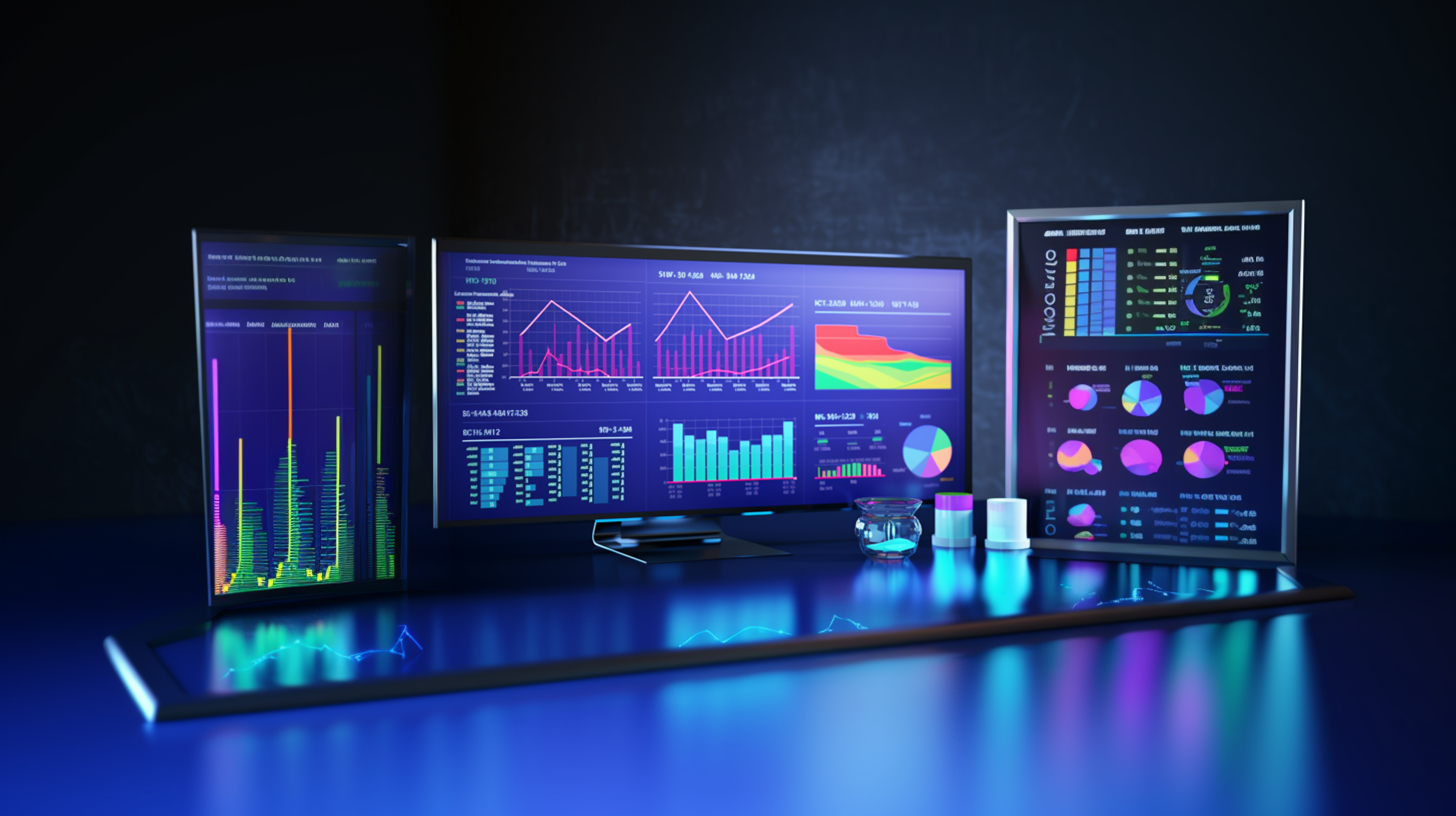
A/B Testing
This course will cover the design and analysis of A/B tests, which are online experiments used throughout tech industry by companies like Google, Amazon, and Netflix.
Last Updated March 7, 2022
Prerequisites:
No experience required
Course Lessons
Overview of a/b testing.
Learn about what A/B testing is and what it can be used for.
Policy and Ethics for Experiments
Find out how to ensure experiment participants are adequately protected, and learn what questions you should be asking regarding the ethicality of experiments.
Choosing and Characterizing Metrics
Learn how to choose and validate metrics to use in your experiment.
Designing an Experiment
Learn about how to design an A/B test.
Analyzing Results
Learn about how to analyze the results of your experiments.
Final Project
Learn about the final project and go through a series of quizzes to verify the quantitative components of your test.
Taught By The Best
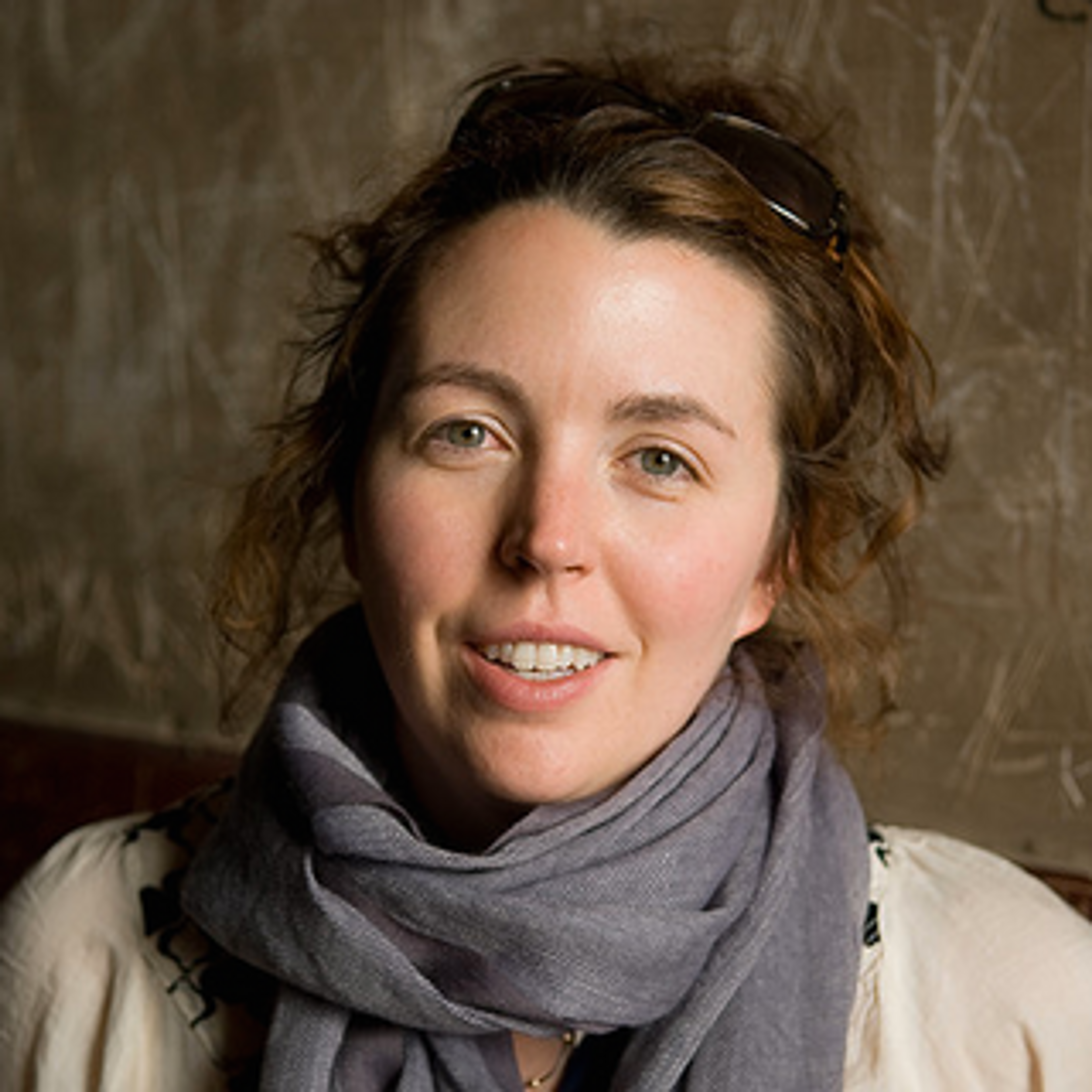
Carrie Grimes
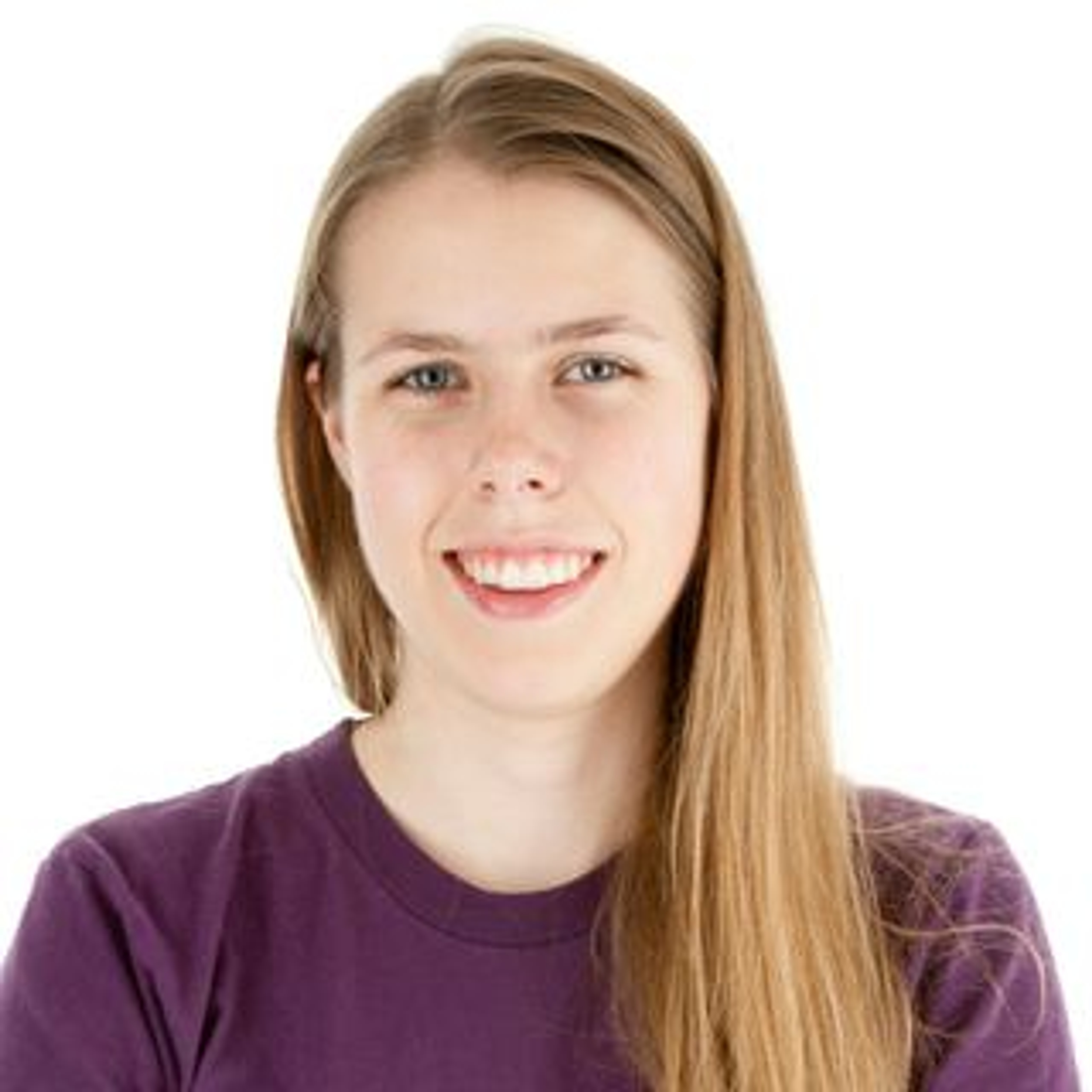
Caroline Buckey
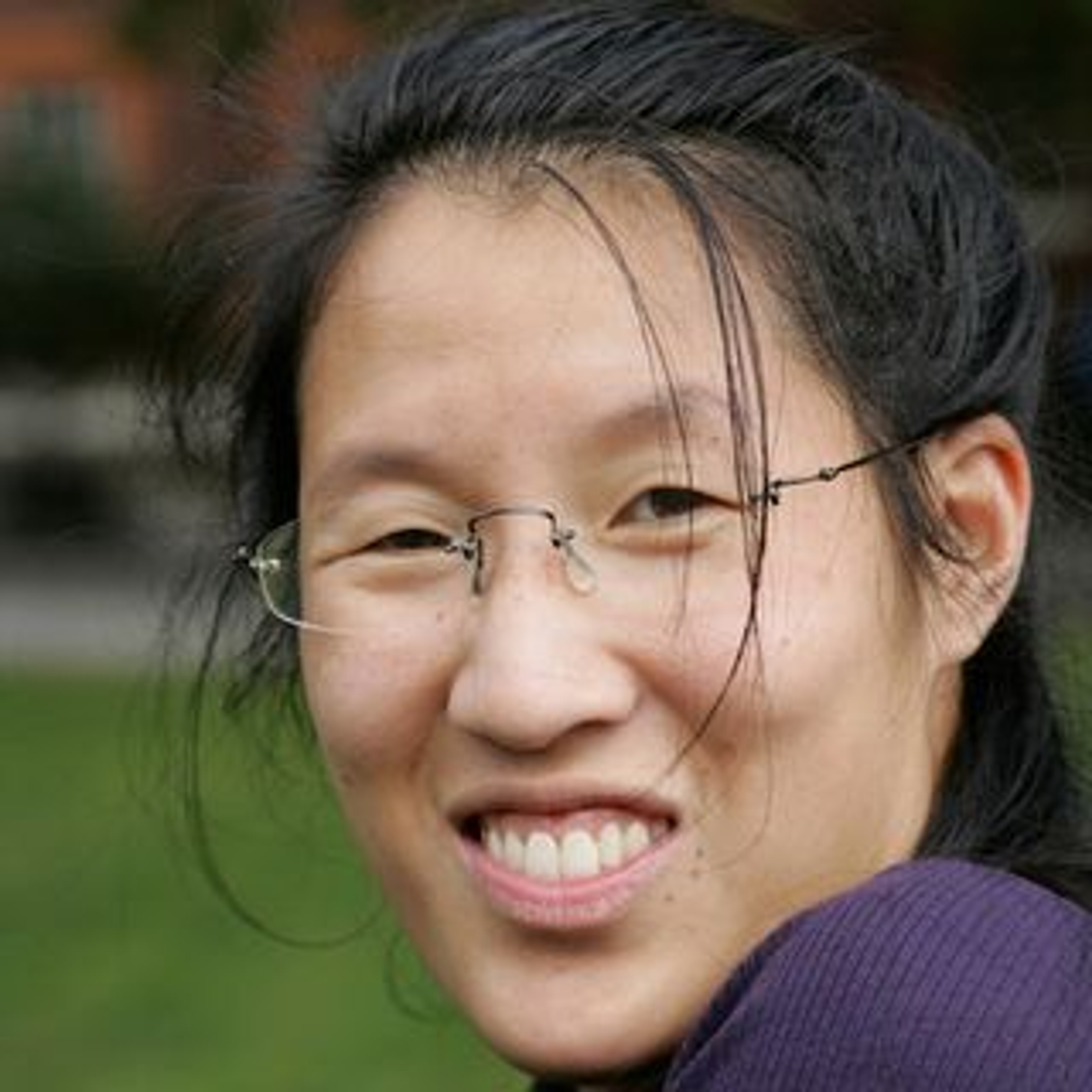
The Udacity Difference
Combine technology training for employees with industry experts, mentors, and projects, for critical thinking that pushes innovation. Our proven upskilling system goes after success—relentlessly.
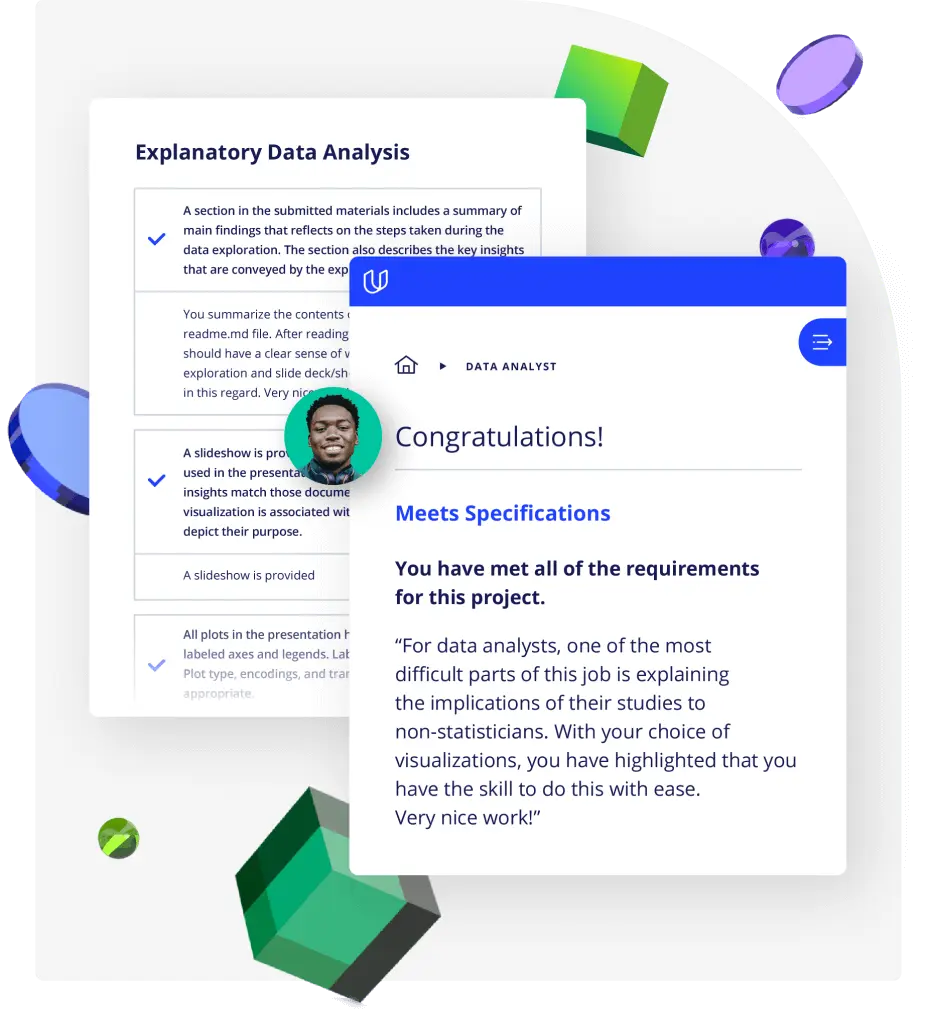
Demonstrate proficiency with practical projects
Projects are based on real-world scenarios and challenges, allowing you to apply the skills you learn to practical situations, while giving you real hands-on experience.
Gain proven experience
Retain knowledge longer
Apply new skills immediately
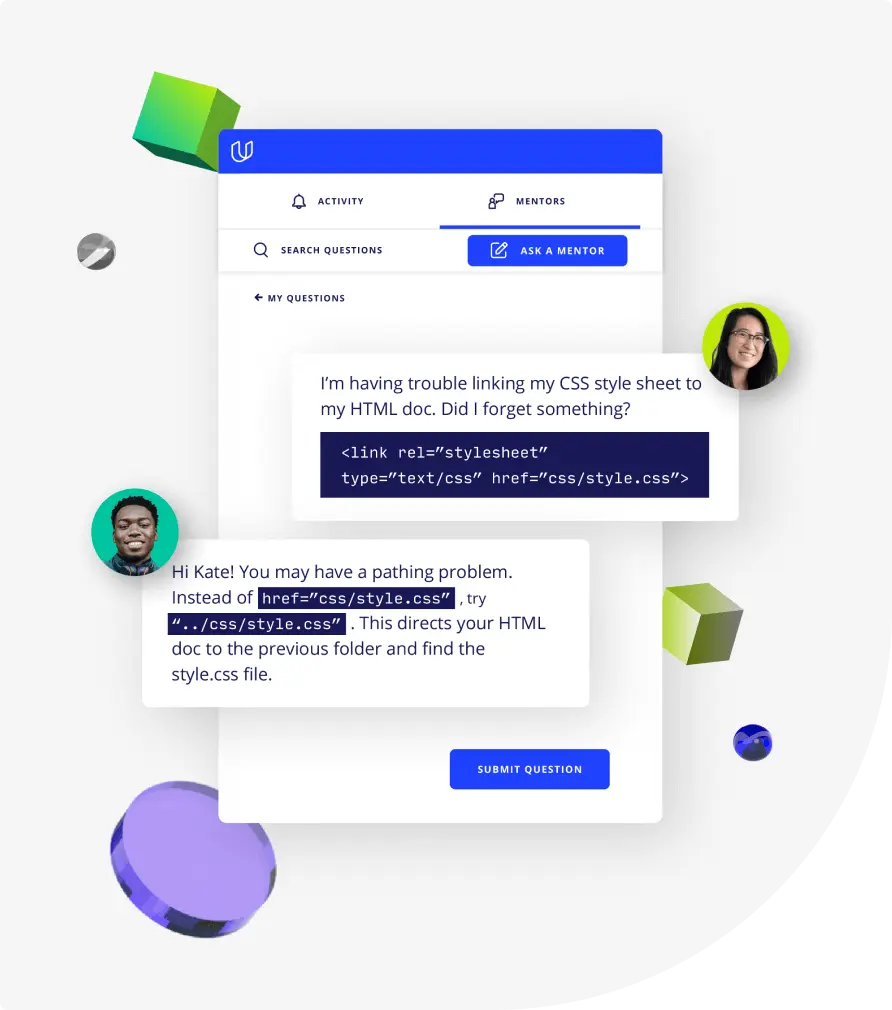
Top-tier services to ensure learner success
Reviewers provide timely and constructive feedback on your project submissions, highlighting areas of improvement and offering practical tips to enhance your work.
Get help from subject matter experts
Learn industry best practices
Gain valuable insights and improve your skills
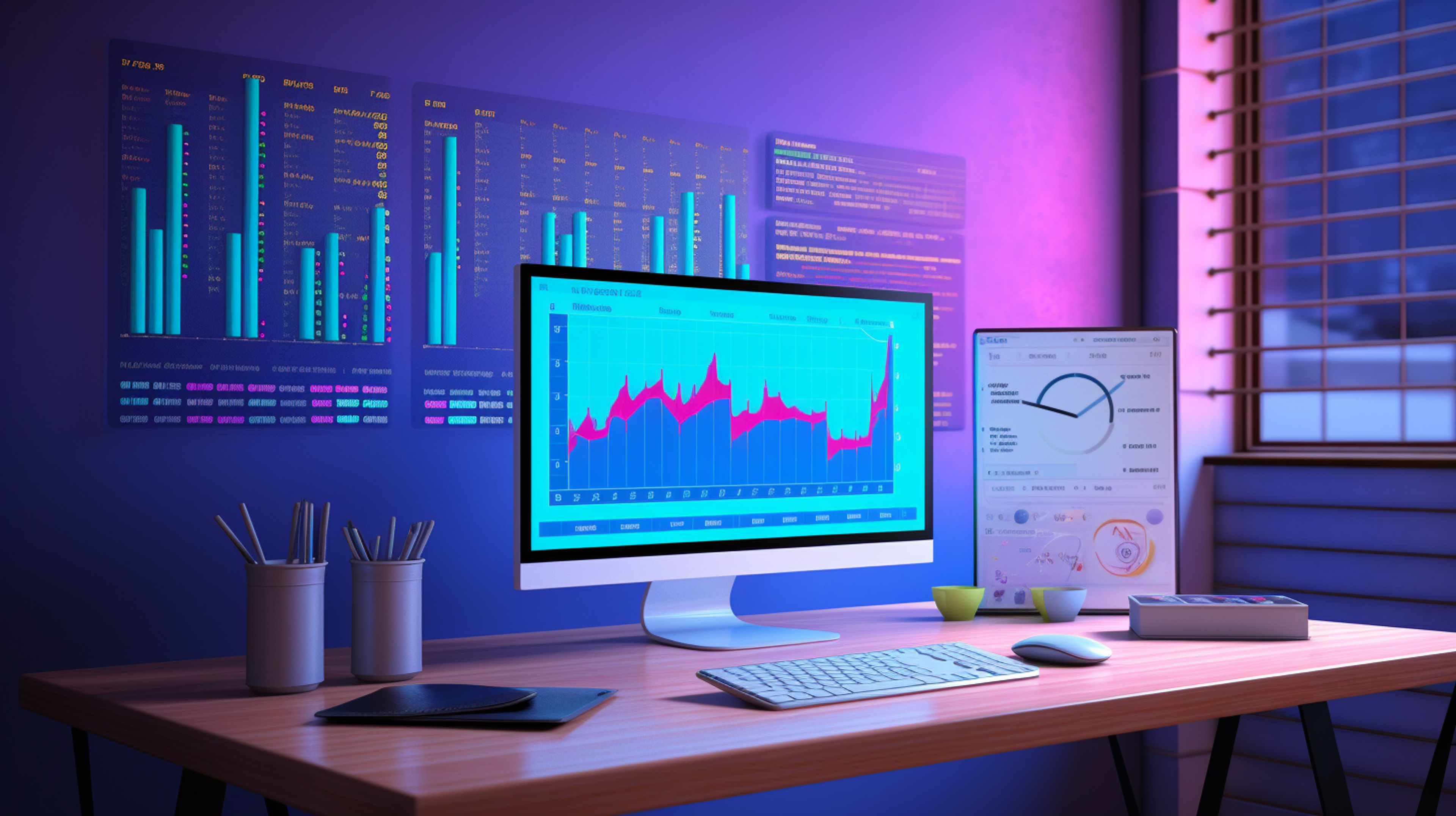
, Intermediate
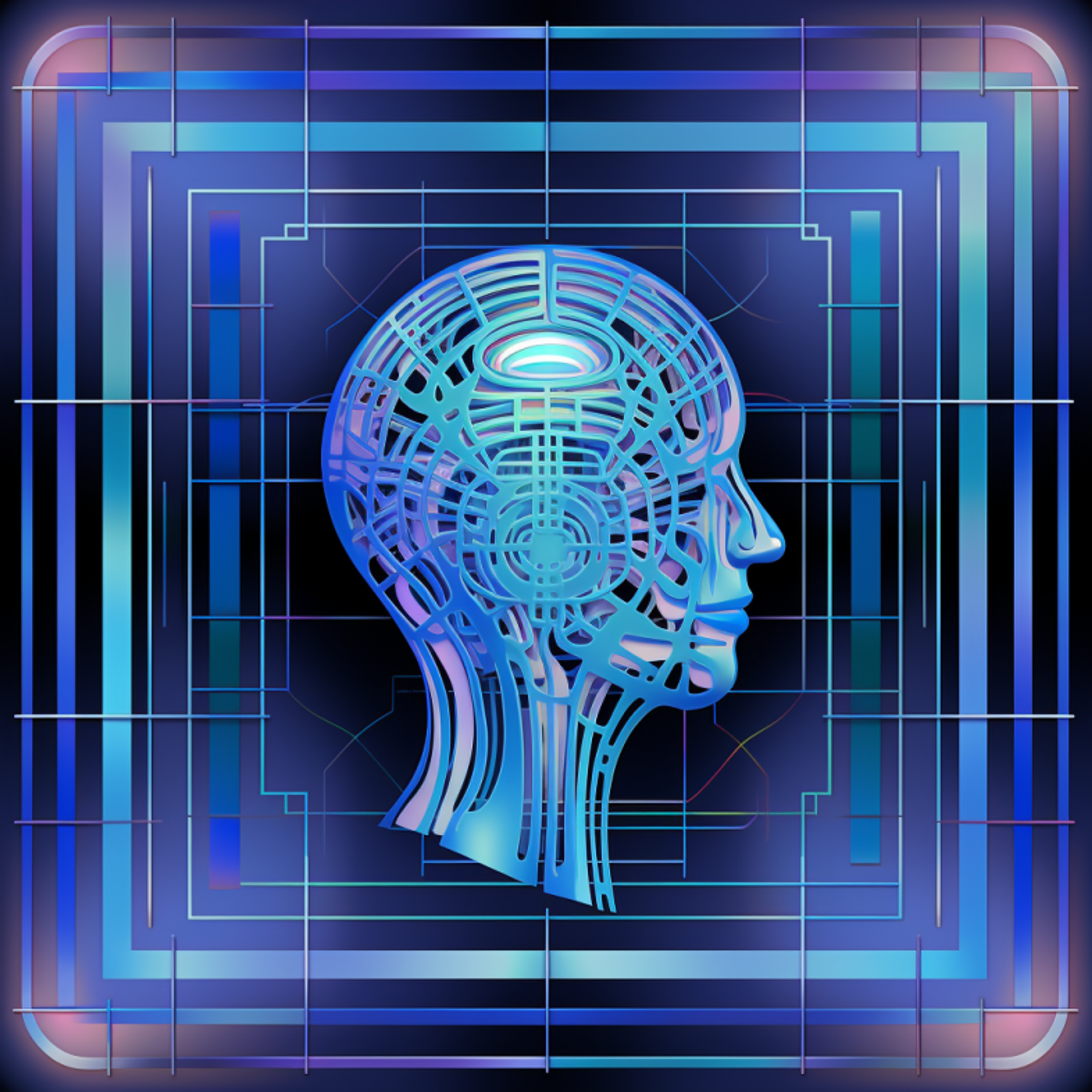
Intermediate
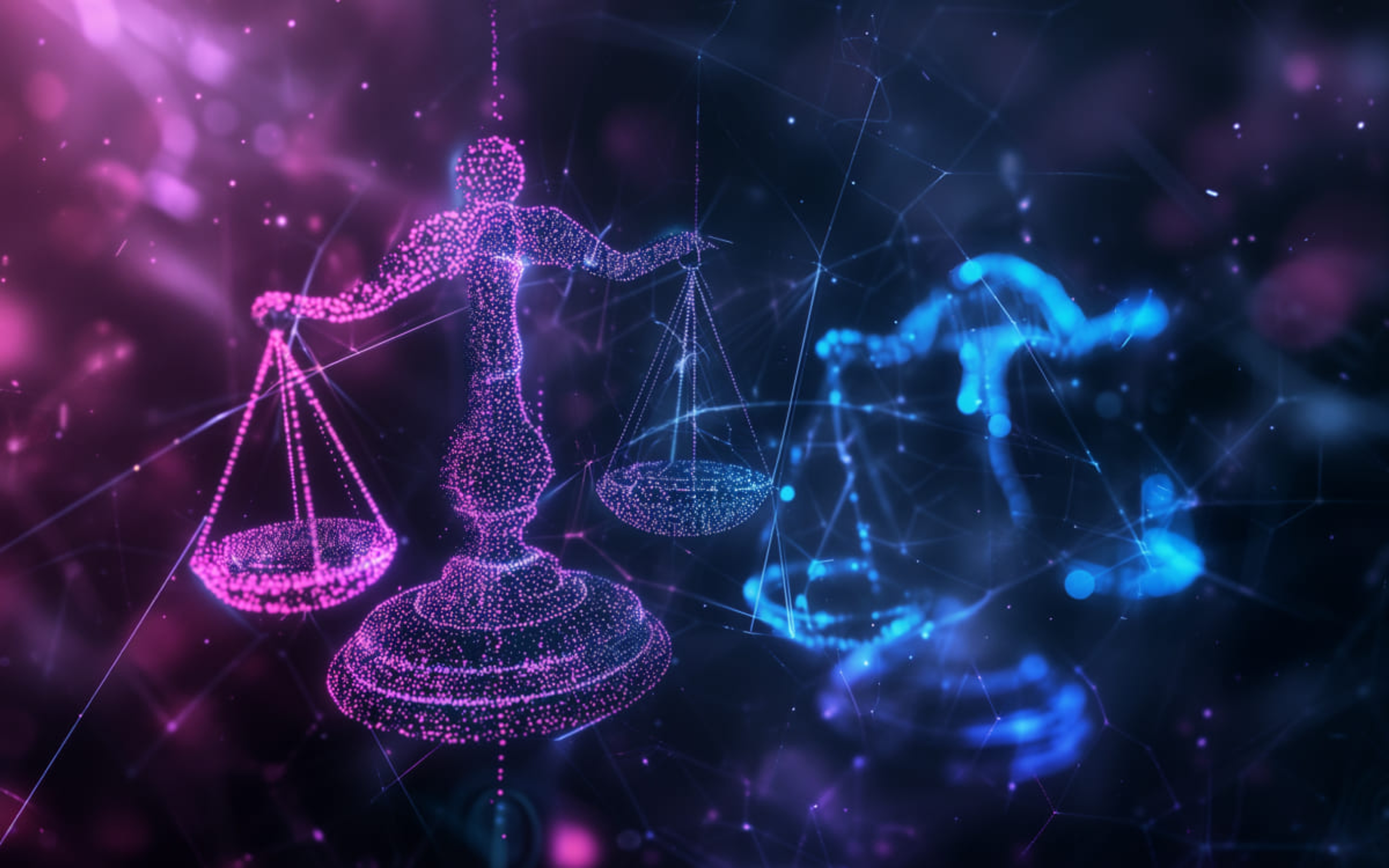
, Discovery
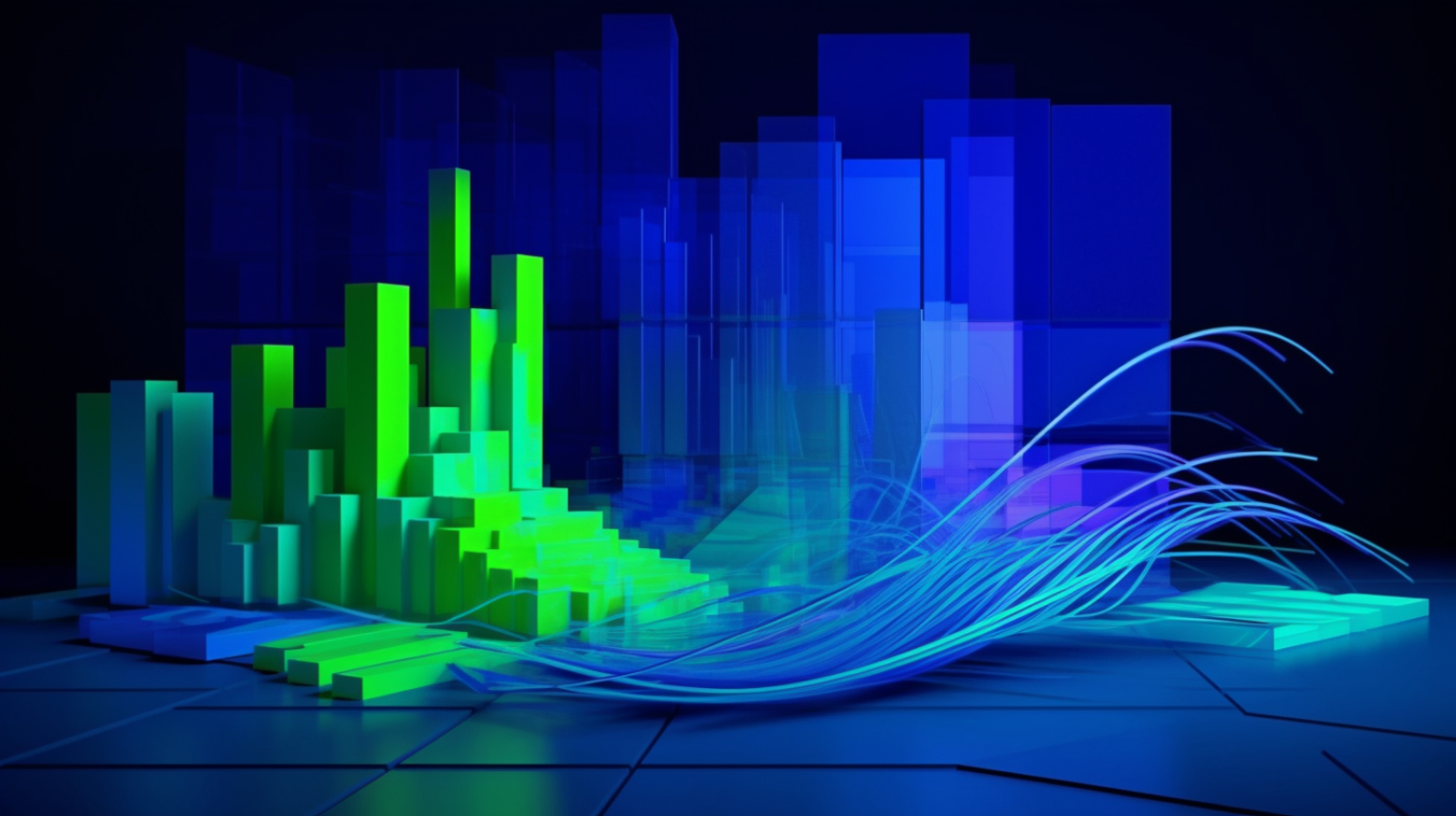
Related Programs
- +1 415-349-0105 +44 800-088-5450 +1 844-822-8378 +61 1-800-614-417
- VWO Engage Login

A/B Testing Guide
Everything you need to know about A/B Testing - from why to how, challenges to examples
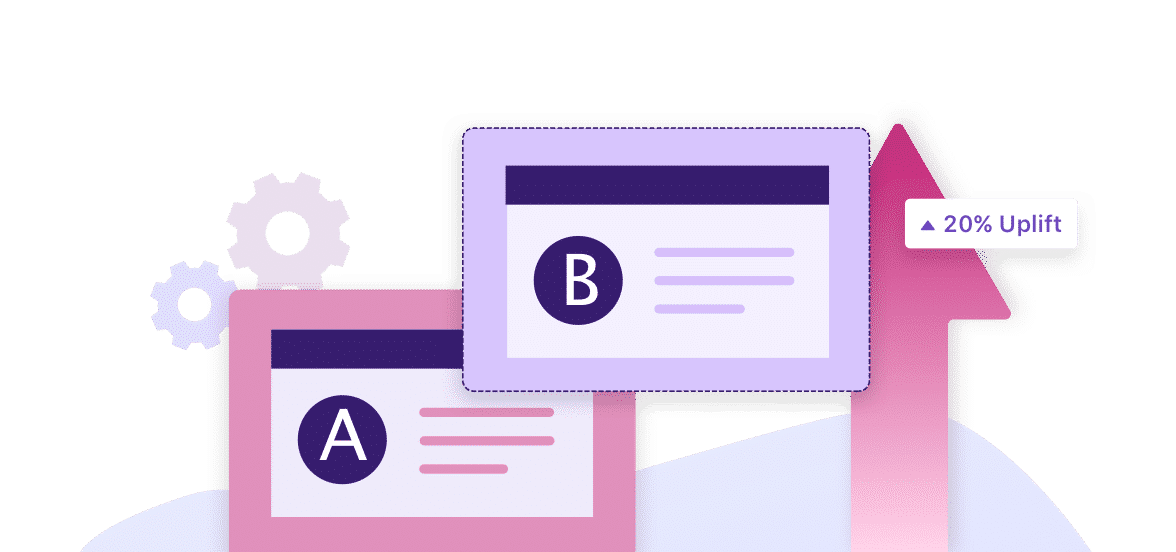
What is A/B testing?
A/B testing compares two versions of an app or webpage to identify the better performer. It’s a method that helps you make decisions based on real data rather than just guessing. It compares options to learn what customers prefer. You can test website/app layouts, email subject lines, product designs, CTA button text, colors, etc.
A/B testing, also known as split testing, refers to a randomized experimentation process wherein two or more versions of a variable (web page, page element, etc.) are shown to different segments of website visitors at the same time to determine which version leaves the maximum impact and drives business metrics.
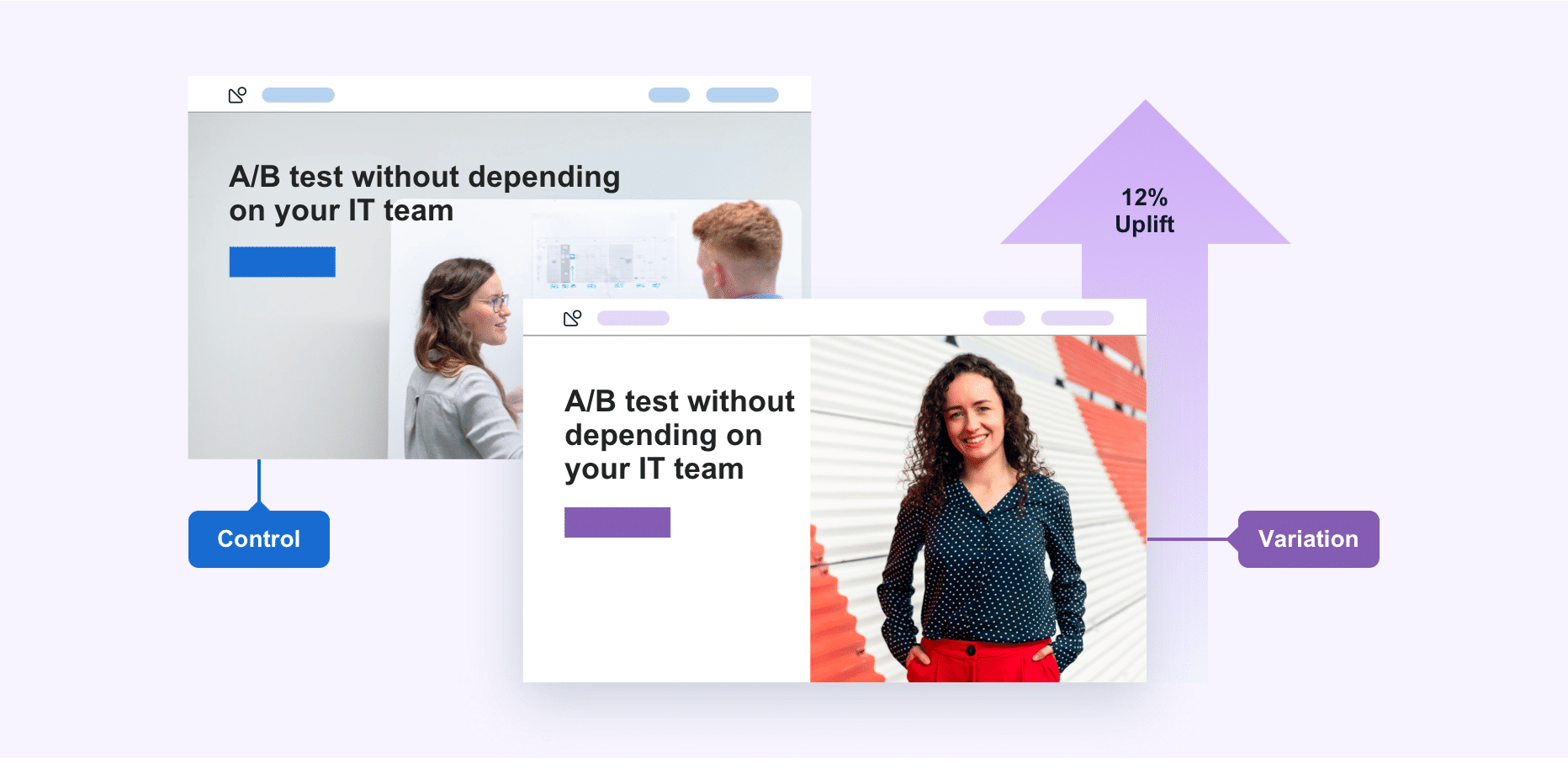
Essentially, A/B testing eliminates all the guesswork out of website optimization and enables experience optimizers to make data-backed decisions. In A/B testing, A refers to ‘control’ or the original testing variable. Whereas B refers to ‘variation’ or a new version of the original testing variable.
The version that moves your business metric(s) in the positive direction is known as the ‘winner.’ Implementing the changes of this winning variation on your tested page(s) / element(s) can help optimize your website and increase business ROI.
The metrics for conversion are unique to each website. For instance, in the case of eCommerce, it may be the sale of the products. Meanwhile, for B2B, it may be the generation of qualified leads.
A/B testing is one of the components of the overarching process of Conversion Rate Optimization (CRO) , using which you can gather both qualitative and quantitative user insights. You can further use this collected data to understand user behavior, engagement rate, pain points, and even satisfaction with website features, including new features, revamped page sections, etc. If you’re not A/B testing your website, you’re surely losing out on a lot of potential business revenue.
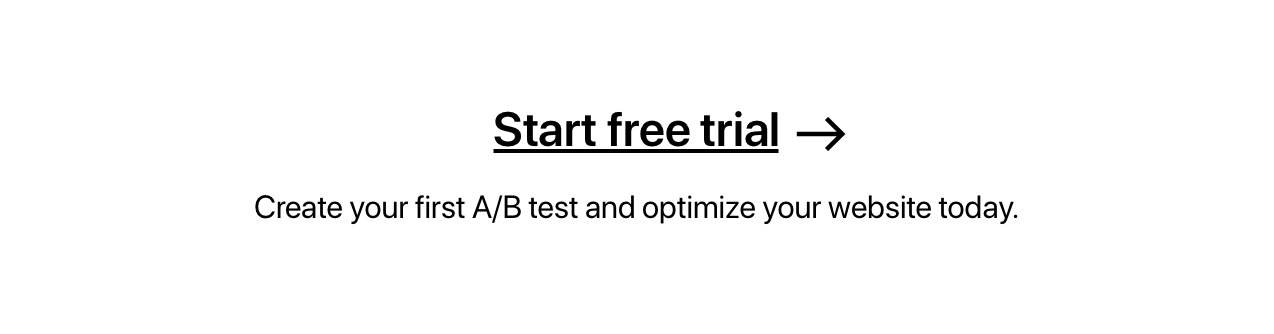
Why should you consider A/B testing?
If B2B businesses today are unhappy with all the unqualified leads they get per month, eCommerce stores, on the other hand, are struggling with a high cart abandonment rate . Meanwhile, media and publishing houses are also dealing with low viewer engagement. These core conversion metrics are affected by some common problems like leaks in the conversion funnel, drop-offs on the payment page, etc.
Let’s see why you should do A/B testing:
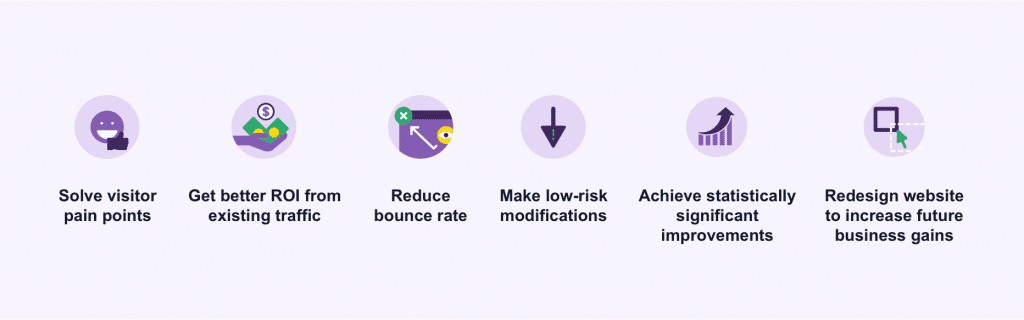
1. Solve visitor pain points
Visitors come to your website to achieve a specific goal that they have in mind. It may be to understand more about your product or service, buy a particular product, read/learn more about a specific topic, or simply browse. Whatever the visitor’s goal may be, they may face some common pain points while achieving their goal. It can be a confusing copy or hard to find the CTA button like buy now, request a demo, etc.
Not being able to achieve their goals leads to a bad user experience. This increases friction and eventually impacts your conversion rates. Use data gathered through visitor behavior analysis tools such as heatmaps, Google Analytics, and website surveys to solve your visitors’ pain points. This stands true for all businesses: eCommerce, travel, SaaS, education, media, and publishing.
2. Get better ROI from existing traffic
As most experienced optimizers have come to realize, the cost of acquiring quality traffic on your website is huge. A/B testing lets you make the most out of your existing traffic and helps you increase conversions without having to spend additional dollars on acquiring new traffic. A/B testing can give you high ROI as sometimes, even the minutest of changes on your website can result in a significant increase in overall business conversions.
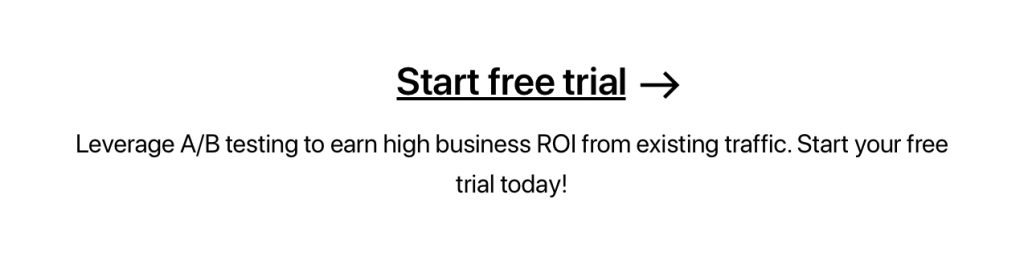
3. Reduce bounce rate
One of the most important metrics to track to judge your website’s performance is its bounce rate. There may be many reasons behind your website’s high bounce rate , such as too many options to choose from, expectations mismatch, confusing navigation, use of too much technical jargon, and so on.
Since different websites serve different goals and cater to different segments of audiences, there is no one-size-fits-all solution to reducing bounce rates. However, running an A/B test can prove beneficial. With A/B testing, you can test multiple variations of an element of your website till you find the best possible version. This not only helps you find friction and visitor pain points but helps improve your website visitors’ overall experience, making them spend more time on your site and even converting into a paying customer.
4. Make low-risk modifications
Make minor, incremental changes to your web page with A/B testing instead of getting the entire page redesigned. This can reduce the risk of jeopardizing your current conversion rate.
A/B testing lets you target your resources for maximum output with minimal modifications, resulting in an increased ROI. An example of that could be product description changes. You can perform an A/B test when you plan to remove or update your product descriptions. You do not know how your visitors are going to react to the change. By running an A/B test, you can analyze their reaction and ascertain which side the weighing scale may tilt.
Another example of low-risk modification can be the introduction of a new feature change. Before introducing a new feature, launching it as an A/B test can help you understand whether or not the new change that you’re suggesting will please your website audience.
Implementing a change on your website without testing it may or may not pay off in both the short and long run. Testing and then making changes can make the outcome more certain.
5. Achieve statistically significant improvements
Since A/B testing is entirely data-driven with no room for guesswork, gut feelings, or instincts, you can quickly determine a “winner” and a “loser” based on statistically significant improvements in metrics like time spent on the page, number of demo requests, cart abandonment rate, click-through rate, and so on.
6. Redesign the website to increase future business gains
Redesigning can range from a minor CTA text or color tweak to particular web pages to completely revamping the website . The decision to implement one version or the other should always be data-driven when A/B testing. Do not quit testing with the design being finalized. As the new version goes live, test other web page elements to ensure that the most engaging version is served to the visitors.
What can you A/B test?
Your website’s conversion funnel determines the fate of your business. Therefore, every piece of content that reaches your target audience via your website must be optimized to its maximum potential. This is especially true for elements that have the potential to influence the behavior of your website visitors and business conversion rate. When undertaking an optimization program, test the following key site elements (the list, however, is not exhaustive):
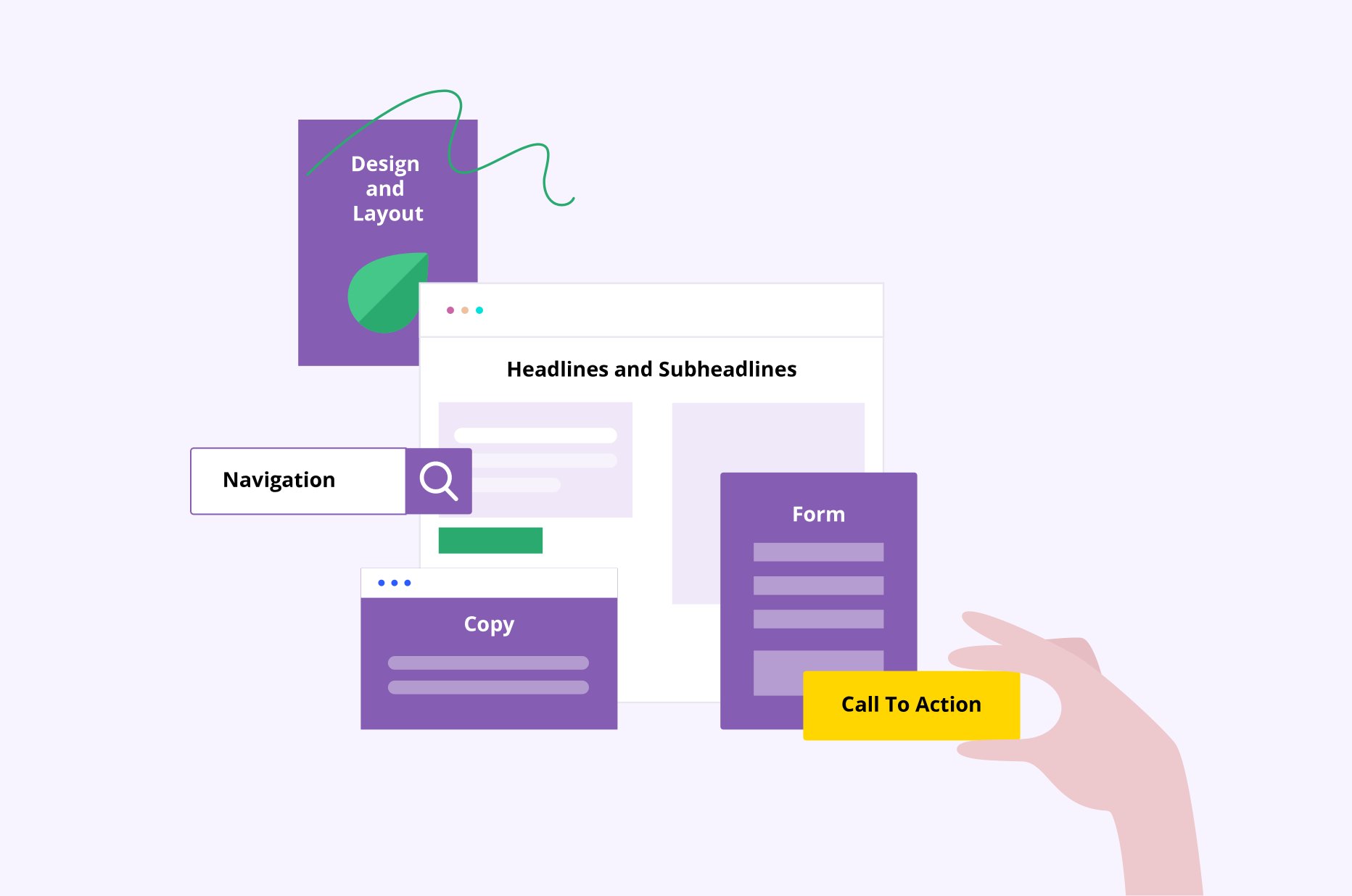
1. Headlines and subheadlines
A headline is practically the first thing that a visitor notices on a web page. It’s also what defines their first and last impression, filling in the blanks on whether or not they’ll go ahead and convert into paying customers. Hence, it’s imperative to be extra cautious about your site’s headlines and subheadlines. Ensure they’re short, to-the-point, catchy, and convey your desired message in the first stance. Try A/B testing a few copies with different fonts and writing styles, and analyze which catches your visitors’ attention the most and compels them to convert. You can also use VWO’s AI-powered text generation system to generate recommendations for the existing copy on your website.
The body or main textual content of your website should clearly state what the visitor is getting – what’s in store for them. It should also resonate with your page’s headline and subheadline. A well-written body can significantly increase the chances of turning your website into a conversion magnet.
While drafting your website’s content, keep the following two parameters in mind:
- Writing style: Use the right tonality based on your target audience. Your copy should directly address the end-user and answer all their questions. It must contain key phrases that improve usability and stylistic elements that highlight important points.
- Formatting: Use relevant headlines and subheadlines, break the copy into small and easy paragraphs, and format it for skimmers using bullet points or lists.
Interestingly, experience optimizers can now take advantage of artificial intelligence to create website copies. GPT-3.5 Turbo or Generative Pre-trained Transformer 3, is an AI-powered neural network that has the ability to produce nearly flawless text content relevant to any given context. Built by OpenAI, GPT-3.5 Turbo uses machine learning to predict and draft content just like a human . The best part? You can now integrate OpenAI’s GPT-3.5 Turbo with the VWO Testing account and create variations for your website copy and deploy them without the help of an expert writer or IT, respectively.
3. Subject lines
Email subject lines directly impact open rates. If a subscriber doesn’t see anything they like, the email will likely wind up in their trash bin.
According to recent research, average open rates across more than a dozen industries range from 25 to 47 percent. Even if you’re above average, only about half of your subscribers might open your emails.
A/B testing subject lines can increase your chances of getting people to click. Try questions versus statements, test power words against one another, and consider using subject lines with and without emojis.
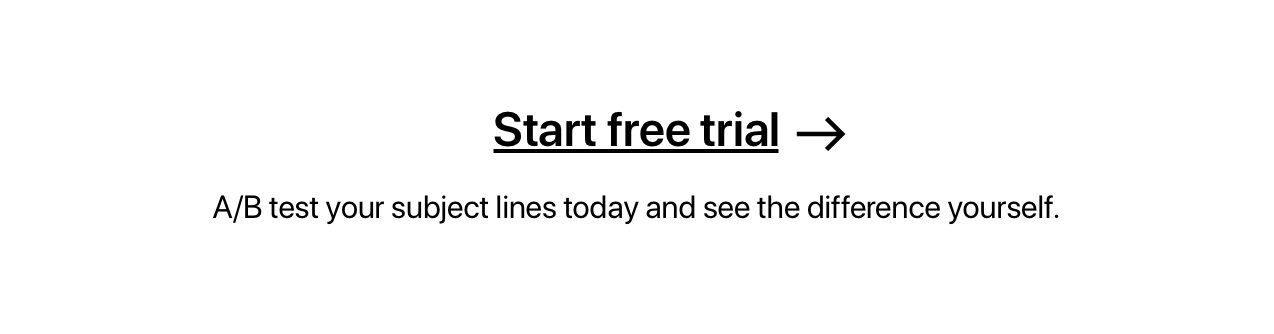
Design and layout
Because everything seems so essential, businesses sometimes struggle with finding only the most essential elements to keep on their website. With A/B testing, this problem can be solved once and for all.
For example, as an eCommerce store, your product page is extremely important from a conversion perspective. One thing for sure is that with technological progress in its current stage, customers like to see everything in high definition before buying it. Therefore, your product page must be in its most optimized form in terms of design and layout .
Along with the copy, the page’s design and layout include images (product images, offer images, etc.) and videos (product videos, demo videos, advertisements, etc.). Your product page should answer all of your visitor’s questions without confusing them and without getting cluttered:
- Provide clear information: Based on the products you sell, find creative ways to provide all necessary context and accurate product descriptions so that prospective buyers do not get overwhelmed with an unorganized copy while looking for answers to their queries. Write clear copies and provide easily noticeable size charts, color options, etc.
- Highlight customer reviews: Add both good and bad reviews for your products. Negative reviews add credibility to your store.
- Write simple content: Avoid confusing potential buyers with complicated language in the quest to decorate your content. Keep it short, simple, and fun to read.
- Create a sense of urgency: Add tags like ‘Only 2 Left In Stock’, countdowns like ‘Offer Ends in 2 Hours and 15 Minutes’, or highlight exclusive discounts and festive offers, etc., to nudge the prospective buyers to purchase immediately.
Other important pages whose design needs to be on point are pages like the home page and landing page. Use A/B testing to discover the most optimized version of these critical pages. Test as many ideas as you can, such as adding plenty of white space and high-definition images, featuring product videos instead of images, and testing out different layouts.
Declutter your pages using insights from heatmaps , clickmaps, and scrollmaps to analyze dead clicks and identify distractions. The less cluttered your home page and landing pages, the more likely it is for your visitors to easily and quickly find what they’re looking for.
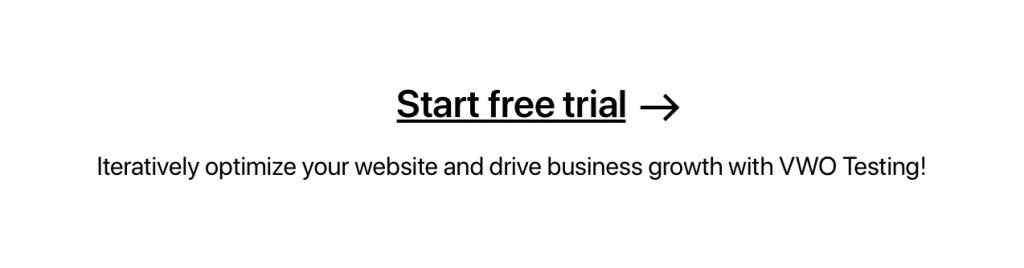
Another element of your website that you can optimize by A/B testing is your website’s navigation. It is the most crucial element when it comes to delivering an excellent user experience. Make sure you have a clear plan for your website’s structure and how different pages will be linked to each other and react within that structure.
Your website’s navigation starts on the home page. The home page is the parent page from which all other pages emerge and link back to each other. Make sure your structure is such that visitors can easily find what they’re looking for and do not get lost because of a broken navigation path. Each click should direct visitors to the desired page.
Mentioned below are some ideas to help you step up your navigation game:
- Match visitor expectations by placing your navigation bar in standard places like horizontal navigation on the top and vertical down the left to make your website easier to use.
- Make your website’s navigation predictable by keeping similarly themed content in the same bucket or in related buckets to reduce your visitor’s cognitive load. For example, as an eCommerce store, you may be selling a variety of earphones and headphones. Some of them may be wired, while others may be wireless or ear-pods. Bucket these in such a way that when a visitor looks for earphones or headphones, they find all these varieties in one place rather than having to search for each kind separately
- Creating a fluid, easy-to-navigate website by keeping its structure simple, predictable, and matching your visitors’ expectations. This will not only increase the chances of getting more conversions but also create a delightful customer experience forcing visitors to come back to your website.
Forms are mediums through which prospective customers get in touch with you. They become even more important if they are part of your purchase funnel. Just as no two websites are the same, no two forms addressing different audiences are the same. While a small comprehensive form may work for some businesses, long forms might do wonders for their lead quality for other businesses.
You can figure out which style works for your audience the best by using research tools/methods like form analysis to determine the problem area in your form and work towards optimizing it.
CTA (Call-to-action)
The CTA is where all the real action takes place – whether or not visitors finish their purchases and convert if they fill out the sign-up form or not, and more such actions that have a direct bearing on your conversion rate. A/B testing enables you to test different CTA copies, their placement across the web page, toy with their size and color scheme, and so on. Such experimentation helps understand which variation has the potential to get the most conversions.
Social proof
Social proof may take the form of recommendations and reviews from experts in particular fields, from celebrities and customers themselves, or can come as testimonials, media mentions, awards and badges, certificates, and so on. The presence of these proofs validates the claims made by your website. A/B testing can help you determine whether or not adding social proof is a good idea. If it is a good idea, what kinds of social proof should you add, and how many should you add? You can test different types of social proofs, their layouts, and placements to understand which works best in your favor.
Content depth
Some website visitors prefer reading long-form content pieces that extensively cover even the minutest of details. Meanwhile, many others just like to skim through the page and deep dive only into the topics that are most relevant to them. In which category does your target audience fall?
A/B test content depth. Creating two pieces of the same content, one that’s significantly longer than the other, provides more details. Analyze which compels your readers the most.
Understand that content depth impacts SEO and many other business metrics such as the conversion rate, page time spent, and bounce rate. A/B testing enables you to find the ideal balance between the two.
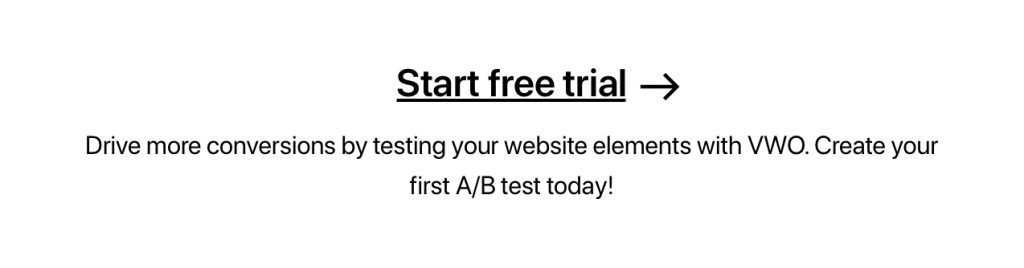
What are the different types of A/B tests?
Post learning about which web page elements to test to move your business metrics in the positive direction, let’s move ahead and learn about the different kinds of testing methods along with their advantages.
Ideally, there are four basic testing methods – A/B testing, Split URL testing, Multivariate testing, and Multipage testing. We’ve already discussed the first kind, namely, A/B testing. Let’s move on to the others.
Split URL testing
Many people in the testing arena confuse Split URL testing with A/B testing. However, the two are fundamentally very different. Split URL testing refers to an experimentation process wherein an entirely new version of an existing web page URL is tested to analyze which one performs better.
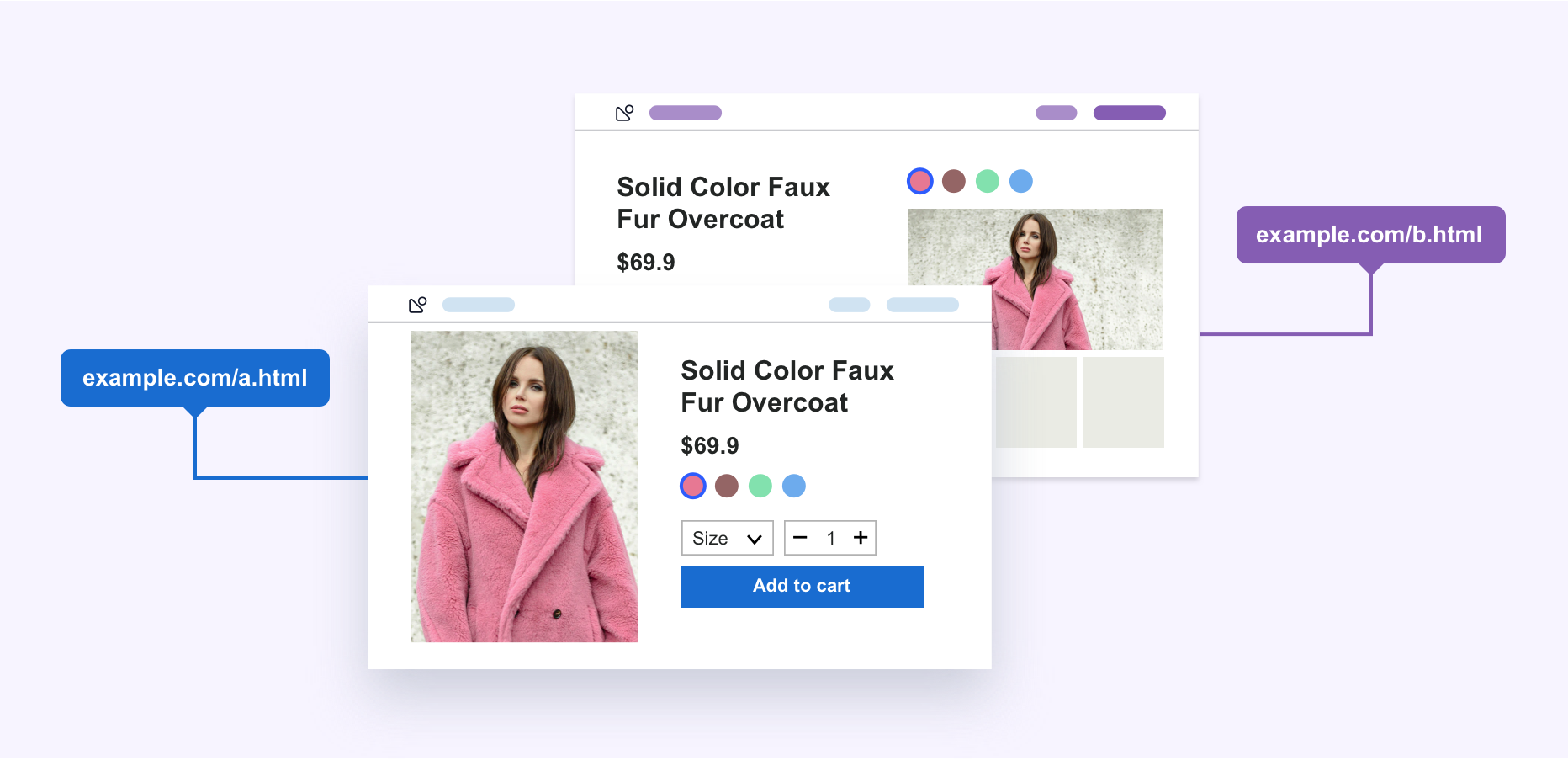
Typically, A/B testing is used when you wish to only test front-end changes on your website. On the other hand, Split URL testing is used when you wish to make significant changes to your existing page, especially in terms of design. You’re not willing to touch the existing web page design for comparison purposes.
When you run a Split URL test, your website traffic is split between the control (original web page URL) and variations (new web page URL), and each of their respective conversion rates is measured to decide the winner.
Advantages of Split URL testing
- Ideal for trying out radical new designs while using the existing page design for comparative analysis.
- Recommended for running tests with non-UI changes, such as switching to a different database, optimizing your page’s load time, etc.
- Change up web page workflows. Workflows dramatically affect business conversions, helping test new paths before implementing changes and determining if any of the sticking points were missed.
- A better and much-recommended testing method for dynamic content.
Multivariate testing (MVT)
Multivariate testing (MVT) refers to an experimentation method wherein variations of multiple-page variables are simultaneously tested to analyze which combination of variables performs the best out of all the possible permutations. It’s more complicated than a regular A/B test and is best suited for advanced marketing, product, and development professionals.
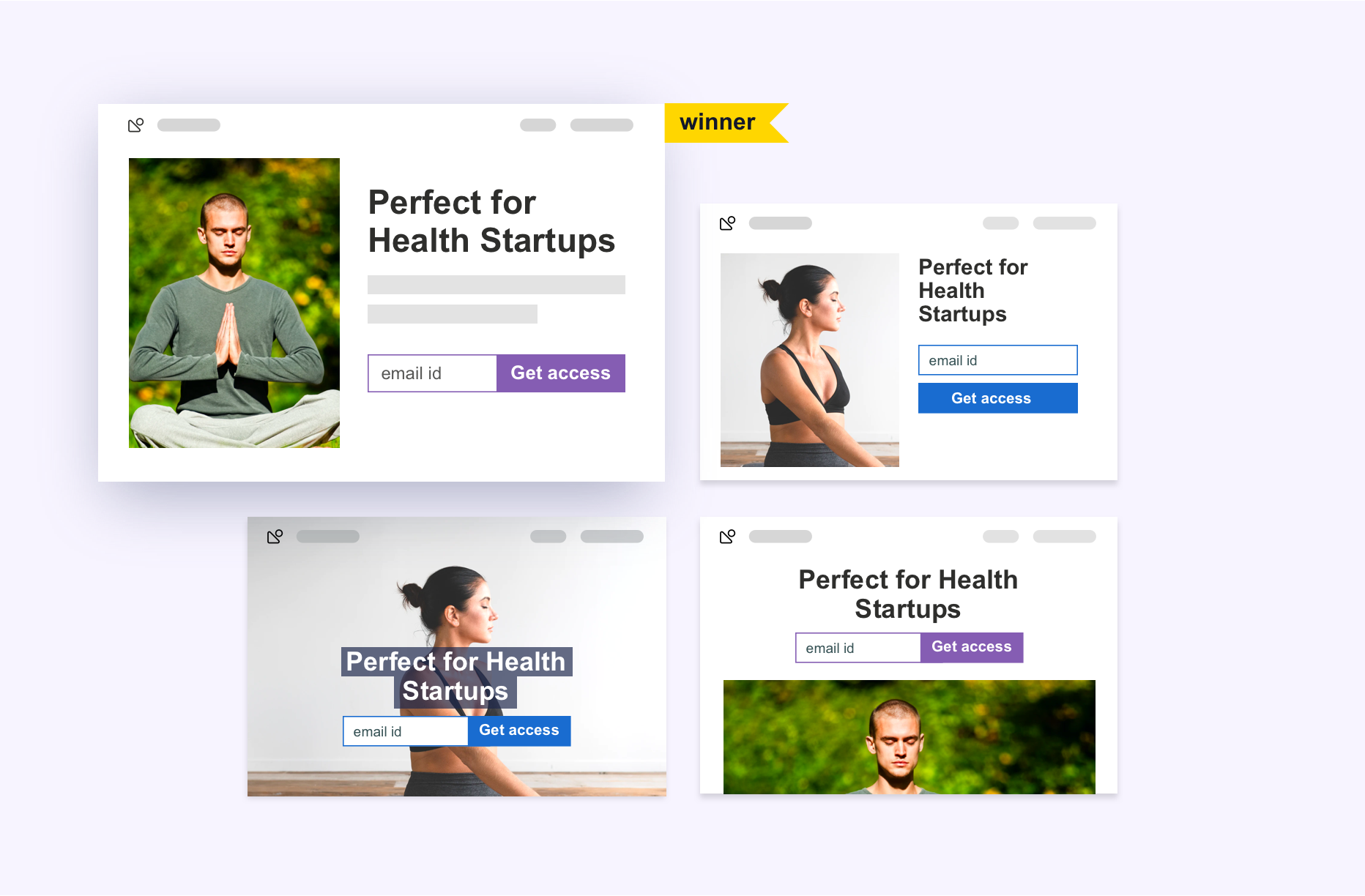
Here’s an example to give you a more comprehensive description of multivariate testing. Let’s say you decide to test 2 versions, each of the hero image, call-to-action button color, and headlines of one of your landing pages. This means a total of 8 variations are created, which will be concurrently tested to find the winning variation.
Here’s a simple formula to calculate the total number of versions in a multivariate test:
[No. of variations of element A] x [No. of variations of element B] x [No. of variations of element C]… = [Total No. of variations]
When conducted properly, multivariate testing can help eliminate the need to run multiple and sequential A/B tests on a web page with similar goals. Running concurrent tests with a greater number of variations helps you save time, money, and effort and come to a conclusion in the shortest possible time.
Advantages of Multivariate testing
Multivariate testing typically offers primary three benefits:
- Helps avoid the need to conduct several sequential A/B tests with the same goal and saves time since you can simultaneously track the performance of various tested page elements.
- Easily analyze and determine the contribution of each page element to the measured gains,
- Map all the interactions between all independent element variations (page headlines, banner images, etc.).
Multipage testing
Multipage testing is a form of experimentation where you can test changes to particular elements across multiple pages.
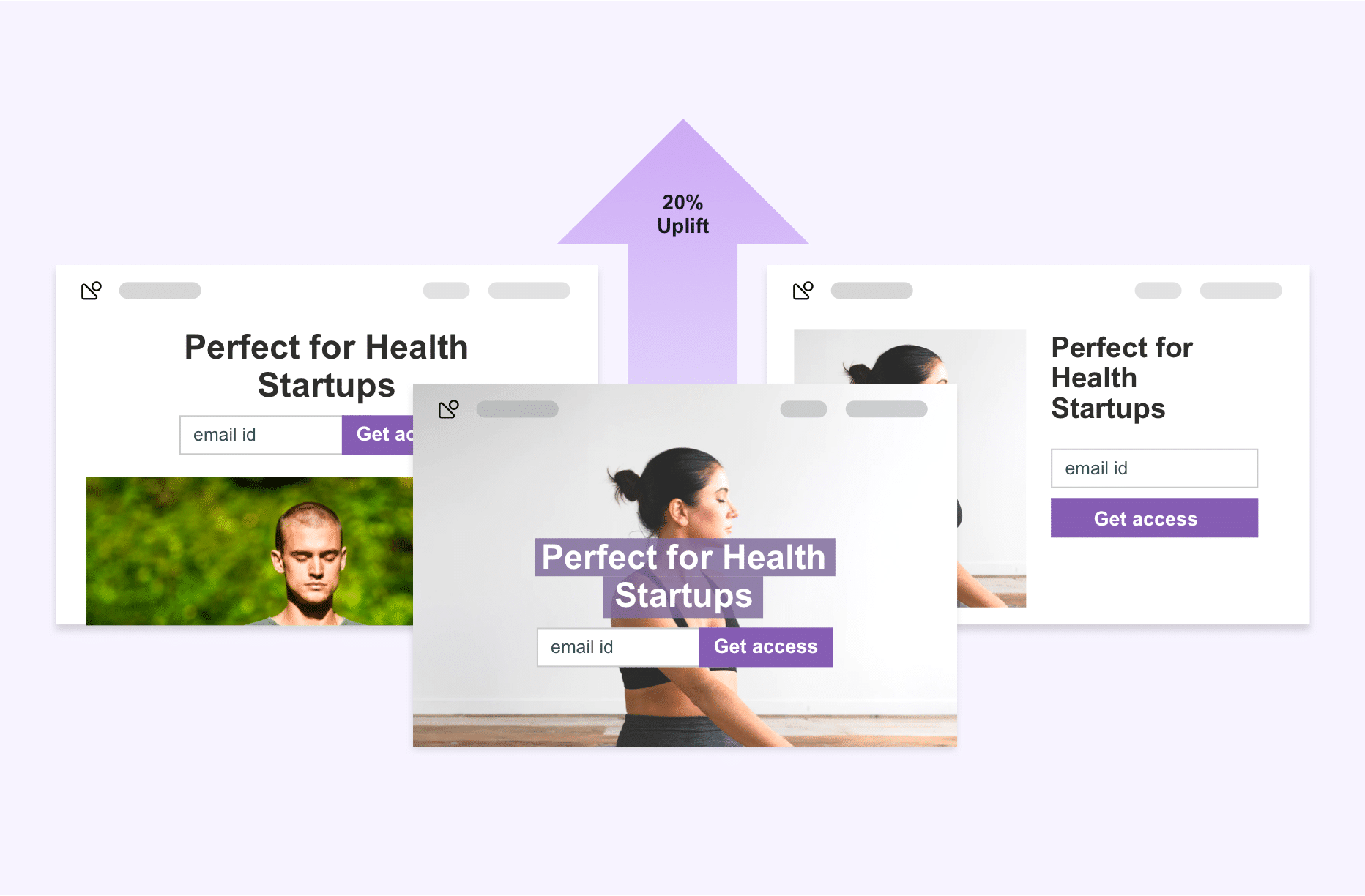
There are two ways to conduct a multipage test. One, you can either take all the pages of your sales funnel and create new versions of each, which makes your challenger the sales funnel, and you then test it against the control. This is called Funnel Multipage testing .
Two, you can test how the addition or removal of recurring element(s), such as security badges, testimonials, etc., can impact conversions across an entire funnel. This is called Classic or Conventional Multipage testing .
Advantages of Multipage testing
Similar to A/B testing, Multipage testing is easy to create and run and provides meaningful and reliable data with ease and in the shortest possible time.
The advantages of multipage testing are as follows:
- It enables you to create consistent experiences for your target audience.
- It helps your target audience see a consistent set of pages, no matter if it’s the control or one of its variations.
- It enables you to implement the same change on several pages to ensure that your website visitors don’t get distracted and bounce off between different variations and designs when navigating through your website.
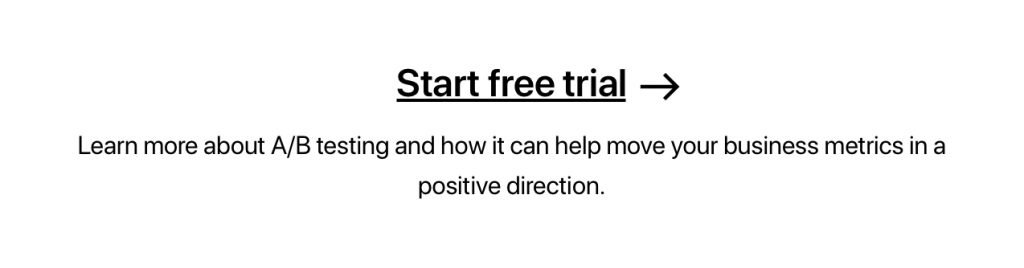
Which statistical approach to use to run an A/B test?
Post learning about four different types of A/B testing experimentation methods, it’s equally important to understand which statistical approach to adopt to successfully run an A/B test and draw the right business conclusion.
Ideally, there are two types of statistical approaches used by A/B/n experimenters across the globe: Frequentist and Bayesian. Each of these approaches has its own pros and cons. However, we, at VWO, use, support, and promote the Bayesian approach.
The comparison between the two approaches given below will help you understand why.
Frequentist approach:
The frequentist approach of probability defines the probability of an event with relation to how frequently (hence the name) a particular event occurs in a large number of trials/data points. When applied to the world of A/B testing, one can see that anyone going with the frequentist approach would need more data (a function of more number of visitors tested and over longer durations) to come to the right conclusions. This is something that limits you in scaling up any A/B testing effort. According to the Frequentist approach, it is essential to define your A/B test’s duration based on sample size to reach the right test conclusions. The tests are based on the fact that every experiment can be repeated infinite times.
Following this approach calls for a lot of attention to detail for every test that you run because for the same set of visitors, you’ll be forced to run longer duration tests than the Bayesian approach. Hence, each test needs to be treated with extreme care because there are only a few tests that you can run in a given timeframe. Unlike Bayesian statistics, the Frequentist approach is less intuitive and often proves difficult to understand.
Bayesian approach:
As compared to the Frequentist approach, Bayesian statistics is a theory-based approach that deals with the Bayesian interpretation of probability , where probability is expressed as a degree of belief in an event. In other words, the more you know about an event, the better and faster you can predict the end outcomes. Rather than being a fixed value, probability under Bayesian statistics can change as new information is gathered. This belief may be based on past information such as the results of previous tests or other information about the event.
Unlike the frequentist approach, the Bayesian approach provides actionable results almost 50% faster while focusing on statistical significance . At any given point, provided you have enough data at hand, the Bayesian approach tells you the probability of variation A having a lower conversion rate than variation B or the control. It does not have a defined time limit attached to it, nor does it require you to have an in-depth knowledge of statistics.
In the simplest of terms, the Bayesian approach is akin to how we approach things in everyday life. For instance, you misplaced your mobile phone in your house. As a frequentist, you would only use a GPS tracker to track it and only check the area the tracker is pointing to. While as a Bayesian, you will not only use a GPS tracker but also check all the places in the house you earlier found your misplaced phone. In the former, the event is considered a fixed value, while in the latter, all past and future knowledge are utilized to locate the phone.
To get a clearer understanding of the two statistical approaches, here’s a comparison table just for you:
Frequentist Statistics follow the ‘Probability as Long-Term Frequency’ definition of probability. | Bayesian Statistics follow the notions of ‘Probability as Degree of Belief’ and ‘Logical Probability.’ |
In this approach, you only use data from your current experiment. The frequentist solution is to conduct tests and draw conclusions. | In this approach, you use your prior knowledge from the previous experiments and try to incorporate that information into your current data. The Bayesian solution is to use existing data to draw conclusions. |
Give an estimated mean (and standard deviation) of samples where A beats B but completely ignores the cases when B beats A. | It takes into account the possibility of A beating B and also calculates the range of the improvement you can expect. |
Requires the test to run for a set period to get correct data from it but can’t figure out how close or far A and B actually are. It fails to tell you the probability of A beating B. | Gives you more control over testing. You can now plan better, have a more accurate reason to end tests, and get into the nitty-gritty of how close or far apart A and B are. |
Once you’ve figured out which testing method and statistical approach you wish to use, it’s time to learn the art and science of performing A/B tests on VWO’s A/B testing platform .
How to perform an A/B test?
A/B testing offers a very systematic way of finding out what works and what doesn’t work in any given marketing campaign. Most marketing efforts are geared toward driving more traffic . As traffic acquisition becomes more difficult and expensive, it becomes paramount to offer your users the best experience who comes to your website. This will help them achieve their goals and allow them to convert in the fastest and most efficient manner possible. A/B testing in marketing allows you to make the most out of your existing traffic and increase revenue inflow.
A structured A/B testing program can make marketing efforts more profitable by pinpointing the most crucial problem areas that need optimization. A/B testing is now moving away from being a standalone activity that is conducted once in a blue moon to a more structured and continuous activity, which should always be done through a well-defined CRO process. Broadly, it includes the following steps:
Step 1: Research
Before building an A/B testing plan, one needs to conduct thorough research on how the website is currently performing. You will have to collect data on everything related to how many users are coming onto the site, which pages drive the most traffic, the various conversion goals of different pages, etc. The A/B testing tool s used here can include quantitative website analytics tools such as Google Analytics, Omniture, Mixpanel, etc., which can help you figure out your most visited pages, pages with most time spent, or pages with the highest bounce rate. For example, you may want to start by shortlisting pages that have the highest revenue potential or the highest daily traffic. Following this, you may want to dive deeper into the qualitative aspects of this traffic.
Heatmap tools are the leading technology used to determine where users are spending the most time, their scrolling behavior, etc. This can help you identify problem areas on your website. Another popular tool used to do more insightful research is website user surveys. Surveys can act as a direct conduit between your website team and the end user and often highlight issues that may be missed in aggregate data.
Further, qualitative insights can be derived from session recording tools that collect data on visitor behavior, which helps in identifying gaps in the user journey. In fact, session recording tools combined with form analysis surveys can uncover insights on why users may not be filling your form. It may be due to some fields that ask for personal information or users, maybe abandoning your forms for too long.
As we can see, both quantitative and qualitative research can help us prepare for the next step in the process, making actionable observations for the next steps.
Step 2: Observe and formulate hypothesis
Get closer to your business goals by logging research observations and creating data-backed hypotheses aimed at increasing conversions. Without these, your test campaign is like a directionless compass. The qualitative and quantitative research tools can only help you with gathering visitor behavior data. It is now your responsibility to analyze and make sense of that data. The best way to utilize every bit of data collated is to analyze it, make keen observations on them, and then draw websites and user insights to formulate data-backed hypotheses. Once you have a hypothesis ready, test it against various parameters such as how much confidence you have of it winning, its impact on macro goals, and how easy it is to set up, and so on.
While brainstorming new testing ideas, if you ever find yourself facing a creativity block, don’t worry—VWO has a solution for you. You can now get AI-generated testing ideas within minutes.
The webpage URL you entered is scanned to show personalized testing ideas for that page. For instance, if you enter the URL of your pricing page, you will be presented with several relevant ideas supported by correct hypotheses, valid scientific principles, and feasible actionables. Additionally, you can generate testing ideas based on a specific goal for that page.
For example, if your objective is to ‘Increase clicks on the contact sales team CTA,’ you will see relevant ideas to help you achieve that goal. You can then add these testing ideas to VWO Plan and create a robust pipeline of tests to be carried out in the future. Swiftly jotting down recommendations and aligning them with specific goals can help save time and accelerate the testing process.
Step 3: Create variations
The next step in your testing program should be to create a variation based on your hypothesis, and A/B test it against the existing version (control). A variation is another version of your current version with changes that you want to test. You can test multiple variations against the control to see which one works best. Create a variation based on your hypothesis of what might work from a UX perspective. For example, enough people not filling forms? Does your form have too many fields? Does it ask for personal information? Maybe you can try a variation with a shorter form or another variation by omitting fields that ask for personal information.
Step 4: Run test
Before we get to this step, it’s important to zero upon the type of testing method and approach you want to use. Once you’ve locked down on either one of these types and approaches based (refer to the above-written chapters) on your website’s needs and business goals, kick off the test and wait for the stipulated time for achieving statistically significant results. Keep one thing in mind – no matter which method you choose, your testing method and statistical accuracy will determine the end results.
For example, one such condition is the timing of the test campaign. The timing and duration of the test have to be on point. Calculate the test duration keeping in mind your average daily and monthly visitors, estimated existing conversion rate, minimum improvement in conversion rate you expect, number of variations (including control), percentage of visitors included in the test, and so on.
Use our Bayesian Calculator to calculate the duration for which you should run your A/B tests for achieving statistically significant results.
Step 5: Analyse results and deploy changes
Even though this is the last step in finding your campaign winner, analysis of the results is extremely important. Because A/B testing calls for continuous data gathering and analysis, it is in this step that your entire journey unravels. Once your test concludes, analyze the test results by considering metrics like percentage increase, confidence level, direct and indirect impact on other metrics, etc. After you have considered these numbers, if the test succeeds, deploy the winning variation. If the test remains inconclusive, draw insights from it, and implement these in your subsequent tests.
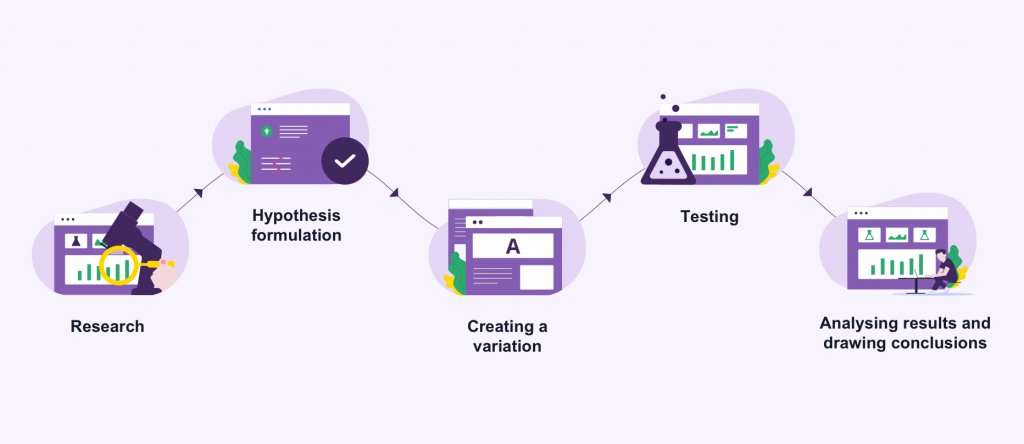
A/B testing lets you systematically work through each part of your website to improve conversions.
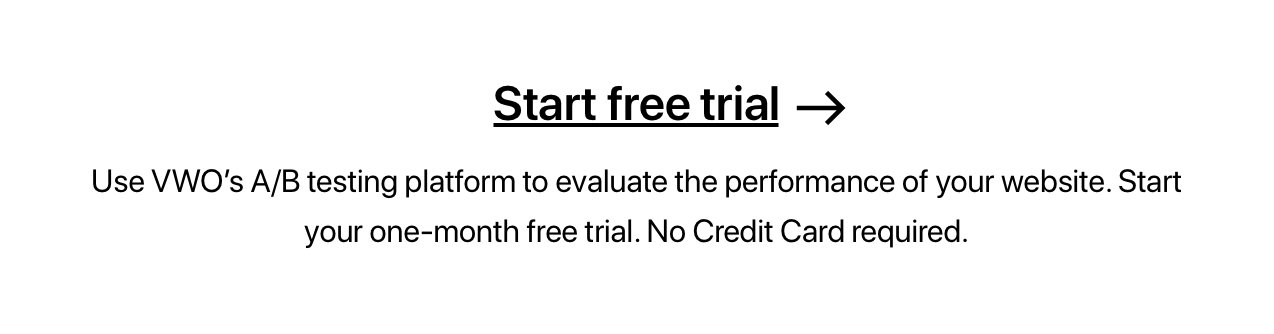
How to make an A/B testing calendar – plan & prioritize
A/B testing should never be considered an isolated optimization exercise. It’s a part of a wider holistic CRO program and should be treated as such. An effective optimization program typically has two parts, namely, plan and prioritize. Waking up one day and deciding to test your website is not how things are done in CRO. A good amount of brainstorming, along with real-time visitor data, is the only way to go about it.
In plain words, you begin by analyzing existing website data and gathering visitor behavior data, then move on to preparing a backlog of action items based on them, further prioritizing each of these items, running tests, and then drawing insights for the future. Eventually, when, as experience optimizers, you conduct enough ad-hoc based tests, you would want to scale your A/B testing program to make it more structured.
The first step to doing this is by making an A/B testing calendar. A good testing calendar or a good CRO program will take you through 4 stages:
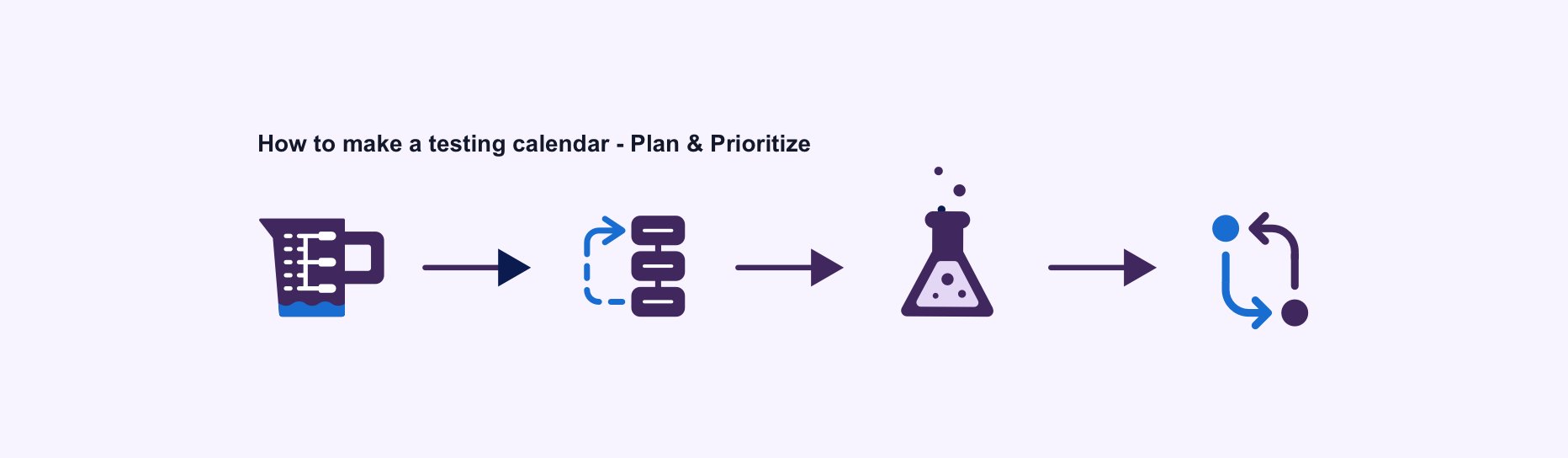
Stage 1: Measure
This stage is the planning stage of your A/B testing program. It includes measuring your website’s performance in terms of how visitors are reacting to it. In this stage, you should be able to figure out what is happening on your website, why it is happening, and how visitors are reacting to it. Everything that goes on in your website should correspond to your business goals. So before everything else, you need to be sure what your business goal/s is (are). Tools like Google Analytics can help you measure your goals. Once you have clearly defined goals, set up GA for your website and define your key performance indicators.
Let’s take an online mobile phone cover store as an example. The business goal for this store is to increase revenue by increasing online orders and sales. The KPI set to track this goal would then be the number of phone covers sold.
This stage, however, does not simply end with defining website goals and KPIs. It also includes understanding your visitors. We have already discussed the various tools that can be used to gather visitor behavior data. Once data is collected, log in observations and start planning your campaign from there. Better data means higher sales.
Once the business goals are defined, KPIs set, and website data and visitor behavior data analyzed, it is time to prepare a backlog.
Backlog: “ an accumulation of tasks unperformed or materials not processed. ”
Your backlog should be an exhaustive list of all the elements on the website that you decide to test based on the data you analyzed. With a data-backed backlog ready, the next step is formulating a hypothesis for each backlog item. With the data gathered in this stage and its analysis, you will now have enough context of what happens on your website and why. Formulate a hypothesis based on them.
For example, after analyzing the data gathered using quantitative and qualitative research tools in the 1st stage, you come to the conclusion that not having multiple payment options led to maximum prospect customers dropping off on the checkout page. So you hypothesize that “adding multiple payment options will help reduce drop off on the checkout page.”
In short, by the end of this stage, you will know the whats and whys of your website.
Stage 2: Prioritize
The next stage involves prioritizing your test opportunities. Prioritizing helps you scientifically sort multiple hypotheses. By now, you should be fully equipped with website data, visitor data and be clear on your goals. With the backlog, you prepared in the first stage and the hypothesis ready for each candidate, you are halfway there on your optimization roadmap. Now comes the main task of this stage: prioritizing.
In stage 2, you should be fully equipped to identify problem areas of your website and leaks in your funnel. But not every action area has equal business potential. So it becomes imperative to weigh out your backlog candidates before picking the ones you want to test. There are a few things to be kept in mind while prioritizing items for your test campaign like the potential for improvement, page value and cost, the importance of the page from a business perspective, traffic on the page, and so on.
But how can you ensure that no subjectivity finds its way in your prioritization framework? Can you be 100% objective at all times? As humans, we give loads of importance to gut feelings, personal opinions, ideas, and values because these are the things that help us in our everyday lives. But, CRO is not everyday life. It is a scientific process that needs you to be objective and make sound data-backed decisions and choices. The best way to weed out these subjectivities is by adopting a prioritization framework.
There are many prioritization frameworks that even experts employ to make sense of their huge backlogs. On this pillar page, you will learn about the most popular frameworks that experience optimizers use – the CIE prioritization framework, the PIE prioritization framework, and the LIFT Model.
1. CIE Prioritization Framework
In the CIE framework, there are three parameters on which you must rate your test on a scale of 1 to 5:
- Confidence: On a scale of 1 to 5 – 1 being the lowest and 5 being the highest – select how confident you are about achieving the expected improvement through the hypothesis.
- Importance: On a scale of 1 to 5 – 1 being the lowest, and 5 being the highest – select how crucial the test (for which the hypothesis is created) is.
- Ease: On a scale of 1 to 5 – 1 being the most difficult, and 5 being the easiest – select the complexity of the test. Rate how difficult it will be to implement the changes identified for the test.
Before you rate your hypotheses, consider these 3 things:
A. How confident are you of achieving the uplift?
Prototyping the user persona, you are targeting can help you determine the potential of a hypothesis. With a sound understanding of your audience, you can make an educated assumption on whether the hypothesis will address the users’ apprehensions and doubts and nudge them to convert or not.
B. How valuable is the traffic you are running this test for?
Your website may be attracting visitors in large numbers, but not all visitors become buyers. Not all convert. For example, a hypothesis built around the checkout page holds a higher importance than the one built around the product features page. This is because visitors on the checkout page are way deep in your conversion funnel and have a higher chance to convert rather than visitors on your product features page.
C. How easy is it to implement this test?
Next comes determining the ease of implementing your test. Try to answer some questions: Would it need a lot of strategizing on your part to implement the hypothesis? What is the effort needed in designing and developing the solution proposed by the hypothesis? Can the changes suggested in the hypothesis be implemented using just the Visual Editor, or does it warrant adding custom code? It is only after you have answered all these and other such questions should you rate your backlog candidate on the easing parameter.
2. PIE Prioritization Framework
The PIE framework was developed to answer the question, “Where should I test first?”. The whole aim of the prioritization stage in your A/B testing journey is to find the answer to this very question. The PIE framework talks about 3 criteria that you should consider while choosing what to test when: potential, importance, and ease.
Potential means a page’s ability to improve. The planning stage should equip you with all the data you need to determine this.
Importance refers to a page’s value: how much traffic comes to the page. If you have identified a problem page, but there is no traffic on that page, then that page is of less importance when compared to other pages with higher traffic.
The third and final criteria is ease. Ease defines how difficult it is to run a test on a particular page or element. One way to determine ease of testing a page is using tools like landing page analyzer to determine the current state of your landing pages, estimate the number and scale of change it would require, and prioritize which ones to do or whether to do it at all. This is important from the perspective of resources. Many businesses drop the idea of undertaking and A/B testing campaign because of the lack of resources. These resources are of 2 kinds:
A. Human resource
Even though businesses have been using CRO and A/B testing for many years, it is only recently that the two concepts gained a front stage. Because of this, a large segment of the market does not have a dedicated optimization team, and when they do, it is usually limited to a handful of people. This is where a planned optimization calendar comes in handy. With a properly planned and prioritized backlog, a small CRO team can focus its limited resources on high stake items.
B. Tools:
As popular as CRO and A/B testing are getting, so are hundreds of A/B testing tools – both low end and high. Without the perspective of an expert, if businesses were to pick one out of the lot, say the cheapest one, and start A/B testing every single item on the backlog, they will reach no statistically significant conclusion. There are 2 reasons for this: one, testing without prioritization is bound to fail and not reap any business profits. Two, not all tools are of the same quality.
Some tools may be costlier, but they are either integrated with good qualitative and quantitative research tools or are brilliant standalone tools making them more than capable of producing statistically significant results. While the other lot may be cheaper and lure businesses during capital crunch and with a huge backlog, these tools will only be an investment loss to them without any benefits. Prioritization will help you make sense of your backlog and dedicate whatever little resources you have to a profitable testing candidate.
Backlog candidates should be marked on how hard they are to test based on technical and economic ease. You can quantify each potential candidate as a business opportunity based on the above criteria and choose the highest scorer. For example, like an eCommerce business, you may want to test your homepage, product page, checkout page, and thank you (rating) page. Now according to the PIE framework, you line these up and mark them potential, importance and ease:
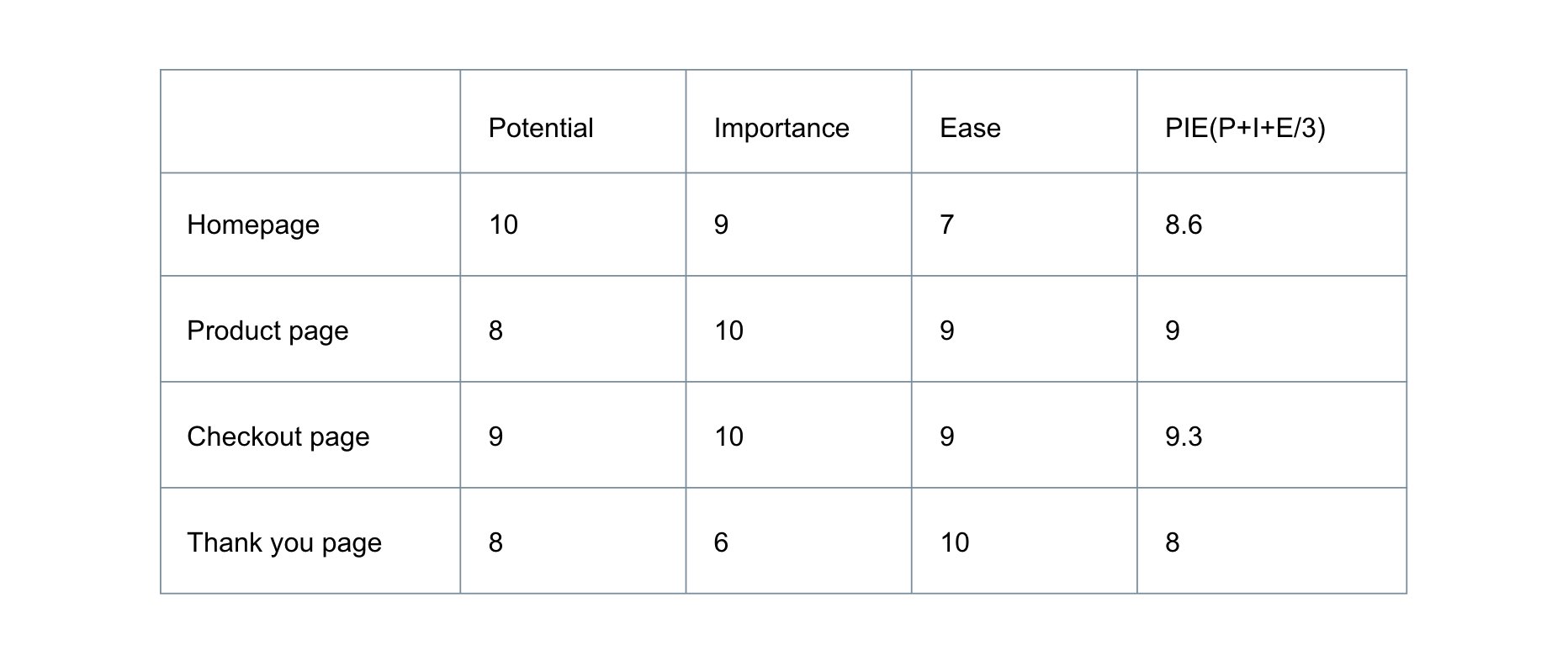
*marked out of a total of 10 points per criteria.
3. The LIFT Model
The LIFT Model is another popular conversion optimization framework that helps you analyze web and mobile experiences, and develop good A/B test hypotheses. It draws on the 6 conversion factors to evaluate experiences from the perspective of your page visitor: Value Proposition, Clarity, Relevance, Distraction, Urgency, and Anxiety.
With prioritization, you can have your A/B testing calendar ready for execution for at least 6 to 12 months. This will not only give you time, and a heads-up to prepare for the test but also plan around your resources.
Stage 3: A/B test
The third and most crucial stage is the testing stage. After the prioritization stage, you will have all the required data and a prioritized backlog. Once you have formulated hypotheses that align to your goal and prioritized them, create variations, and flag off the test. While your test is running, make sure it meets every requirement to produce statistically significant results before closure, like testing on accurate traffic, not testing too many elements together, testing for the correct amount of duration, and so on.
Stage 4: Repeat
This stage is all about learning from your past and current test and applying them in future tests. Once your test runs for the stipulated amount of time, stop the test and start analyzing the data thus gathered. The first thing you will realize is one of the many versions that were being tested had performed better than all others and won. It’s time for you and your team to now figure out why that happened. There can be 3 outcomes of your test:
- Your variation or one of your variations will have won with statistical significance.
- Your control was the better version and won over the variation/s.
- Your test failed and produced insignificant results. Determine the significance of your test results with the help of tools like the A/B test significance calculator.
In the first two scenarios, do not stop testing just because you have a winner. Make improvements to that version and keep testing. In the third scenario, recall all the steps and identify where you went wrong in the process and re-do the test after rectifying the mistake.
Here is a downloadable A/B testing calendar sample for your reference. To use this spreadsheet, click on the ‘File’ option in the main menu and then click on ‘Make a copy.’
File > Make a copy
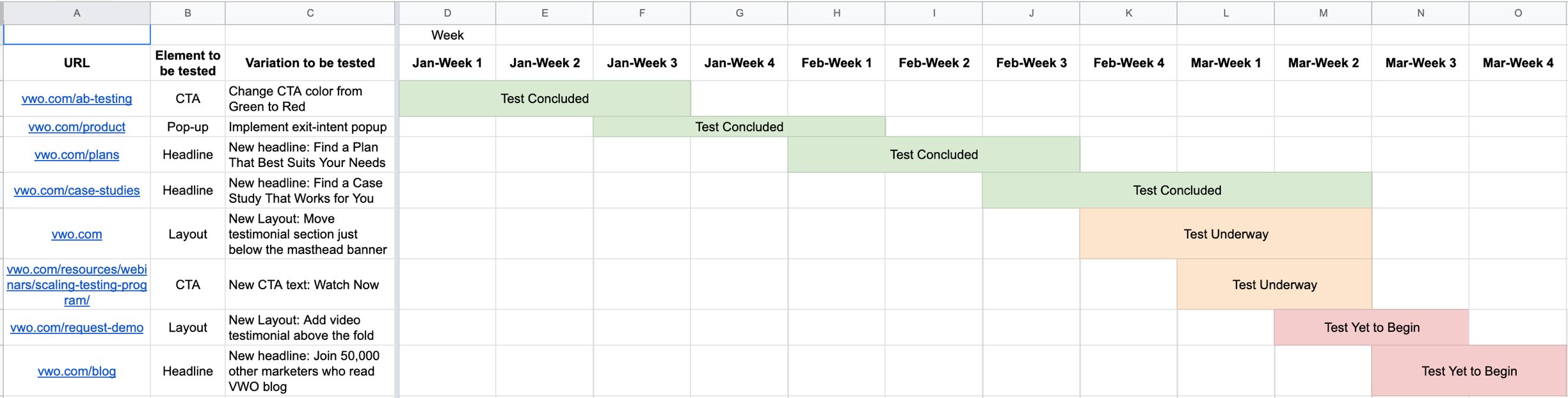
When scaling your A/B testing program, keep in mind the following points:
A. Revisiting previously concluded test:
With a prioritized calendar in place, your optimization team will have a clear vision of what they will test next and which test needs to be run when. Once you have tested each element or most elements in the backlog, revisit each successful as well as failed campaigns. Analyze the test results and determine whether there is enough data to justify running another version of the test. If there is, then run the test again – with necessary edits and modifications.
B. Increasing testing frequency:
While you should always be cautious of testing too many elements together, increasing your testing frequency is essential in scaling your testing program. Your optimization team will have to plan it in such a way that none of the tests affect others or your website’s performance. One way to do this is by running tests simultaneously on different web pages of your website or testing elements of the same web page at different time periods. This will not only increase your testing frequency but also, none of the tests will affect others. For instance, you can simultaneously test one element each of your homepage, checkout page, and sign-up page at one time and other elements (1 element at a time) of these pages after the current test concludes.
C. Spacing out your test:
This flows from the previous point. If you look at the calendar above, you will see that not more than two tests overlap each other at any given week. In a quest to increase your testing frequency, do not compromise with your website’s overall conversion rate. If you have two or more critical elements to be tested on the same web page, space the two out. As pointed earlier, testing too many elements of a web page together makes it difficult to pinpoint which element influenced the success or failure of the test most.
Let’s say, for example, you want to test one of your ad’s landing pages . You lock in on testing the CTA to increase sign-ups and banners to decrease the bounce rate and increase time spent. For the CTA, based on your data, you decide to change the copy. For the banner, you decide to test a video against a static image. You deploy both tests at the same time, and at the conclusion, both your goals were met. The problem here is that data showed that while sign-ups did increase from the new CTA, the video (apart from reducing the bounce rate and increasing average time spent on the page) too helped in this. Most of the people who watched the video also ended up signing up.
The problem now is that, because you did not space the two tests, it became impossible to tell which element contributed most to the sign-up increase. Had you timed the two tests better, much more significant insights could have been gathered?
D. Tracking multiple metrics:
You usually measure an A/B test’s performance based on a single conversion goal and put all your trust on that goal to help you find the winning variation. But sometimes, the winning variation affects other website goals as well. The example above is applicable here too. The video, in addition to reducing bounce rate and increasing time spent, also contributed to increased sign-ups. To scale your A/B testing program, track multiple metrics so that you can draw more benefits with less effort.
Having a thoroughly built calendar helps to streamline things to a great extent. VWO has an inbuilt calendar-making feature known as the Kanban board that helps track your tests’ progress at various stages.
What are the mistakes to avoid while A/B testing?
A/B testing is one of the most effective ways to move business metrics in a positive direction and increase the inward flow of revenue. However, as stated above, A/B testing demands planning, patience, and precision. Making silly mistakes can cost your business time and money, which you can’t afford. To help you avoid making blunders, here’s a list of some of the most common mistakes to remember when running an A/B test:
Mistake #1: Not planning your optimization Roadmap
A. Invalid hypothesis:
In A/B testing, a hypothesis is formulated before conducting a test. All the next steps depend on it: what should be changed, why should it be changed, what the expected outcome is, and so on. If you start with the wrong hypothesis, the probability of the test succeeding decreases.
B. Taking others’ word for it:
Sure, someone else changed their sign-up flow and saw a 30% uplift in conversions. But it is their test result, based on their traffic, their hypothesis, and their goals. Here’s why you should not implement someone else’s test results as is onto your website: no two websites are the same – what worked for them might not work for you. Their traffic will be different; their target audience might be different; their optimization method may have been different than yours, and so on.
Mistake #2: Testing too many elements together
Industry experts caution against running too many tests at the same time. Testing too many elements of a website together makes it difficult to pinpoint which element influenced the test’s success or failure the most. The more the elements tested, the more needs to be the traffic on that page to justify statistically significant testing. Thus, prioritization of tests is indispensable for successful A/B testing.
Mistake #3: Ignoring statistical significance
If gut feelings or personal opinions find a way into hypothesis formulation or while you are setting the A/B test goals, it is most likely to fail. Irrespective of everything, whether the test succeeds or fails, you must let it run through its entire course so that it reaches its statistical significance.
For a reason, that test results, no matter good or bad, will give you valuable insights and help you plan your upcoming test in a better manner.
You can get more information about the different types of errors while dealing with the maths of A/B testing.
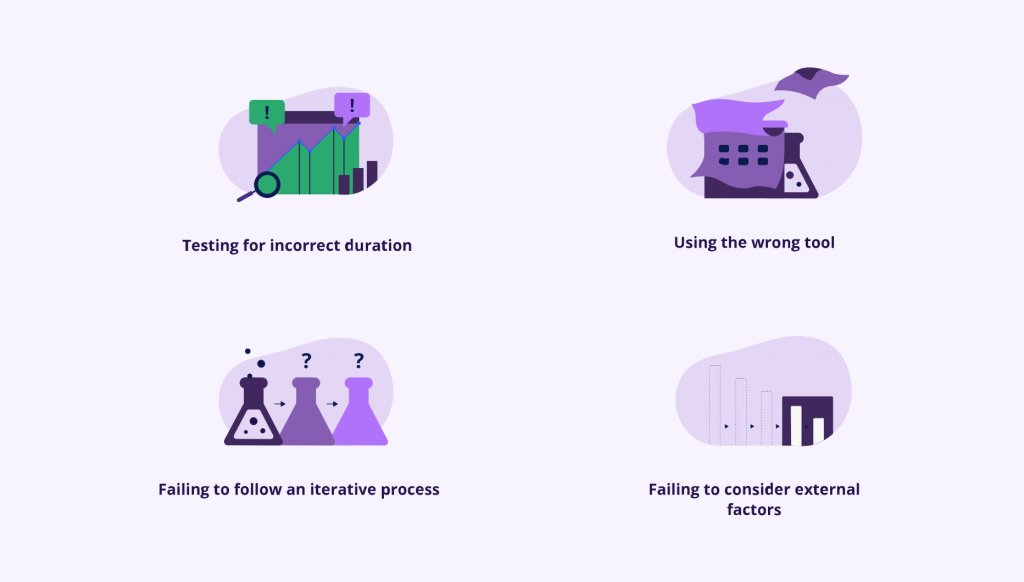
Mistake #4: Using unbalanced traffic
Businesses often end up testing unbalanced traffic. A/B testing should be done with the appropriate traffic to get significant results. Using lower or higher traffic than required for testing increases the chances of your campaign failing or generating inconclusive results.
Mistake #5: Testing for incorrect duration
Based on your traffic and goals, run A/B tests for a certain length of time to achieve statistical significance. Running a test for too long or too short a period can result in the test failing or producing insignificant results. Because one version of your website appears to be winning within the first few days of starting the test does not mean that you should call it off before time and declare a winner. Letting a campaign run for too long is also a common blunder that businesses commit. The duration for which you need to run your test depends on various factors like existing traffic, existing conversion rate, expected improvement, etc.
Learn how long you should run your test .
Mistake #6: Failing to follow an iterative process
A/B testing is an iterative process, with each test building upon the results of the previous tests. Businesses give up on A/B testing after their first test fails. But to improve the chances of your next test succeeding, you should draw insights from your last tests while planning and deploying your next test. This increases the probability of your test succeeding with statistically significant results.
Additionally, do not stop testing after a successful one. Test each element repetitively to produce the most optimized version of it even if they are a product of a successful campaign.
Mistake #7: Failing to consider external factors
Tests should be run in comparable periods to produce meaningful results. It is wrong to compare website traffic on the days when it gets the highest traffic to the days when it witnesses the lowest traffic because of external factors such as sales, holidays, and so on. Because the comparison here is not made between likes, the chances of reaching an insignificant conclusion increase. Use VWO’s A/B Test Significance Calculator to know if the results your test achieved were significant or not.
Mistake #8: Using the wrong tools
With A/B testing gaining popularity, multiple low-cost tools have also come up. Not all of these tools are equally good. Some tools drastically slow down your site, while others are not closely integrated with necessary qualitative tools ( heatmaps , session recordings, and so on), leading to data deterioration. A/B testing with such faulty tools can risk your test’s success from the start.
Mistake #9: Sticking to plain vanilla A/B testing method
While most experience optimizers recommend that you must start your experimentation journey by running small A/B tests on your website to get the hang of the entire process. But, in the long run, sticking to plain vanilla A/B testing methods won’t work wonders for your organization. For instance, if you are planning to revamp one of your website’s pages entirely, you ought to make use of split testing . Meanwhile, if you wish to test a series of permutations of CTA buttons, their color, the text, and the image of your page’s banner, you must use multivariate testing.
What are the challenges of A/B testing?
The ROI from A/B testing can be huge and positive. It helps you direct your marketing efforts to the most valuable elements by pinpointing exact problem areas. But every once in a while, as an experience optimizer, you may face some challenges when deciding to undertake A/B testing. The 6 primary challenges are as follows:
Challenge #1: Deciding what to test
You can’t just wake up one day and decide to test certain elements of your choice. A bitter reality that experience optimizers are now coming to realize is that not all small changes that are easy to implement are always the best when you consider your business goals and often fail to prove significant. The same goes for complex tests. This is where website data and visitor analysis data come into play. These data points help you overcome the challenge of ‘not knowing what to test’ out of your unending backlog by generally pointing to the elements which may have the most impact on your conversion rates or by directing you to pages with the highest traffic.
Challenge #2: Formulating hypotheses
In great resonance with the first challenge is the second challenge: formulating a hypothesis. This is where the importance of having scientific data at your disposal comes in handy. If you are testing without proper data, you might as well be gambling away your business. With the help of data gathered in the first step (i.e., research) of A/B testing, you need to discover where the problems lie with your site and come up with a hypothesis. This will not be possible unless you follow a well-structured and planned A/B testing program.
Challenge #3: Locking in on sample size
Not many experience optimizers are statisticians. We often make the mistake of calling conclusive results too quickly because we are more often than not after quick results. As experience optimizers, we need to learn about sample sizes, in particular, how large should our testing sample size be based on our web page’s traffic.
Challenge #4: Analyzing test results
With A/B testing, you will witness success and failure at each step. This challenge, however, is pertinent to both successful and failed tests:
1. Successful campaigns:
It’s great that you ran two tests, and both of them were successful in producing statistically significant results. What next? Yes, deploying the winner, but what after that? What experience optimizers often fail to do or find difficult is interpreting test results. Interpreting test results after they conclude is extremely important to understand why the test succeeded. A fundamental question to be asked is – why? Why did customers behave the way they did? Why did they react a certain way with one version and not with the other versions? What visitor insights did you gather, and how can you use them? Many experience optimizers often struggle or fail to answer these questions, which not only help you make sense of the current test but also provide inputs for future tests.
2. Failed campaigns:
Sometimes, experience optimizers don’t even look back at failed tests. They either have a hard time dealing with them, for example, while telling the team about the failed tests or have no clue what to do with them. No failed test is unsuccessful unless you fail to draw learnings from them. Failed campaigns should be treated like pillars that would ultimately lead you to success. The data gathered during the entire A/B testing process, even if in the end, the test failed, is like an unopened pandora box. It contains a plethora of valuable data and insights that can give you a head start for your next test.
Additionally, with the lack of proper knowledge on how to analyze the gathered data, the chances of data corruption increase manifold. For example: without having a process in place, there will be no end to scrolling through heatmaps data or sessions recording data. Meanwhile, if you are using different tools for these, then the chances of data leakage while attempting to integrate them also increase. You may also fail to draw any significant insights while wandering directionless through data and just drown under them.
Challenge #5: Maintaining a testing culture
One of the most crucial characteristics of optimization programs like CRO and A/B testing is that it is an iterative process. This is also one of the major obstacles that businesses and experience optimizers face. For your optimization efforts to be fruitful in the long run, they should form a cycle that roughly starts with research and ends in research.
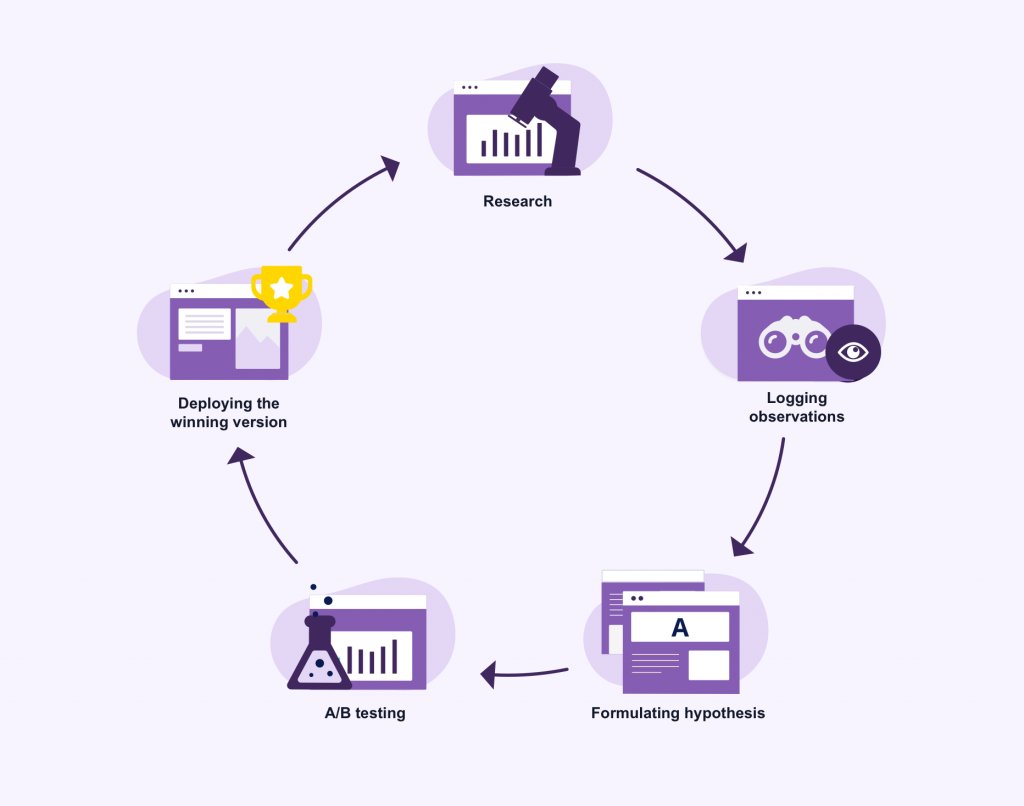
This challenge is not just a matter of putting in effort or about having the required knowledge. Sometimes due to resource crunch, businesses rarely or intermittently use A/B testing and fail to develop a proper testing culture.
Challenge #6: Changing experiment settings in the middle of an A/B test
When you launch an experiment, you must commit to it completely. Try and not to change your experiment settings, edit or omit your test goals, or play with the design of the control or the variation while the test is running. Moreso, do not try and change the traffic allocations to variations as well because doing so will not only alter the sampling size of your returning visitors but massively skew your test results as well.
So, given all these challenges, is A/B testing worth undertaking?
From all the evidence and data available on A/B testing, even after these challenges, A/B testing generates great ROI. From a marketing perspective, A/B testing takes the guesswork out of the optimization process. Strategic marketing decisions become data-driven, making it easier to craft an ideal marketing strategy for a website with well-defined ends. Without an A/B testing program, your marketing team will simply test elements at random or based on gut feelings and preferences. Such data-less testing is bound to fail.
If you start strong with a good website and visitor data analysis, the first three challenges can easily be solved. With the extensive website and visitor data at your disposal, you can prioritize your backlog, and you won’t even have to decide on what to test. The data will do all the talking. With such quality data coupled with your business expertise, formulating a working hypothesis becomes just a matter of going through the available data and deciding what changes will be best for your end goal. To overcome the third challenge, you can calculate the apt sample size for your testing campaign with the help of many tools available today.
The last two challenges are related to how you approach A/B testing. If you treat A/B testing like an iterative process, half of the fourth challenge may not even be on your plate. And the other half can be solved by hiring experts in the field or by getting trained on how to analyze research data and results correctly. The right approach to tackle the last challenge is to channel your resources on the most business-critical elements and plan your testing program in a way that, with the limited resource, you can build a testing culture.
A/B testing and SEO
As far as implications of SEO on A/B testing are concerned, Google has cleared the air on their blog post titled “Website Testing And Google Search . The important bits from that post are summarized below:
No cloaking
Cloaking – showing one set of content to humans, and a different set to Googlebot – is against our Webmaster Guidelines, whether you’re running a test or not. Make sure that you’re not deciding whether to serve the test or which content variant to serve, based on user-agent. An example of this would always be serving the original content when you see the user-agent “Googlebot.” Remember that infringing our Guidelines can get your site demoted or even removed from Google search results – probably not the desired outcome of your test.
Only use 302 redirects
If you’re running an A/B test that redirects users from the original URL to a variation URL, use a 302 (temporary) redirect, not a 301 (permanent) redirect. This tells the search engines that this redirect is temporary – it will only be in place as long as you’re running the experiment – and that they should keep the original URL in their index rather than replacing it with the target of the redirect (the test page). JavaScript-based redirects also got a green light from Google.
Run experiments for the appropriate duration
The amount of time required for a reliable test will vary depending on factors like your conversion rates, and how much traffic your website gets. A good testing tool should tell you when you’ve gathered enough data to be able to draw reliable conclusions. Once you have concluded the test, you should update your site with the desired variation(s) and remove all elements of the test as soon as possible, such as alternate URLs or testing scripts and markup.
Use rel=”canonical” links
Google suggests using rel=“canonical” link attribute on all alternate URLs for you to be able to highlight that the original URL is actually the preferred one . This suggestion stems from the fact that rel=“canonical” more closely matches your intent in this situation when compared to other methods like no index meta tag. For instance, if you are testing variations of your product page, you don’t want search engines not to index your product page. You just want them to understand that all the test URLs are close duplicates or variations on the original URL and should be grouped together, with the original URL as the hero. Sometimes, in these instances, using no index rather than rel=“canonical” in such a situation can sometimes have unexpected bad effects.
A/B testing examples
A/b testing in media & publishing industry.
Some goals of a media and publishing business may be to increase readership and audience , to increase subscriptions, to increase time spent on their website by visitors, or to boost video views and other content pieces with social sharing and so on. You may try testing variations of email sign-up modals, recommended content, social sharing buttons, highlighting subscription offers, and other promotional options.
Any of us who is a Netflix user can vouch for their streaming experience. But not everyone knows how they manage to make it so good. Here’s how – Netflix follows a structured and rigorous A/B testing program to deliver what other businesses struggle to deliver even today despite many efforts – a great user experience. Every change that Netflix makes to its website goes through an intense A/B testing process before getting deployed. One example to show how they do it is the use of personalization.
Netflix uses personalization extensively for its homepage. Based on each user’s profile, Netflix personalizes the homepage to provide the best user experience to each user . They decide how many rows go on the homepage and which shows/movies go into the rows based on the users streaming history and preferences.
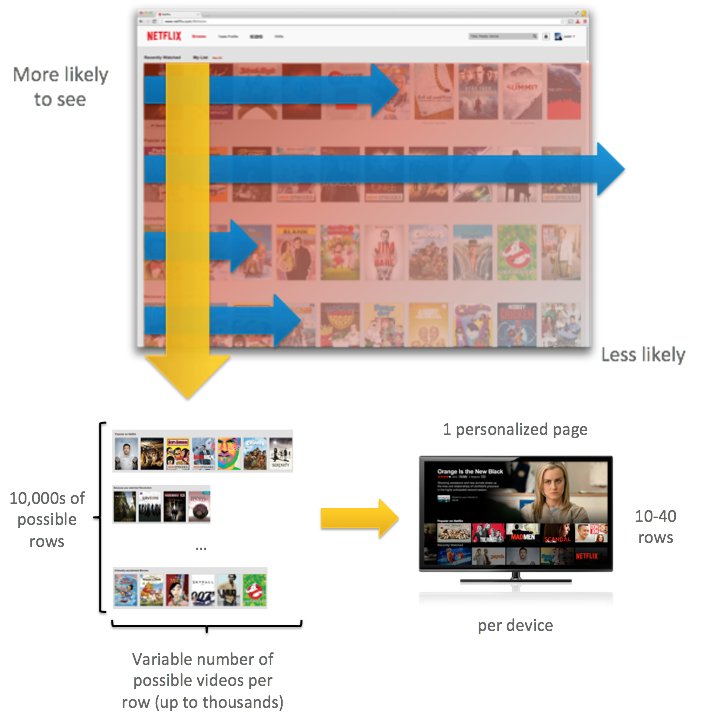
They follow the same exercise with media title pages as well. Within these pages, Netflix personalizes what titles are we most likely to watch, the thumbnails we see on them, what title text entices us to click, or if social proof helps make our decision easier, and so on. And this is just the tip of the iceberg.
A/B Testing in eCommerce Industry
Through A/B testing, online stores can increase the average order value, optimize their checkout funnel, reduce cart abandonment rate, and so on. You may try testing: the way shipping cost is displayed and where, if, and how the free shipping feature is highlighted, text and color tweaks on the payment page or checkout page, the visibility of reviews or ratings, etc.
In the eCommerce industry, Amazon is at the forefront in conversion optimization partly due to the scale they operate at and partly due to their immense dedication to providing the best customer experience. Amongst the many revolutionary practices they brought to the eCommerce industry, the most prolific one has been their ‘1-Click Ordering’. Introduced in the late 1990s after much testing and analysis, 1-Click Ordering lets users make purchases without having to use the shopping cart at all.
Once users enter their default billing card details and shipping address, all they need to do is click on the button and wait for the ordered products to get delivered. Users don’t have to enter their billing and shipping details again while placing any orders. With the 1-Click Ordering, it became impossible for users to ignore the ease of purchase and go to another store. This change had such a huge business impact that Amazon got it patented (now expired) in 1999. In fact, in 2000, even Apple bought a license for the same to be used in their online store.
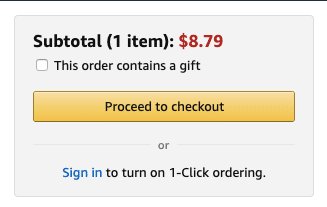
People working to optimize Amazon’s website do not have sudden ‘Eureka’ moments for every change they make. It is through continuous and structured A/B testing that Amazon is able to deliver the kind of user experience that it does. Every change on the website is first tested on their audience and then deployed. If you were to notice Amazon’s purchase funnel, you would realize that even though the funnel more or less replicates other websites’ purchase funnels, each and every element in it is fully optimized, and matches the audience’s expectations.
Every page, starting from the homepage to the payment page, only contains the essential details and leads to the exact next step required to push the users further into the conversion funnel. Additionally, using extensive user insights and website data, each step is simplified to their maximum possible potential to match their users’ expectations.
Take their omnipresent shopping cart, for example.
There is a small cart icon at the top right of Amazon’s homepage that stays visible no matter which page of the website you are on.
The icon is not just a shortcut to the cart or reminder for added products. In its current version, it offers 5 options:
- Continue shopping (if there are no products added to the cart)
- Learn about today’s deals (if there are no products added to the cart)
- Wish List (if there are no products added to the cart

- Proceed to checkout (when there are products in the cart)
- Sign in to turn on 1-Click Checkout (when there are products in the cart)
With one click on the tiny icon offering so many options, the user’s cognitive load is reduced, and they have a great user experience. As can be seen in the above screenshot, the same cart page also suggests similar products so that customers can navigate back into the website and continue shopping. All this is achieved with one weapon: A/B Testing.
A/B Testing in Travel Industry
Increase the number of successful bookings on your website or mobile app, your revenue from ancillary purchases, and much more through A/B testing. You may try testing your home page search modals, search results page, ancillary product presentation, your checkout progress bar, and so on.
In the travel industry, Booking.com easily surpasses all other eCommerce businesses when it comes to using A/B testing for their optimization needs. They test like it’s nobody’s business. From the day of its inception, Booking.com has treated A/B testing as the treadmill that introduces a flywheel effect for revenue. The scale at which Booking.com A/B tests is unmatched, especially when it comes to testing their copy. While you are reading this, there are nearly 1000 A/B tests running on Booking.com’s website .
Even though Booking.com has been A/B testing for more than a decade now, they still think there is more that they can do to improve user experience. And this is what makes Booking.com the ace in the game. Since the company started, Booking.com incorporated A/B testing into its everyday work process. They have increased their testing velocity to its current rate by eliminating HiPPOs and giving priority to data before anything else. And to increase the testing velocity, even more, all of Booking.com’s employees were allowed to run tests on ideas they thought could help grow the business.
This example will demonstrate the lengths to which Booking.com can go to optimize their users’ interaction with the website. Booking.com decided to broaden its reach in 2017 by offering rental properties for vacations alongside hotels. This led to Booking.com partnering with Outbrain, a native advertising platform, to help grow their global property owner registration.
Within the first few days of the launch, the team at Booking.com realized that even though a lot of property owners completed the first sign-up step, they got stuck in the next steps. At this time, pages built for the paid search of their native campaigns were used for the sign-up process.
Both the teams decided to work together and created three versions of landing page copy for Booking.com. Additional details like social proof, awards, and recognitions, user rewards, etc. were added to the variations.
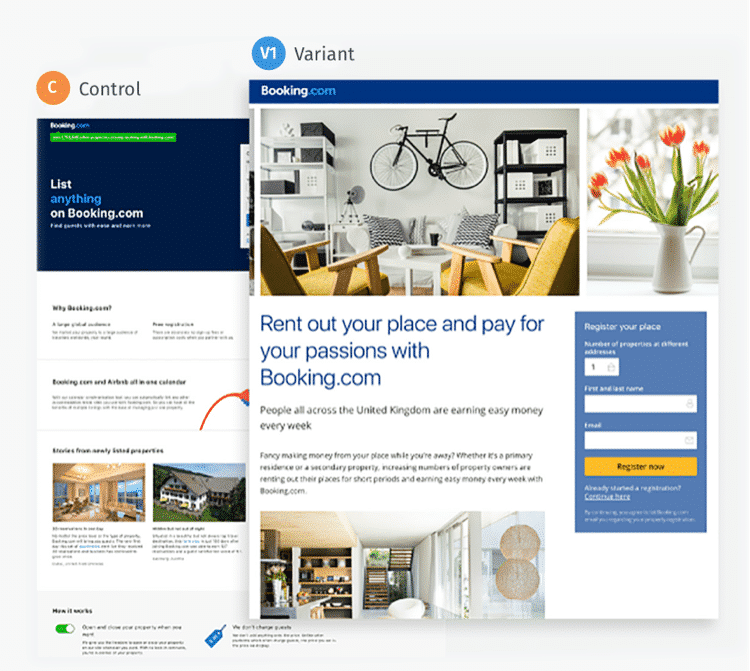
The test ran for two weeks and produced a 25% uplift in owner registration. The test results also showed a significant decrease in the cost of each registration.
A/B Testing in B2B/SaaS Industry
Generate high-quality leads for your sales team, increase the number of free trial requests, attract your target buyers, and perform other such actions by testing and polishing important elements of your demand generation engine. To get to these goals, marketing teams put up the most relevant content on their website, send out ads to prospect buyers, conduct webinars, put up special sales, and much more. But all their effort would go to waste if the landing page which clients are directed to is not fully optimized to give the best user experience.
The aim of SaaS A/B testing is to provide the best user experience and to improve conversions. You can try testing your lead form components, free trial sign-up flow, homepage messaging, CTA text, social proof on the home page, and so on.
POSist, a leading SaaS-based restaurant management platform with more than 5,000 customers at over 100 locations across six countries, wanted to increase their demo requests.
Their website homepage and Contact Us page are the most important pages in their funnel. The team at POSist wanted to reduce drop-off on these pages. To achieve this, the team created two variations of the homepage as well as two variations of the Contact Us page to be tested. Let’s take a look at the changes made to the homepage. This is what the control looked like:
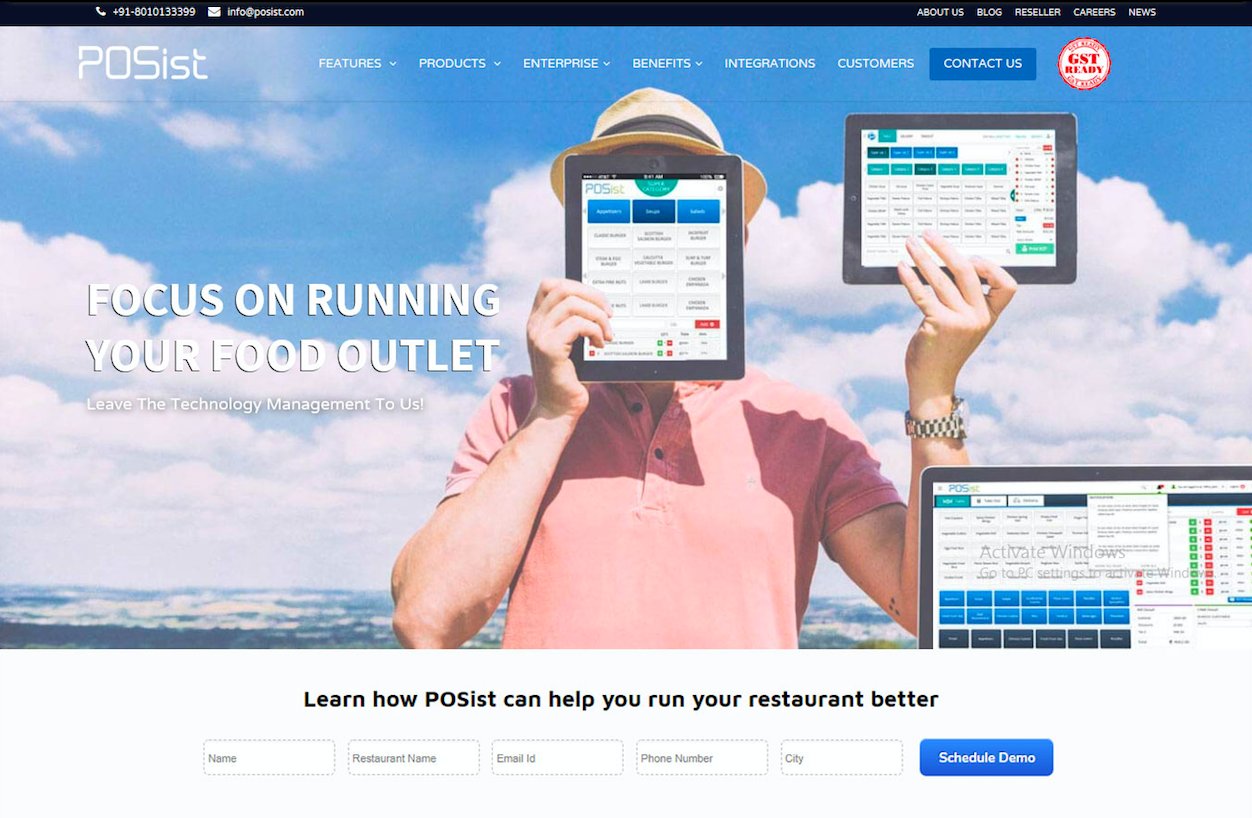
The team at POSist hypothesized that adding more relevant and conversion-focused content to the website will improve user experience, as well as generate higher conversions. So they created two variations to be tested against the control. This is what the variations looked like:
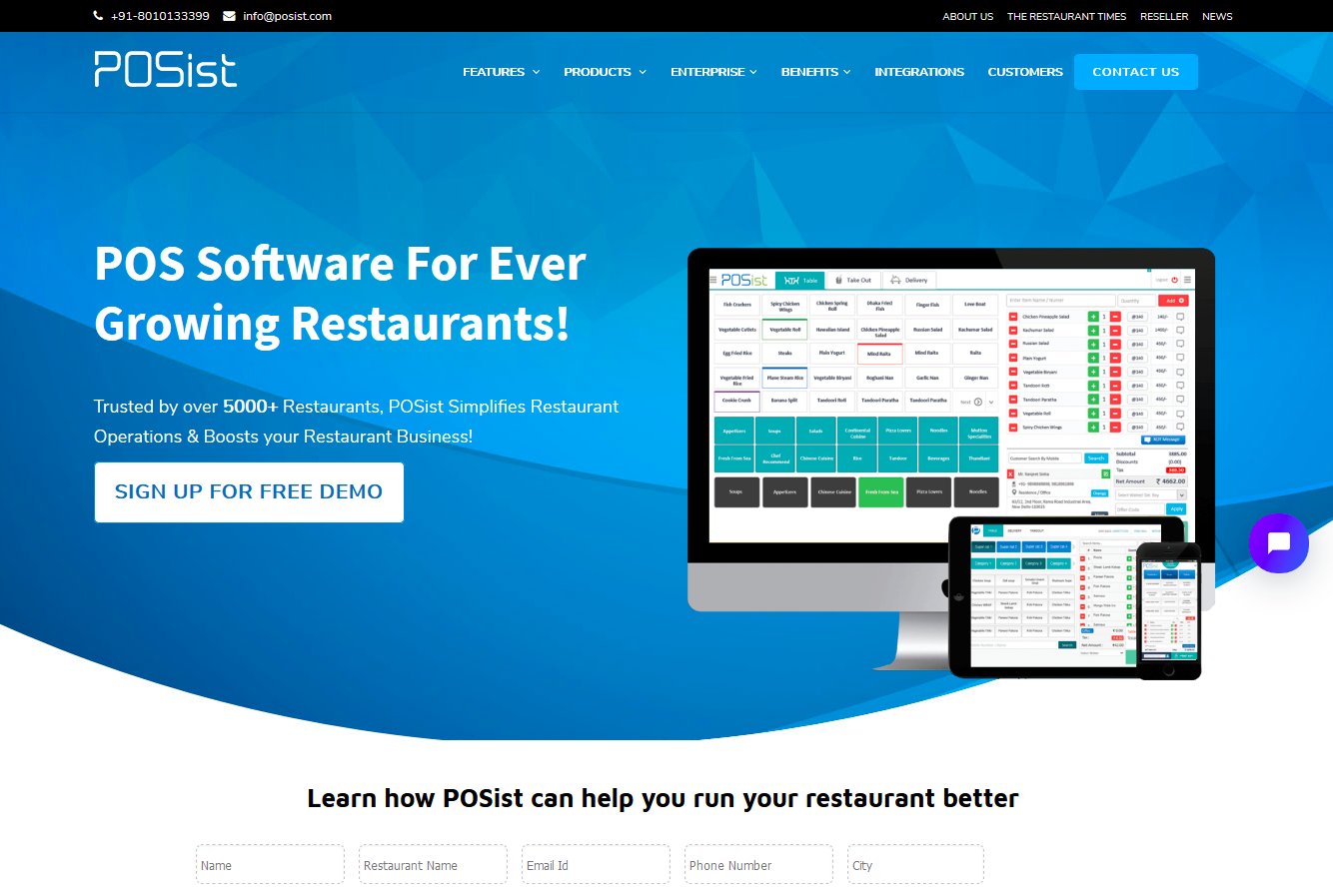
Control was first tested against Variation 1, and the winner was Variation 1. To further improve the page, variation one was then tested against variation two, and the winner was variation 2. The new variation increased page visits by about 5%.
After reading this comprehensive piece on A/B testing, you should now be fully equipped to plan your own optimization roadmap. Follow each step involved diligently and be wary of all major and minor mistakes that you can commit if you do not give data the importance it deserves. A/B testing is invaluable when it comes to improving your website’s conversion rates.
If done with complete dedication, and with the knowledge you now have, A/B testing can reduce a lot of risks involved when undertaking an optimization program. It will also help you significantly improve your website’s UX by eliminating all weak links and finding the most optimized version of your website.
If you found this guide useful, spread the word and help fellow experience optimizers A/B test without falling for the most common pitfalls. Happy testing!
Frequently asked questions on A/B testing
A/B testing is the process of comparing two variations of a page element, usually by testing users’ response to variant A vs. variant B and concluding which of the two variants is more effective.
In digital marketing, A/B testing is the process of showing two versions of the same web page to different segments of website visitors at the same time and then comparing which version improves website conversions.
There are various reasons why we do A/B testing. A few of them include solving visitor pain points, increasing website conversions or leads, and decreasing the bounce rate. Please read our guide to know the rest of the reasons.
In A/B testing, traffic is split amongst two or more completely different versions of a webpage. In multivariate testing, multiple combinations of a few key elements of a page are tested against each other to figure out which combination works best for the goal of the test.

Download this Guide
Deliver great experiences. grow faster, starting today..
Talk to a sales representative
Get in touch
Thank you for writing to us.
One of our representatives will get in touch with you shortly.
Signup for a full-featured trial
Free for 30 days. No credit card required
Set up your password to get started
Awesome! Your meeting is confirmed for at
Thank you, for sharing your details.
Hi 👋 Let's schedule your demo
To begin, tell us a bit about yourself
While we will deliver a demo that covers the entire VWO platform, please share a few details for us to personalize the demo for you.
Select the capabilities that you would like us to emphasise on during the demo., which of these sounds like you, please share the use cases, goals or needs that you are trying to solve., please share the url of your website..
We will come prepared with a demo environment for this specific website.
I can't wait to meet you on at
, thank you for sharing the details. Your dedicated VWO representative, will be in touch shortly to set up a time for this demo.
We're satisfied and glad we picked VWO. We're getting the ROI from our experiments. Christoffer Kjellberg CRO Manager
VWO has been so helpful in our optimization efforts. Testing opportunities are endless and it has allowed us to easily identify, set up, and run multiple tests at a time. Elizabeth Levitan Digital Optimization Specialist
As the project manager for our experimentation process, I love how the functionality of VWO allows us to get up and going quickly but also gives us the flexibility to be more complex with our testing. Tara Rowe Marketing Technology Manager
You don't need a website development background to make VWO work for you. The VWO support team is amazing Elizabeth Romanski Consumer Marketing & Analytics Manager
Trusted by thousands of leading brands
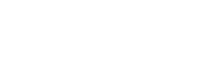
Chapter 1- AB testing book
Please ensure wole team has reasonably good understanding of these topics |
The article will provide a step-by-step walkthrough on all steps required for ab testing |
Before you start doing ab testing or run experiment, ascertain your hypothesis, a practical significance boundary, and a few metrics. Ensure these are disucssed and reviewed.Here are some tips for designing an A/B test: We should check the randomization of the sample that we will use for the control and treatment. We also should pay attention to how large the sample is to be used for running the experimentation. If we are concerned about detecting a small change or being more confident about the conclusion, we have to consider using more samples and a lower p-value threshold to get a more accurate result. However, If we are no longer care about small changes, we could reduce the sample to detect the practical significance. After you run experiment, plan how will you do following |
There are a few different ways to collect data for A/B testing. The most common way is to use a tool like Google Analytics or Optimizely. These tools allow you to track the number of visitors to your site, the pages they visit, and the actions they take. You can then use this data to compare the performance of different versions of your site or email. Another way to collect data for A/B testing is to use surveys or interviews. This can be a good way to get feedback on specific elements of your site or email, such as the design, the content, or the call to action. Once you have collected data, you need to analyze it to see which variant performed better. You can use a variety of statistical tests to do this. The most common test is the A/B test, which compares the conversion rates of two variants. Once you have identified the winning variant, you need to implement it on your live site or email. Then, you can continue to test to see if there are other ways to improve your results. Choose the right metrics to track. Not all metrics are created equal. When you're running an A/B test, you need to choose metrics that are directly related to your goal. For example, if you're trying to increase sales, you might track the number of purchases or the average order value. |
Make sure your test is statistically significant. This means that you have enough data to be confident that the results of your test are not due to chance. Here are some additional tips for running a successful A/B test: |
Here are some additional tips for interpreting A/B testing results correctly: |
In A/B testing, the statistical or practical significance threshold is the minimum amount of improvement that you need to see in your results before you can be confident that the change you made is actually making a difference. The statistical significance threshold is typically set at 0.05, which means that you need to be 95% confident that the change you made is not due to chance. The practical significance threshold is typically set at a higher level, such as 0.10 or 0.15, which means that you need to be more confident that the change you made is actually making a difference. The choice of statistical or practical significance threshold will depend on a number of factors, such as the cost of making the change, the potential impact of the change, and the level of risk you are willing to take. It is important to note that the statistical significance threshold is just a starting point. You may need to adjust the threshold based on other factors, such as the amount of data you have collected and the confidence level you are comfortable with. |
- Causal inference
- Offline experiments
- What to experiment
- Why experiment

Statistical Modelling for Data Science
A/b testing, a/b testing ¶.
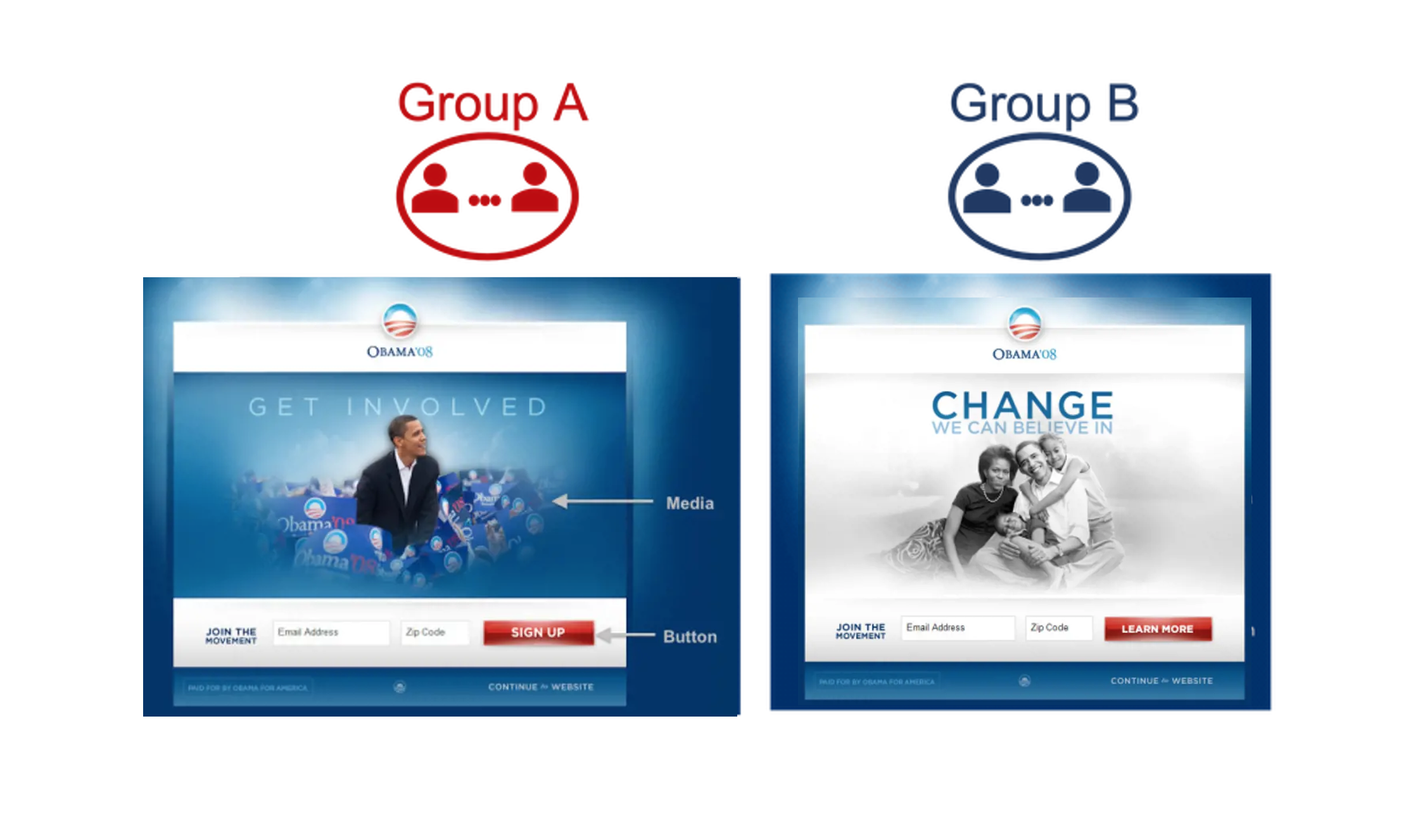
Photos/Images by Optimizely
This chapter provides an introduction to A/B testing and the early peeking problem. The goal is that you understand the main principles of A/B testing that will allow you to properly design, run, and analyze data from A/B tests. While you probably know the main concepts behind A/B testing, the usage of online platforms to collect, store and analyze data from A/B tests open new challenges. For example, people get tempted to peek at results before the A/B test ended and take actions usually result in false discoveries.
A/B Testing is a technique used to compare two variations of a product or service: control (A) and variation (B)
A/B testing became very popular in the context of updating and improving websites.
It’s main concepts are founded in the context of hypothesis testing and inference to compare population quantities from 2 distributions
for example: comparison of two population means or population proportions
Analysis Design ¶
As in any other statistical anlaysis, important steps of an A/B testing experiment include,
post the question(s) you want to answer using data
design the experiment to address your question(s)
identify appropriate methodologies to analyze the data
run the experiment to collect data
analyze the data according to the experimental design and make decisions
Case study ¶
Obama’s 60 million dollar experiment ¶.
In 2008, Obama’s campaign was looking to increase the total amount of donations to the campaign. Organizers run an experiment to compare visitors’ responses to different versions of the website.
A full description of this case study can be found here .
While there is not a unique way to design an A/B testing experiment, randomized controlled experiments are commonly used in practice. When participants are randomly assigned to the experimental group or the control group, you only expect to observe differences driven by the controlled effect (e.g., a new website) and not by other confounding factors.
Other decisions need to be made when designing an A/B testing experiment, including sample size, number of tests, methodology. Since the choices made would influence the results, companies usually run some calibration tests before running the actual experiment.
Question : Does visitors of the new website contribute with larger donations for the political campaign?
Design : Randomly allocate 1000 visitors to each website (control and new)
Method : Analyze the data sequentially in batches of 50 visitors per website. Use a classical \(t\) -test to run the analysis, compute \(p\) -values and confidence intervals
Decision : Stop the experiment if the \(p\) -value of a test drops below a significance level of \(0.05\)
So, what is new about A/B testing?
Early stopping ¶
New platforms have been developed to assist companies to analyze, report and visualize the results of their experiments in real time

Figure by D. Meisner in Towards Data Science
These platforms allow the users to continuously monitor the \(p\) -values and confidence intervals in order to re-adjust their experiment dynamically.
In classical hypothesis testing theory, the sample size must be fixed in advance when the experiment is designed!! Is it ok to peek at results before all the data are collected??
In general, there are large opportunity costs associated with longer experiments. Thus, users may prefer to adaptively determine the sample size of the experiments and make decisions before the planned experiment ends.
Early stopping refers to ending the experiment earlier than originally designed.
Can we stop or re-design the experiment earlier if we have supporting evidence to do so?? Let’s answer this question using data!
A/A Testing ¶
To examine the problem of early stopping, let’s simulate data for which \(H_0\) is true (i.e., there is no effect)
All users are allocated to the same version of a website.
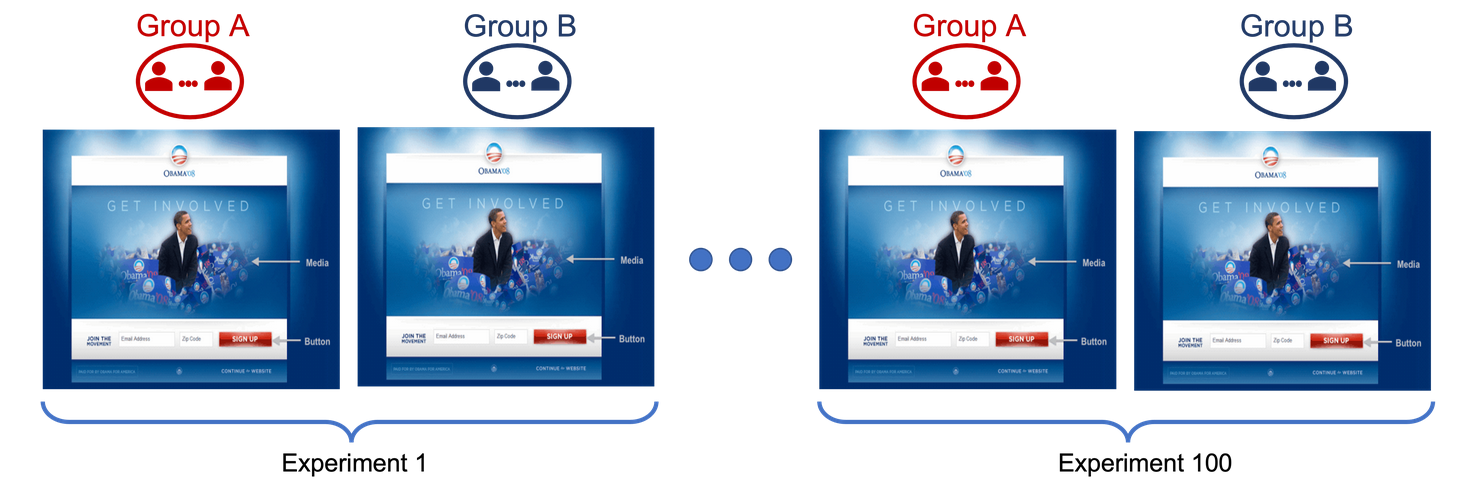
In such a scenario, data from both groups are generated from the same distribution. Thus, we know that rejecting \(H_0\) is a mistake!
Simulation Example
generate 1000 data points per group (control and variation) from the same distribution (total sample size of 2000)
sequentially analyze the data in batches of 50 observations per group using a two-sample \(t\) -tests
sequentially compute and monitor the (raw) \(p\) -values
reject the null hypothesis if a \(p\) -value drops below \(0.05\) and stop the experiment.
Simulating this kind of data and running the same analysis many times allows you examine the properties of the procedure used. For example, you can estimate the type I error rate by counting in how many experiments the \(p\) -value has dropped below the significance level and you erroneously reject the null hypothesis
Wrong Rejection
The data were generated from the same distribution (e.g., all users are allocated to the same version of a website). Thus, in principle, the \(p\) -value should not drop below 0.05. However, due to randomness, you may still find significant differences between the two groups. But how often does that occur??
Let’s start by simulating one such experiments and visualizing the results.

Interpretation ¶
After collecting data from 100 participants the \(p\) -value drops below \(0.05\) so the experiment is stopped. But we know that claiming a significance difference in this experiment is a mistake.
Changing the website is costly and may not really increase the size of the donations as expected
But, isn’t this mistake one potential result of the test?
In statistical hypothesis testing, rejecting \(H_0\) when it is true is known as the type I error . The probability of a type I error is equal to the significance level of the test.
In our example, the test was planned so that the probability to falsely rejecting \(H_0\) is 5%. So, why is this a problem??
Type I error rate inflation
The problem is that the probability of falsely rejecting \(H_0\) may be larger than expected!
To know if the probability of falsely rejecting \(H_0\) is larger than 5%, we need to run many of these experiments!!
The figure below shows the p-value trajectory of 100 experiments. We see that the p-values of more than 5% of the experiments are below the significance level.
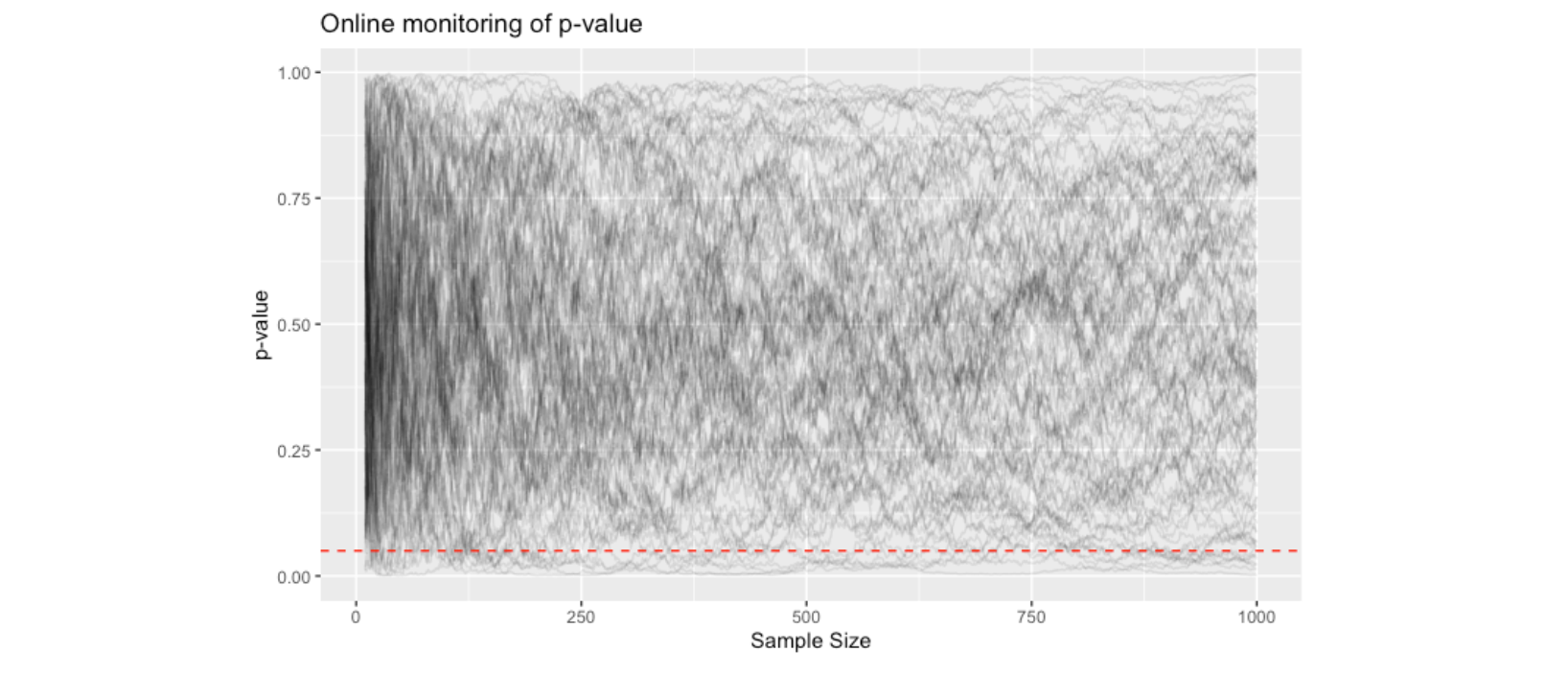
It can be proved, mathematically, that under the null hypothesis, the classical \(p\) -value will always cross \(\alpha\) if the experimenter waits long enough \(^{*}\) . This means that with increasing data, the probability of falsely rejecting a true \(H_0\) approaches to 1!
[*] David Siegmund. 1985. Sequential analysis: tests and confidence intervals. Springer.
Summary and key concepts learned ¶
A/B testing refers to an experiment, in which users are randomly assigned to one of two variations of a product or service: control (A) and variation (B) to see if variation B should be used for improvement.
The statistic used to test a hypothesis, the sample size calculation, the type I error rate specification and the desired power are all important and interconnected pieces of the experimental design!
In classical hypothesis testing theory, the sample size must be fixed in advance when the experiment is designed!!
Modern platforms allow the users to continuously monitor the p-values and confidence intervals of their tests as data are collected (peeking) in order to re-adjust their experiment dynamically.
Stopping an experiment and rejecting \(H_0\) as soon as the \(p\) -value is below the specified significance level can drastically inflate the type I error rate
Controlling the risk of wrongly rejecting the null hypothesis is not an easy task in A/B testing if peeking and early stops are allowed!
Statistical modelling for Data Science
Principled Peeking in A/B Testing
Click through the PLOS taxonomy to find articles in your field.
For more information about PLOS Subject Areas, click here .
Loading metrics
Open Access
Peer-reviewed
Research Article
Evaluating the perceived affective qualities of urban soundscapes through audiovisual experiments
Roles Conceptualization, Data curation, Formal analysis, Funding acquisition, Investigation, Methodology, Project administration, Validation, Visualization, Writing – original draft, Writing – review & editing
* E-mail: [email protected]
Affiliations Faculty of Visual Arts, Federal University of Goiás, Goiânia, Brazil, Chair of Acoustics and Haptics, Technische Universität Dresden, Dresden, Germany

Roles Conceptualization, Data curation, Formal analysis, Project administration, Supervision, Validation, Visualization, Writing – original draft, Writing – review & editing
Affiliations Acoustics Research Centre, University of Salford, Manchester, United Kingdom, Environmental Research & Innovation Centre, University of Salford, Manchester, United Kingdom
Roles Conceptualization, Resources, Supervision, Validation, Writing – review & editing
Affiliation Chair of Acoustics and Haptics, Technische Universität Dresden, Dresden, Germany
Roles Conceptualization, Resources, Supervision, Writing – review & editing
- Maria Luiza de Ulhôa Carvalho,
- Margret Sibylle Engel,
- Bruno M. Fazenda,
- William J. Davies
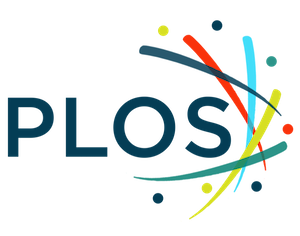
- Published: September 5, 2024
- https://doi.org/10.1371/journal.pone.0306261
- Reader Comments
The study of the perceived affective qualities (PAQs) in soundscape assessments have increased in recent years, with methods varying from in-situ to laboratory. Through technological advances, virtual reality (VR) has facilitated evaluations of multiple locations in the same experiment. In this paper, VR reproductions of different urban sites were presented in an online and laboratory environment testing three locations in Greater Manchester (‘Park’, ‘Plaza’, and pedestrian ‘Street’) in two population densities (empty and busy) using ISO/TS 12913–2 (2018) soundscape PAQs. The studied areas had audio and video recordings prepared for 360 video and binaural audio VR reproductions. The aims were to observe population density effects within locations (Wilcoxon test) and variations between locations (Mann-Whitney U test) within methods. Population density and comparisons among locations demonstrated a significant effect on most PAQs. Results also suggested that big cities can present homogenous sounds, composing a ‘blended’ urban soundscape, independently of functionality. These findings can support urban design in a low-cost approach, where urban planners can test different scenarios and interventions.
Citation: Carvalho MLdU, Engel MS, Fazenda BM, Davies WJ (2024) Evaluating the perceived affective qualities of urban soundscapes through audiovisual experiments. PLoS ONE 19(9): e0306261. https://doi.org/10.1371/journal.pone.0306261
Editor: Shazia Khalid, National University of Medical Sciences, PAKISTAN
Received: October 31, 2023; Accepted: June 13, 2024; Published: September 5, 2024
Copyright: © 2024 Carvalho et al. This is an open access article distributed under the terms of the Creative Commons Attribution License , which permits unrestricted use, distribution, and reproduction in any medium, provided the original author and source are credited.
Data Availability: All relevant data (scores for the perceived affective qualities - PAQs) are within the manuscript and its Supporting Information files.
Funding: The work was funded by the Coordenação de Aperfeiçoamento de Pessoal de Nível Superior (CAPES) - Finance Code 001 and the Universidade Federal de Goiás pole Goiânia, Brazil. The funders had no role in study design, data collection and analysis, decision to publish, or preparation of the manuscript.
Competing interests: The authors have declared that no competing interests exist.
1. Introduction
Since the publication of the ISO/TS 12913–2 [ 1 ], the characterisation of the affective attributes regarding the sonic environment has increased significantly over the years [ 2 – 7 ]. These affective attributes, or Perceived Affective Qualities (PAQs), originated from Axelsson et al. [ 8 ] research. They helped to detect the sound qualities of the investigated area, resulting in tools for urban sound management, effective urban planning, and noise control [ 9 ]. Studies point out that understanding emotional responses to soundscape supports design decisions [ 10 ], a better opportunity to achieve users’ satisfaction [ 11 ], and quality of life [ 12 ].
Regarding the emotional assessment of the acoustic environments, the work of Axelsson et al. [ 8 ] has been the reference for soundscape research. Their model was based on Russell’s circumplex affective model for environments [ 13 ]. Axelsson et al. [ 8 ] synthesised the semantic scales into a two-dimensional space constructed by pleasantness and eventfulness, which later was adopted as the PAQs in method A of the standard ISO/TS 12913–2 [ 1 ]. When rotating these two axes at 45 degrees, their diagonals result in additional dimensions, composed of the mixture related to the pleasant and eventful orthogonal axes. Thus, the standard ISO/TS 12913–2 introduces and describes the resulting eight attributes’ pairs: ‘eventful-uneventful’, ‘pleasant-annoying’, ‘vibrant-monotonous’, and ‘calm-chaotic’. However, this model is still under investigation and validation in other languages through the Soundscape Attributes Translation Project [ 14 ]. For instance, soundscape investigators lack consensus in identifying the origins and effects of emotional responses to sounds [ 4 , 15 , 16 ]. To assess these scales, researchers use self-reports, where people perceive these sounds through methods ranging from in-situ experiments to laboratory experiments, including virtual reality (VR).
The main methods for subjective data collection in soundscape studies have been soundwalks, interviews, listening tests, and focus groups [ 17 ]. The ISO/TS 12.913–2 suggests the first two methods [ 1 ]. However, the systematic review from Engel et al. [ 17 ] demonstrated that most recent studies use listening tests with the main topic of ‘soundscape quality’, using semantic differential tools to evaluate the stimuli of parks, squares, shopping areas, and traffic sounds, with students and academic staff as participants [ 17 ]. The controlled environment of the experiments happens in acoustically treated rooms with calibrated audio reproduction systems [ 18 ]. These studies allow the investigation of various aspects influencing auditory codification and perception [ 19 ], guaranteeing purity and control of factors [ 18 ], and enabling analyses of complex interactions or distinct effects [ 20 ]. In the laboratory, there are several listening experiment modalities, including with and without visual material [ 21 ], from simple (mono) [ 22 ] to complex audio reproduction (spatial audio) [ 23 ], multimodality (different sensorial stimuli), potentially implemented through Virtual Reality (VR) experiments.
Furthermore, VR technology can facilitate the evaluation of multiple locations in the same experiment under safe conditions [ 18 ] in a more engaging experiment [ 24 ], allowing observations of the effects on presence, realism, involvement, distraction level, and auditory aspect [ 25 ]. Participants are immersed in realist scenarios, giving them a ‘sense of presence’ [ 26 ], representing a similar experience of being in the real place. Audio, visual, tactile, and smells can enhance the multimodal experience. Regarding the virtual sonic environment, reproduction formats vary from mono to spatial audio [ 27 ]. Binaural audio played by headphones and ambisonics audio through loudspeakers are the main forms of audio reproduction in soundscape studies. In Sun et al. [ 28 , 29 ] study, when testing spatial audio through headphones and loudspeakers in a VR experiment, participants subjective responses demonstrated that the sense of immersion and realism were not affected by the type of audio reproduction.
Nevertheless, field and VR laboratory tests should sustain the experimental ‘ecological validity’. To guarantee this experimental condition, the laboratory reproduction of real-life audiovisual stimuli should create a similar sense of immersion and realism as in the original scenery [ 30 ]. If similarities are maintained between real and VR reproductions, laboratory experiments can support research with controlled factors. However, this may amplify results and biased conclusions, thus, outcomes should be interpreted cautiously [ 6 ]. So far, most studies have confirmed similar soundscape perceptions between in-situ and laboratory VR listening experiments [ 6 , 31 – 33 ], pointing out VR methods as a good strategy for soundscape research.
Another self-report data collection method is online experiments, which increased significantly during COVID-19. For example, the Lucid platform for online data collection in research tripled in purchases from 2019 to 2020 [ 34 ]. The drawbacks of online experiments are reduced attentiveness [ 34 ], the lack of controlled audio reproductions and system calibration used by the participants [ 32 ], the absence of assistants during the experiment, and unreliable responses given by different participants due to their context, among others [ 35 ]. The advantages of using a web-based approach in soundscape studies include a higher number of participants, ease of sharing, and engagement of citizens in sound design and urban planning. Regarding the urban sound design, ‘local experts’, people who live and use the studied location [ 36 ], local authorities, planners, designers and whoever is related to the site, should discuss their interests to indicate activities to the urban place [ 37 ]. Diversity in activities tends to create a more dynamic atmosphere in urban places. In these circumstances, acoustic zoning consists in giving the distance in space, time, or both [ 37 ]. Bento Coelho describes in his soundscape design process that a sound catalogue or sound identity map should be developed, where sounds are correlated to functions, activities, other senses, and preferred sounds of the place [ 38 ]. Additionally, appropriateness [ 7 ], and the expectations [ 39 ] of the sonic environment should reach towards a coherent soundscape. The guidelines mentioned above can delimit the acoustic zones based on sound sources, avoiding ‘lo-fi’ soundscapes. The latter represents sounds that are not easily located in an obscure population of sounds [ 40 ]—which may represent a ‘blended’ sonic environment. Its opposite is the ‘hi-fi’ soundscape with a clear distinction between foreground and background sounds [ 40 ], making it simple to identify the predominant sound source in the sonic environment.
The acoustically delimitated zones can correlate to the characteristics and functions of the locations. Urban soundscape studies have sites varying among natural places, public areas, squares, pedestrian streets, and shopping areas [ 17 ]. However, vibrant places are less studied. These are related to pleasant and eventful attributes linked to busy contexts in specific human activities [ 41 ]. Previous works confirm that the ‘presence of people’ in places leads to the ‘eventful’ dimension and may define a vibrant experience [ 3 , 29 ]. Most soundscape studies investigate parks, where natural sounds indicate psychological restoration [ 42 ], places for human de-stress [ 5 , 42 ], and improvement in the sonic environment evaluation [ 43 ]. These locations may represent pleasant places that can flourish feelings of joy and facilitate the public into fulfilling self-selected activities.
Based on the presented factors, this work adopts VR experiments through an online VR experiment, The Manchester Soundscape Experiment Online (MCR online), carried out in 2020, and a laboratory VR experiment, The Laboratory VR Soundscape Experiment (LAB VR), carried out in 2022, using spatial audio and 360° video recordings. Participants will be exposed to three urban sites (Peel Park—an urban park; Market Street—a pedestrian street; and Piccadilly Gardens—a plaza) in two population densities (empty and busy), followed by a self-report of the soundscape PAQs. The investigated hypotheses are four statements stated below. The Wilcoxon signed-rank test will be applied for comparisons within the two experiments, empty and busy conditions for the same location. In this case, the null and alternative hypotheses are:
- H 01 = The perceptual response (PAQs) will change when in different population densities in the same location and experiment; and
- H a1 = The perceptual response (PAQs) will not change when in different population densities in the same location and experiment.
The Mann–Whitney U test will be applied to compare the different soundscape locations for each data collection method, being their hypotheses as follows:
- H 02 = The perceptual response (PAQs) will change according to the different urban locations for each data collection method; and
- H a2 = The perceptual response (PAQs) will not change according to the different urban locations for each data collection method.
The PAQs of the ISO/TS 12913–2 [ 1 ] were selected as subjective responses given its international standardization. The aim is to observe the PAQ results from the previous two perspectives. The first view concerns an evaluation within each experiment where differences between the two population densities are analysed. Second, the variation between locations for each experimental method is investigated. Findings are considered to enhance comprehension of how people perceive the studied urban soundscape conditions through different VR methods, supporting urban sound design and future urban development appraisal [ 44 ].
2. Materials and methods
Fig 1 illustrates the investigated areas defined according to a previous study by Carvalho et al. [ 45 ]. They were derived from a structured interview to identify locations within the four quadrants of the ISO/TS 12913–2 [ 1 ] PAQs quadrants (‘vibrant’, ‘calm’, ‘monotonous’, and ‘chaotic’ attributes).
- PPT PowerPoint slide
- PNG larger image
- TIFF original image
The top illustrates all locations on the Manchester map. The middle row shows the ‘Street’ map, pictures of empty and busy conditions, the ‘Plaza’ map, and pictures of empty and busy conditions. The bottom row illustrates the ‘Park’ map, pictures of empty and busy conditions, north, and the UK map with Manchester’s position. The yellow dots are the evaluated sites. The areas shaded in blue are the areas studied. Pictures of Carvalho taken between 2019 to 2020.
https://doi.org/10.1371/journal.pone.0306261.g001
2.1 Study areas
Piccadilly Gardens (a popular plaza in the city centre) represented the ‘vibrant’ attribute called ‘Plaza’ from now on in the paper. Peel Park (a park at the University of Salford) exemplified the ‘calm’ attribute referred to as ‘Park’ hereafter. A bus stop (common bus stop in front of the University of Salford) corresponded to the ‘monotonous’ attribute, and Market Street (pedestrian commercial street) was selected for the ‘chaotic’ attribute, hereinafter, referred to as ‘Street’. The bus stop was excluded because the LAB VR experiment did not use this condition.
Piccadilly Gardens is the largest public space in central Manchester, with 1.49 Ha and various functions such as crossing, eating places, children’s play, and places for small and large events [ 46 ]. A contemporary design changed the garden into a Plaza in 2002 [ 46 ] that included a water fountain, playground, café store, a barrier by Japanese architect Tadao Ando that also served as protection of the central plaza, grass areas, and trees where people sit on sunny days. The location is surrounded by Piccadilly Street at the north, Mosley Street at the west, Parker Street at the south, and One Piccadilly Gardens building at the east side. The constant sound source in both population densities was sounds originating from the water fountain. In the empty condition, the fountain sound was predominant, but mechanical sounds were also present in the background. In the busy condition, the predominant sound was a rich presence of human sounds, such as chat and kids shouting, while traffic sounds from nearby trams and their breaks were audible in the background.
Peel Park has 9.40 Ha and is one of the oldest public parks in the world, dating from 1846 [ 47 ]. Today, it integrates with the Peel Park Campus of the University of Salford, including walking paths, tall and scattered trees, a playground structure, sculptures, a garden with flowerbeds, lots of green area, and benches to sit. The park is surrounded by the Student Accommodation and access to the David Lewis Sports Ground at the north; the River Irwell with a bridge to The Meadow, a public green space, and a housing area at the east; the Maxwell Building, and the Salford Museum and Art Gallery on the south; and the University House, the Clifford Library, and the Cockcroft Building at the westside. The local population uses the location for ‘passive’ recreation, exercise, and crossing paths to other sites. The constant sound source in both population densities was sounds of nature, specifically from the calls of birds. In the empty condition, four different bird calls were predominant and identified, them being ‘Pica Pica’, ‘Eurasian Wren’, ‘Redwing’, and the ‘Eurasian Tree Cree’. In the busy conditions, the bird call was not recognized, given the masking effects of human sounds, placing the nature sounds in the background, while the predominant foreground sounds were children talking, shouting, and playing football.
Market Street is approximately 370 meters long, with a 280-meter pedestrian zone occupying around 0.91 Ha. Exchange Street delimits it on the west until High Street on the east. The pedestrian zone is between High Street and Corporation Street, with primarily commercial activities such as clothes and shoe stores, banks, grocery stores, street food huts, gyms, bookstores, mobile stores, pharmacies, coffee stores, and three accesses to the Manchester Arndale Shopping. When the street gains traffic, commercial activities are more related to beauty products, confectionery, stationary, clothing and footwear, coffee shops, and access to the Royal Exchange Building. The constant sound source in both population densities was the ‘hoot’ from the nearby tram. In the empty condition, the predominant sounds were mechanical sounds, such as snaps of machinery in different rhythms and frequency intervals. Traffic and chats were also present in this condition. In the busy condition, snaps were still present, but predominance was related to human-made sounds, such as babble and footsteps.
2.2 Audiovisual preparation
Two different footages of the same studied areas were tested with two methods: an online VR questionnaire (MCR online) and a laboratory VR experiment (LAB VR). Audiovisual stimuli were different recordings in each experiment because participants of the MCR online complained about the video resolution. Thus, new recordings with a higher resolution camera occurred for the LAB VR. Nevertheless, all recordings were done in the same position. The study was conducted and approved by the Research, Innovation and Academic Engagement Ethical Approval Panel of the University of Salford (protocol code STR1819-31). Fig 2 illustrates the workflow for constructing the VR environments for the experiments.
Each column represents a stage.
https://doi.org/10.1371/journal.pone.0306261.g002
The Sound field microphone ST250 and the sound pressure level meter, type BSWA 308, were used in recordings with a sampling rate of 44.1 kHz. For the MCR online, the microphone was plugged into a ZOOM H6 Handy Recorder for the audios, and the Ricoh Theta S camera was used for the 360° videos. In the LAB VR, the microphone was plugged into an Edirol R44 Recorder, and an Insta 360 Pro2 360° video camera was used for video recording.
Given ethical approval restrictions, a sign warning ‘Filming in progress’ was displayed with the equipment for public awareness before recordings. With a previously calibrated sound pressure level meter, a one-minute sample of A-weighted equivalent continuous sound pressure (L Aeq,60 ) registered sound levels to adjust the field levels to laboratory reproductions. After initiating the microphone and camera, the researcher clapped in front of the equipment for future audiovisual alignment.
Recordings were done in the early hours (4 to 6 am) of a weekday for empty, and the afternoon (2 to 4 pm) at the weekend for busy conditions. On arrival, the locations were established so, as to not interrupt circulation. The experimenter merged into the scenery, and the recordings lasted 10 to 12 minutes [ 29 ]. These procedures resembled those done by the ‘Urban Soundscapes of the World’ project group [ 28 , 29 , 48 ].
Video files were transformed into equirectangular format (MCR online) or edited together (VR LAB). Audio and video stimuli were synchronised in time with the initial clap, verified and corrected when necessary. On the MCR online, the selected audiovisual stimuli had a 30-second duration following a previous study [ 49 ]. The stimuli duration changed to 8 seconds in the LAB VR, using as reference a fMRI soundscape experiment [ 50 ], because of a physiological test in another stage of the experiment.
A population density calculation occurred using the footage to select the audiovisual stimuli. The people-counting criteria followed a previous study that measured the number of individuals from a selected frame [ 51 ]. Surveys with ten participants were used to certify selected footage for empty and busy conditions. When the criteria failed, new stimuli selection took place. A descriptive analysis of the sound events, foreground and background sounds, was done of the footage with empty and busy conditions to select fragments rich in soundscape diversity [ 52 ], identity [ 53 ], character [ 54 ], and sound signal [ 40 ]. The LAB VR also had controlled sound signals, such as the water fountain at the ‘Plaza’, the tram hoot at the ‘Street’, and the bird calls at the ‘Park’ in empty and busy conditions.
Audio files were calibrated to the field sound levels using a pre-calibrated High-frequency Head and Torso Simulator (HATS) connected to a PULSE software of Brüel & Kjær [ 6 ]. Audiovisual stimuli were aligned through audio rotation using the azimuth angle θ from the first-order ambisonics equations, that is, audio X from front-back positions of B-format audio recordings—WXYZ) [ 22 ]. The audio and video files were rendered into 3D head-tracked stimuli for VR reproduction. Stimuli reproductions were tested through the final experimental VR and headphone setup, recorded for calibration, verified in each step, and corrected when necessary.
2.3 Participants and experimental procedures
Participants were recruited by the Acoustics Research Centre of Salford mailing list representing people with connections to the University of Salford, and above 18 years old in both experiments. The MCR online also had respondents recruited by convenience sampling over the internet on social networks, such as Facebook, Instagram, Twitter, and LinkedIn, and participated voluntarily from August 26 to November 30, 2020. The LAB VR received a compensation of £25 in Amazon voucher. These subjects were recruited from June 27 to August 5, 2022.
Conditions were three locations (‘Park’, ‘Plaza’, and ‘Street’) in two population densities (empty and busy) responding to the eight PAQs questions. MCR online had 80 individuals rating the ‘Plaza’ and ‘Street’ (80 x 2-sites x 2-densities x 8-PAQs = 2560 results), and 75 assessing the ‘Park’ (75 x 2-densities x 8-PAQs = 1200 results). LAB VR had 36 participants (36 x 3-sites x 2-densities x 8-PAQs = 1728 results).
At the beginning of both experiments, participants signed a written consent form and received an information sheet describing the experiment and its procedure. Given the MCR online also had Brazilian participants, the questionnaires were translated to the Portuguese language. Subjects were divided into two groups to reduce experimental time: ‘Plaza’ and ‘Street’, and ‘Park’ and a bus stop. Recommendations were to use headphones and, when using mobile phones, to turn into a landscape orientation for better performance.
In the LAB VR, tests were done inside a semi-anechoic chamber at the Acoustics Research Centre of the University of Salford, Manchester, UK. Considering that cases of COVID were still occurring (July 2022), an email detailed COVID-free protocol before arriving. Participants sat in the centre of the semi-anechoic chamber, watched a short video explaining the research, answered the general information questions, and conducted a training session. They watched the six audiovisual stimuli through the VIVE HMD with a Beyerdynamic DT 1990 Pro headset as many times as they wished and answered the subjective questions presented on a laptop.
Questionnaires were developed in an online platform. For the MCR online, the questionnaire began with a written consent form. General questions were asked about demographics (gender, age, nationality, and residency), auditory health (evidence of hearing loss, and tinnitus), and digital settings (what audio and video system they used during the experiment). Questions were responded to after watching each video. They were phrased: ‘Please, slide to the word that best describes the sounds you just heard. To the left (-) is NEGATIVE, and to the right (+) is POSITIVE.’ Paired PAQs presented with three synonyms each were ‘unpleasant-pleasant’, ‘uneventful-eventful’, ‘chaotic-calm’, and ‘monotonous-vibrant’ PAQs. Scores ranged from -10 to +10 for negative to positive semantic values of terms through a slider.
In the LAB VR, video and questions were randomly presented. General questions were demographic, auditory health (as in the MCR online), number of languages spoken, education level, and acoustic or music background (no, a little, moderate, and expert level). The experimental questions were formulated: ‘To what extent do you think the sound environment you just experienced was. . . 0 = Not at all, 50 = Neutral, and 100 = Extremely’. The PAQs were presented individually and rated through a slider. The soundscape attributes tested were ‘pleasant’, ‘calm’, ‘uneventful’, ‘monotonous’, ‘annoying’, ‘chaotic’, ‘eventful’, and ‘vibrant’ PAQs separately. In both experiments, there was a final open question to have feedback regarding experiments.
2.4 Statistical analysis
Since data collection had different scales, the MCR online results separated the Paired PAQs, and -10 to +10 ratings inverted to zero (0) to one hundred (100) scores, while the LAB VR maintained as in the original scale. A summary of collected data is presented in Table 1 . Statistical analysis included the Wilcoxon signed-rank test for comparisons of the empty and busy conditions within the same location, and the Mann–Whitney U test for comparing the different locations for the same population density, being both tests within the same experiment. Given comparisons were only between two conditions and data collection was on a continuous scale, a correction for multiple comparisons (Bonferroni) was unnecessary. Significant group differences were tested with the help of the statistical package IBM SPSS Statistics 29.0.1.0 ®.
https://doi.org/10.1371/journal.pone.0306261.t001
3.1 Descriptive analysis of participants
Table 2 presents the demographic information for the MCR online and LAB VR experiments. The MCR online occurred online from August to November 2020. The 155 participants came from 63 countries: 52% from Brazil, 12% from the UK, and 14% from other parts of the world, including Europe, Africa, North and South America, Asia, and the Middle East. In Group 1, 80% used a computer screen and 20% a smartphone to watch the videos, while 76% used headphones and 24% external audio to reproduce audio signals during the experiment. 89% declared they had no hearing loss, and 11% had some hearing loss. 77% mentioned not to have tinnitus, and 23% to have signs of tinnitus [ 45 ]. In Group 2, 86% used a computer screen and 14% a smartphone to watch the videos, while 65% used headphones and 35% external audio to reproduce audio signals during the experiment. 90% declared they had no hearing loss, and 10% had some hearing loss. 81% mentioned not to have tinnitus, and 19% to have signs of tinnitus [ 55 ].
https://doi.org/10.1371/journal.pone.0306261.t002
For the LAB VR, participants originated from 11 countries, with 47% from the United Kingdom, 17% from India, and 36% from other parts of the world including Europe, Africa, South America, and Asia. 97% declared no hearing loss, and 3% mild hearing loss. 83% mentioned not having tinnitus, and 17% heard infrequently or regularly signs of tinnitus.
The MCR online counted 4.3 times more participants (N = 155) compared to the LAB VR (N = 36). In summary, over 50% of Brazilians participated in the MCR online, followed by 12% of British with a predominant age range of 26 to 35 years old (35%) and balanced gender distribution.
3.2 Descriptive analysis of auditory stimuli
The acoustic and psychoacoustic characteristics of the auditory stimuli for each tested scenario are demonstrated in Tables 3 and 4 . For the MCR online, 17 visits from January to December 2019 on days with no precipitation were done at Peel Park, Piccadilly Gardens, and Market Street in empty and busy conditions to collect audio recordings for the online experiment. For the LAB VR, a total of nine visits to execute field recordings were done from December 2020 to July 2021 on days with no precipitation forecast in the empty and busy conditions at Piccadilly Gardens (Plaza), Market Street (Street), and Peel Park (Park).
https://doi.org/10.1371/journal.pone.0306261.t003
Loudness (N), Sharpness (S), Roughness (R), Fluctuation Strength (FS), and Tonality (T).
https://doi.org/10.1371/journal.pone.0306261.t004
As observed in Table 3 , the higher value for 1 min L Aeq on the MCR online was for the ‘Plaza’ busy scenario with 70 dB(A), while the smallest value was observed for the ‘Park’ empty scenario with 46 dB(A). In the LAB VR, the superior value was for the ‘Plaza’ empty with 64.5 dB(A), and the smallest appeared for the ‘Park’ empty scenario with 47.1 dB(A).
Table 4 shows the psychoacoustic metrics of each scenario’s auditory stimuli used for the LAB VR. Greater values are observed at the ‘Plaza’ busy for Loudness (N = 23.01 sone), Sharpness (S = 1.84 acum), and Tonality (T = 0.25 tu); at the ‘Park’ empty for Roughness (R = 0.03 asper); at the ‘Park’ busy for Roughness (R = 0.03 asper) and Tonality (T = 0.25 tu); and at the ‘Street ‘ busy for Roughness (R = 0.03 asper) and Fluctuation Strength (FS = 0.04 vacil). The smallest values are observed at the ‘Street’ empty for Loudnes (N = 10.61 sone), Sharpness (S = 1.31 acum), Roughness (R = 0.02 asper), Fluctuation Strength (FS = 0.02 vacil), and Tonality (T = 0.02 tu). It was also observed the smaller values of Sharpness(S = 1.31 acum) at the ‘Park’ busy, Roughness (R = 0.02 asper), at the ‘Plaza’ busy; Roughness (R = 0.02 asper), and Fluctuation Strength (FS = 0.02 vacil) at the ‘Plaza’ empty.
3.3 Wilcoxon signed-ranks test results for busy versus empty conditions
The Wilcoxon signed-ranks test evaluated how the spaces were rated in busy and empty conditions for each location and the data collection method. Table 5 shows the Wilcoxon signed-ranks test results, which suit two related samples with a non-normal distribution. Values with significant p-values indicate that there are differences between samples. 85.4% (41 PAQs) of results presented significant differences between empty and busy conditions in the studied locations, and 14.6% (7 PAQs) of results had an unexpected similarity. Fig 3 shows a set of boxplots for each studied area and data collection method, where comparing the results in busy and empty conditions is possible. It also represents the significance level of the Wilcoxon signed rank test using * for p-values below 0.05 and ** for p-values inferior to 0.001. In the boxplots, there is a higher distribution in busy conditions on positive qualities such as ‘calm’, ‘eventful’, ‘pleasant’ and ‘vibrant’ in all samples (3a-3f), while in empty conditions, ratings concentrated over the neutral answer. A smaller distribution of negative qualities such as ‘uneventful’ and ‘monotonous’ is also observed.
Columns for ‘Plaza’ (3a & 3d), ‘Park’ (3b & 3e), and ‘Street’ (3c & 3f); and rows for MCR online (3a-3c), and LAB VR (3d-3f). * for significant p-value at < .05, and ** for significant p-value at < .001.
https://doi.org/10.1371/journal.pone.0306261.g003
Where * represents the p-value for 2-tailed significance.
https://doi.org/10.1371/journal.pone.0306261.t005
As observed in Table 5 , the significant results for the MCR Online dataset between busy and empty presented in descending order were as follows: the ‘eventful’ PAQ in the ‘Street’ (Z = -7.16, p<0.001); the ‘vibrant’ PAQ in the ‘Plaza’ (Z = -6.888, p<0.001); the ‘uneventful’ PAQ in the ‘Street’ (Z = -6.647, p<0.001); the ‘calm’ in the ‘Park’ (Z = -6.645, p<0.001); the ‘monotonous’ PAQ in the ‘Street’ (Z = -6.629, p<0.001); the ‘pleasant’ PAQ in the ‘Park’ (Z = -5.791, p<0.001); the ‘chaotic’ PAQ in the ‘Street’ (Z = -4.626, p<0.001); and the ‘annoying’ PAQ in the ‘Plaza’ (Z = -3.685, p<0.001).
As observed in Table 5 , the PAQ with non-significant values on the MCR Online and LAB VR is the quality of ‘annoying’ with a score of zero in all studied areas, except on the MCR Online at the ‘Plaza’. Non-significant level ratings regarding the quality ‘pleasant’ were observed with a score around 50 at the ‘Plaza’ and ‘vibrant’ with a neutral score at the ‘Street’ studied areas. The non-significant p-values from the qualities mentioned above indicate no perceived acoustic differences between the empty and busy conditions.
For the LAB VR dataset, the superior difference between busy and empty were in descending order as follows: the ‘vibrant’ PAQ at the ‘Plaza’ (Z = -4.611, p<0.001); the ‘uneventful’ PAQ at the ‘Street’ (Z = -4.577, p<0.001); the ‘eventful’ PAQ at the ‘Park’ (Z = -4.263, p<0.001); the ‘monotonous’ PAQ at the ‘Street’ (Z = -4.229, p<0.001); the ‘calm’ PAQ at the ‘Park’ (Z = -4.227, p<0.001); the ‘chaotic’ PAQ at the ‘Street’ (Z = -3.99, p<0.001); and the ‘pleasant’ PAQ at the ‘Street’ (Z = -3.359, p<0.05).
3.4 Mann-Whitney U test results for comparison between locations
The Mann-Whitney U test helped compare the same population density condition among different locations in each data collection method. Table 6 shows the results of the Mann-Whitney U test, which suits two independent samples with non-normal distribution. Significant p-values indicate that there are differences between locations. Some PAQs had no differences among locations, meaning no significance with a p-value higher than 0.05. Figs 4 and 5 show the set of boxplots for each studied area comparisons and data collection, where it is possible to compare the results in busy and empty conditions. It also represents the significance level of the Mann-Whitney U tests using * for p-values below 0.05 and ** for p-values inferior to 0.001.
Columns for comparisons of ‘Plaza’ vs. ‘Street’ (4a & 4d), Park’ vs. ‘Street’ (4b & 4e), and ‘Park’ vs. ‘Plaza’ (4c & 4f); and rows for empty (4a-4c), and busy (4d-4f) conditions. * for significant p-value at < .05, and ** for significant p-value at < .001.
https://doi.org/10.1371/journal.pone.0306261.g004
Columns for comparisons of ‘Plaza’ vs. ‘Street’ (5a & 5d), Park’ vs. ‘Street’ (5b & 5e), and ‘Park’ vs. ‘Plaza’ (5c & 5f); and rows for empty (5a-5c), and busy (5d-5f) conditions. * for significant p-value at < .05, and ** for significant p-value at < .001.
https://doi.org/10.1371/journal.pone.0306261.g005
https://doi.org/10.1371/journal.pone.0306261.t006
For MCR online, 64.6% (31 PAQs) of results presented significant differences when comparing different locations, and 35.4% (17 PAQs) had similar results. Fig 4 shows the results from MCR online. It is possible to observe in the comparison of ‘Plaza’ vs. ‘Street’ that in the empty condition, there is a higher dispersion of results on the attribute ‘calm’ ( Fig 4A ). In contrast, in busy conditions, the same dispersion occurs on ‘vibrant’, ‘eventful’, ‘annoying’, ‘chaotic’, and ‘pleasant’ ( Fig 4D ). For the ‘Park’ vs. ‘Street’ comparison, the dispersion of responses in the empty condition happens on the ‘calm’, ‘monotonous’, and ‘uneventful’ attributes ( Fig 4B ), meanwhile, for the busy condition dispersion was on the ‘eventful’, ‘pleasant’, ‘vibrant’, ‘annoying’, and ‘chaotic’ attributes ( Fig 4E ). In the ‘Park’ vs. ‘Plaza’ comparison, the attributes with superior dispersion on the empty condition are ‘calm’, ‘monotonous’, and ‘uneventful’ ( Fig 4C ), while in the busy condition on the ‘eventful’, ‘vibrant’, ‘annoying’, and ‘chaotic’ attributes ( Fig 4F ).
Derived from Table 6 , the significant U values for each location comparison are presented in descending order. In the MCR Online dataset, the greatest differences between population density were as follows: for ‘Street’ vs. ‘Park’ busy, the ‘uneventful’ PAQ (U = 2754.5, p<0.05); for ‘Plaza’ vs. ‘Park’ busy, the ‘chaotic’ PAQ (U = 2471.5, p<0.05); for the same locations in the empty condition, the ‘monotonous’ PAQ (U = 2424.0, p<0.05); in the ‘Plaza’ vs. ‘Street’ busy, the ‘calm’ PAQ (U = 2405.0, p<0.05); and in the ‘Street’ vs. ‘Park’ empty, the ‘eventful’ PAQ (U = 2374.0, p<0.05).
Regarding the non-significant results also presented in Fig 4 for the MCR online, ratings around zero were observed in different PAQs, as follows: ‘uneventful’ in the ‘Plaza’ vs. ‘Street’ ( Fig 4D ), and ‘Park’ vs. ‘Plaza’ ( Fig 4F ) both for the busy condition; ‘eventful’ in the ‘Plaza’ vs. ‘Street’ ( Fig 4A ), and ‘Park’ vs. ‘Plaza’ ( Fig 4C ) both for the empty condition; ‘annoying’ for the ‘Park’ vs. ‘Plaza’ ( Fig 4C and 4F ) in both conditions; ‘calm’ in the ‘Park’ vs. ‘Plaza’ ( Fig 4C ) for the empty condition; and ‘chaotic’ in the ‘Park’ vs. ‘Plaza’ ( Fig 4C ) for the empty condition. Additionally, the ‘eventful’ scale had similar scores of around 50 for the ‘Plaza’ vs. ‘Street’ ( Fig 4D ) in the busy conditions. For the ‘uneventful’ scale, the comparisons of ‘Plaza’ vs. ‘Street’ ( Fig 4A ), and ‘Park’ vs. ‘Plaza’ ( Fig 4C ) in the empty condition had values around 20. The ‘pleasant’ PAQ scores were around 60 and 25 in the ‘Park’ vs. ‘Plaza’ for the empty ( Fig 4C ) and busy ( Fig 4F ) conditions, respectively. The ‘calm’ scores were around 60 in the ‘Park’ vs. ‘Plaza’ Fig 4C ) in the empty condition. For the busy condition, the ‘vibrant’ scores were around 25 in the ‘Park’ vs. ‘Plaza’ ( Fig 4F ).
For the LAB VR, 62.5% (30 PAQs) of results presented significant differences when comparing different locations, and 37.5% (18 PAQs) had similar results. Fig 5 shows the results from the LAB VR. Regarding the ‘Plaza’ vs. ‘Street’ comparison, the dispersion occurs on the attributes ‘calm’, ‘monotonous’, and ‘uneventful’ for the empty condition ( Fig 5A ); and ‘pleasant’, ‘eventful’, ‘vibrant’, ‘annoying’, and ‘chaotic’ on the busy condition ( Fig 5D ). In the ‘Park’ vs. ‘Street’ comparison, the dispersion of results occurs on the attributes ‘calm’, ‘monotonous’, and ‘ uneventful’ in the empty ( Fig 5B ), while ‘vibrant’, ‘chaotic’, and ‘annoying’ in the busy condition ( Fig 5E ). Finally, in the ‘Park’ vs. ‘Plaza’ comparison, the attributes with higher dispersion in the empty condition are ‘calm’, ‘pleasant’, ‘monotonous’, and ‘uneventful’ ( Fig 5C ). In the busy condition ( Fig 5F ), the dispersion was observed in ‘eventful’, ‘vibrant’, ‘annoying’, and ‘chaotic’ scales.
Derived from Table 6 , the significant U values for each location comparison are presented in descending order as follows: the ‘chaotic’ PAQ in the ‘Street’ vs. ‘Park’ empty (U = 563.0, p<0.05); the ‘annoying’ PAQ in the ‘Plaza’ vs. ‘Park’ busy (U = 506.5, p<0.05); the ‘uneventful’ PAQ in the ‘Plaza’ vs. ‘Park’ empty (P = 473.5, p<0.05); the ‘monotonous’ PAQ in the ‘Plaza’ vs. ‘Street’ empty (U = 457.5, p<0.05); the ‘monotonous’ PAQ in the ‘Street’ vs. ‘Park’ busy (U = 365.0, p<0.001); and the ‘calm’ PAQ in the ‘Plaza’ vs. ‘Street’ busy (U = 333.5, p<0.001).
Meanwhile, the non-significant results also noticed in Fig 5 , ratings around zero were observed in different PAQs, as follows: ‘uneventful’ in the ‘Park’ vs. ‘Street’ ( Fig 5E ), and ‘Park’ vs. ‘Plaza’ ( Fig 5F ) both in the busy condition; ‘monotonous’ in the ‘Park’ vs. ‘Plaza’ for both conditions ( Fig 5C and 5F ); ‘chaotic’ in the ‘Plaza’ vs. ‘Street’ empty; and ‘eventful’ in the ‘Plaza’ vs. ‘Park’ empty. Four out of six location comparisons had around zero scores for the ‘annoying’ attribute: the ‘Street’ vs. ‘Park’ empty, the ‘Plaza’ vs. ‘Park’ empty, and the ‘Plaza’ vs. ‘Street’ in both conditions ( Fig 5A and 5D ). Two comparisons scored around 50 for the ‘pleasant’ and ‘eventful’ scales in the ‘Park’ vs. ‘Plaza’ busy ( Fig 5F ). The two comparisons scored around 40 for the ‘calm’ attribute in the ‘Plaza’ vs. ‘Street’ empty ( Fig 5A ), and the ‘pleasant’ scale in the ‘Plaza’ vs. ‘Street’ busy ( Fig 5D ). A score of around 30 appeared for ‘pleasant’ in the ‘Plaza’ vs. ‘Street’ empty ( Fig 5A ). Meanwhile, the ‘uneventful’ score in the ‘Park’ vs. ‘Street’ for the empty condition ( Fig 5B ) was around -50, the ‘vibrant’ scale was around 10, and 60 in the ‘Park’ vs. ‘Plaza’ for the empty ( Fig 5C ), and busy conditions ( Fig 5F ), respectively.
4. Discussion
When verifying the hypothesis (H 01 ) regarding different population densities at the same site and experiment, the Wilcoxon signed-rank test demonstrated that 85% of comparisons were significantly different. The PAQs for ‘calm’, ‘eventful’, ‘pleasant’, ‘chaotic’, ‘monotonous’, and ‘uneventful’ corroborated with the null hypothesis, that is, they changed with the number of people in the scenario ( Fig 3A–3F ). The ‘annoying’ in the ‘Plaza’ for the LAB VR ( Fig 3A ), the ‘vibrant’ of all locations in the MCR online ( Fig 3A–3C ), and the same attribute at the ‘Park’ in the LAB VR ( Fig 3E ) were also significantly different with population densities. When relating to the ‘Plaza’, results corroborate with the strategic urban plan done in 2016 to improve Piccadilly Gardens (‘Plaza’) into a vibrant location [ 56 ]. These similar results may indicate that both experiment methods were equivalent, given recordings, methods, and locations were the same, but in different moments. That is, perceptions of calmness always changed with population density at the ‘Park’ as did perceptions of eventfulness, pleasantness, uneventfulness, chaotic, and monotonous changed at the pedestrian street (‘Street’). This observation points out that these attributes may be sound qualities to consider when studying similar locations.
In the ‘Plaza’, there was a constant water fountain sound. This sound could mask the background traffic noise, which can cause a positive sensation that could justify the same pleasant rating. This masking effect was also observed in the study related to environmental noise [ 57 ]. Similar results related to the ‘pleasant’ and ‘vibrant’ qualities of water features showed that three Naples waterfront sites had no differences among laboratory and online experiments [ 32 ]. This finding corroborates the concept of using water sound as a tool [ 58 , 59 ] to support urban sound management and planning [ 9 , 38 ].
When verifying the hypothesis (H 02 ) regarding differences among urban locations in the same population density and experimental method, the Mann-Whitney test presented 63% and 58% significant differences for the MCR online and the LAB VR, respectively. The ‘calm’ PAQ was significantly different among four comparing sites for the MCR online ( Fig 4A, 4B, 4D and 4E ). Meanwhile, the LAB VR had five comparing sites ( Fig 5B–5F ) which corroborates with the null hypothesis. This tendency indicates that the ‘calm’ soundscape quality may be easier to assess since quiet areas are the opposite of noise pollution. However, there is a misconception of the definition of ‘calm’, which is easily confused with the term ‘quiet’. The ‘calm’ term represents pleasant and harmonic sound sources, while the ‘quiet’ term refers to the absence of sound sources. The calmness is more associated with silence, relaxation, and a tranquil area [ 60 ]. In addition, regarding the empty locations, resemblances among scores may be expected, given early hours may evoke similar perceptions. The tendency of similar results was unexpected for the comparison among the park and plaza ( Fig 4F ), given that different space functionalities may indicate different soundscape ‘characters’ as observed by Bento Coelho [ 38 ] and Siebein [ 53 ].
In both experiments, neutral responses, considered here as values around zero, were observed with 56% for the Wilcoxon signed-ranked test, and 54% and 44% for the Mann-Whitney test at the MCR online and LAB VR, respectively (Figs 3 – 5 ). Such behaviour might be related to neutral emotions which are also common in public opinion polls, because people avoid conflicting issues, especially when indifferent, and not used to the research topic or location [ 61 , 62 ]. Furthermore, neutrality may be because of a lack of familiarity with location due to the absence of retrieved sound memory [ 63 ]. Since semantic memory consists of facts, concepts, data, general information, and knowledge [ 64 ], individuals’ opinions must be grounded in these elements to interpret and rate the sonic environment [ 65 ]. For example, in the Wilcoxon signed-rank test the busy condition, the ‘monotonous’ and ‘uneventful’ scales were around zero in the same compared locations in both methods ( Fig 3 ). Meanwhile, in the Mann-Whiteney test, unexpected similarities were observed in the MCR online within half compared locations for the ‘monotonous’ scale with values over zero ( Fig 4 ). Similar zero scores were observed in the location comparisons for the ‘chaotic’, ‘annoying’, and ‘eventful’ qualities in the ‘Plaza’ vs. ‘Park’ empty in both experimental methods (Figs 4 and 5 ).
Another possibility for the neutrality of responses may be due to the uniformity of soundscapes which gives an impression of ‘blended’ sounds. This sound could be denominated as a ‘blended urban soundscape’, common in big cities due to similar sound sources in different functioning landscapes, also identified by Schafer as a ‘lo-fi’ sound [ 40 ]. When the environment is excessively urbanised, where the population exceeds three million inhabitants, the sonic environment is somehow normalised, so that people do not identify differences among the diverse urban soundscapes. These urban sonic environments are dominant in traffic and human-made sounds, constantly present in the background, and natural sounds have become rare. These noises could cause neurological stress on the population, where they become anesthetised due to overwhelming urban sounds. As Le Van Quyen [ 66 ] recommended, urban citizens should practice a ‘mental detox’, which includes being in a quiet environment. Such a principle reinforces the importance of maintaining and preserving quiet areas. It is also important to notice that these ‘blended soundscapes’ should be avoided when designing urban sound zones, to give character [ 38 , 53 ] and create diversity [ 67 ] within each site.
Another factor may be socio-cultural differences since 50% of participants from the MCR online were Brazilian Portuguese speakers. Some PAQ English words may not represent a common term in the Brazilian Portuguese language, as observed in Antunes et al. [ 68 ]. These inconsistencies in translations were also encountered in participating countries of the SATP group [ 14 ], as observed in the Indonesian study [ 15 ]. Therefore, further investigations should continue to consolidate the English terminology [ 4 ] so that translations can improve. However, even though there was a neutrality of perceived responses, the psychoacoustic indicators for the ‘Plaza’ busy scene showed higher values in loudness, sharpness, and tonality due to the sound source characteristics of the location. The most common sound sources in this location were the water sound from the fountain, children playing and shouting (sharpness, loudness, and tonality), tram circulation and sounds of tram brakes (sharpness and tonality), and babble sounds (loudness) [ 17 , 69 ]. Most psychoacoustic indicators in the other locations and densities presented similar results, corroborating with the characteristics of the ‘blended’ soundscapes.
Limitations of this work consist of audio levels and different smartphone audio reproduction in the online experiment, as well as lack of familiarity with the study areas, ‘social desirability’ in which participants desire to please the researcher [ 70 ], and ‘experimenter effect’ where individuals need to use their critical thinking in a way they never had to do before [ 71 ]. Recommendations are to adjust audio levels to the field sound levels at the beginning of an online experiment [ 72 ]. In the case of smartphone use in the online experiments, it is also recommended to ask the participant to inform the brand of the device to verify the factory calibration of loudspeakers.
5. Conclusions
This work aimed to observe the PAQ results regarding differences among the two population densities for each location, and comparisons among locations for each experimental method. The study highlighted that there were significant results regarding the effect of population density and comparison among locations in the subjective responses. Still, the neutrality of results did not contribute to characterising the soundscape diversity in a megalopolis city. Meanwhile, the second hypothesis verified that the differences among locations within each experimental method demonstrated similar unexpected results. Such behaviour was discussed and could be related to the participants’ unfamiliarity with the location, and homogeneities of the urban sonic environment characterized here as ‘blended urban soundscapes’.
Based on the identified ‘blended soundscapes’, it is highlighted the importance of managing and planning the sonic environment by the clear delimitation of the acoustic zones in line with the functionality of the space. Furthermore, soundscape tools should be investigated to increase the diversity of sound sources, enhancing the sonic environment with elements such as masking, bio-phony, noise reduction, noise barriers, selection of urban materials, and sound art installations, among others.
Future works include evaluating other cities with lower population density to highlight the PAQs to avoid ‘blended’ soundscapes and enrich the sonic environment for VR experiments. Further neurologic evaluations must include more objective metrics in assessing cognitive responses to urban soundscapes and understanding how social-cultural differences are reflected in VR experiments. These VR findings can support urban design in a low-cost approach where urban planners can test different scenarios and interventions.
Supporting information
https://doi.org/10.1371/journal.pone.0306261.s001
Acknowledgments
The authors thank participants and the Acoustic Research Centre staff from the University of Salford, UK for their contributions.
- 1. International Organization for Standardization. ISO/TS 12913–2. Acoustics–Soundscape. Part 2: Methods and measurements in soundscape studies. Geneva, Switzerland. 2018.
- View Article
- Google Scholar
- PubMed/NCBI
- 14. Aletta F, et al. Soundscape assessment: Towards a validated translation of perceptual attributes in different languages. In: Inter-noise and noise-con congress and conference proceedings 2020 Oct 12 (Vol. 261, No. 3, pp. 3137–3146). Institute of Noise Control Engineering.
- 27. Rumsey F. Spatial audio. Routledge; 2012 Sep 10.
- 28. Sun K, Botteldooren D, De Coensel B. Realism and immersion in the reproduction of audio-visual recordings for urban soundscape evaluation. In: INTER-NOISE and NOISE-CON Congress and Conference Proceedings 2018 Dec 18 (Vol. 258, No. 4, pp. 3432–3441). Institute of Noise Control Engineering.
- 38. Coelho JB. Approaches to urban soundscape management, planning, and design. Soundscape and the built environment. Jian Kang & Brigitte Schulte-Fortkamp (editors). CRC Press, 2016: 197–214. Boca Raton, USA. https://doi.org/10.1201/b19145-11
- 40. Schafer RM. The soundscape: Our sonic environment and the tuning of the world. Simon and Schuster; 1993 Oct 1.
- 44. Sanchez GM, Alves S, Botteldooren D. Urban sound planning: an essential component in urbanism and landscape architecture. In: Handbook of research on perception-driven approaches to urban assessment and design 2018 (pp. 1–22). IGI Global.
- 48. De Coensel B, Sun K, Botteldooren D. Urban Soundscapes of the World: Selection and reproduction of urban acoustic environments with soundscape in mind. In: INTER-NOISE and NOISE-CON Congress and Conference Proceedings 2017 Dec 7 (Vol. 255, No. 2, pp. 5407–5413). Institute of Noise Control Engineering.
- 53. Siebein GW. ‘Creating and Designing Soundscape’, in Kang J. et al. (eds) Soundscape of European Cities and Landscapes—COST. 2013, Oxford: Soundscape-COST, pp. 158–162.
- 55. Carvalho ML, Davies WJ, Fazenda B. Manchester Soundscape Experiment Online 2020: an overview. In: INTER-NOISE and NOISE-CON Congress and Conference Proceedings 2023 Feb 1 (Vol. 265, No. 1, pp. 5993–6001). Institute of Noise Control Engineering.
- 63. Engel MS, Carvalho ML, Davies WJ. The influence of memories on soundscape perception responses. In: DAGA 2022 Proceedings. 2022. DAGA Stuttgart, pp. 1–4.
- 72. Sudarsono AS, Sarwono J. The Development of a Web-Based Urban Soundscape Evaluation System. InIOP Conference Series: Earth and Environmental Science 2018 May (Vol. 158, No. 1, p. 012052). IOP Publishing. https://doi.org/10.1088/1755-1315/158/1/012052
An Experiment for the Validation of Force Reconstruction Techniques on Flexible Structures
- Applications paper
- Published: 05 September 2024
Cite this article
- Z. T. Jones 1 &
- N. A. Vlajic 1
Dynamic force measurements are often corrupted by the structural dynamics of the surrounding support structure. Force reconstruction techniques aim to correct for these structural effects by using additional information such as a modal characterization of the structure, a finite element model of the assembly, or additional instrumentation. In practice, accurately measuring input forces to validate the techniques is often difficult or impossible. This work proposes a novel experiment that allows for measurement of the true input spatial force distribution acting on a structure for the purposes of experimentally validating force reconstruction techniques. In the proposed experiment, independently-controlled electromagnets are supported by force gages and used to excite a flexible structure. The reaction force from the electromagnet gives a measure of the applied forces over a given bandwidth, which can be used to validate force reconstruction techniques. This paper focuses on the design of such an experimental arrangement, and presents a numerical model which can also be used to validate force reconstruction techniques. Key components of this experiment are characterized to validate the measurements and methodology. The independently-controlled electromagnets can mimic different types of physical excitation forces, which allow for validation of various force reconstruction techniques aimed at niche applications. For example, the main application of the proposed experiment is to reconstruct unsteady fluid-borne forces generated on a flexible test structure. As such, a sample measurement mimicking forces generated by turbulent flow across a beam using electromagnets is provided.
This is a preview of subscription content, log in via an institution to check access.
Access this article
Subscribe and save.
- Get 10 units per month
- Download Article/Chapter or eBook
- 1 Unit = 1 Article or 1 Chapter
- Cancel anytime
Price includes VAT (Russian Federation)
Instant access to the full article PDF.
Rent this article via DeepDyve
Institutional subscriptions
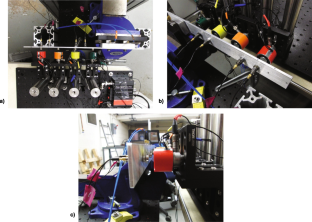
Collopy A, Lee S, Marineau EC (2014) Development of dynamic force measurement capabilities at AEDC tunnel 9. In: 52nd Aerospace sciences meeting. https://doi.org/10.2514/6.2014-0983
Draper JW, Lee S, Marineau EC (2017) Development and implementation of a hybrid dynamic force measurement system at AEDC tunnel 9. https://doi.org/10.2514/6.2017-1593
Bogatirev MM, Bolshakova AA, Gorbushin AR, Kolesnikov AI, Rogozkin PA (2018) Measuring of the dynamic load by use of strain-gauge balance axial component. AIP Conference Proceedings 2027(1)
Burns DE, Vlajic N, Chijioke A, Parker PA (2022) Aerodynamic metrologists guide to dynamic force measurement. J Aircr 59(5):1195–1206. https://doi.org/10.2514/1.c036676
Article Google Scholar
Fu L, Song A (2018) Dynamic characteristics analysis of the six-axis force/torque sensor. Journal of sensors 2018:1–11. https://doi.org/10.1155/2018/6216979
Jayalakshmi V, Lakshmi K, Rao ARM (2018) Dynamic force reconstruction techniques from incomplete measurements. J Vib Control 24(22):5321–5344. https://doi.org/10.1177/1077546317752709
Zheng Z, Wu C, Wang D (2019) A novel method for force identification based on filter and FEM. J Vib Control 25(19–20):2656–2666. https://doi.org/10.1177/1077546319866032
Mendrok K, Dworakowski Z, Dziedziech K, Holak K (2021) Indirect measurement of loading forces with high-speed camera. Sensors (Basel, Switzerland) 21(19):6643
Article PubMed Google Scholar
Liu R, Dobriban E, Hou Z, Qian K (2022) Dynamic load identification for mechanical systems: a review. Archives of Computational Methods in Engineering 29(2):831–863
Sanchez J, Benaroya H (2014) Review of force reconstruction techniques. J Sound Vib 333(14):2999–3018. https://doi.org/10.1016/j.jsv.2014.02.025
Axtell W (2016) Force reconstruction using force gauges and modal analysis. Master’s thesis, The Pennsylvania State University
Logan P, Avitabile P, Dodson J (2019) Reconstruction of external forces beyond measured points using a modal filtering decomposition approach. Exp Tech 44(1):113–125. https://doi.org/10.1007/s40799-019-00340-0
Logan P, Fowler D, Avitabile P, Dodson J (2020) Reconstruction of nonlinear contact forces beyond limited measurement locations using an SVD modal filtering approach. Exp Tech 44(4):485–495. https://doi.org/10.1007/s40799-020-00371-y
Logan PE (2020) Force reconstruction beyond measured points. PhD thesis, University of Massachusetts Lowell
Fowler D, Logan P, Avitabile P (2021) Force reconstruction at mechanical interfaces using a modal filtering decomposition approach. Exp Tech 46(1):115–136. https://doi.org/10.1007/s40799-021-00467-z
Fowler DM (2021) On the use of linear dynamic models with limited measured data to predict nonlinear response. PhD thesis, University of Massachusetts Lowell
El-Bakari A, Khamlichi A, Jacquelin E, Dkiouak R (2014) Assessing impact force localization by using a particle swarm optimization algorithm. J Sound Vib 333(6):1554–1561. https://doi.org/10.1016/j.jsv.2013.11.032
Ghaderi P, Dick AJ, Foley JR, Falbo G (2015) Practical high-fidelity frequency domain force and location identification. Computers & Structures 158:30–41. https://doi.org/10.1016/j.compstruc.2015.05.028
Aucejo M, De Smet O (2016) Bayesian source identification using local priors. Mech Syst Signal Process 66–67:120–136. https://doi.org/10.1016/j.ymssp.2015.05.004
Aucejo M, De Smet O (2017) A multiplicative regularization for force reconstruction. Mech Syst Signal Process 85:730–745. https://doi.org/10.1016/j.ymssp.2016.09.011
Aucejo M, De Smet O (2018) A space-frequency multiplicative regularization for force reconstruction problems. Mech Syst Signal Process 104:1–18. https://doi.org/10.1016/j.ymssp.2017.10.027
Miao B, Zhou F, Jiang C, Chen X, Yang S et al (2018) A comparative study of regularization method in structure load identification. Shock and Vibration 2018
Perotin L, Granger S (1999) An inverse method for the identification of a distributed random excitation acting on a vibrating structure part 2: flow-induced vibration application. Mech Syst Signal Process 13(1):67–81. https://doi.org/10.1006/mssp.1998.0200
Granger S, Perotin L (1999) An inverse method for the identification of a distributed random excitation acting on a vibrating structure part 1: Theory. Mech Syst Signal Process 13(1):67–81. https://doi.org/10.1006/mssp.1998.0200
Lysak PD (2011) Unsteady lift of thick airfoils in incompressible turbulent flow. PhD thesis, The Pennsylvania State University
Bucher I, Rosenstein M (2005) Determination of external forces-application to the calibration of an electromagnetic actuator. J Vib Acoust 128(5):545–554. https://doi.org/10.1115/1.2346699 . https://asmedigitalcollection.asme.org/vibrationacoustics/articlepdf/128/5/545/5649592/545_1.pdf
Jonson ML, Young SD (1995) Decomposition of structural and hydrodynamic contributions for unsteady rotor thrust. In: Proceedings of the 1995 ASME international mechanical engineering congress and exposition
Anderson JM, Catlett MR, Forest J, Joiner J, Kaler Z, Manar F. Determining Unsteady Aerodynamic Lift due to Turbulent Flow about Elastic Airfoils with Thick, Wavy Leading Edges. https://doi.org/10.2514/6.2019-2527
Lysak PD, Capone DE, Jonson ML (2016) Measurement of the unsteady lift of thick airfoils in incompressible turbulent flow. J Fluids Struct 66:315–330. https://doi.org/10.1016/j.jfluidstructs.2016.07.018
Smith DR, Gildfind DE, Mee DJ, James CM, Allsop BV (2020) Magnetohydrodynamic drag force measurements in an expansion tunnel using a stress wave force balance. Exp Fluids 61:1–15
Griffiths DJ (2013) Introduction to Electrodynamics, 4th edn. Pearson, Boston
Google Scholar
Peeters B, Auweraer HV, Guillaume P, Leuridan J (2004) The PolyMAX frequency-domain method: a new standard for modal parameter estimation? Shock Vib 11(3–4):395–409. https://doi.org/10.1155/2004/523692
Lyons RG (1997) Understanding digital signal processing. Personal Education
Download references
Acknowledgements
COMSOL modeling for this research was performed on The Pennsylvania State University’s Institute for Computational and Data Sciences’ Roar supercomputer.
Author information
Authors and affiliations.
The Graduate Program in Acoustics, Applied Research Laboratory, The Pennsylvania State University, University Park, PA, USA, 16802
Z. T. Jones & N. A. Vlajic
You can also search for this author in PubMed Google Scholar
Corresponding authors
Correspondence to Z. T. Jones or N. A. Vlajic .
Additional information
Publisher's note.
Springer Nature remains neutral with regard to jurisdictional claims in published maps and institutional affiliations.
Z.T. Jones and N.A. Vlajic are members of SEM.
Rights and permissions
Springer Nature or its licensor (e.g. a society or other partner) holds exclusive rights to this article under a publishing agreement with the author(s) or other rightsholder(s); author self-archiving of the accepted manuscript version of this article is solely governed by the terms of such publishing agreement and applicable law.
Reprints and permissions
About this article
Jones, Z.T., Vlajic, N.A. An Experiment for the Validation of Force Reconstruction Techniques on Flexible Structures. Exp Tech (2024). https://doi.org/10.1007/s40799-024-00738-5
Download citation
Received : 24 February 2024
Accepted : 18 July 2024
Published : 05 September 2024
DOI : https://doi.org/10.1007/s40799-024-00738-5
Share this article
Anyone you share the following link with will be able to read this content:
Sorry, a shareable link is not currently available for this article.
Provided by the Springer Nature SharedIt content-sharing initiative
- Force reconstruction
- Flexible structures
- Measurement
- Find a journal
- Publish with us
- Track your research

IMAGES
VIDEO
COMMENTS
The first step in reading your A/B test results is looking at your goal metric, which is usually conversion rate. After you've plugged your results into your A/B testing calculator, you'll get two results for each version you're testing. You'll also get a significant result for each of your variations. 2.
To design a robust experiment, it is highly recommended to decide metrics for invariant checking. These metrics shouldn't change between control and experiment groups and can be used for sanity checking. What is important in an A/B test is to define the sample size for the experiment and control groups that represent the overall population.
3.2 Power for the test of the difference between two sample means This type of estimation can be used in cases where the target of a test is a continuous variable. In our example, it will be the ...
A/B testing is a way to compare two versions of something to figure out which performs better. While it's most often associated with websites and apps, the method is almost 100 years old and it ...
This process takes you from the one-set solution you started with to test against the control, to a range of about 10 solutions and variations that can help you bring positive results. In an hour of work, you increase your chances to create a winning experiment significantly. Now you have your solutions, we're almost ready to start the ...
A/B testing is one of the most popular controlled experiments used to optimize web marketing strategies. It allows decision makers to choose the best design for a website by looking at the analytics results obtained with two possible alternatives A and B. In this article we'll see how different statistical methods can be used to make A/B ...
3. Use a Representative Sample Size. Having a representative sample size is another critical component of successful A/B testing. It's the key to obtaining reliable and statistically significant results. In A/B testing, your sample size refers to the number of users who are exposed to each version of your test.
A/B testing, or split testing, is a quantitative user research method. In A/B testing, researchers show different users two versions of the same design to identify which one performs better. The A refers to the original design, while the B refers to the variation of the A design. A/B testing has applications in many fields, like marketing ...
4 most popular A/B testing and product experimentation tools 1. Google Optimize. Google Optimize is a free platform for website experimentation, specifically A/B testing, multivariate testing, and redirect testing. Powered by advanced targeting options, it allows you to test multiple changes on a single page, compare their performance, and measure the results against a company objective.
What Is A/B Testing? A/B testing is a quantitative research method that tests two or more design variations with a live audience to determine which variation performs best according to a predetermined set of business-success metrics.. In an A/B test, you create two or more variations of a design in a live product. Most commonly, you'll compare the original design A, also called the control ...
A/B testing (also known as bucket testing, split-run testing, or split testing) is a user experience research method. [1] A/B tests consist of a randomized experiment that usually involves two variants (A and B), [2][3][4] although the concept can be also extended to multiple variants of the same variable.
Running an A/B test involves several steps: 1. Experiment Prerequisites: Before running experiments, we need to define key metrics to measure the goal of an experiment. We also need to ensure that ...
What A/B testing won't show you. An A/B test compares the performance of two items or variations against one another. On its own, A/B testing only shows you which variation is winning, not why. To design better experiments and impactful website changes, you need to understand the deeper motivations behind user behavior and avoid ineffective solutions like guesswork, chance, or stakeholder ...
A/B testing is a popular method of experimentation in the fields of digital marketing and web design. For example, a marketer looking to increase e-commerce sales may run an experiment to determine whether the location of the "buy now" button on the product page impacts a particular product's number of sales.
Now that you've got the basics down of A/B testing, here's how you get started. Step 1. Specify the problem to solve. Your testing success hinges on how well you've defined and documented the problem you're solving. A well-defined problem statement creates a launchpad for ideation and generating hypotheses.
To help you plan your AB tests, we've designed a free template in a spreadsheet format. This guide should provide you with: A list of ideas to test on your website. A tool to help you prioritize your experiments using the ICE score. A calculator to estimate how long you should run your tests. A template for documenting your experiments.
This involves implementing the changes you're testing and collecting quantitative data. 4. Analyzing the results. Once the experiment is complete, it's time to analyze the A/B test results. This involves looking at the data you've collected and determining whether your testing hypothesis was supported or not. 5.
An A/B/n test splits traffic equally among a control and multiple page variations. A/B/n tests are great for implementing more variations of the same hypothesis, but they require more traffic because they split it among more pages. , are just one type of online experiment. You can also run.
AB testing (AKA "split testing") is the process of directing your traffic to two or more variations of a web page. AB testing is pretty simple to understand: A typical AB test uses AB testing software to divide traffic. Our testing software is the "Moses" that splits our traffic for us.
A/B Testing. Course. This course will cover the design and analysis of A/B tests, which are online experiments used throughout tech industry by companies like Google, Amazon, and Netflix. Enroll Now. Last Updated March 7, 2022. Prerequisites:
Challenge #6: Changing experiment settings in the middle of an A/B test. When you launch an experiment, you must commit to it completely. Try and not to change your experiment settings, edit or omit your test goals, or play with the design of the control or the variation while the test is running.
A/B testing steps. The article will provide a step-by-step walkthrough on all steps required for ab testing. Designing the experimentation. Dunning the experimentation. Getting data. Rnterpreting the results. Using results to decision-making and creating impact.
As in any other statistical anlaysis, important steps of an A/B testing experiment include, post the question (s) you want to answer using data. design the experiment to address your question (s) identify appropriate methodologies to analyze the data. run the experiment to collect data. analyze the data according to the experimental design and ...
Laboratory impact experiments have proven critical to enable analyses of ground-based radar and optical observations with on-orbit events and measurements. Existing Department of Defense and NASA satellite breakup models are based on a previous laboratory test, conducted in the 1990s, which has supported many applications and matched on-orbit ...
The study of the perceived affective qualities (PAQs) in soundscape assessments have increased in recent years, with methods varying from in-situ to laboratory. Through technological advances, virtual reality (VR) has facilitated evaluations of multiple locations in the same experiment. In this paper, VR reproductions of different urban sites were presented in an online and laboratory ...
Dynamic force measurements are often corrupted by the structural dynamics of the surrounding support structure. Force reconstruction techniques aim to correct for these structural effects by using additional information such as a modal characterization of the structure, a finite element model of the assembly, or additional instrumentation. In practice, accurately measuring input forces to ...